Introduction
This beginner's guide is an introduction to AutoML. To understand key differences between AutoML and custom training see Choosing a training method.
Imagine:
- You're a coach on a soccer team.
- You're in the marketing department for a digital retailer.
- You're working on an architectural project that is identifying types of buildings.
- Your business has a contact form on its website.
The work of manually curating videos, images, texts, and tables is tedious and time consuming. Wouldn't it be easier to teach a computer to automatically identify and flag the content?
Image
You work with an architectural preservation board that's attempting to identify
neighborhoods that have a consistent architectural style in your city. You have hundreds of
thousands of snapshots of homes to sift through. However, it's tedious and error-prone when
trying to categorize all these images by hand. An intern labeled a few hundred of them a
few months ago, but nobody else has looked at the data. It'd be so useful if you could just teach
your computer to do this review for you!
Tabular
You work in the marketing department for a digital retailer. You and your team are
creating a personalized email program based on customer personas. You've created the personas
and the marketing emails are ready to go. Now you must create a system that buckets customers
into each persona based on retail preferences and spending behavior, even when they're new
customers. To maximize customer engagement, you also want to predict their spending habits so you
can optimize when to send them the emails.
Because you're a digital retailer, you have data on your customers and the purchases they've made. But what about new customers? Traditional approaches can calculate these values for existing customers with long purchase histories, but don't do well with customers with little historical data. What if you could create a system to predict these values and increase the speed at which you deliver personalized marketing programs to all your customers?
Fortunately, machine learning and Vertex AI is well positioned to solve these problems.
Text
Your business has a contact form on its website. Every day you get many messages from
the form, many of which are actionable in some way. Because they all come in together, it's
easy to fall behind on dealing with them. Different employees handle different message types.
It would be great if an automated system could categorize them so that the right person sees
the right comments.
You need a system to look at the comments and decide whether they represent complaints, praise for past service, involve an attempt to learn more about your business, a request to schedule an appointment, or is an attempt to establish a relationship.
Video
You have a large video library of games that you'd like to use to analyze. But there are hundreds of hours of video to review. The work of watching each video and manually marking the segments to highlight each action is tedious and time-consuming. And you need to repeat this work each season. Now imagine a computer model that can automatically identify and flag these actions for you whenever they appear in a video.
Here are some objective specific scenarios.
- Action Recognition: Find actions such as scoring a goal, causing a foul, making a
penalty kick. Useful to coaches for studying their team's strengths and weaknesses.
- Classification: Classify each video shot as either half time, game view, audience view, or coach view. Useful to coaches for browsing only the video shots of interest.
- Object tracking: Track the soccer ball or the players. Useful to coaches for getting players' statistics such as heatmap in the field, successful pass rate.
This guide walks you through how Vertex AI works for AutoML datasets and models, and illustrates the kinds of problems Vertex AI is designed to solve.
A note about fairness
Google is committed to making progress in following responsible AI practices. To achieve this, our ML products, including AutoML, are designed around core principles such as fairness and human-centered machine learning. For more information about best practices for mitigating bias when building your own ML system, see Inclusive ML guide - AutoML
Why is Vertex AI the right tool for this problem?
Classical programming requires the programmer to specify step-by-step instructions for a computer to follow. But consider the use case of identifying specific actions in soccer games. There's so much variation in color, angle, resolution, and lighting that it would require coding far too many rules to tell a machine how to make the correct decision. It's hard to imagine where you'd even begin. Or, where customer comments, which use a broad and varied vocabulary and structure, are too diverse to be captured by a simple set of rules. If you tried to build manual filters, you'd quickly find that you weren't able to categorize most of your customer comments. You need a system that can generalize to a wide variety of comments. In a scenario where a sequence of specific rules is bound to expand exponentially, you need a system that can learn from examples.
Fortunately, machine learning is in a position to solve these problems.
How does Vertex AI work?
Vertex AI involves supervised learning tasks to achieve a chosen outcome.
The specifics of the algorithm and training methods change based on the
data type and
use case. There are many different subcategories of machine learning,
all of which solve different problems and work within different constraints.
Image
You train, test, and validate the machine learning model with example images that are annotated with labels for classification, or annotated with labels and bounding boxes for object detection. Using supervised learning, you can train a model to recognize the patterns and content that you care about in images.
Tabular
You train a machine learning model with example data. Vertex AI
uses tabular (structured) data to train a machine learning model to make
predictions on new data. One column from your dataset, called the target, is
what your model will learn to predict. Some number of the other data columns
are inputs (called features) that the model will learn patterns from. You can
use the same input features to build multiple kinds of models just by changing
the target column and training options. From the email marketing example, this
means you could build models with the same input features but with different
target predictions. One model could predict a customer's persona (a
categorical target), another model could predict their monthly spending (a
numerical target), and another could forecast daily demand of your products
for the next three months (series of numerical targets).
Text
Vertex AI enables you to perform supervised learning. This involves training a computer to recognize patterns from labeled data. Using supervised learning, you can train an AutoML model to recognize content that you care about in text.
Video
You train, test, and validate the machine learning model with videos you've already labeled. With a trained model, you can then input new videos to the model, which then outputs video segments with labels. A video segment defines the start and end time offset within a video. The segment could be the whole video, user-defined time segment, automatically detected video shot, or just a timestamp for when start time is the same as end time. A label is a predicted "answer" from the model. For instance, in the soccer use cases mentioned earlier, for each new soccer video, depending on the model type:
- a trained action recognition model outputs video time offsets with labels describing action shots like "goal", "personal foul", and so on.
- a trained classification model outputs automatically detected shot segments with user-defined labels like "game view", "audience view".
- a trained object tracking model outputs tracks of the soccer ball or the players by way of bounding boxes in frames where the objects appear.
Vertex AI workflow
Vertex AI uses a standard machine learning workflow:
- Gather your data: Determine the data you need for training and testing your model based on the outcome you want to achieve.
- Prepare your data: Make sure your data is properly formatted and labeled.
- Train: Set parameters and build your model.
- Evaluate: Review model metrics.
- Deploy and predict: Make your model available to use.
But before you start gathering your data, you need to think about the problem you are trying to solve. This will inform your data requirements.
Data Preparation
Assess your use case
Start with your problem: What is the outcome you want to achieve?
Image
While putting together the dataset, always start with your use case. You can begin with the following questions:
- What is the outcome you're trying to achieve?
- What kinds of categories or objects would you need to recognize to achieve this outcome?
- Is it possible for humans to recognize those categories? Although Vertex AI can handle a greater magnitude of categories than humans can remember and assign at any one time, if a human cannot recognize a specific category, then Vertex AI will have a hard time as well.
- What kinds of examples would best reflect the type and range of data your system will see and try to classify?
Tabular
What kind of data is the target column? How much data do you have access to? Depending on yours answers, Vertex AI creates the necessary model to solve your use case:
- A binary classification model predicts a binary outcome (one of two classes). Use this for yes or no questions, for example, predicting whether a customer would buy a subscription (or not). All else being equal, a binary classification problem requires less data than other model types.
- A multi-class classification model predicts one class from three or more discrete classes. Use this to categorize things. For the retail example, you'd want to build a multi-class classification model to segment customers into different personas.
- A forecasting model predicts a sequence of values. For example, as a retailer, you might want to forecast daily demand of your products for the next 3 months so that you can appropriately stock product inventories in advance.
- A regression model predicts a continuous value. For the retail example, you'd want to build a regression model to predict how much a customer will spend next month.
Text
While putting together the dataset, always start with your use case. You can begin with the following questions:
- What outcome are you trying to achieve?
- What kinds of categories do you need to recognize to achieve this outcome?
- Is it possible for humans to recognize those categories? Although Vertex AI can handle more categories than humans can remember and assign at any one time, if a human can't recognize a specific category, then Vertex AI will have a hard time as well.
- What kinds of examples would best reflect the type and range of data your system will classify?
Video
Depending on the outcome you are trying to achieve, select the appropriate model objective:
- To detect action moments in a video such as identifying scoring a goal, causing a foul, or making a penalty kick use the action recognition objective.
- To classify TV shots into the following categories, commercial, news, TV shows, and so on, use the classification objective.
- To locate and track objects in a video, use the object tracking objective.
See Preparing video data for more information about best practices when preparing datasets for action recognition, classification, and object tracking objectives.
Gather your data
After you have established your use case, you need to gather the data that lets you create the model you want.
Image
After you've established what data you need, you have to find a way to source it. You can
begin by considering all the data your organization collects. You may find that you're
already collecting the relevant data you need to train a model. In case you don't have that data,
you can obtain it manually or outsource it to a third-party provider.
Include enough labeled examples in each category
The bare minimum required by Vertex AI Training is 100 image examples per category/label for classification.
The likelihood of successfully recognizing a label goes up with the number of high-quality
examples for each; in general, the more labeled data you can bring to the training process,
the better your model will be. Target at least 1000 examples per label.
Distribute examples equally across categories
It's important to capture roughly similar amounts of training examples for each category. Even
if you have an abundance of data for one label, it is best to have an equal distribution for
each label. To see why, imagine that 80% of the images you use to build your model are pictures
of single-family homes in a modern style. With such an unbalanced distribution of labels, your
model is very likely to learn that it's safe to always tell you a photo is of a modern
single-family house, rather than going out on a limb to try to predict a much less common label.
It's like writing a multiple-choice test where almost all the correct answers are "C" - soon
your savvy test-taker will figure out it can answer "C" every time without even looking at
the question.
We understand it may not always be possible to source an approximately equal number of examples for each label. High quality, unbiased examples for some categories may be harder to source. In those circumstances, you can follow this rule of thumb - the label with the lowest number of examples should have at least 10% of the examples as the label with the highest number of examples. So if the largest label has 10,000 examples, the smallest label should have at least 1,000 examples.
Capture the variation in your problem space
For similar reasons, try to ensure that your data captures the variety and diversity of your
problem space. The broader a selection the model training process gets to see, the more readily
it will generalize to new examples. For example, if you're trying to classify photos of consumer
electronics into categories, the wider a variety of consumer electronics the model is exposed to
in training, the more likely it'll be able to distinguish between a novel model of tablet,
phone, or laptop, even if it's never seen that specific model before.
Match data to the intended output for your model
Find images that are visually similar to what you're planning to make predictions on. If you are
trying to classify house images that were all taken in snowy winter weather, you probably won't
get great performance from a model trained only on house images taken in sunny weather even if
you've tagged them with the classes you're interested in, as the lighting and scenery may be
different enough to affect performance. Ideally, your training examples are real-world data
drawn from the same dataset you're planning to use the model to classify.
Tabular
After you've established your use case, you'll need to gather data to train your model.
Data sourcing and preparation are critical steps for building a machine learning model.
The data you have available informs the kind of problems you can solve. How much data do
you have available? Are your data relevant to the questions you're trying to answer? While
gathering your data, keep in mind the following key considerations.
Select relevant features
A feature is an input attribute used for model training. Features are how your model identifies patterns to make predictions, so they need to be relevant to your problem. For example, to build a model that predicts whether a credit card transaction is fraudulent or not, you'll need to build a dataset that contains transaction details like the buyer, seller, amount, date and time, and items purchased. Other helpful features could be historic information about the buyer and seller, and how often the item purchased has been involved in fraud. What other features might be relevant?
Consider the retail email marketing use case from the introduction. Here's some feature columns you might require:
- List of items purchased (including brands, categories, prices, discounts)
- Number of items purchased (last day, week, month, year)
- Sum of money spent (last day, week, month, year)
- For each item, total number sold each day
- For each item, total in stock each day
- Whether you're running a promotion for a particular day
- Known demographic profile of shopper
Include enough data
In general, the more training examples you have, the better your outcome. The amount of example
data required also scales with the complexity of the problem you're trying to solve. You won't
need as much data to get an accurate binary classification model compared to a multi-class model
because it's less complicated to predict one class from two rather than many.
There's no perfect formula, but there are recommended minimums of example data:
- Classification problem: 50 rows x the number features
- Forecasting problem:
- 5000 rows x the number of features
- 10 unique values in the time series identifier column x the number of features
- Regression problem: 200 x the number of features
Capture variation
Your dataset should capture the diversity of your problem space. The more diverse examples a model sees during training, the more readily it can generalize to new or less common examples. Imagine if your retail model was trained only using purchase data from the winter. Would it be able to successfully predict summer clothing preferences or purchase behaviors?
Text
After you've established what data you'll require, you need to find a way to source it. You can
begin by taking into account all the data your organization collects. You may find that you're
already collecting the data you would need to train a model. In case you don't have the data you
need, you can obtain it manually or outsource it to a third-party provider.
Include enough labeled examples in each category
The likelihood of successfully recognizing a label goes up with the number of high-quality
examples for each; in general, the more labeled data that you can bring to the training process,
the better your model will be. The number of samples needed also varies with the degree of
consistency in the data you want to predict and on your target level of accuracy. You can use
fewer examples for consistent datasets or to achieve 80% accuracy rather than 97% accuracy.
Train a model and then evaluate the results. Add more examples and
retrain until you meet your accuracy targets, which could require hundreds or even thousands of
examples per label. For more information about data requirements and recommendations, see
Preparing
text training data for AutoML models.
Distribute examples equally across categories
It's important to capture a roughly similar number of training examples for each category. Even
if you have an abundance of data for one label, it is best to have an equal distribution for
each label. To see why, imagine that 80% of the customer comments you use to build your model
are estimate requests. With such an unbalanced distribution of labels, your model is very likely
to learn that it's safe to always tell you a customer comment is an estimate request, rather than
trying to predict a much less common label. It's like writing a multiple-choice test where almost
all the correct answers are "C" - soon your savvy test-taker will figure out it can answer "C"
every time without even looking at the question.
It might not always be possible to source an approximately equal number of examples for each label. High quality, unbiased examples for some categories may be harder to source. In those circumstances, the label with the lowest number of examples should have at least 10% of the examples as the label with the highest number of examples. So if the largest label has 10,000 examples, the smallest label should have at least 1,000 examples.
Capture the variation in your problem space
For similar reasons, try to have your data capture the variety and diversity of your problem
space. When you provide a broader set of examples, the model is better able to generalize to
new data. Say you're trying to classify articles about consumer electronics into topics. The more
brand names and technical specifications you provide, the easier it will be for the model to
figure out the topic of an article – even if that article is about a brand that didn't make it
into the training set at all. You might also consider including a "none_of_the_above" label for
documents that don't match any of your defined labels to further improve model performance.
Match data to the intended output for your model
Find text examples that are similar to what you're planning to make predictions on. If you are
trying to classify social media posts about glassblowing, you probably won't get great
performance from a model trained on glassblowing information websites, since the vocabulary and
style may be different. Ideally, your training examples are real-world data drawn from the
same dataset you're planning to use the model to classify.
Video
After you've established your use case, you'll need to gather the video data that will let you
create the model you want. The data you gather for training informs the kind of problems you
can solve. How many videos can you use? Do the videos contain enough examples for what you want
your model to predict? While gathering your video data, keep in mind the following considerations.
Include enough videos
Generally, the more training videos in your dataset, the better your outcome. The number of
recommended videos also scales with the complexity of the problem you're trying to solve. For
example, for classification you'll need less video data for a binary classification problem
(predicting one class from two) than a multi-label problem (predicting one or more classes
from many).
The complexity of what you're trying to do also determines how much video data you need. Consider the soccer use case for classification, which is building a model to distinguish action shots, versus training a model able to classify different styles of swimming. For example, to distinguish between breast stroke, butterfly, backstroke, and so on, you'll need more training data to identify the different swimming styles to help the model learn how to identify each type accurately. See Preparing video data for guidance to understand your minimal video data needs for action recognition, classification, and object tracking.
The amount of video data required may be more than you currently have. Consider obtaining more videos through a third-party provider. For example, you could purchase or obtain more hummingbird videos if you don't have enough for your game action identifier model.
Distribute videos equally across classes
Try to provide a similar number of training examples for each class. Here's why: Imagine that
80% of your training dataset is soccer videos featuring goal shots, but only 20% of the videos
depict personal fouls or penalty kicks. With such an unequal distribution of classes, your model
is more likely to predict that a given action is a goal. It's similar to writing a multiple-choice
test where 80% of the correct answers are "C": The savvy model will quickly figure out that "C"
is a good guess most of the time.
It may not be possible to source an equal number of videos for each class. High quality, unbiased examples may also be difficult for some classes. Try to follow a 1:10 ratio: if the largest class has 10,000 videos, the smallest should have at least 1,000 videos.
Capture variation
Your video data should capture the diversity of your problem space. The more diverse examples a model sees during training, the more readily it can generalize to new or less common examples. Think about the soccer action classification model: Be sure to include videos with a variety of camera angles, day and night times, and variety of player movements. Exposing the model to a diversity of data improves the model's ability to distinguish one action from another.
Match data to the intended output

Find training videos that are visually similar to the videos you plan to input into the model for prediction. For example, if all of your training videos are taken in the winter or in the evening, the lighting and color patterns in those environments will affect your model. If you then use that model to test videos taken in the summer or daylight, you may not receive accurate predictions.
Consider these additional factors: Video resolution, Video frames per second, Camera angle, Background.
Prepare your data
Image
After you've decided which is right for you—a manual or the default split—you can add data in
Vertex AI by using one of the following methods:
- You can import data either from your computer or from Cloud Storage in an available format (CSV or JSON Lines) with the labels (and bounding boxes, if necessary) inline. For more information on import file format, see Preparing your training data. If you want to split your dataset manually, you can specify the splits in your CSV or JSON Lines import file.
- If your data hasn't been annotated, you can upload unlabeled images and use the Google Cloud console to apply annotations. You can manage these annotations in multiple annotation sets for the same set of images. For example, for a single set of images you can have one annotation set with bounding box and label information to do object detection, and also have another annotation set with just label annotations for classification.
Tabular
After you've identified your available data, you need to make sure it's ready for training.
If your data is biased or contains missing or erroneous values, this affects the quality of
the model. Consider the following before you start training your model.
Learn more.
Prevent data leakage and training-serving skew
Data leakage is when you use input features during training that "leak" information about the target that you are trying to predict which is unavailable when the model is actually served. This can be detected when a feature that is highly correlated with the target column is included as one of the input features. For example, if you're building a model to predict whether a customer will sign up for a subscription in the next month and one of the input features is a future subscription payment from that customer. This can lead to strong model performance during testing, but not when deployed in production, since future subscription payment information isn't available at serving time.
Training-serving skew is when input features used during training time are different from the ones provided to the model at serving time, causing poor model quality in production. For example, building a model to predict hourly temperatures but training with data that only contains weekly temperatures. Another example: always providing a student's grades in the training data when predicting student dropout, but not providing this information at serving time.
Understanding your training data is important to preventing data leakage and training-serving skew:
- Before using any data, make sure you know what the data means and whether or not you should use it as a feature
- Check the correlation in the Train tab. High correlations should be flagged for review.
- Training-serving skew: make sure you only provide input features to the model that are available in the exact same form at serving time.
Clean up missing, incomplete, and inconsistent data
It's common to have missing and inaccurate values in your example data. Take time to review and, when possible, improve your data quality before using it for training. The more missing values, the less useful your data will be for training a machine learning model.
- Check your data for missing values and correct them if possible, or leave the value blank if the column is set to be nullable. Vertex AI can handle missing values, but you are more likely to get optimal results if all values are available.
- For forecasting, check that the interval between training rows is consistent. Vertex AI can impute missing values, but you are more likely to get optimal results if all rows are available.
- Clean your data by correcting or deleting data errors or noise. Make your data consistent: Review spelling, abbreviations, and formatting.
Analyze your data after importing
Vertex AI provides an overview of your dataset after it's been imported. Review your imported dataset to make sure each column has the correct variable type. Vertex AI will automatically detect the variable type based on the columns values, but it's best to review each one. You should also review each column's nullability, which determines whether a column can have missing or NULL values.
Text
After you've decided which is right for you—a manual or the default split—you
can add data in Vertex AI by using one of the following
methods:
- You can import data either from your computer or Cloud Storage in the CSV or JSON Lines format with the labels inline, as specified in Preparing your training data. If you want to split your dataset manually, you can specify the splits in your CSV or JSON Lines file.
- If your data hasn't been labeled, you can upload unlabeled text examples and use the Vertex AI console to apply labels.
Video
After you've gathered the videos you want to include in your dataset, you need to make sure the
videos contain labels associated with video segments or bounding boxes. For action recognition,
the video segment is a timestamp and for classification the segment can be a video shot, a
segment or the whole video. For object tracking, the labels are associated with bounding boxes.
Why do my videos need bounding boxes and labels?
For object tracking, how does a Vertex AI model learn to identify patterns? That's what bounding boxes and labels are for during training. Take the soccer example: each example video will need to contain bounding boxes around objects you are interested in detecting. Those boxes also need labels like "person," and "ball," assigned to them. Otherwise the model won't know what to look for. Drawing boxes and assigning labels to your example videos can take time.
If your data hasn't been labeled yet, you can also upload the unlabeled videos and use the Google Cloud console to apply bounding boxes and labels. For more information, see Label data using the Google Cloud console.
Train model
Image
Consider how Vertex AI uses your dataset in creating a custom model
Your dataset contains training, validation and testing sets. If you do not specify the splits
(see Prepare your data), then Vertex AI automatically uses 80% of your images for training,
10% for validating, and 10% for testing.
Training Set
The vast majority of your data should be in the training set. This is the data your model "sees"
during training: it's used to learn the parameters of the model, namely the weights of the
connections between nodes of the neural network.
Validation Set
The validation set, sometimes also called the "dev" set, is also used during the training process.
After the model learning framework incorporates training data during each iteration of the
training process, it uses the model's performance on the validation set to tune the model's
hyperparameters, which are variables that specify the model's structure. If you tried to use the
training set to tune the hyperparameters, it's quite likely the model would end up overly focused
on your training data, and have a hard time generalizing to examples that don't exactly match it.
Using a somewhat novel dataset to fine-tune model structure means your model will generalize
better.
Test Set
The test set is not involved in the training process at all. Once the model has completed its
training entirely, we use the test set as an entirely new challenge for your model. The
performance of your model on the test set is intended to give you a pretty good idea of how your
model will perform on real-world data.
Manual splitting
You can also split your dataset yourself. Manually splitting your data is a good choice when
you want to exercise more control over the process or if there are specific examples that you're
sure you want included in a certain part of your model training lifecycle.
Tabular
After your dataset is imported, the next step is to train a model. Vertex AI will generate a reliable machine learning model with the training defaults, but you may want to adjust some of the parameters based on your use case.
Try to select as many feature columns as possible for training, but review each to make sure that it's appropriate for training. Keep in mind the following for feature selection:
- Don't select feature columns that will create noise, like randomly assigned identifier columns with a unique value for each row.
- Make sure you understand each feature column and its values.
- If you're creating multiple models from one dataset, remove target columns that aren't part of the current prediction problem.
- Recall the fairness principles: Are you training your model with a feature that could lead to biased or unfair decision-making for marginalized groups?
How Vertex AI uses your dataset
Your dataset will be split into training, validation and testing sets. The
default split Vertex AI applies depends on the type of model that
you are training. You can also specify the splits (manual splits) if
necessary. For more information, see About data splits for AutoML
models.
Training Set
The vast majority of your data should be in the training set. This is the data your model "sees"
during training: it's used to learn the parameters of the model, namely the weights of the
connections between nodes of the neural network.
Validation Set
The validation set, sometimes also called the "dev" set, is also used during the training process.
After the model learning framework incorporates training data during each iteration of the
training process, it uses the model's performance on the validation set to tune the model's
hyperparameters, which are variables that specify the model's structure. If you tried to use the
training set to tune the hyperparameters, it's quite likely the model would end up overly focused
on your training data, and have a hard time generalizing to examples that don't exactly match it.
Using a somewhat novel dataset to fine-tune model structure means your model will generalize
better.
Test Set
The test set is not involved in the training process at all. After the model has completed its
training entirely, Vertex AI uses the test set as an entirely new challenge for your model.
The performance of your model on the test set is intended to give you a pretty good idea of how
your model will perform on real-world data.
Text
Consider how Vertex AI uses your dataset to create a custom model
Your dataset contains training, validation and testing sets. If you don't
specify the splits as explained in Prepare your
data, Vertex AI automatically uses 80% of your content
documents for training, 10% for validating, and 10% for testing.
Training Set
The vast majority of your data should be in the training set. This is the data your model "sees"
during training: it's used to learn the parameters of the model, namely the weights of the
connections between nodes of the neural network.
Validation Set
The validation set, sometimes also called the "dev" set, is also used during the training process.
After the model learning framework incorporates training data during each iteration of the
training process, it uses the model's performance on the validation set to tune the model's
hyperparameters, which are variables that specify the model's structure. If you tried to use the
training set to tune the hyperparameters, it's quite likely the model would end up overly focused
on your training data, and have a hard time generalizing to examples that don't exactly match it.
Using a somewhat novel dataset to fine-tune model structure means your model will generalize
better.
Test Set
The test set is not involved in the training process at all. After the model has completed its
training entirely, we use the test set as an entirely new challenge for your model. The
performance of your model on the test set is intended to give you a pretty good idea of how your
model will perform on real-world data.
Manual splitting
You can also split your dataset yourself. Manually splitting your data is a good choice when
you want to exercise more control over the process or if there are specific examples that you're
sure you want included in a certain part of your model training lifecycle.
Video
After your training video data is prepared, you're ready to create a machine learning model. Note you can create annotation sets for different model objectives in the same dataset. See Creating an annotation set.
One of the benefits of Vertex AI is that the default parameters will guide you to a reliable machine learning model. But you may need to adjust parameters depending on your data quality and the outcome you're looking for. For example:
- Prediction type is the level of granularity which your videos are processed.
- Frame rate is important if the labels you are trying to classify are sensitive to motion changes, as in action recognition. For example, take running vs walking. A low Frames Per Second (FPS) walk clip could look like running. As for object tracking, it is also sensitive to frame rate. Basically the object being tracked needs to have enough overlap between adjacent frames.
- Resolution for object tracking is more important than it is for action recognition or video classification. When the objects are small, be sure to upload higher resolution videos. The current pipeline uses 256x256 for regular training or 512x512 if there are too many small objects (whose area is less than 1% of the image area) in user data. The recommendation is to use videos that are at least 256p. Using higher resolution videos may not help with improving the model performance because internally the video frames are downsampled to boost training and inference speed.
Evaluate, test, and deploy your model
Evaluate model
Image
After your model is trained, you will receive a summary of the model's performance. Click evaluate or see full evaluation to view a detailed analysis.
Debugging a model is more about debugging the data than the model itself. If at any point your
model starts acting in an unexpected manner as you're evaluating its performance before and
after pushing to production, you should return and check your data to see where it might be
improved.
What kinds of analysis can I perform in Vertex AI?
In the Vertex AI evaluate section, you can assess your custom model's performance using the model's output on test examples, and common machine learning metrics. In this section, we will cover what each of these concepts mean.
- The model output
- The score threshold
- True positives, true negatives, false positives, and false negatives
- Precision and recall
- Precision/recall curves
- Average precision
How do I interpret the model's output?
Vertex AI pulls examples from your test data to present entirely new challenges for your
model. For each example, the model outputs a series of numbers that communicate how strongly it
associates each label with that example. If the number is high, the model has high confidence
that the label should be applied to that document.
What is the Score Threshold?
We can convert these probabilities into binary 'on'/'off' values by setting a score threshold.
The score threshold refers to the level of confidence the model must have to assign a category to
a test item. The score threshold slider in the Google Cloud console is a visual tool to test
the effect of different thresholds for all categories and individual categories in your dataset.
If your score threshold is low, your model will classify more images, but runs the risk of
misclassifying a few images in the process. If your score threshold is high, your model classifies
fewer images, but it has a lower risk of misclassifying images. You can tweak the per-category
thresholds in the Google Cloud console to experiment. However, when using your model in
production, you must enforce the thresholds you found optimal on your side.
What are True Positives, True Negatives, False Positives, False Negatives?
After applying the score threshold, the predictions made by your model will fall in one of the
following four categories:
The thresholds you found optimal on your side.
We can use these categories to calculate precision and recall — metrics that help us gauge the effectiveness of our model.
What are precision and recall?
Precision and recall help us understand how well our model is capturing information, and how much
it's leaving out. Precision tells us, from all the test examples that were assigned a label, how
many actually were supposed to be categorized with that label. Recall tells us, from all the test
examples that should have had the label assigned, how many were actually assigned the label.
Should I optimize for precision or recall?
Depending on your use case, you may want to optimize for either precision or recall. Consider the following two use cases when deciding which approach works best for you.
Use Case: Privacy in images
Suppose you want to create a system that automatically detects sensitive information and blurs
it out.
False positives in this case would be, things that don't need to be blurred that get blurred,
which can be annoying but not detrimental.
False negatives in this case would be things that need to be blurred that fail to get blurred,
like a credit card, which can lead to identity theft.
In this case, you would want to optimize for recall. This metric measures, for all the predictions made, how much is being left out. A high-recall model is likely to label marginally relevant examples. This is useful for cases where your category has scarce training data.
Use case: Stock photo search
Suppose you want to create a system that finds the best stock photo for a given keyword.
A false positive in this case would be returning an irrelevant image. Since your product prides itself on returning only the best-match images, this would be a major failure.
A false negative in this case would be failing to return a relevant image for a keyword search.
Since many search terms have thousands of photos that are a strong potential match, this is fine.
In this case, you would want to optimize for precision. This metric measures, for all the predictions made, how correct they are. A high-precision model is likely to label only the most relevant examples, which is useful for cases where your class is common in the training data.
How do I use the Confusion Matrix?
How do I interpret the precision-recall curves?
The score threshold tool lets you explore how your chosen score threshold affects
precision and recall. As you drag the slider on the score threshold bar, you can see where that
threshold places you on the precision-recall tradeoff curve, as well as how that threshold
affects your precision and recall individually (for multiclass models, on these graphs, precision
and recall means the only label used to calculate precision and recall metrics is the top-scored
label in the set of labels we return). This can help you find a good balance between false
positives and false negatives.
Once you've chosen a threshold that seems to be acceptable for your model as a whole, click the individual labels and see where that threshold falls on their per-label precision-recall curve. In some cases, it might mean you get a lot of incorrect predictions for a few labels, which might help you decide to choose a per-class threshold that's customized to those labels. For example, say you look at your houses dataset and notice that a threshold at 0.5 has reasonable precision and recall for every image type except "Tudor", perhaps because it's a very general category. For that category, you see tons of false positives. In that case, you might decide to use a threshold of 0.8 just for "Tudor" when you call the classifier for predictions.
What is average precision?
A useful metric for model accuracy is the area under the precision-recall curve. It measures how well your model performs across all score thresholds. In Vertex AI, this metric is called Average Precision. The closer to 1.0 this score is, the better your model is performing on the test set; a model guessing at random for each label would get an average precision around 0.5.
Tabular
After model training, you'll receive a summary of its performance. Model evaluation metrics are
based on how the model performed against a slice of your dataset (the test dataset). There are a
couple of key metrics and concepts to consider when determining whether your model is ready to be
used with real data.
Classification metrics
Score threshold
Consider a machine learning model that predicts whether a customer will buy a jacket in the next
year. How sure does the model need to be before predicting that a given customer will buy a
jacket? In classification models, each prediction is assigned a confidence score – a numeric
assessment of the model's certainty that the predicted class is correct. The score threshold is
the number that determines when a given score is converted into a yes or no decision; that is,
the value at which your model says "yes, this confidence score is high enough to conclude that
this customer will purchase a coat in the next year.
If your score threshold is low, your model will run the risk of misclassification. For that reason, the score threshold should be based on a given use case.
Prediction outcomes
After applying the score threshold, predictions made by your model will fall into one of four categories. To understand these categories, imagine again a jacket binary classification model. In this example, the positive class (what the model is attempting to predict) is that the customer will purchase a jacket in the next year.
- True positive: The model correctly predicts the positive class. The model correctly predicted that a customer purchased a jacket.
- False positive: The model incorrectly predicts the positive class. The model predicted that a customer purchased a jacket, but they didn't.
- True negative: The model correctly predicts the negative class. The model correctly predicted that a customer didn't purchase a jacket.
- False negative: The model incorrectly predicts a negative class. The model predicted that a customer didn't purchase a jacket, but they did.
Precision and recall
Precision and recall metrics help you understand how well your model is capturing information and what it's leaving out. Learn more about precision and recall.
- Precision is the fraction of the positive predictions that were correct. Of all the predictions of a customer purchase, what fraction were actual purchases?
- Recall is the fraction of rows with this label that the model correctly predicted. Of all the customer purchases that could have been identified, what fraction were?
Depending on your use case, you may need to optimize for either precision or recall.
Other classification metrics
- AUC PR: The area under the precision-recall (PR) curve. This value ranges from zero to one, where a higher value indicates a higher-quality model.
- AUC ROC: The area under the receiver operating characteristic (ROC) curve. This ranges from zero to one, where a higher value indicates a higher-quality model.
- Accuracy: The fraction of classification predictions produced by the model that were correct.
- Log loss: The cross-entropy between the model predictions and the target values. This ranges from zero to infinity, where a lower value indicates a higher-quality model.
- F1 score: The harmonic mean of precision and recall. F1 is a useful metric if you're looking for a balance between precision and recall and there's an uneven class distribution.
Forecasting and regression metrics
After your model is built, Vertex AI provides a variety of standard metrics for you to review. There's no perfect answer on how to evaluate your model; consider evaluation metrics in context with your problem type and what you want to achieve with your model. The following list is an overview of some metrics Vertex AI can provide.
Mean absolute error (MAE)
MAE is the average absolute difference between the target and predicted values. It measures the average magnitude of the errors--the difference between a target and predicted value--in a set of predictions. And because it uses absolute values, MAE doesn't consider the relationship's direction, nor indicate underperformance or overperformance. When evaluating MAE, a smaller value indicates a higher-quality model (0 represents a perfect predictor).
Root mean square error (RMSE)
RMSE is the square root of the average squared difference between the target and predicted values. RMSE is more sensitive to outliers than MAE, so if you're concerned about large errors, then RMSE can be a more useful metric to evaluate. Similar to MAE, a smaller value indicates a higher-quality model (0 represents a perfect predictor).
Root mean squared logarithmic error (RMSLE)
RMSLE is RMSE in logarithmic scale. RMSLE is more sensitive to relative errors than absolute ones and cares more about underperformance than overperformance.
Observed quantile (forecasting only)
For a given target quantile, the observed quantile shows the actual fraction of observed values below the specified quantile prediction values. The observed quantile shows how far or close the model is to the target quantile. A smaller difference between the two values indicates a higher-quality model.
Scaled pinball loss (forecasting only)
Measures the quality of a model at a given target quantile. A lower number indicates a higher-quality model. You can compare the scaled pinball loss metric at different quantiles to determine the relative accuracy of your model between those different quantiles.
Text
After your model has trained, you receive a summary of your model performance. To view a detailed analysis, click evaluate or see full evaluation.
What should I keep in mind before evaluating my model?
Debugging a model is more about debugging the data than the model itself. If your model starts
acting in an unexpected manner as you're evaluating its performance before and after pushing
to production, you should return and check your data to see where it might be improved.
What kinds of analysis can I perform in Vertex AI?
In the Vertex AI evaluate section, you can assess your custom model's performance using the model's output on test examples and common machine learning metrics. This section covers what each of the following concepts means:
- The model output
- The score threshold
- True positives, true negatives, false positives, and false negatives
- Precision and recall
- Precision/recall curves.
- Average precision
How do I interpret the model's output?
Vertex AI pulls examples from your test data to present new challenges for your
model. For each example, the model outputs a series of numbers that communicate how strongly it
associates each label with that example. If the number is high, the model has high confidence
that the label should be applied to that document.
What is the Score Threshold?
The score threshold lets Vertex AI convert probabilities into binary 'on'/'off'
values. The score threshold refers to the level of confidence the model must have to assign a
category to a test item. The score threshold slider in the console is a visual tool to test the
impact of different thresholds in your dataset. In the preceding example, if we set the score
threshold to 0.8 for all categories, "Great Service" and "Suggestion" will be assigned but not
"Info Request." If your score threshold is low, your model will classify more text items, but
runs the risk of misclassifying more text items in the process. If your score threshold is high,
your model will classify fewer text items, but it will have a lower risk of misclassifying text
items. You can tweak the per-category thresholds in the Google Cloud console to experiment.
However, when using
your model in production, you must enforce the thresholds you found optimal on your side.
What are True Positives, True Negatives, False Positives, False Negatives?
After applying the score threshold, the predictions made by your model will fall in one of the
following four categories.
You can use these categories to calculate precision and recall — metrics that help gauge the effectiveness of your model.
What are precision and recall?
Precision and recall help us understand how well our model is capturing information, and how much
it's leaving out. Precision tells us, from all the test examples that were assigned a label, how
many actually were supposed to be categorized with that label. Recall tells us, from all the test
examples that should have had the label assigned, how many were actually assigned the label.
Should I optimize for precision or recall?
Depending on your use case, you may want to optimize for either precision or recall. Consider the following two use cases when deciding which approach works best for you.
Use case: Urgent documents
Say you want to create a system that can prioritize documents that are urgent from ones
that are not.
A false positive in this case would be a document that is not urgent, but gets marked as such.
The user can dismiss them as non-urgent and move on.
A false negative in this case would be, a document that is urgent, but the system fails to flag it as such. This could cause problems!
In this case, you would want to optimize for recall. This metric measures, for all the predictions made, how much is being left out. A high recall model is likely to label marginally relevant examples. This is useful for cases where your category has scarce training data.
Use case: Spam filtering
Say you want to create a system that automatically filters email messages that are spam
from messages that are not.
A false negative in this case would be a spam email that does not get caught and that you
see in your inbox. Usually, this is just a bit annoying.
A false positive in this case would be an email that is falsely flagged as spam and gets removed from your inbox. If the email was important, the user might be adversely affected.
In this case, you would want to optimize for precision. This metric measures, for all the predictions made, how correct they are. A high-precision model is likely to label only the most relevant examples, which is useful for cases where your category is common in the training data.
How do I use the Confusion Matrix?
We can compare the model's performance on each label using a confusion matrix. In an ideal model,
all the values on the diagonal will be high, and all the other values will be low. This shows
that the desired categories are being identified correctly. If any other values are high, it
gives us a clue into how the model is misclassifying test items.
How do I interpret the Precision-Recall curves?
The score threshold tool lets you explore how your chosen score threshold affects your
precision and recall. As you drag the slider on the score threshold bar, you can see where that
threshold places you on the precision-recall tradeoff curve, as well as how that threshold
affects your precision and recall individually (for multiclass models, on these graphs,
precision and recall means the only label used to calculate precision and recall metrics is
the top-scored label in the set of labels we return). This can help you find a good balance
between false positives and false negatives.
After you've chosen a threshold that seems to be acceptable for your model on the whole, you can click individual labels and see where that threshold falls on their per-label precision-recall curve. In some cases, it might mean you get a lot of incorrect predictions for a few labels, which might help you decide to choose a per-class threshold that's customized to those labels. For example, say you look at your customer comments dataset and notice that a threshold at 0.5 has reasonable precision and recall for every comment type except "Suggestion", perhaps because it's a very general category. For that category, you see tons of false positives. In that case, you might decide to use a threshold of 0.8 just for "Suggestion" when you call the classifier for predictions.
What is Average Precision?
A useful metric for model accuracy is the area under the precision-recall curve. It measures how well your model performs across all score thresholds. In Vertex AI, this metric is called Average Precision. The closer to 1.0 this score is, the better your model is performing on the test set; a model guessing at random for each label would get an average precision around 0.5.
Video
After model training, you will receive a summary of its performance. Model evaluation metrics are
based on how the model performed against a slice of your dataset (the test dataset). There
are a couple of key metrics and concepts to consider when determining whether your model is ready
to be used with new data.
Score threshold
How does a machine learning model know when a soccer goal is really a goal? Each prediction is
assigned a confidence score – a numeric assessment of the model's certainty that a given video
segment contains a class. The score threshold is the number that determines when a given score
is converted into a yes or no decision; that is, the value at which your model says "yes, this
confidence number is high enough to conclude that this video segment contains a goal."
If your score threshold is low, your model will run the risk of mislabeling video segments. For that reason, the score threshold should be based on a given use case. Imagine a medical use case like cancer detection, where the consequences of mislabeling are higher than mislabeling sports videos. In cancer detection, a higher score threshold is appropriate.
Prediction outcomes
After applying the score threshold, predictions made by your model will fall into one of four categories. To understand these categories, imagine that you built a model to detect whether a given segment contains a soccer goal (or not). In this example, a goal is the positive class (what the model is attempting to predict).
- True positive: The model correctly predicts the positive class. The model correctly predicted a goal in the video segment.
- False positive: The model incorrectly predicts the positive class. The model predicted a goal was in the segment, but there wasn't one.
- True negative: The model correctly predicts the negative class. The model correctly predicted there wasn't a goal in the segment.
- False negative: The model incorrectly predicts a negative class. The model predicted there wasn't a goal in the segment, but there was one.
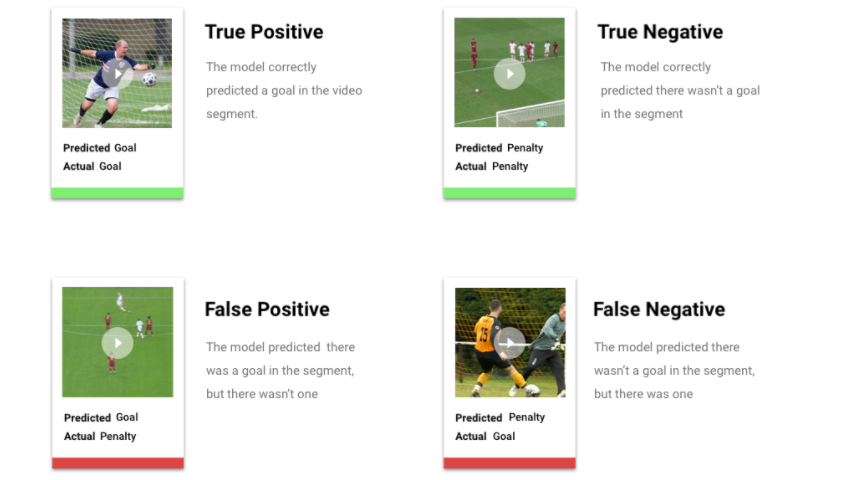
Precision and recall
Precision and recall metrics help you understand how well your model is capturing information and what it's leaving out. Learn more about precision and recall
- Precision is the fraction of the positive predictions that were correct. Of all the predictions labeled "goal," what fraction actually contained a goal?
- Recall is the fraction of all positive predictions that were actually identified. Of all the soccer goals that could have been identified, what fraction were?
Depending on your use case, you may need to optimize for either precision or recall. Consider the following use cases.
Use Case: Private information in videos
Imagine you're building software that automatically detects sensitive information in a video and blurs it out. The ramifications of false outcomes may include:
- A false positive identifies something that doesn't need to be censored, but gets censored
anyway. This might be annoying, but not detrimental.
- A false negative fails to identify information that needs to be censored, like a credit
card number. This would release private information and is the worst case scenario.
In this use case, it's critical to optimize for recall to ensure that the model finds all relevant cases. A model optimized for recall is more likely to label marginally relevant examples, but also likelier to label incorrect ones (blurring more than it needs to).
Use case: Stock video search
Say you want to create software that lets users search a library of videos based on a keyword. Consider the incorrect outcomes:
- A false positive returns an irrelevant video. Since your system is trying to provide only
relevant videos, then your software isn't really doing what it's built to do.
- A false negative fails to return a relevant video. Since many keywords have hundreds of
videos, this issue isn't as bad as returning an irrelevant video.
In this example, you'll want to optimize for precision to ensure your model delivers highly relevant, correct results. A high-precision model is likely to label only the most relevant examples, but may leave some out. Learn more about model evaluation metrics.
Test your model
Image
Vertex AI uses 10% of your data automatically (or, if you chose your data split yourself, whatever percentage you opted to use) to test the model, and the "Evaluate" page tells you how the model did on that test data. But just in case you want to confidence check your model, there are a few ways to do it. The easiest is to upload a few images on the "Deploy & test" page, and look at the labels the model chooses for your examples. Hopefully, this matches your expectations. Try a few examples of each type of image you expect to receive.
If you'd like to use your model in your own automated tests instead, the "Deploy & test" page also tells you how to make calls to the model programmatically.
Tabular
Evaluating your model metrics is primarily how you can determine whether your model is ready to deploy, but you can also test it with new data. Upload new data to see if the model's predictions match your expectations. Based on the evaluation metrics or testing with new data, you may need to continue improving your model's performance.
Text
Vertex AI uses 10% of your data automatically (or, if you chose the data split yourself, whatever percentage you opted to use) to test the model, and the Evaluate page tells you how the model did on that test data. But just in case you want to check your model, there are a few ways to do it. After you deploy your model, you can input text examples into the field on the Deploy and test page, and look at the labels the model chooses for your examples. Hopefully, this matches your expectations. Try a few examples of each type of comment you expect to receive.
If you want to use your model in your own automated tests, the Deploy and test page provides a sample API request that shows you how to make calls to the model programmatically.
If you want to use your model in your own automated tests, the Deploy and test page provides a sample API request that shows you how to make calls to the model programmatically.
On the Batch predictions page, you can create a batch prediction, which bundles many prediction requests in one. A batch prediction is asynchronous, meaning that the model will wait until it processes all of the prediction requests before returning results.
Video
Vertex AI video uses 20% of your data automatically—or, if you chose your data split yourself, whatever percentage you opted to use—to test the model. The Evaluate tab in the console tells you how the model did on that test data. But just in case you want to check your model, there are a few ways to do it. One way is to provide a CSV file with video data for testing in the "Test & Use" tab, and look at the labels the model predicts for the videos. Hopefully, this matches your expectations.
You can adjust the threshold for the predictions visualization and as well to look the predictions at 3 temporal scales: 1 second intervals, video camera shots after automated shot boundary detection, and entire video segments.
Deploy your model
Image
When you're satisfied with your model's performance, it's time to use the model. Perhaps that means production-scale usage, or maybe it's a one-time prediction request. Depending on your use case, you can use your model in different ways.
Batch prediction
Batch prediction is useful for making many prediction requests at once. Batch prediction is asynchronous, meaning that the model will wait until it processes all of the prediction requests before returning a JSON Lines file with prediction values.
Online prediction
Deploy your model to make it available for prediction requests using a REST API. Online prediction is synchronous (real-time), meaning that it will quickly return a prediction, but only accepts one prediction request per API call. Online prediction is useful if your model is part of an application and parts of your system are dependent on a quick prediction turnaround.
Tabular
When you're satisfied with your model's performance, it's time to use the model. Perhaps that means production-scale usage, or maybe it's a one-time prediction request. Depending on your use case, you can use your model in different ways.
Batch prediction
Batch prediction is useful for making many prediction requests at once. Batch prediction is asynchronous, meaning that the model will wait until it processes all of the prediction requests before returning a CSV file or BigQuery Table with prediction values.
Online prediction
Deploy your model to make it available for prediction requests using a REST API. Online prediction is synchronous (real-time), meaning that it will quickly return a prediction, but only accepts one prediction request per API call. Online prediction is useful if your model is part of an application and parts of your system are dependent on a quick prediction turnaround.
Video
When you're satisfied with your model's performance, it's time to use the model. Vertex AI uses batch prediction, which lets you upload a CSV file with paths to videos hosted on Cloud Storage. Your model will process each video and output predictions in another CSV file. Batch prediction is asynchronous, meaning that the model processes all of the prediction requests first before outputting the results.
Clean up
To help avoid unwanted charges, undeploy your model when it's not in use.
When you're finished using your model, delete the resources that you created to avoid incurring unwanted charges to your account.
- Hello image data: Clean up your project
- Hello tabular data: Clean up your project
- Hello text data: Clean up your project
- Hello video data: Clean up your project