简介
此新手指南是 AutoML 的简介。如需了解 AutoML 和自定义训练之间的主要区别,请参阅选择训练方法。
假设:
- 您是足球队的教练。
- 您在一家数字零售商的营销部门工作。
- 您要处理可识别建筑类型的建筑项目。
- 您的公司在网站上设置了一张联系表单。
手动处理视频、图片、文本和表格的工作既繁琐又耗时。教机器自动识别和标记内容不是会更加简单吗?
图片
您正在与建筑保护委员会合作,尝试发现您所在城市里建筑风格一致的街区。有数十万张的房屋快照需要仔细筛查。然而,在尝试手动分类所有这些图片时,这将是一项单调乏味且容易出错的工作。几个月前,一名实习生为其中的几百张图片添加了标签,但其他人都没有查看过数据。如果您可以教计算机来代您完成这项审核工作,一定会非常有用!
表格
您在一家数字零售商的营销部门工作。您和您的团队正在根据客户角色创建个性化的电子邮件程序。您已经创建了角色和营销电子邮件。现在,您必须创建这样一个系统,即使是新客户,也可以根据零售偏好和消费行为将其划分为相应角色。为了最大限度地提高客户互动度,您还需要预测其消费习惯,以便优化发送电子邮件时间。
因为您是数字零售商,所以您拥有有关客户及其购买的数据。但新客户呢?传统方法能够为具有较长消费历史的现有客户计算这些值,但面对历史数据很少的客户时则表现不佳。如果能创建一个系统来预测这些值,加快对所有客户开展个性化营销的速度该有多好?
幸运的是,机器学习和 Vertex AI 可以很好地解决这些问题。
文本
您的公司在网站上设置了一张联系表单。公司每天都会通过该表单收到大量消息,其中很多建议在某方面都是具有可操作性的。因为它们全部一起涌入,就很容易造成处理不及时。而不同的消息类型由不同的员工进行处理。
如果能有一个自动化系统对它们进行分类,让处理人员看到由自己负责的评论,那就太好了。
您需要利用某种系统来查看评论,然后确定它们是投诉还是对过去服务的赞扬,是想要详细了解您的业务、请求安排预约还是想要建立关系。
视频
假设您有一个想用于分析的庞大的游戏视频库。但要查看的视频达数百个小时。您需要观看每个视频并手动标记片段以突显每个动作,这既枯燥又耗时。而每个赛季都需要您重复这项工作。现在,假设计算机模型能够在视频中出现时自动识别并标记这些操作。
以下是一些与目标相关的具体场景。
- 操作识别:查找对目标进行评分、导致违规、进行处罚等操作。有助于教练研究团队的优势和弱势。
- 分类:将每个视频分类为半场、游戏视图、观众视图或教练视图。这可帮助教授仅浏览感兴趣的视频片段。
- 对象跟踪:跟踪足球或球员。可协助教练获得球员的统计数据,例如现场热图、成功通过率。
本指南将引导您逐步了解如何将 Vertex AI 用于 AutoML 数据集和模型,并说明 Vertex AI 旨在解决的问题类型。
有关公平性的说明
Google 致力于在遵循负责任的 AI 做法方面取得进展。 为此,我们的机器学习产品(包括 AutoML)围绕公平性和以人为本的机器学习等核心原则进行设计。如需详细了解在构建自己的机器学习系统时消除偏见的最佳实践,请参阅包容性机器学习指南 - AutoML。
为什么说 Vertex AI 是最适合解决这类问题的工具?
传统编程要求程序员指定分步说明,以使计算机按照这些说明操作。但我们来设想一下识别足球比赛中特定动作的用例。颜色、角度、分辨率和光线千变万化,需要编写大量规则来告知机器如何做出正确决策。甚至难以想象应该从哪里开始入手。客户评论使用的词汇和结构各种各样,变化多端,以致于无法通过一套简单的规则来捕获如果您尝试构建手动过滤器,很快就会发现,您无法对大多数客户评论进行分类。您需要一个可以通用于各种评论的系统。在一系列特定规则必然会以指数级扩展的使用场景下,您需要一个能够通过样本学习的系统。
幸运的是,机器学习应该能够解决这些问题。
Vertex AI 的工作原理是什么?
Vertex AI 涉及监管式学习任务来实现想要的结果。算法和训练方法的具体细节因数据类型和用例而异。机器学习有许多不同的子类别,所有子类别均用于解决不同的问题,并具有不同的限制条件。
图片
您可以使用示例图像训练,测试和验证机器学习模型,这些示例图像带有用于分类的标签,或者带有标签和用于对象检测的边界框。通过监督式学习,您可以训练模型来识别图片中您需要关注的模式和内容。
表格
您使用示例数据训练机器学习模型。Vertex AI 使用表格(结构化)数据来训练机器学习模型,以便对新数据进行预测。数据集中的一列(称为目标)是您的模型将学习预测的内容。其他一些数据列是模型将从中学习模式的输入(称为特征)。只需更改目标列和训练选项,即可使用同一些输入特征构建多种模型。在电子邮件营销的示例中,这意味着您可以利用相同的输入特征但不同的目标预测结果来构建模型。一个模型可以预测客户的角色(分类目标),另一个模型可以预测客户的每月支出(数值目标),再一个模型可以预测未来 3 个月产品的每日需求(多个数值目标)。
文本
Vertex AI 允许您执行监督式学习。这涉及训练计算机来识别已加标签的数据的模式。通过监督式学习,您可以训练 AutoML 模型来识别文本中您关注的内容。
视频
您可以使用已标记的视频来训练、测试和验证机器学习模型。通过经过训练的模型,您可以向模型输入新视频,然后输出带有标签的视频片段。视频片段可定义视频中的开始时间和结束时间偏移量。片段可以是整个视频、用户定义的时间细分、自动检测的视频片段,或只是开始时间与结束时间相同的时间戳。标签是通过模型预测的“答案”。例如,在上述足球用例中,为每个新足球视频,根据模型类型执行以下操作:
- 经过训练的操作识别模型会输出视频时间偏移值,并采用描述“射门”“个人犯规”等动作镜头的标签。
- 经过训练的分类模型自动输出检测到的带有用户定义标签(例如“游戏视图”“观众视图”)的镜头细分。
- 经过训练的对象跟踪模型通过对象出现在屏幕内的边界框来输出足球或玩家的轨迹。
Vertex AI 工作流
Vertex AI 使用标准机器学习工作流:
- 收集数据:根据您想要实现的结果,确定训练和测试模型所需的数据。
- 准备数据:确保您的数据格式正确且已加标签。
- 训练:设置参数并构建模型。
- 评估:审核模型指标。
- 部署和预测:使您的模型可供使用
但是,在开始收集数据之前,您需要思考您要尝试解决的问题,从而了解您的数据要求。
数据准备
评估您的用例
从您的问题着手:您想要实现什么结果?
图片
在整合数据集时,应始终以用例为基础。您可以从以下问题入手:
- 您想要实现什么样的结果?
- 为了实现这个结果,您需要识别哪些种类的类别或对象?
- 人类能否识别这些类别?尽管 Vertex AI 可以处理比人类在任何时候都无法记住和分配的类别更大的类别,但是如果人类无法识别特定类别,那么 Vertex AI 也会遇到困难。
- 哪些种类的样本最能反映您的系统将看到并尝试分类的数据类型和范围?
表格
目标列是什么类型的数据?您可以访问多少数据? 根据您的答案,Vertex AI 会创建必要的模型来解析您的用例:
- 二元分类模型可预测二元结果(二者选一)。 该模型适合用于是非问题,例如预测客户是否会购买订阅。在其他条件相同的情况下,二元分类问题所需的数据比其他模型类型少。
- 多类别分类模型可从三个或更多个互不关联的类别中预测一个类别,可用于对事物进行分类。对于零售示例,您需要构建一个多类别分类模型,以将客户细分为不同的角色。
- 预测模型可预测一系列值。例如,作为零售商,您可能希望预测未来 3 个月的产品每日需求,以便提前备妥适当数量的商品库存。
- 回归模型可预测连续值。对于零售示例,您需要构建一个回归模型来预测客户下个月的支出。
文本
在整合数据集时,应始终以用例为基础。您可以从以下问题入手:
- 您想要实现什么结果?
- 为了实现这个结果,您需要识别哪些种类的类别?
- 人类能否识别这些类别?虽然 Vertex AI 可以处理的类别比人类在任何时候都无法记住和分配的类别要多,但是如果人类无法识别特定类别,那么 Vertex AI 也会遇到困难。
- 哪些种类的样本最能反映您的系统要分类的数据类型和范围?
视频
根据您想要实现的结果,选择合适的模型目标:
- 要检测视频中的动作时刻,例如识别进球得分、导致违规或进行罚球,请使用操作识别目标。
- 如需将电视节目镜头分成以下类别(商业、新闻、电视节目等),请使用分类目标。
- 要定位和跟踪视频中的对象,请使用对象跟踪目标。
如需详细了解为操作识别、分类和对象跟踪目标准备数据集的最佳做法,请参阅准备视频数据。
收集数据
建立用例后,您需要收集用于创建所需模型的数据。
图片
确定了所需的数据后,您必须找到一种方法来获取数据。首先,您可以考虑使用组织收集的所有数据。您可能会发现自己已经在收集训练模型所需的相关数据。如果您没有该数据,可以手动获取或将其外包给第三方提供商。
在每个类别中添加足够的加标签样本
Vertex AI 所需的最低要求是每个类别/标签分类 100 个图片示例。每个标签的高质量样本数量越多,成功识别标签的可能性就越大;一般而言,您带入训练过程的已加标签数据越多,模型的表现就会越出众。每个标签至少有 1000 个样本。
在所有类别中平均分配样本
每个类别获取数量大致相同的训练样本非常重要。即使某一个标签有大量的数据,最好还是为每个标签分配数量大致相等的数据。为了说明原因,我们假设您用于构建模型的图片中有 80% 都是现代风格的单户式住宅照片。由于标签的分配如此不均衡,您的模型很可能会发现,总是将照片预测为现代风格的单户式住宅非常安全,而尝试预测它是一个不那么常见的标签则会有风险。这就像是编写一份单选题试卷,其中几乎所有正确答案都是“C”;在这种情况下,聪明的应试者很快就会发现,每次都可以回答“C”,甚至都不需要看题。
我们理解,并不总是可以为每个标签找到数量大致相同的样本。某些类别的高质量、无偏见样本可能更难获取。在这些情况下,您可以遵循一个经验法则,即样本数量最少的标签所具有的样本数应至少达到样本数最多的标签的 10%,因此,如果最大的标签有 10000 个样本,则最小的标签应至少有 1000 个样本。
捕获问题空间中的各种变化
出于类似的原因,请尝试确保您的数据能够捕获问题空间的变化和多样性。模型在训练过程中看到的选择项越广泛,就越容易泛化新的样本。例如,如果您尝试将消费类电子产品的照片进行分类,则模型在训练中接触到的各种消费类电子产品越多,它就越有可能区分出新型平板电脑、手机或笔记本电脑,即使之前从未见过这种特定型号也是如此。
将数据与模型的预期输出相匹配
您所搜集的图片在视觉上应与您计划进行预测的图片类似。如果您正在尝试对在冬季多雪天气条件下拍摄的房屋图片进行分类,而您的模型仅使用了在阳光明媚天气条件下拍摄的房屋图片进行训练,那么您可能无法从中获得出色的结果,即使您已经使用自己所需的类别标记了这些图片也是如此,因为光线和背景的差异可能足以影响模型的表现。理想情况下,您的训练样本是从您计划使用模型进行分类的数据集中提取的真实数据。
表格
确定用例后,您需要收集数据来训练模型。数据搜寻和准备是构建机器学习模型的关键步骤。可用的数据会决定您能够解决什么类型的问题。您有多少可用数据?您的数据是否与您要回答的问题相关?在收集数据时,请牢记以下关键注意事项。
选择相关特征
特征是用于模型训练的输入属性。特征帮助模型识别模式以进行预测,因此它们需要与您的问题相关。例如,要构建一个预测信用卡交易是否属于欺诈的模型,您需要构建一个包含交易详细信息(如买方、卖方、金额、日期和时间以及购买的商品)的数据集。其他有用的特征包括买方和卖方的相关历史信息,以及购买的商品涉及欺诈的频率。还有哪些可能相关的其他特征?
请考虑简介中提到的零售商电子邮件营销用例。以下是您可能需要的一些特征列:
- 购买的商品清单(包括品牌、类别、价格、折扣)
- 购买的商品数量(前一天、过去一周、过去一个月、过去一年)
- 消费金额(前一天、过去一周、过去一个月、过去一年)
- 每件商品每天的售出总数
- 每件商品每天的库存总数
- 是否针对特定日期开展促销活动
- 已知的购物者统计学特征概况
包括足够的数据
一般而言,您拥有的训练样本越多,得到的结果就越好。所需的样本数据量也会随着您试图解决的问题的复杂性而变化。与多类别模型相比,生成一个精确的二元分类模型所需的数据更少,因为从两个类别中预测一个比从多个类别中预测一个来得简单。
完美的公式是不存在的,但我们建议至少具有以下示例数据:
- 分类问题:50 行 x 特征数量
- 预测问题:
- 5000 行 x 特征数
- 时序标识符列中的 10 个唯一值 x 特征数量
- 回归问题:200 x 特征数量
捕获差异
您的数据集应捕获问题空间的多样性。模型在训练过程中看到的样本越多样,就越容易对新的或较不常见的样本具备普适性。设想一下,如果您仅使用冬季的购买数据来训练零售模型,那么模型是否能够成功预测夏季服装偏好或购买行为?
文本
确定了所需的数据后,您需要找到一种方法来获取数据。首先,您可以考虑使用组织收集的所有数据。此时您可能会发现,自己已经在收集训练模型所需的数据了。如果没有收集到所需的数据,您可以手动获取或将这项任务外包给第三方提供商。
在每个类别中添加足够的加标签样本
每个标签的高质量样本数量越多,成功识别该标签的可能性越大;一般而言,您为训练过程提供的加标签数据越多,您的模型就越出众。所需的样本数量也会随着您想要预测的数据的一致性程度以及您的目标准确度而变化。您可以使用更少的示例来获得一致的数据集,或者达到 80%的准确度,而不是 97%的准确度。训练模型,然后评估结果。添加更多示例并进行重新培训,直到达到准确性目标为止,每个标签可能需要数百甚至数千个示例。如需详细了解数据要求和建议,请参阅准备 AutoML 模型的文本训练数据。
在所有类别中平均分配样本
每个类别都获取数量大致相似的训练样本非常重要。 即使某一个标签有大量的数据,最好还是为每个标签分配数量大致相等的数据。为了说明原因,我们假设您用于构建模型的客户评论中有 80% 都是预估请求。由于标签的分配如此不均衡,您的模型很可能会发现,总是将客户评论预测为预估请求非常安全,而尝试预测它是一个不那么常见的标签则会有风险。这就像是编写一份单选题试卷,其中几乎所有正确答案都是“C”;在这种情况下,聪明的应试者很快就会发现,每次都可以回答“C”,甚至都不需要看题。
因此,您有可能无法为每个标签找到数量大致相同的样本。某些类别的高质量、无偏见样本可能更难获取。在这些情况下,样本数量最少的标签所具有的样本数应至少达到样本数最多的标签的 10%。因此,如果最大的标签有 10000 个样本,则最小的标签应至少有 1000 个样本。
捕获问题空间中的各种变化
出于类似的原因,请尝试让数据能够捕获问题空间的变化和多样性。当您提供一组范围更广的样本时,模型将能更好地泛化到新数据。假设您正尝试将有关消费电子产品的文章分入不同的主题。您提供的品牌名称和技术规格越多,模型就越容易找出文章应该归入的主题,即使该文章是介绍根本没有收入训练集的品牌也是如此。为了进一步提高模型性能,您还可以考虑为不符合任何已定义标签的文档添加“none_of_the_above”标签。
将数据与模型的预期输出相匹配
寻找的文本样本应与您计划进行预测的文本样本类似。如果您正在尝试对关于玻璃吹制术的社交媒体帖子进行分类,那么在玻璃吹制术信息网站上训练的模型可能无法有出色的表现,因为两者的词汇和风格可能不相同。理想情况下,您的训练样本是从您计划使用模型进行分类的数据集中提取的真实数据。
视频
确定用例后,您将需要收集用于创建所需模型的视频数据。您收集的用于训练的数据说明了您可以解决的问题种类。您可以使用多少个视频?对于您希望模型预测的类别,视频是否包含足够多的样本?在收集视频数据时,请牢记以下注意事项。
包括足够多的视频
一般而言,数据集中的训练视频越多,结果就越好。建议的视频数量也随着您尝试解决的问题的复杂度变化而增加或减少。例如,与多标签问题(根据许多类别预测一个或多个类别)相比,二元分类问题(根据两个类别预测一个类别)所需的视频数据较少。
您尝试执行的操作的复杂程度也决定了您需要多少视频数据。考虑用于分类的足球用例,该用例正在建立一个区分动作镜头的模型,还训练一个能够对不同游泳风格进行分类的模型。例如,为了区分蛙泳、蝶泳、仰泳等,您需要更多的训练数据来识别不同的游泳风格,以帮助模型学习如何准确地识别每种类型。如需获取关于操作识别、分类和对象跟踪的最少视频数据的指导,请参阅准备视频数据。
所需的视频数据量可能比您目前拥有的视频数据要多。您可以考虑通过第三方提供商获取更多视频。例如,如果您的游戏操作标识符模型没有足够多的视频,您可以购买或获取更多蜂鸟视频。
在类别之间平均分配视频
尽量为每个类别提供数量差不多的训练样本。原因如下:假设您 80% 的训练数据集是关于射门镜头的足球视频,但只有 20% 的视频显示了个人犯规或罚点球。由于类别的分配如此不均衡,您的模型更有可能会预测给定动作是射门。这就像是编写了一份单选题试卷,其中 80% 的正确答案都是“C”:聪明的模型很快就会发现,在大多数时候,猜测“C”的正确率相当高。
您有可能无法为每个类别都找到相同数量的视频。对于某些类别而言,高质量、无偏见的样本可能也很难获取。请尽量遵循 1:10 的比率:如果最大的类别有 10000 个视频,则最小的类别应至少有 1000 个视频。
捕获差异
您的视频数据应捕获问题空间的多样性。模型在训练过程中看到的样本越多样,就越容易对新的或较不常见的样本具备普适性。以足球动作分类模型为例:务必提供从各个取镜角度、分别在白天和晚上拍摄的包含球员各种动作的视频。为模型提供多样性数据可以提高模型区分不同动作的能力。
将数据与预期输出相匹配

您需要找到在视觉上与您计划输入到模型中进行预测的视频相似的训练视频。例如,如果您的所有训练视频都拍摄于冬季或晚上,这些环境下的光线和颜色模式将影响您的模型。如果您之后使用该模型来测试在夏季或白天拍摄的视频,获得的预测结果可能不是很准确。
您还需要考虑以下额外因素:视频分辨率、每秒视频帧数、取镜角度、背景。
准备数据
图片
确定适合您的方式(手动或默认拆分方式)后,您可以使用以下方法之一在 Vertex AI 中添加数据:
表格
在确定可用数据后,您需要确保数据适合用于训练。如果您的数据存在偏差或包含缺失或错误的值,这会影响模型的质量。在开始训练模型之前,请注意以下事项。
了解详情。
防止数据泄露和训练-应用偏差
数据泄露是指您在训练期间使用的输入特征“泄露”了试图预测的目标的相关信息,而这类信息在应用模型时无从获得。要检测是否存在数据泄露,您可检测是否有与目标列高度相关的特征被包括为输入特征之一。例如,如果您正在构建一个模型来预测客户是否将在下个月注册订阅,而其中一个输入特征是该客户的未来订阅付款情况。这会使模型在测试期间展现出超高的性能,但部署到生产环境中后却黯然失色,因为在应用模型时无法获得未来的订阅付款信息。
训练-应用偏差是指训练时使用的输入特征与在应用模型时提供给模型的输入特征不同,导致模型在生产环境中效果不佳。例如,建立一个模型来预测每小时的温度,但训练时使用的数据只包含每周的温度。另一个例子:对于预测学生辍学的模型,在训练数据中始终提供学生的分数,但在应用模型时却不提供此信息。
了解您的训练数据对于防止数据泄露和训练-应用偏差非常重要:
- 在使用任何数据之前,请确保您知道数据的含义以及是否应将其用作特征
- 在“训练”标签页中检查相关性。应将高相关性数据标记出来,进行检查。
- 训练-应用偏差:确保仅为模型提供在应用模型时以完全相同的形式提供的输入特征。
清理缺失、不完整和不一致的数据
样本数据中有缺失和不准确的值是很常见的。在将数据用于训练之前,请花些时间进行检查,并尽可能提高数据质量。缺失值越多,您的数据对训练机器学习模型的效用就越小。
- 请检查您的数据是否存在缺失值。如果可能,请更正这些值;如果列设置为可以为 Null,则将这些值保留为空。Vertex AI 可以处理缺失值,但如果所有值都可用,您更有可能获得最佳结果。
- 对于预测,请检查训练行之间的时间间隔是否一致。Vertex AI 可以处理缺失值,但如果所有行都可用,您更有可能获得最佳结果。
- 通过更正或删除数据错误或干扰数据来清理数据。使数据保持一致:检查拼写、缩写和格式设置。
分析导入的数据
导入数据集后,Vertex AI 可以提供您的数据集的概览。检查导入的数据集以确保每一列都具有正确的变量类型。 Vertex AI 将根据列值自动检测变量类型,但您最好逐个检查。您还应该检查每列是否可为 Null,这一属性决定了列是否可以包含缺失值或 NULL 值。
文本
确定适合您的方式(手动或默认拆分方式)后,您可以使用以下方法之一在 Vertex AI 中添加数据:
视频
收集好要添加到数据集中的视频后,您需要确保视频包含与视频片段或边界框关联的标签。对于动作识别,视频片段是一个时间戳,对于分类,视频片段可以是视频镜头、片段或整个视频。对于对象跟踪、标签则是与边界框相关联。
我的视频为什么需要边界框和标签?
对于对象跟踪,Vertex AI 模型如何学习识别模式?这就是边界框和标签在训练过程中的作用。以足球为例:每个示例视频都需要在您希望检测的对象周围包含边界框。此外,需要为这些边界框分配“人”和“球”等标签。否则,模型不知道该查找什么。 为样本视频绘制边界框和分配标签可能需要花费一些时间才能完成。
如果您的数据尚未添加标签,您还可以上传未加标签的视频并使用 Google Cloud 控制台应用边界框和标签。 如需了解详情,请参阅使用 Google Cloud 控制台为数据加标签。
训练模型
图片
考虑 Vertex AI 在创建自定义模型时如何使用您的数据集
数据集包含训练集、验证集和测试集。如果您未指定分组(请参阅准备数据),Vertex AI 会自动将 80% 图片用于训练,10% 图片用于验证,另外 10% 图片用于测试。
训练集
您的绝大部分数据都应该划分到训练集中。训练集包含您的模型在训练期间“看到”的数据:它用于学习模型的参数,即神经网络节点之间连接的权重。
验证集
验证集(有时也称为“开发集”)也用于训练过程。模型学习框架在训练过程的每次迭代期间吸纳了训练数据之后,会根据模型在验证集上的表现来调整模型的超参数,这些超参数是指定模型结构的变量。如果您尝试使用训练集来调整超参数,则很可能会导致模型过度关注训练数据,因而很难泛化到并非精确匹配的样本。使用在一定程度上的新数据集微调模型结构,意味着您的模型将会有更好的泛化效果。
测试集
测试集完全不参与训练过程。当模型完成全部训练后,我们会利用测试集来对模型提出全新的挑战。通过模型在测试集上的表现,您可以较好地了解模型在使用真实数据时会有怎样的表现。
手动拆分
您也可以自行拆分数据集。如果您想要对流程施加更多控制,或者您确定要在模型训练生命周期的某个阶段添加特定的样本,则手动拆分数据是个不错的选择。
表格
导入数据集后,下一步是训练模型。Vertex AI 使用默认设置也能生成可靠的机器学习模型,但建议您根据自己的用例调整一些参数。
选择尽可能多的特征列来进行训练,但要检查每列以确保其适合训练。在选择特征时,请牢记以下事项:
- 不要选择会产生干扰数据的特征列,例如每行具有唯一值的随机分配的标识符列。
- 确保您理解每个特征列及其值。
- 如果要利用一个数据集创建多个模型,请移除不属于当前预测问题的目标列。
- 回想一下公平原则:您训练模型使用的特征是否会导致对边缘群体的偏见或不公平决策?
Vertex AI 如何使用您的数据集
您的数据集将被拆分为训练集、验证集和测试集。默认拆分 Vertex AI 应用取决于您要训练的模型类型。您还可以根据需要指定拆分(手动拆分)。如需了解详情,请参阅 AutoML 模型的数据拆分简介。
训练集
您的绝大部分数据都应该划分到训练集中。训练集包含您的模型在训练期间“看到”的数据:它用于学习模型的参数,即神经网络节点之间连接的权重。
验证集
验证集(有时也称为“开发集”)也用于训练过程。模型学习框架在训练过程的每次迭代期间吸纳了训练数据之后,会根据模型在验证集上的表现来调整模型的超参数,这些超参数是指定模型结构的变量。如果您尝试使用训练集来调整超参数,则很可能会导致模型过度关注训练数据,因而很难泛化到并非精确匹配的样本。使用在一定程度上的新数据集微调模型结构,意味着您的模型将会有更好的泛化效果。
测试集
测试集完全不参与训练过程。当模型完成全部训练后,Vertex AI 会将测试集用作一项新挑战来测试模型。通过模型在测试集上的表现,您可以较好地了解模型在使用真实数据时会有怎样的表现。
文本
考虑 Vertex AI 如何使用您的数据集创建自定义模型
数据集包含训练集、验证集和测试集。如果您未指定拆分方式(如准备数据中所述),那么 Vertex AI 会自动将内容文档的 80% 用于训练,10% 用于验证,另外 10% 用于测试。
训练集
您的绝大部分数据都应该划分到训练集中。训练集包含您的模型在训练期间“看到”的数据:它用于学习模型的参数,即神经网络节点之间连接的权重。
验证集
验证集(有时也称为“开发集”)也用于训练过程。模型学习框架在训练过程的每次迭代期间吸纳了训练数据之后,会根据模型在验证集上的表现来调整模型的超参数,这些超参数是指定模型结构的变量。如果您尝试使用训练集来调整超参数,则很可能会导致模型过度关注训练数据,因而很难泛化到并非精确匹配的样本。使用在一定程度上的新数据集微调模型结构,意味着您的模型将会有更好的泛化效果。
测试集
测试集完全不参与训练过程。当模型完成全部训练后,我们会利用测试集来对模型提出全新的挑战。通过模型在测试集上的表现,您可以较好地了解模型在使用真实数据时会有怎样的表现。
手动拆分
您也可以自行拆分数据集。如果您想要对流程施加更多控制,或者您确定要在模型训练生命周期的某个阶段添加特定的样本,则手动拆分数据是个不错的选择。
视频
训练视频数据准备好后,您就可以创建机器学习模型了。请注意,您可以为同一数据集中的不同模型目标创建注释集。请参阅创建注释集。
Vertex AI 的优势之一是,默认参数将指导您使用可靠的机器学习模型。不过,您可能需要根据您的数据质量和所需的结果来调整参数。例如:
- 预测类型是视频处理的细化程度
- 如果您尝试分类的标签对运动变化敏感,则帧速率会非常重要,如动作识别中所示。例如,执行奔跑与步行。较低的每秒帧数 (FPS) 步行 剪辑看起来就像是奔跑一样。对于对象跟踪,它对于帧速率也很敏感。基本上,所跟踪的对象需要在相邻帧之间具有足够的重叠性。
- 用于对象跟踪的解决方法比用于操作识别或视频分类的解决方案更重要。当对象较小时,请务必上传较高分辨率的视频。当前流水线使用 256x256 进行常规训练,或者如果用户数据中的对象数量太多(且其区域面积不到 1%)则为 512x512。建议使用至少 256p 的视频。使用更高分辨率的视频可能无助于改进模型性能,因为视频帧在内部被降采样以提高训练和推断速度。
评估、测试和部署模型
评估模型
映像
模型训练完成后,您将收到模型性能的总结。点击评估或查看完整评估可以查看详细分析。
调试模型主要是调试数据,而非模型本身。如果在推送到生产环境前后,您的模型在您评估其性能时开始以非预期的方式运行,您应返回并检查数据,看看有哪些方面可以改进。
我可以在 Vertex AI 中执行哪些类型的分析?
在 Vertex AI 的评估部分,您可以根据自定义模型基于测试样本的输出以及常见的机器学习指标来评估其性能。在本部分中,我们将介绍下面每个概念的含义。
- 模型输出
- 分数阈值
- 真正例、真负例、假正例和假负例
- 精确率和召回率
- 精确率/召回率曲线
- 平均精确率
如何解读模型的输出?
Vertex AI 会从您的测试数据中提取样本,对模型提出全新的挑战。模型会针对每个样本输出一系列数字,用于表示每个标签与该样本相关的程度。如果该数字较大,表示关于该标签应该应用于该文档的模型置信度较高。
什么是分数阈值?
我们可以通过设置分数阈值将这些概率转换为二进制的“开”/“关”值。分数阈值是指若要将某一类别分配给某一测试项,模型必须具有的置信度水平。Google Cloud 控制台中的分数阈值滑块是一种直观的工具,用于测试不同阈值对数据集中的所有类别和个别类别的影响。如果分数阈值较低,那么您的模型需要分类的图片会较多,但在该过程中,它对一些图片的分类可能会出错。如果分数阈值较高,那么您的模型需要分类的图片会较少,但出现图片分类错误的风险较低。您可以在 Google Cloud 控制台中调整每个类别的阈值以进行实验。但是,在生产环境中使用模型时,您必须实施您认为最佳的阈值。
什么是真正例、真负例、假正例和假负例?
应用分数阈值后,模型所做的预测可分为以下四类:
您认为最佳的阈值。
我们可以使用这些类别来计算精确率和召回率,这两个指标可帮助我们衡量模型的效果。
什么是精确率和召回率?
精确率和召回率有助于我们了解模型捕获信息的精准度以及遗漏的信息量。通过精确率指标我们可以了解到,在分配了某标签的所有测试样本中,实际有多少样本应该分类到该标签中。通过召回率指标可以了解到,在本应分配该标签的所有测试样本中,有多少样本实际分配了该标签。
我应该针对精确率还是召回率进行优化?
根据您的用例,您可能需要针对精确率或召回率进行优化。 在确定哪种方法最适合您时,请考虑以下两个用例。
使用场景:图片含有隐私信息
假设您要创建一个自动检测敏感信息并对该信息进行模糊处理的系统。
在此使用场景中,那些本不需要模糊处理、却被如此处理的内容属于假正例,它们可能会让您感到有些烦恼,但并不会带来负面影响。
在此使用场景中,那些需要模糊处理、却未能进行此项处理的内容(如信用卡)属于假负例,它们可能会导致身份盗用。
在这种情况下,您需要针对召回率进行优化。该指标衡量所有的预测中遗漏了多少信息。高召回率的模型可能会向略微相关的样本分配标签,这对于类别缺少训练数据的情况非常有用。
使用场景:搜索图库照片
假设您想要创建一个用于查找与给定关键字最为匹配的图库照片的系统。
此使用场景中的假正例返回不相关的图片。由于您的产品是以仅返回最佳匹配图片为目标,因此这是一个完全失败的响应。
此使用场景中的假负例无法返回与搜索关键字相关的图片。由于许多搜索字词都会有数以千计的最佳匹配照片,因此这没什么问题。
在这种情况下,您需要针对精确率进行优化。该指标衡量所进行的所有预测的正确程度。高精确率的模型可能只会为最相关的样本添加标签,这对于类别在训练数据中很常见的情况非常有用。
如何使用混淆矩阵?
如何解读精确率/召回率曲线?
通过分数阈值工具,您可以研究所选分数阈值会如何影响精确度和召回率。当您拖动分数阈值栏上的滑块时,您可以看到该阈值在精确率与召回率权衡曲线上的对应位置,以及该阈值对您的精确率和召回率分别有何影响(对于多类别模型,这些图表上的精确率和召回率意味着用于计算精确率和召回率指标的唯一标签是我们返回的标签集中得分最高的标签)。这可以帮助您在假正例和假负例之间取得较好的平衡。
在选择了对于整个模型而言似乎可接受的阈值后,可点击各个标签,并查看该阈值在各个标签的精确率与召回率曲线上处于的位置。在某些情况下,这可能意味着针对一些标签进行的很多预测都不正确,这可能有助于您决定为每个类别选择针对这些标签定制的阈值。例如,假设您查看了房屋数据集,并注意到如果阈值设为 0.5,“都铎风格”以外的其他所有图片类型都会有合理的精确度和召回率,这可能是因为“都铎风格”类别过于普通。就该类别而言,您会看到大量的假正例。在这种情况下,当您调用分类模型进行预测时,您可能决定仅为"都铎风格"使用阈值 0.8。
什么是平均精确率?
精确率/召回率曲线下的面积是衡量模型准确率的有用指标。 它可以衡量模型在所有分数阈值上的表现。在 Vertex AI 中,此指标称为“平均精确率”。此分数越接近 1.0,您的模型在测试集上的表现就越好;如果是随机猜测使用哪个标签的模型,平均精确率约为 0.5。
表格
模型训练结束后,您将收到其性能总结。模型评估指标根据模型针对数据集切片(测试数据集)的表现情况得出。在确定模型是否已准备好用于真实数据时,需要考虑一些关键指标和概念。
分类指标
得分阈值
考虑一个用于预测客户明年是否会购买夹克的机器学习模型。模型的确定程度需要有多高,才能做出给定客户将购买夹克的预测?在分类模型中,每个预测都分配有一个置信度分数,这个数值衡量模型在多大程度上确定预测的类别是正确的。分数阈值是确定给定分数何时转换为是或否决策的数字;也就是说,分数为该值时,您的模型表示“是的,这个置信度分数足够高,可以得出这个客户将在明年购买一件外套的结论”。
如果分数阈值较低,那么您的模型的分类可能会出错。 因此,分数阈值应基于给定用例确定。
预测结果
应用分数阈值后,模型所做的预测可分为四类。要理解这些类别,我们再次假设一个夹克的二元分类模型。 在这个例子中,正类别(模型尝试预测的内容)是客户“会”在明年购买夹克。
- 真正例:模型对正类别的预测正确。模型正确预测到顾客购买了夹克。
- 假正例:模型对正类别的预测错误。模型预测客户会购买夹克,但他们没有。
- 真负例:模型对负类别的预测正确。模型正确预测到客户没有购买夹克。
- 假负例:模型对负类别的预测错误。模型预测客户不会购买夹克,但他们买了。
精确率和召回率
精确率和召回率指标有助于您了解模型捕获信息的精准度以及遗漏的信息量。详细了解精确率和召回率。
- 精确率是指正确预测的正类别所占的比例。 在客户购买的所有预测中,实际购买的比例占多少?
- 召回率是模型正确预测的具有此标签的行所占的比例。在本可以识别的所有客户购买中,实际识别出的比例占多少?
根据您的用例,您可能需要针对精确率或召回率进行优化。
其他分类指标
- AUC PR:精确率-召回率 (PR) 曲线下的面积。此值的范围在 0 到 1 之间,值越大表示模型质量越高。
- AUC ROC:接收者操作特征 (ROC) 曲线下的面积。 此值的范围在 0 到 1 之间,值越大表示模型质量越高。
- 准确率:模型生成的正确分类预测所占的比例。
- 对数损失函数:模型预测与目标值之间的交叉熵。此值的范围在零到无穷大之间,值越小表示模型质量越高。
- F1 得分:精确率和召回率的调和平均数。F1 是一个很实用的指标,当您希望在精确率和召回率之间取得平衡,而类别分布又不均匀时,该指标会非常有帮助。
预测和回归指标
模型构建完成后,Vertex AI 会提供各种标准回归指标供您查看。关于如何评估模型,并不存在完美的答案;您应该根据您的问题类型以及希望使用模型实现的目标来考虑评估指标。以下列表简要介绍了 Vertex AI 可以提供的一些指标。
平均绝对误差 (MAE)
MAE 指的是目标值与预测值之间的平均绝对差。 它衡量的是一组预测中误差的平均大小,即目标值与预测值之间的差值。由于 MAE 使用的是绝对值,因此它不考虑关系的方向,也不能说明是性能不佳还是性能过高。评估 MAE 时,值越小表示模型质量越高(0 表示完美的预测模型)。
均方根误差 (RMSE)
RMSE 指的是目标值和预测值之间的平均平方差的平方根。RMSE 对离群值比 MAE 更敏感,因此如果您担心大的误差,那么 RMSE 可能是一个更有用的评估指标。与 MAE 类似,值越小表示模型质量越高(0 表示完美的预测模型)。
均方根对数误差 (RMSLE)
RMSLE 进行对数转换后的 RMSE。RMSLE 对相对误差比对绝对误差更敏感,并且更关心性能不佳而不是性能过高的情况。
观察到的分位数(仅限预测)
对于给定的目标分位数,观察到的分位数显示观察值低于指定的分位数预测值的实际比例。观察到的分位数显示模型与目标分位数的差距或接近程度。两个值之间的差值越小,表示模型质量越高。
弹力 Pinball 损失(仅限预测)
衡量给定目标分位数的模型质量。数字越小表示模型质量越高。您可以比较不同分位数上的已调整的 pinball 损失指标,以确定模型在这些不同分位数之间的相对准确率。
文本
模型训练完成后,您将收到模型性能总结。如需查看详细分析,请点击评估或查看完整评估。
在评估模型前,需要注意哪些事项?
调试模型主要是调试数据,而非模型本身。如果在推送到生产环境前后,模型在您评估其性能时有出乎意料的表现,则您应返回并检查数据,以查找能够改进的地方。
我可以在 Vertex AI 中执行哪些类型的分析?
在 Vertex AI 的评估部分,您可以根据自定义模型基于测试样本的输出以及常见的机器学习指标来评估其性能。本节将介绍以下每个概念的含义:
- 模型输出
- 分数阈值
- 真正例、真负例、假正例和假负例
- 精确率和召回率
- 精确率/召回率曲线。
- 平均精确率
如何解读模型的输出?
Vertex AI 会从您的测试数据中提取样本,对模型提出全新的挑战。模型会针对每个样本输出一系列数字,用于表示每个标签与该样本相关的程度。如果该数字较大,表示关于该标签应该应用于该文档的模型置信度较高。
什么是分数阈值?
通过分数阈值,Vertex AI 将概率转换为二进制的“开”/“关”值。分数阈值是指若要将某一类别分配给某一测试项,模型必须具有的置信度水平。控制台中的分数阈值滑块是一种直观的工具,用于测试不同阈值对数据集的影响。在上面的示例中,如果我们将所有类别的分数阈值设置为 0.8,则会分配标签“Great Service”(优质服务)和“Suggestion”(建议),但不会分配“Info Request”(信息请求)。如果分数阈值很低,您的模型需要分类的文本项就会较多,但在该过程中对更多文本项的分类就有可能出错。如果分数阈值很高,您的模型需要分类的文本项会较少,但是将文本项错误分类的风险会较低。您可以在 Google Cloud 控制台中调整每个类别的阈值以进行实验。但是,在生产环境中使用模型时,您必须实施您认为最佳的阈值。
什么是真正例、真负例、假正例和假负例?
应用分数阈值后,模型所做的预测可分为以下四类。
您可以使用这些类别来计算精确率和召回率,这两个指标可帮助我们衡量模型的效果。
什么是精确率和召回率?
精确率和召回率有助于我们了解模型捕获信息的精准度以及遗漏的信息量。通过精确率指标我们可以了解到,在分配了某标签的所有测试样本中,实际有多少样本应该分类到该标签中。通过召回率指标可以了解到,在本应分配该标签的所有测试样本中,有多少样本实际分配了该标签。
我应该针对精确率还是召回率进行优化?
根据您的用例,您可能需要针对精确率或召回率进行优化。 在确定哪种方法最适合您时,请考虑以下两个用例。
用例:紧急文档
假设您要创建一个可以优先处理紧急文档的系统。
在该用例中,如果一个并不紧急的文档被系统标记为紧急文档,这种情况即为假正例。用户可以将其作为非紧急文档忽略并继续后续步骤。
在该用例中,如果一个紧急文档并未被系统标记为紧急文档,这种情况即为假负例。这可能会引起问题!
在这种情况下,您可能需要针对召回率进行优化。该指标衡量所有的预测中遗漏了多少信息。高召回率的模型可能会向略微相关的样本分配标签,这对于类别缺少训练数据的情况非常有用。
用例:垃圾邮件过滤
假设您要创建一个自动过滤垃圾邮件的系统。
在此用例中,垃圾邮件未被捕获,从而出现在收件箱中,这就是一个假负例。这通常只是有点儿烦人。
如果一封电子邮件被错误地标记为垃圾邮件而清除出收件箱,即为本用例中的假正例。如果电子邮件至关重要,则用户可能会受到严重影响。
在这种情况下,您可能希望优化精确率。 该指标衡量所进行的所有预测的正确程度。高精确率模型可能仅向最相关的样本分配标签,这对于类别在训练数据中很常见的用例非常有用。
如何使用混淆矩阵?
我们可以使用混淆矩阵比较模型在每个标签上的表现。 在理想模型中,对角线上的所有值都将较高,而其他所有值都将较低。这表明目标类别都得到了正确识别。如果有其他任何值较高,我们可以由此了解模型如何对测试图片进行了错误分类。
如何解读精确率/召回率曲线?
通过分数阈值工具,您可以研究所选分数阈值会如何影响您的精确度和召回率。当您拖动分数阈值栏上的滑块时,您可以看到该阈值在精确率与召回率权衡曲线上的对应位置,以及该阈值对您的精确率和召回率分别有何影响(对于多类别模型,这些图表上的精确率和召回率意味着用于计算精确率和召回率指标的唯一标签是我们返回的标签集中得分最高的标签)。这可以帮助您在假正例和假负例之间取得较好的平衡。
在选择了对于整个模型而言似乎可接受的阈值后,您就可以点击各个标签,并查看该阈值在各个标签的精确率与召回率曲线上处于什么位置。在某些情况下,这可能意味着针对一些标签进行的很多预测都不正确,这可能有助于您决定为每个类别选择针对这些标签定制的阈值。例如,假设您查看了客户评论数据集,并注意到如果阈值设为 0.5,“Suggestion”(建议)以外的其他所有评论类型都会有合理的精确率和召回率,这可能是因为“Suggestion”(建议)类别过于普通。就该类别而言,您会看到大量的假正例。在这种情况下,当您调用分类模型进行预测时,您可能决定只有"Suggestion"(建议)使用阈值 0.8。
什么是平均精确率?
精确率/召回率曲线下的面积是衡量模型准确率的有用指标。 它可以衡量模型在所有分数阈值上的表现。在 Vertex AI 中,此指标称为“平均精确率”。此分数越接近 1.0,您的模型在测试集上的表现就越好;如果是随机猜测使用哪个标签的模型,平均精确率约为 0.5。
视频
模型训练结束后,您将收到其性能总结。模型评估指标根据模型针对数据集切片(验证数据集)的表现情况得出。在确定模型是否已准备好在新数据中使用时,需要考虑一些关键指标和概念。
得分阈值
机器学习模型如何知道足球射门在什么情况下是真正的射门?每个预测分配有一个置信度分数,即模型确定给定视频片段包含某个类别的数值评估结果。分数阈值是确定给定分数何时转换为是或否决策的数字;也就是说,分数为该值时,您的模型表示“是,这个置信度数字足够高,可以得出视频片段包含射门的结论。”
如果分数阈值较低,那么您的模型对视频片段加的标签可能有误。因此,分数阈值应基于给定用例确定。想象一下,在癌症检测这样的医疗用例中,加错标签的后果要比加错体育视频标签的后果更加严重。在癌症检测中,应使用较高的分数阈值。
预测结果
应用分数阈值后,模型所做的预测可分为四类。为了理解这些类别,假设您构建了一个模型,用于检测给定片段是否包含足球射门(或不包含)。在此示例中,射门是正类别(模型尝试预测的内容)。
- 真正例:模型对正类别的预测正确。模型正确预测到视频片段中有射门。
- 假正例:模型对正类别的预测错误。模型预测到片段中有射门,但其实没有。
- 真负例:模型对负类别的预测正确。模型正确预测到片段中没有射门。
- 假负例:模型对负类别的预测错误。模型预测到片段中没有射门,但其实有。
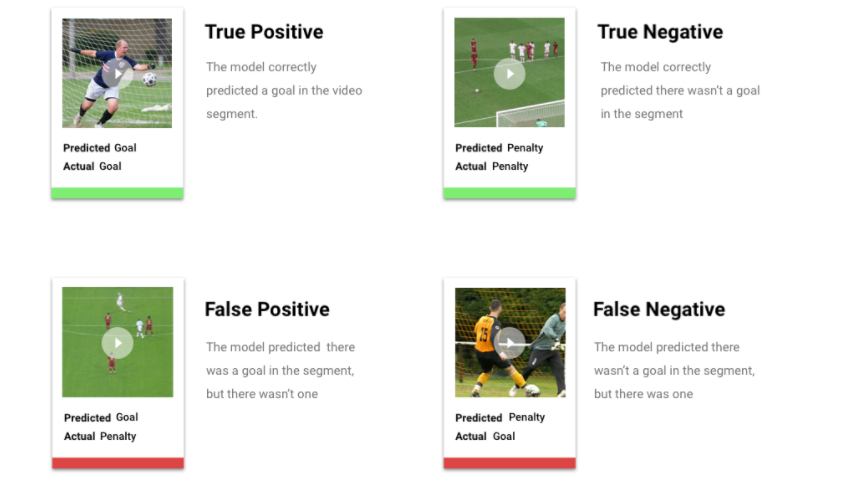
精确率和召回率
精确率和召回率指标有助于您了解模型捕获信息的精准度以及遗漏的信息量。详细了解精确率和召回率。
- 精确率是指正确预测的正类别所占的比例。 在所有标为“射门”的预测中,实际包含射门的比例占多少?
- 召回率是指实际识别出的所有正类别预测所占的比例。在本能识别出的所有足球射门中,实际识别出的比例占多少?
根据您的用例,您可能需要针对精确率或召回率进行优化。请参考以下用例。
用例:视频中的隐私信息
假设您正在构建一个软件,该软件用于自动检测视频中的敏感信息并对该信息进行模糊处理。假结果产生的影响可能包括:
- 假正例识别出不需要屏蔽的信息,但仍然将其屏蔽。这种情况可能会让人感到很烦,但并不会造成有害结果。
- 假负例未能识别出需要屏蔽的信息,如信用卡号。隐私信息将会被公之于众,这是最糟糕的情况。
在该用例中,必须要优化召回率以确保模型可找到所有相关用例。已针对召回率进行优化的模型更可能会向略微相关的样本分配标签,但是向错误样本分配标签的可能性也会提高(进行了模糊处理的信息多于需要模糊处理的信息)。
用例:库存视频搜索
假设您想要创建一个软件,让用户能够根据关键字搜索视频库。请考虑以下错误结果:
- 假正例返回不相关的视频。由于您的系统试图仅提供相关视频,因此您的软件并没有按照其预期构建目的工作。
- 假负例没有返回相关视频。由于许多关键字都对应着数百个视频,因此这个问题的影响并没有返回不相关视频那么糟糕。
在此示例中,您将需要优化精确率,以确保模型提供高度相关的正确结果。高精确率模型可能仅向最相关的样本分配标签,但可能会遗漏一些样本。详细了解模型评估指标。
测试模型
图片
Vertex AI 自动使用 10% 的数据(如果您选择自行拆分数据,则为您选择使用的任何百分比)来测试模型,通过“评估”页面,您可以了解模型在该测试数据上的表现。但是,如果您想对模型进行置信度检查,有几种方法可以使用。最简单的方法是在“部署和测试”页面上上传几张图片,然后查看模型为您的样本选择的标签。希望这符合您的期望。请针对您希望收到的每种图片尝试一些样本。
如果您想在自己的自动化测试中使用模型,“部署和测试”页面还会告诉您如何以编程方式调用模型。
表格
评估模型指标是判断模型是否已准备好部署的主要方法,但您也可以使用新数据对其进行测试。上传新数据以查看模型的预测结果是否符合您的预期。根据评估指标或使用新数据进行测试的结果,您可能需要继续改进模型的性能。
文本
Vertex AI 自动使用 10% 的数据(如果您选择自行拆分数据,则为您选择使用的任何百分比)来测试模型,通过评估页面,您可以了解模型在该测试数据上的表现。 但是,如果您想对模型进行检查,有几种方法可以使用。部署模型后,您可以在部署和测试页面上的字段中输入文本样本,并查看模型为您的示例选择的标签。希望这符合您的期望。请针对您希望收到的每种评论尝试一些样本。
如果您想在自己的自动化测试中使用模型,部署和测试页面会提供示例 API 请求,向您展示了如何以编程方式调用模型。
如果您想在自己的自动化测试中使用模型,部署和测试页面会提供示例 API 请求,向您展示了如何以编程方式调用模型。
在批量预测页面上,您可以创建批量预测,以便将多个预测请求打包到一个中。批量预测是异步的,也就是说,模型会先处理所有预测请求,然后再返回结果。
视频
Vertex AI 视频会自动使用 20% 的数据,如果您选择自行拆分数据,则选择使用任意百分比的数据来测试模型。控制台中的“评估”标签页显示了该模型在该测试数据上的表现。但是,如果您想对模型进行检查,有几种方法可以使用。一种方法是在“测试和使用”标签页中提供带有视频数据的 CSV 文件进行测试,然后查看模型为视频预测的标签。希望这符合您的期望。
您可以调整预测可视化的阈值,并在 3 个时间尺度上查看预测结果:1 秒间隔、自动镜头边界检测后的视频相机镜头、整个视频片段。
部署模型
图片
如果您对模型的性能感到满意,就可以使用该模型了。可能是生产规模的使用,也可能是一次性的预测请求。您可以根据用例的情况以不同方式使用模型。
批量预测
批量预测适合一次处理大量预测请求的情况。批量预测是异步的,也就是说,模型会先处理所有预测请求,然后再返回含有预测值的 JSON 行文件。
在线预测
部署模型使其可用于通过 REST API 处理预测请求。 在线预测是同步的(实时),这意味着它将快速返回预测,但每次 API 调用仅接受一个预测请求。如果您的模型是应用的一部分并且您系统的某些部分依赖于快速的预测周转,则在线预测会非常有用。
表格
如果您对模型的性能感到满意,就可以使用该模型了。可能是生产规模的使用,也可能是一次性的预测请求。您可以根据用例的情况以不同方式使用模型。
批量预测
批量预测适合一次处理大量预测请求的情况。批量预测是异步的,也就是说,模型会先处理所有预测请求,然后再返回具有预测值的 CSV 文件或 BigQuery 表格。
在线预测
部署模型使其可用于通过 REST API 处理预测请求。 在线预测是同步的(实时),这意味着它将快速返回预测,但每次 API 调用仅接受一个预测请求。如果您的模型是应用的一部分并且您系统的某些部分依赖于快速的预测周转,则在线预测会非常有用。
视频
如果您对模型的性能感到满意,就可以使用该模型了。 Vertex AI 使用批量预测,您可以在 CSV 文件中包含托管于 Cloud Storage 上的视频的路径,并将该文件上传到模型中执行预测。您的模型将处理每个视频,并在另一个 CSV 文件中输出预测结果。批量预测是异步的,也就是说,模型会先处理所有预测请求,然后再输出结果。
清理
为避免产生不必要的费用,请在模型未使用时将其取消部署。
使用完模型后,请删除您创建的资源,以避免账号产生不必要的费用。