Hosting Applications on Google Cloud
Google Cloud offers a wide range of options for application hosting. Take a look at the charts below to find out which one is right for your needs.
Use cases
- Flask
- Django
- Express.js
- Symfony
- Spring Boot
- Post a comment on Slack channel following a GitHub commit
- Statistical analysis
- Image thumbnail generation
- Designed for event-driven workloads
- Scales to meet sudden demand
- Minimal configuration
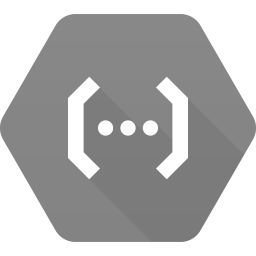
- Software written in any languages using specific system packages
- Off-the-shelf software provided as container images
- Supports industry-standard Docker containers
- Scales your containerized app automatically
- Custom binaries and system dependencies
- Supports API endpoints
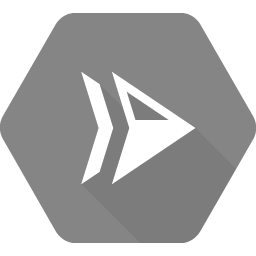
- Relational databases, SAP HANA
- CRM systems
- Legacy ERP systems
- Operating system-level control
- Supports custom machine types
- Autoscaling support
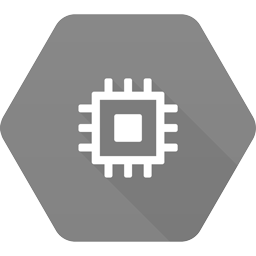
Key terms
Deployment format
How is your code packaged upon deployment to a given platform?
- VM images - snapshots of an entire filesystem, including the underlying OS.
- Containers - portable cross-platform filesystems isolated from the underlying OS.
- Sources - one service contained in a directory with one or more use cases.
- Functions - individual code snippets each corresponding to a single use case.
Custom URLs
Can you create multi-layer URL paths (such as /abc/def
) and/or use your own
domain name?
Persistent disks
Can disk state be saved when an instance shuts down, or must long-term data be stored outside the instance?
Scale-to-zero
Can this product scale down to zero instances and avoid billing me for periods of zero requests?
WebSockets
Does this product support the WebSocket protocol?
Run any language
Can this product run code in arbitrary programming languages?
Request timeout
What is the maximum amount of time the platform will wait for a response from the application's code?
Background processes
Can this product run ongoing background operations outside a request period?
Use GPUs
Does this product support hardware acceleration for GPU-optimized workloads?
VPC connectivity
Can this product access resources within a Virtual Private Cloud?