소개
이 초보자 가이드는 AutoML에 대한 소개입니다. AutoML과 커스텀 학습 간 주요 차이점을 알아보려면 학습 방법 선택을 참조하세요.
상상해 보세요.
- 축구팀 코치라고 가정해 보세요.
- 디지털 소매업체의 마케팅 부서에서 일한다고 가정해 보세요.
- 건물의 유형을 식별하는 설계 프로젝트를 진행 중이라고 가정해 보세요.
- 회사 웹사이트에 문의 양식이 있다고 가정해 보세요.
동영상, 이미지, 텍스트, 표를 수동으로 선별하는 작업은 지루하고 시간이 오래 걸립니다. 컴퓨터가 자동으로 콘텐츠를 식별하고 플래그를 지정하는 방법을 가르치는 일이 더 쉽지 않을까요?
이미지
도시에서 일관성 있는 건축 스타일을 가진 지역을 파악하려고 하는 건축물 보존 위원회에서 일한다고 가정해 보겠습니다. 수십만 개에 달하는 주택 사진을 살펴볼 수 있지만, 이 많은 이미지를 수동으로 분류하려면 오랜 시간이 걸리고 오류가 발생하기 쉽습니다. 몇 달 전에 인턴 직원이 수백 개의 사진에 라벨을 붙였지만 아무도 데이터를 살펴보지 않았습니다. 컴퓨터가 대신 검토하도록 가르칠 수 있다면 아주 유용할 것입니다.
테이블 형식
디지털 소매업체의 마케팅 부서에서 일한다고 가정해보세요. 귀하와 귀하의 팀은 고객 페르소나에 따른 맞춤형 이메일 프로그램을 제작하고 있습니다. 캐릭터를 만들었고 마케팅 이메일을 보낼 준비가 되었습니다. 이제 신규 고객 여부에 관계없이 소매업체 선호도와 지출 행동에 따라 고객을 각 캐릭터에 담는 시스템을 만들어야 합니다. 고객 참여를 극대화하려면 고객의 지출 행동을 예측하고 이메일을 가장 적절한 순간에 보내야 하죠.
디지털 소매업체에서 일하기 때문에 고객과 고객의 구매에 관한 데이터를 보유하고 있습니다. 하지만 신규 고객은 어떨까요? 전통적인 방식은 오랜 기간 구매해 온 기존 고객을 상대로 이러한 데이터를 계산하지만, 고객의 기록 데이터가 많지 않다면 성능이 떨어집니다. 이러한 값을 예측해 맞춤형 마케팅 프로그램을 모든 고객에게 빠르게 전달하는 시스템을 만들 수 있다면 어떨까요?
다행히 머신러닝과 Vertex AI를 이용하면 이러한 문제를 쉽게 해결할 수 있습니다.
텍스트
회사 웹사이트에 문의 양식이 있다고 가정해 보세요. 매일 이 양식을 통해 많은 메시지가 들어옵니다. 그중 대부분은 어떤 방식으로든 조치를 취할 수 있습니다. 하지만 한꺼번에 밀려 들어오므로 메시지 처리가 지체되기 쉽습니다. 직원마다 상이한 유형의 메시지를 처리하기 때문입니다.
자동화된 시스템이 메시지를 분류해 적절한 사람이 적절한 메시지를 확인할 수 있다면 얼마나 좋을까요?
의견을 살펴본 후 그 내용이 불만사항인지, 과거 서비스에 대한 칭찬인지, 회사에 대해 더 알아보려고 하는 것인지, 약속을 잡으려는 요청인지 또는 관계를 맺으려는 시도인지를 판단하는 시스템이 필요합니다.
동영상
분석에 사용할 게임의 대용량 동영상 라이브러리가 있습니다. 하지만 수백 시간 분량의 동영상을 검토해야 합니다. 각 동영상을 시청하면서 직접 세그먼트를 마킹하여 각각의 동작을 강조표시하는 것은 상당히 지루하고 시간이 오래 걸리는 작업입니다. 그리고 시즌마다 이 작업을 반복해야 합니다. 이제 동영상에 표시될 때마다 이러한 작업을 자동으로 식별하여 플래그를 지정할 수 있는 컴퓨터 모델을 생각해 보세요.
다음은 몇 가지 목표별 시나리오입니다.
- 동작 인식: 골을 넣거나 파울을 유발하거나 페널티킥과 같은 동작을 찾습니다. 팀의 강점과 약점을 분석하는 코치에게 도움이 됩니다.
- 분류: 각 동영상 장면을 하프 타임, 경기 뷰, 청중 뷰 또는 코치 뷰로 분류합니다. 관심 있는 동영상 장면만 탐색할 때 유용합니다.
- 객체 추적: 축구공이나 선수를 추적합니다. 경기의 히트맵, 패스 성공률과 같은 선수 통계를 얻는 데 유용합니다.
이 가이드에서는 Vertex AI가 AutoML 데이터 세트 및 모델에 대해 작동하는 방법을 설명하고 Vertex AI가 해결할 수 있는 문제의 유형을 보여줍니다.
공정성에 대한 참고사항
Google은 책임감 있는 AI 관행을 따르기 위해 노력하고 있습니다. 이를 위해 AutoML을 포함한 Google ML 제품은 공정성 및 인간 중심의 머신러닝과 같은 핵심 원칙을 기반으로 설계되었습니다. 자체 ML 시스템을 빌드할 때 편향을 완화할 수 있는 권장사항에 대한 자세한 내용은 포괄적인 ML 가이드 - AutoML을 참조하세요.
Vertex AI가 왜 이 문제에 적합한 도구일까요?
기존의 프로그래밍에서는 프로그래머가 컴퓨터가 따라야 하는 단계별 지침을 지정해야 합니다. 그러나 축구 경기에서 특정 동작을 식별하는 사용 사례를 생각해보세요. 색상, 각도, 해상도, 조명의 변화가 너무 많아서 정확한 결정을 내리는 방법을 컴퓨터에 알려주려면 너무 많은 규칙을 코딩해야 합니다. 어디부터 시작해야 할지 생각하는 것조차 어렵습니다. 또한, 고객 의견은 간단한 규칙으로 담아내기에는 너무 다양한 어휘와 구조로 되어 있습니다. 수동 필터를 구성한다면 고객 의견 대부분을 분류할 수 없다는 것을 곧 알게 될 것입니다. 다양한 의견을 일반화할 수 있는 시스템이 필요합니다. 일련의 특정한 규칙이 기하급수적으로 확장되는 시나리오에서는 예시를 통해 학습할 수 있는 시스템이 필요합니다.
다행히 이러한 문제를 해결하는 데 머신러닝이 중요한 역할을 하고 있습니다.
Vertex AI 작동 원리
Vertex AI는 선택한 결과를 얻기 위해 지도 학습 작업을 포함합니다.
세부적인 알고리즘과 학습 방법은 데이터 유형 및 사용 사례에 따라 달라집니다. 머신러닝에는 여러 하위 카테고리가 있으며 각 하위 카테고리는 서로 다른 문제를 해결하고 서로 다른 제약조건하에서 작동합니다.
이미지
분류용 라벨이 달린 주석이나 객체 감지를 위한 라벨 및 경계 상자로 주석 처리된 이미지 예를 사용하여 머신러닝 모델을 학습하고 테스트하며 검증합니다. 지도 학습을 이용하여 이미지에서 중요한 패턴과 콘텐츠를 인식하도록 모델을 학습시킬 수 있습니다.
테이블 형식
예시 데이터를 사용하여 머신러닝 모델을 학습시킵니다. Vertex AI는 표 형식(구조화) 데이터를 이용해 머신러닝 모델이 새 데이터를 바탕으로 예측을 수행하게 합니다. 데이터 세트에 있는 타겟이라는 열이 모델이 예측을 위해 학습하는 열입니다. 다른 데이터 열 중 일부는 모델이 패턴을 학습하는 대상인(특성이라고 함) 입력입니다. 같은 입력 특성을 사용하고 타겟 열과 학습 옵션만 바꾸면 여러 종류의 모델을 만들 수 있습니다. 본 이메일 마케팅 예시에서는 다른 타겟 예측의 같은 입력 특성으로 모델을 만들 수 있습니다. 고객의 캐릭터(범주형 타겟)를 예측하는 모델, 월간 지출(숫자 타겟)을 예측하는 모델, 향후 3개월 동안 제품의 일일 수요(일련의 숫자 타겟)를 예측하는 모델입니다.
텍스트
Vertex AI를 통해 지도 학습을 수행할 수 있습니다. 여기에는 라벨이 지정된 데이터의 패턴을 인식하도록 컴퓨터를 학습시키는 작업이 포함됩니다. 지도 학습을 이용하여 AutoML 모델을 학습시키고 텍스트에서 중요한 콘텐츠를 인식하도록 할 수 있습니다.
동영상
이미 라벨이 지정된 동영상으로 머신러닝 모델을 학습하고 테스트하고 검증합니다. 그 다음 학습된 모델을 사용하여 새 동영상을 모델에 입력하면 라벨이 지정된 동영상 세그먼트를 출력할 수 있습니다. 동영상 세그먼트는 동영상 내 시작 및 종료 시간 오프셋을 정의합니다. 이 세그먼트는 전체 동영상, 사용자 정의 시간 세그먼트, 자동 감지된 동영상 장면, 시작 시간이 종료 시간이 같을 때의 타임스탬프일 수 있습니다. 라벨은 모델에서 예측된 '답변'입니다. 예를 들어 앞에서 언급된 축구 사용 사례에서는 모델 유형에 따라 각각의 새로운 축구 동영상에 대해 다음과 같이 처리합니다.
- 학습된 동작 인식 모델은 '골', '신체 접촉 반칙' 등과 같은 동작 장면을 설명하는 라벨이 지정된 동영상 타임스탬프를 출력합니다.
- 학습된 분류 모델은 '경기 뷰', '청중 뷰'와 같은 사용자 정의 라벨이 지정된 자동으로 감지된 장면 세그먼트를 출력합니다.
- 학습된 객체 추적 모델은 축구 공 또는 선수가 나타나는 프레임의 경계 상자들로 구성된 해당 객체의 추적을 출력합니다.
Vertex AI 워크플로
Vertex AI는 표준 머신러닝 워크플로를 사용합니다.
- 데이터 수집: 달성하려는 결과를 바탕으로 모델을 학습 및 테스트하는 데 필요한 데이터를 결정합니다.
- 데이터 준비: 데이터의 형식 및 라벨이 올바른지 확인합니다.
- 학습: 매개변수를 설정하고 모델을 빌드합니다.
- 평가: 모델 측정항목을 검토합니다.
- 배포 및 예측: 모델을 사용할 수 있게 만듭니다.
데이터 수집을 시작하기 전에, 해결해야 하는 문제에 대해 생각해보면 데이터 요구사항을 알 수 있습니다.
데이터 준비
사용 사례 평가
문제부터 살펴봅니다. 달성하려는 결과는 무엇인가요?
이미지
데이터 세트를 구성할 때는 항상 사용 사례로 시작해야 합니다. 다음과 같은 질문으로 시작할 수 있습니다.
- 달성하려는 결과가 무엇인가요?
- 이 결과를 달성하기 위해 인식해야 하는 카테고리 또는 객체는 무엇인가요?
- 인간도 그러한 카테고리를 인식할 수 있나요? Vertex AI는 인간이 한 번에 기억하고 지정할 수 있는 것보다 훨씬 큰 규모의 카테고리를 처리할 수 있지만, 인간이 인식할 수 없는 카테고리라면 Vertex AI도 마찬가지로 어려움을 겪습니다.
- 시스템에서 보고 분류할 데이터 유형과 범위를 가장 잘 보여주는 예시는 무엇인가요?
테이블 형식
타겟 열은 어떤 데이터인가요? 얼마나 많은 데이터에 액세스할 수 있나요? 답변에 따라 Vertex AI에서 사용 사례를 해결하는 데 필요한 모델을 만듭니다.
- 이진 분류 모델은 이진 결과(2개 클래스 중 하나)를 예측합니다. 고객의 정기 결제 여부 예측처럼 예나 아니요로 대답하는 문제에는 이 모델을 사용합니다. 다른 조건이 동일하다면, 이진 분류 문제는 다른 모델 유형에 비해 적은 데이터를 요구합니다.
- 다중 클래스 분류 모델은 3개 이상의 개별 클래스 중 하나를 예측합니다. 이 모델은 대상을 분류할 때 사용합니다. 소매업체를 예로 든다면, 다중 클래스 분류 모델을 만들어 고객을 여러 페르소나로 구분할 수 있습니다.
- 예측 모델은 일련의 값을 예측합니다. 예를 들어 소매업체의 경우 앞으로 3개월 동안 일일 제품 수요를 예측하여 제품 인벤토리에 재고를 사전에 공급할 수 있습니다.
- 회귀 모델은 연속된 값을 예측합니다. 소매업체를 예로 든다면 회귀 모델을 빌드하여 고객이 다음 달에 지출할 금액을 예측할 수 있습니다.
텍스트
데이터 세트를 구성할 때는 항상 사용 사례로 시작해야 합니다. 다음과 같은 질문으로 시작할 수 있습니다.
- 달성하려는 결과가 무엇인가요?
- 이 결과를 달성하기 위해 인식해야 하는 카테고리 종류는 무엇인가요?
- 인간도 그러한 카테고리를 인식할 수 있나요? Vertex AI는 인간이 한 번에 기억하고 지정할 수 있는 것보다 더 많은 카테고리를 처리할 수 있지만, 인간이 인식할 수 없는 카테고리라면 Vertex AI도 마찬가지로 어려움을 겪습니다.
- 시스템이 분류할 데이터의 유형과 범위를 가장 잘 보여주는 예시는 무엇인가요?
동영상
달성하려는 결과에 따라 적절한 모델 목표를 선택합니다.
- 동영상에서 골을 넣거나 파울을 유발하거나 페널티킥과 같은 동작이 발생하는 순간을 감지하려면 동작 인식 목표를 사용하세요.
- TV 장면을 다음 카테고리와 같이 광고, 뉴스, TV 프로그램 등으로 분류하려면 분류 목표를 사용하세요.
- 동영상에서 객체를 찾고 추적하려면 객체 추적 목표를 사용하세요.
동작 인식, 분류, 객체 추적 목표를 위한 데이터 세트를 준비할 때 권장사항에 대한 자세한 내용은 동영상 데이터 준비를 참조하세요.
데이터 수집
사용 사례를 설정한 후에는 원하는 모델을 만들 수 있도록 데이터를 수집해야 합니다.
이미지
필요한 데이터가 결정되면 소싱 방법을 찾아야 합니다. 조직에서 수집하는 모든 데이터를 살펴보면서 시작할 수 있습니다. 이렇게 하면 모델 학습에 필요한 데이터가 이미 수집되고 있다는 것을 알 수도 있습니다. 데이터가 없으면 데이터를 직접 확보하거나 타사 제공업체에 아웃소싱할 수 있습니다.
라벨이 지정된 예제를 각 카테고리에 충분히 포함시키기
Vertex AI Training은 분류에 카테고리/라벨당 최소 100개의 이미지 예시가 필요합니다.
라벨을 성공적으로 인식할 가능성은 각각에 고품질 예시 수가 많을수록 증가합니다. 일반적으로 학습 프로세스에 라벨이 지정된 데이터를 많이 제공할수록 모델이 향상됩니다. 라벨당 1,000개 이상의 예시를 목표로 하세요.
카테고리별로 예시를 균등하게 배분하기
각 카테고리에 대체로 비슷한 개수의 학습 예시를 수집하는 것이 중요합니다. 한 라벨에 많은 양의 데이터가 있더라도 각 라벨에 균등하게 배분하는 것이 가장 좋습니다. 그 이유를 알아보기 위해 모델을 만드는 데 사용하는 이미지의 80%가 현대적 스타일의 단독 주택 사진이라고 가정하겠습니다. 이렇게 라벨이 불균형하게 분포되어 있으면 모델은 훨씬 덜 일반적인 라벨을 예측하는 위험을 감수하는 대신 사진이 항상 현대적인 단독 주택이라고 답하는 것이 안전하다고 학습할 가능성이 높습니다.
이는 거의 모든 정답이 'C'인 객관식 시험을 보는 것과 같습니다. 예리한 응시자는 곧 문제를 보지 않고도 매번 'C'라고 답하면 된다는 점을 알아차립니다.
각 라벨에 거의 동일한 수의 예시를 제공하는 것이 항상 가능하지 않을 수도 있습니다. 일부 카테고리에서는 고품질의 비편향적인 예시를 제공하기가 더 어려울 수 있습니다. 이러한 경우에는 예시가 가장 적은 라벨의 예시 수가 예시가 가장 많은 라벨의 예시 수의 10% 이상이어야 한다는 일반적인 원칙을 따르세요. 따라서 예시가 가장 많은 라벨의 예시가 10,000개라면 예시가 가장 적은 라벨의 예시는 최소 1,000개여야 합니다.
문제 공간의 다양성 포착
비슷한 이유로 데이터가 문제 공간의 다양성과 상이성을 포착하도록 해야 합니다. 모델 학습 과정에서 더 광범위한 예시를 접할수록 새로운 예시를 더 쉽게 일반화할 수 있게 됩니다. 예를 들어 소비자 전자제품 사진을 카테고리로 분류하려는 경우, 모델이 학습 과정에서 더 다양한 소비자 전자제품에 노출될수록 태블릿, 휴대전화, 노트북의 새로운 모델을 이전에 접해본 적이 없어도 더 잘 구분할 가능성이 높아집니다.
데이터를 모델에 의도된 출력과 매칭
예측을 수행할 이미지와 시각적으로 비슷한 이미지를 찾으세요. 눈덮인 겨울에 촬영한 집의 이미지를 분류하려는 경우, 화창한 날씨에 촬영한 집의 이미지로만 학습한 모델에서는 만족스러운 결과를 얻을 수 없습니다. 화창한 날씨의 이미지에 원하는 클래스의 태그를 지정했더라도 조명과 배경이 결과에 영향을 미칠 만큼 다르기 때문입니다. 학습 예시는 모델을 사용하여 분류하려는 데이터 세트에서 실제 데이터를 그대로 가져오는 것이 가장 좋습니다.
테이블 형식
사용 사례 작성이 끝나면, 모델을 학습시킬 데이터를 수집해야 합니다.
데이터 소스 설정과 준비는 머신러닝 모델 구축의 주요 단계입니다.
가용 데이터는 해결할 수 있는 문제가 무엇인지 알려주는 역할을 합니다. 가용 데이터가 어느 정도인가요? 데이터가 답을 얻으려는 질문과 관련이 있나요? 데이터를 수집할 때는 다음과 같은 핵심 사항을 고려해야 합니다.
관련 특성 선택
특성은 모델 학습에 사용하는 입력 속성입니다. 특성은 모델이 패턴을 식별해 예측을 수행하는 방식이며, 따라서 문제와 밀접한 관련이 있어야 합니다. 예를 들어 신용카드 거래 사기 여부를 예측하는 모델을 만들려면 구매자, 판매자, 금액, 날짜와 시간, 구매한 품목 같은 거래 내역이 포함된 데이터 세트를 작성해야 합니다. 구매자와 판매자의 기존 내역, 구매한 품목의 사기 빈도 등도 유용한 특성에 속합니다. 다른 관련 특성에는 무엇이 있을까요?
소개에서 다룬 소매업체 이메일 마케팅 사용 사례를 생각해 보세요. 이때는 아래와 같은 특성 열이 필요합니다.
- 구매한 품목 목록(브랜드, 카테고리, 가격, 할인 포함)
- 구매한 품목 개수(지난 요일, 주, 월, 년)
- 지출 금액 합계(지난 요일, 주, 월, 년)
- 각 품목에 대해 매일 판매되는 총 수량
- 품목마다 일일 재고 총 수량
- 특정 날짜에 프로모션을 진행 여부
- 쇼핑객의 확인된 인구통계 프로필
충분한 데이터 포함
일반적으로 학습 예시가 많을수록 좋은 결과가 나옵니다. 그리고 해결하려는 문제가 복잡할수록 필요한 데이터의 양도 늘어납니다. 다중 클래스 모델과 비교하면 이진 분류 모델은 데이터가 많지 않아도 정확한 모델을 얻을 수 있습니다. 다중 클래스가 아닌 클래스 2개 중에서 하나를 예측하는 일은 훨씬 단순하기 때문입니다.
완벽한 수식은 존재하지 않지만 권장되는 예시 데이터 최소 기준은 있습니다.
- 분류 문제: 50개 행 x 특성 수
- 예측 문제:
- 5,000개 행 x 특성 수
- 시계열 식별자 열의 고유한 값 10개 x 특성 수
- 회귀 문제: 특성 수의 200배
편차 포착
데이터 세트는 문제 공간의 다양성을 포착해야 합니다. 학습 중에 모델이 다양한 예시를 확인할수록, 새롭거나 덜 일반적인 예시를 쉽게 일반화할 수 있습니다. 소매업체 모델을 겨울철 구매 데이터만으로 학습시켰다고 상상해 보세요. 여름 의류 선호도나 구매 행동을 제대로 예측할 수 있을까요?
텍스트
필요한 데이터가 결정되면 소싱 방법을 찾아야 합니다. 먼저 조직에서 수집하는 모든 데이터를 살펴보는 것으로 시작할 수 있습니다. 이렇게 하면 모델 학습에 필요한 데이터를 이미 수집하고 있었다는 것을 알게 될 수도 있습니다. 필요한 데이터가 없는 경우 직접 확보하거나 타사 제공업체에 아웃소싱할 수 있습니다.
라벨이 지정된 예제를 각 카테고리에 충분히 포함시키기
라벨을 제대로 인식할 가능성은 고품질 예시의 수가 많을수록 증가합니다. 일반적으로 학습 프로세스에 라벨이 지정된 데이터가 많이 제공될수록 모델이 향상됩니다. 필요한 샘플 수는 예측하려는 데이터의 일관성과 대상 정확도 수준에 따라 달라집니다. 일관된 데이터 세트의 예시를 더 적게 사용하거나 97% 정확성 대신 80% 정확성을 달성할 수 있습니다.
모델을 학습한 후 결과를 평가합니다. 더 많은 예를 추가하고 정확도 목표를 달성할 때까지 재교육합니다(라벨당 수백 또는 수천 개의 예시가 필요할 수 있음). 데이터 요구사항 및 권장사항에 대한 자세한 내용은 AutoML 모델의 텍스트 학습 데이터 준비를 참조하세요.
카테고리별로 예시를 균등하게 배분하기
각 카테고리에 대체로 비슷한 개수의 학습 예시를 수집하는 것이 중요합니다. 한 라벨에 많은 양의 데이터가 있더라도 각 라벨에 균등하게 배분하는 것이 가장 좋습니다. 그 이유를 알아보기 위해 모델을 만드는 데 사용하는 고객 의견 중 80%가 견적 요청이라고 가정해 보겠습니다. 모델은 이렇게 불균형적인 라벨 배분으로 인해 일반적이지 않은 라벨을 예측하려고 시도하기 보다는 고객 의견이 견적 요청이라고 말하는 것이 항상 안전하다고 학습하게 될 가능성이 높습니다. 이는 거의 모든 정답이 'C'인 객관식 시험을 보는 것과 같습니다. 예리한 응시자는 곧 문제를 보지 않고도 매번 'C'라고 답하면 된다는 점을 알아차립니다.
각 라벨에 거의 동일한 수의 예시를 제공하는 것이 항상 가능하지 않을 수도 있습니다. 일부 카테고리에서는 고품질의 비편향적인 예시를 제공하기가 더 어려울 수 있습니다. 이러한 경우에는 예시가 가장 적은 라벨의 예시 수가 예시가 가장 많은 라벨의 예시 수의 10% 이상이어야 합니다. 따라서 예시가 가장 많은 라벨의 예시가 10,000개라면 예시가 가장 적은 라벨의 예시는 최소 1,000개여야 합니다.
문제 공간의 다양성 포착
비슷한 이유로 데이터가 문제 공간의 다양성과 상이성을 포착하도록 시도하세요. 모델은 더 넓은 범위의 예시를 제공하면 쉽게 새 데이터로 일반화할 수 있습니다. 예를 들어 소비자 가전에 관한 기사를 주제별로 분류한다고 가정해 보겠습니다. 브랜드 이름과 기술 사양을 많이 입력할수록 모델은 학습 세트에 없는 브랜드에 관한 기사라 하더라도 더 쉽게 기사의 주제를 파악할 수 있습니다. 정의된 라벨과 일치하지 않는 문서에 'none_of_the_above' 라벨을 지정해 모델 성능을 향상시키는 방법도 고려할 수 있습니다.
데이터를 모델에 의도된 출력과 매칭
예측하려는 텍스트와 유사한 텍스트 예시를 찾아보세요. 유리 세공에 관한 소셜 미디어 게시물을 분류하려는 경우, 유리 세공 정보 웹사이트에서 학습한 모델에서는 만족스러운 결과를 얻을 수 없습니다. 이는 어휘와 스타일이 결과에 영향을 미친 만큼 다르기 때문입니다. 학습 예시는 모델을 사용하여 분류하려는 데이터 세트에서 실제 데이터를 그대로 가져오는 것이 가장 좋습니다.
동영상
사용 사례를 설정한 후에는 원하는 모델을 만들 수 있는 동영상 데이터를 수집해야 합니다. 학습을 위해 수집하는 데이터를 통해 해결할 수 있는 문제를 알 수 있습니다. 얼마나 많은 동영상을 사용할 수 있나요? 동영상에 모델에서 예측하려는 예시가 충분히 포함되어 있나요? 동영상 데이터를 수집하는 동안 다음 사항을 고려해야 합니다.
충분한 동영상 포함
일반적으로 데이터 세트에 학습 동영상이 많을수록 더 나은 결과를 얻을 수 있습니다. 또한 권장되는 동영상 수는 해결하려는 문제의 복잡성에 따라 조정됩니다. 예를 들어 이진 분류 문제(2개 클래스에서 하나의 클래스 예측)의 경우에는 다중 라벨 문제(여러 클래스에서 하나 이상의 클래스 예측)에 비해 동영상 데이터가 덜 필요합니다.
수행하려는 작업의 복잡성에 따라 필요한 동영상 데이터의 양도 결정할 수 있습니다. 동작 장면을 구별하기 위해 모델을 빌드하는 축구 사용 사례와 다른 스타일의 수영을 분류할 수 있는 모델을 학습시키는 것을 비교해서 생각해보세요. 예를 들어 평영, 접영, 배영 등을 구별하려면 모델이 각 유형을 정확하게 식별하는 방법을 학습하는 데 도움이 되도록 다양한 수영 스타일을 식별하는 더 많은 학습 데이터가 필요합니다. 동작 인식, 분류, 객체 추적에 필요한 동영상 데이터에 대한 최소 요구사항을 이해하려면 동영상 데이터 준비를 참조하세요.
필요한 동영상 데이터가 현재 보유하고 있는 동영상 데이터보다 많을 수 있습니다. 타사 제공업체를 통해 더 많은 동영상을 확보하세요. 예를 들어 경기 동작 식별자 모델에 축구 동영상이 충분하지 않은 경우 더 많은 축구 동영상을 구입하거나 확보할 수 있습니다.
클래스별로 동영상을 균등하게 배분
클래스마다 유사한 수의 학습 예를 제공하세요. 그 이유는 다음과 같습니다.
학습 데이터 세트의 80%가 골 장면이 나오는 축구 동영상이고, 20%만이 신체 접촉 반칙 또는 페널티킥이 나오는 동영상이라고 가정해 보겠습니다. 이렇게 클래스에 균등하지 않게 배분되는 경우 모델에서 주어진 동작을 골로 예측할 가능성이 높아집니다. 이는 정답의 80%가 'C'인 객관식 시험을 보는 것과 비슷합니다. 예리한 모델은 대부분 'C'가 꽤 괜찮은 추측임을 알아차립니다.
각 클래스에 동일한 수의 동영상을 제공하는 것이 가능하지 않을 수 있습니다. 일부 클래스의 경우 고품질의 비편향적인 예를 제공하기가 더 어려울 수 있습니다. 1:10 비율을 사용하세요. 즉, 동영상 예가 가장 많은 클래스의 예가 10,000개라면 동영상 예가 가장 적은 클래스의 예는 최소 1,000개여야 합니다.
편차 포착
동영상 데이터는 문제 공간의 다양성을 포착해야 합니다. 학습 중에 모델이 다양한 예시를 확인할수록, 새롭거나 덜 일반적인 예시를 쉽게 일반화할 수 있습니다. 축구 동작 분류 모델에 대해 생각해보세요. 동영상에 다양한 카메라 앵글, 주야간, 선수들의 다양한 동작을 포함해야 합니다. 모델을 다양한 데이터에 노출하면 모델에서 동작 간에 구별할 수 있는 기능이 향상됩니다.
데이터를 예상된 출력과 매칭

예측할 모델에 입력하려는 동영상과 시각적으로 비슷한 학습 동영상을 찾습니다. 예를 들어 모든 학습 동영상을 겨울 또는 저녁에 촬영한 경우 해당 환경의 조명과 색상 패턴이 모델에 영향을 미치게 됩니다. 해당 모델을 사용하여 여름 또는 낮에 촬영한 동영상을 테스트하는 경우 정확하게 예측하지 못할 수 있습니다.
다음과 같은 추가적인 요소를 고려해보세요. 동영상 해상도, 초당 동영상 프레임 수, 카메라 앵글, 배경.
데이터 준비
이미지
수동 또는 기본 분할 중에서 적합한 방식을 결정한 후 다음 방법 중 하나를 사용하여 Vertex AI에 데이터를 추가할 수 있습니다.
- 라벨(필요한 경우 경계 상자) 인라인을 사용하여 컴퓨터 또는 Cloud Storage 중에서 사용 가능한 형식(CSV 또는 JSON Lines)으로 데이터를 가져올 수 있습니다. 가져오기 파일 형식에 대한 자세한 내용은 학습 데이터 준비를 참조하세요. 데이터 세트를 수동으로 분할하려면 CSV 또는 JSON Lines 가져오기 파일에 분할을 지정하면 됩니다.
- 데이터에 아직 주석이 추가되지 않은 경우 라벨이 지정되지 않은 이미지를 업로드하고 Google Cloud Console을 사용하여 주석을 적용할 수 있습니다. 동일한 이미지 모음의 여러 주석 세트에서 이러한 주석을 관리할 수 있습니다. 예를 들어 단일 이미지 모음의 경우 경계 상자 및 라벨 정보가 있는 주석 세트를 사용하여 객체 감지를 수행할 수 있으며 분류를 위한 라벨 주석만 있는 또 다른 주석 세트가 있을 수 있습니다.
테이블 형식
가용 데이터를 식별한 후에는 데이터를 학습에 사용할 수 있는지 확인해야 합니다.
데이터가 편향되거나 누락 또는 잘못된 값이 있으면 이는 모델 품질에 영향을 미칩니다. 모델 학습을 시작하기 전에 다음 사항을 고려하세요.
자세히 알아보기
데이터 유출 및 학습/서빙 편향 방지
데이터 유출은 예측 타겟 정보를 '유출'하는 입력 특성을 학습 중에 사용하는 것을 의미하며 실제 모델 서빙 시에는 사용할 수 없습니다. 데이터 유출은 타겟 열과 상관관계가 밀접한 특성이 입력 특성 중 하나에 있을 때 감지됩니다. 예를 들어 고객의 익월 구독 신청 여부를 예측하는 모델을 제작 중이며 입력 특성 중 하나가 고객의 향후 구독 결제라고 가정해 보겠습니다. 이때 모델은 테스트 과정에서는 강력한 성능을 발휘하지만 프로덕션 단계에 배포했을 때는 그렇지 못합니다. 서빙 시점에서는 향후 구독 결제 정보를 사용할 수 없기 때문입니다.
학습/서빙 편향은 학습 시점에서 사용한 입력 특성이 서빙 시점에 모델에 제공한 특성과 달라 프로덕션 단계에서 모델 품질이 떨어지는 현상을 말합니다. 시간별 기온을 예측하는 모델을 만드는데 주간 온도만 있는 데이터로 학습하는 상황이 여기에 속합니다. 학생 중퇴를 예측할 때 학습 데이터에 항상 학생의 성적을 입력했지만 서빙 시점에서는 입력하지 않은 경우도 마찬가지입니다.
데이터 유출과 학습/서빙 편향을 방지하려면 학습 데이터를 이해해야 합니다.
- 데이터를 사용하기 전에 데이터의 의미와 데이터를 특성으로 사용할지 여부를 파악합니다.
- 학습 탭에서 상관관계를 확인합니다. 높은 상관관계는 검토가 필요하다고 표시해야 합니다.
- 학습/서빙 편향: 서빙 시점에서 사용한 것과 정확하게 같은 형식의 입력 특성만 제공해야 합니다.
누락, 불완전, 비일관적 데이터 삭제
예시 데이터에는 자주 값이 누락되거나 부정확한 값이 존재합니다. 가능하다면 학습에 사용하기 전에 시간을 내 데이터를 검토하고 품질을 개선하세요. 누락된 값이 많을수록 머신러닝 모델 학습에서의 데이터 효과가 떨어집니다.
- 데이터에 누락된 값이 있는지 확인하고 가능하면 수정하세요. 또는 열을 null 허용으로 설정한 경우에는 값을 비워 두세요. Vertex AI는 누락된 값을 처리할 수 있지만 모든 값을 사용할 수 있을 때 최적의 결과를 얻을 가능성이 더 높습니다.
- 예측의 경우 학습 행 간격이 일관적인지 확인하세요. Vertex AI는 누락된 값을 귀속시킬 수 있지만 모든 행을 사용할 수 있을 때 최적의 결과를 얻을 가능성이 더 높습니다.
- 오류나 노이즈를 수정 또는 제거해 데이터를 정리하세요. 데이터의 일관성을 유지하세요. 철자, 약어, 서식을 확인해야 합니다.
가져온 후 데이터 분석
Vertex AI는 데이터 세트를 가져오면 데이터 세트의 개요를 제공합니다. 가져온 데이터 세트를 검토해 각 열의 변수 유형이 올바른지 확인하세요. Vertex AI는 열 값에 따라 변수 유형을 자동으로 감지하지만 각 유형을 검토하는 것이 가장 좋습니다. 각 열의 Null 허용 여부도 검토해야 합니다. 이 항목은 누락되거나 NULL인 값이 열에 있어도 되는지를 결정합니다.
텍스트
수동 또는 기본 분할 중에서 적합한 방식을 결정한 후 다음 방법 중 하나를 사용하여 Vertex AI에 데이터를 추가할 수 있습니다.
- 학습 데이터 준비에 나와 있는 대로 컴퓨터나 Cloud Storage에서 데이터를 인라인 라벨이 있는 CSV 또는 JSON Lines 형식으로 가져올 수 있습니다. 데이터 세트를 수동으로 분할하려면 CSV 또는 JSON Lines 파일에 분할을 지정하면 됩니다.
- 데이터에 라벨이 지정되지 않았으면 라벨이 지정되지 않은 텍스트 예시를 업로드하고 Vertex AI 콘솔을 사용하여 라벨을 적용할 수 있습니다.
동영상
데이터 세트에 포함할 동영상을 수집한 후에는 동영상에 동영상 세그먼트 또는 경계 상자와 관련된 라벨이 포함되어 있는지 확인해야 합니다. 동작 인식의 경우 동영상 세그먼트는 타임스탬프이고 세그먼트 분류는 동영상 장면, 세그먼트 또는 전체 동영상일 수 있습니다. 객체 추적의 경우 라벨은 경계 상자와 연결됩니다.
동영상에 경계 상자와 라벨이 필요한 이유는 무엇인가요?
객체 추적의 경우 Vertex AI 모델은 패턴을 식별하는 방법을 어떻게 학습하나요? 이것이 바로 경계 상자와 라벨이 학습 중에 수행하는 일입니다. 축구 예를 살펴보세요. 각 동영상 예에는 감지하려는 객체 주변에 경계 상자가 있어야 합니다. 또한 해당 상자에는 '사람', '공'과 같은 라벨이 할당되어 있어야 합니다. 그렇지 않으면 찾는 내용을 모델에서 알 수 없습니다. 상자를 그리고 라벨을 동영상 예에 할당하는 데 시간이 걸릴 수 있습니다.
데이터에 아직 라벨이 지정되지 않았으면 라벨이 지정되지 않은 동영상을 업로드하고 Google Cloud 콘솔을 사용하여 경계 상자 및 라벨을 적용할 수도 있습니다. 자세한 내용은 Google Cloud 콘솔을 사용하여 데이터 라벨 지정을 참조하세요.
모델 학습
이미지
커스텀 모델 생성 시 Vertex AI가 데이터 세트를 사용하는 방법 고려
데이터 세트에는 학습, 검증, 테스트 세트가 있습니다. 분할을 지정하지 않으면(데이터 준비 참조) Vertex AI에서 자동으로 이미지의 80%를 학습에, 10%를 검증에, 10%를 테스트에 사용합니다.
학습 세트
데이터의 대부분은 학습 세트에 있어야 합니다. 이러한 데이터는 모델이 학습 중에 '보는' 데이터이며 모델의 매개변수, 즉 신경망 노드 간의 연결 가중치를 학습하는 데 사용됩니다.
검증 세트
'개발' 세트라고도 하는 검증 세트가 학습 프로세스에 사용됩니다.
모델 학습 프레임워크는 학습 프로세스가 반복될 때마다 학습 데이터를 반영한 후, 검증 세트에 대한 모델의 성능을 사용하여 모델의 초매개변수, 즉 모델의 구조를 지정하는 변수를 조정합니다. 학습 세트를 사용하여 초매개변수를 조정하려고 시도하면 모델이 학습 데이터에 지나치게 집중하고, 정확하게 일치하지 않는 예시를 일반화하는 데 어려움을 겪을 가능성이 높습니다.
어느 정도 새로운 데이터 세트를 사용하여 모델을 세밀하게 조정하면 모델의 일반화 성능도 개선됩니다.
테스트 세트
테스트 세트는 학습 프로세스에 전혀 관여하지 않습니다. 모델이 학습을 모두 완료하면 테스트 세트를 모델을 위한 완전히 새로운 도전과제로 사용합니다. 테스트 세트에서 측정된 모델 성능을 통해 모델이 실제 데이터에서 어떤 성능을 보일지를 상당히 가늠할 수 있습니다.
수동 분할
데이터 세트를 직접 분할할 수도 있습니다. 데이터 수동 분할은 프로세스를 더욱 세분화하여 제어해야 하는 경우 또는 모델 학습 주기의 특정 부분에 포함하려는 특정 예시가 있는 경우 적절한 선택입니다.
테이블 형식
데이터 세트 가져오기가 끝나면, 이제 모델을 학습시켜야 합니다. Vertex AI는 학습 기본값으로도 안정적인 머신러닝 모델을 만들지만, 사용 사례에 맞게 매개변수 일부를 조정해도 됩니다.
학습에 적용할 열을 최대한 많이 선택하고, 각 열이 학습용으로 적절하게 확인하세요. 특성을 선택할 때는 다음 사항에 유의해야 합니다.
- 각 행에 고유한 값이 있는 무작위로 할당된 식별자 열처럼, 노이즈를 만드는 특성 열을 선택하면 안 됩니다.
- 각 특성 열과 열의 값을 이해하고 있는지 확인하세요.
- 같은 데이터 세트에서 여러 모델을 만든다면 현재 예측 문제에 속하지 않는 타겟 열은 제거해야 합니다.
- 공정성 원칙을 떠올리세요. 소외된 그룹에 대한 편향되거나 공정하지 못한 의사 결정을 이끄는 특성으로 모델을 학습시키면 안 됩니다.
Vertex AI가 데이터 세트를 사용하는 방법
데이터 세트는 학습, 검증, 테스트 세트로 구분됩니다. 기본 Vertex AI가 적용되는 기본 분할은 학습 중인 모델 유형에 따라 다릅니다. 필요한 경우 분할(수동 분할)을 지정할 수도 있습니다. 자세한 내용은 AutoML 모델의 데이터 분할 정보를 참조하세요.
학습 세트
데이터의 대부분은 학습 세트에 있어야 합니다. 이러한 데이터는 모델이 학습 중에 '보는' 데이터이며 모델의 매개변수, 즉 신경망 노드 간의 연결 가중치를 학습하는 데 사용됩니다.
검증 세트
'개발' 세트라고도 하는 검증 세트가 학습 프로세스에 사용됩니다.
모델 학습 프레임워크는 학습 프로세스가 반복될 때마다 학습 데이터를 반영한 후, 검증 세트에 대한 모델의 성능을 사용하여 모델의 초매개변수, 즉 모델의 구조를 지정하는 변수를 조정합니다. 학습 세트를 사용하여 초매개변수를 조정하려고 시도하면 모델이 학습 데이터에 지나치게 집중하고, 정확하게 일치하지 않는 예시를 일반화하는 데 어려움을 겪을 가능성이 높습니다.
어느 정도 새로운 데이터 세트를 사용하여 모델을 세밀하게 조정하면 모델의 일반화 성능도 개선됩니다.
테스트 세트
테스트 세트는 학습 프로세스에 전혀 관여하지 않습니다. 모델이 학습을 모두 끝내면 Vertex AI는 테스트 세트를 모델을 위한 완전히 새로운 과제로 사용합니다.
테스트 세트에서 측정된 모델 성능을 통해 모델이 실제 데이터에서 어떤 성능을 보일지를 상당히 가늠할 수 있습니다.
텍스트
Vertex AI가 데이터 세트를 사용해 커스텀 모델을 만드는 방법 고려
데이터 세트에는 학습, 검증, 테스트 세트가 있습니다. Vertex AI는 분할을 지정하지 않으면(데이터 준비 참조) 자동으로 콘텐츠 문서 80%를 학습에, 10%를 검증에, 10%를 테스트에 사용합니다.
학습 세트
데이터의 대부분은 학습 세트에 있어야 합니다. 이러한 데이터는 모델이 학습 중에 '보는' 데이터이며 모델의 매개변수, 즉 신경망 노드 간의 연결 가중치를 학습하는 데 사용됩니다.
검증 세트
'개발' 세트라고도 하는 검증 세트가 학습 프로세스에 사용됩니다.
모델 학습 프레임워크는 학습 프로세스가 반복될 때마다 학습 데이터를 반영한 후, 검증 세트에 대한 모델의 성능을 사용하여 모델의 초매개변수, 즉 모델의 구조를 지정하는 변수를 조정합니다. 학습 세트를 사용하여 초매개변수를 조정하려고 시도하면 모델이 학습 데이터에 지나치게 집중하고, 정확하게 일치하지 않는 예시를 일반화하는 데 어려움을 겪을 가능성이 높습니다.
어느 정도 새로운 데이터 세트를 사용하여 모델을 세밀하게 조정하면 모델의 일반화 성능도 개선됩니다.
테스트 세트
테스트 세트는 학습 프로세스에 전혀 관여하지 않습니다. 모델이 학습을 모두 완료하면 테스트 세트를 모델을 위한 완전히 새로운 도전과제로 사용합니다. 테스트 세트에서 측정된 모델 성능을 통해 모델이 실제 데이터에서 어떤 성능을 보일지를 상당히 가늠할 수 있습니다.
수동 분할
데이터 세트를 직접 분할할 수도 있습니다. 데이터 수동 분할은 프로세스를 더욱 세분화하여 제어해야 하는 경우 또는 모델 학습 주기의 특정 부분에 포함하려는 특정 예시가 있는 경우 적절한 선택입니다.
동영상
학습 동영상 데이터가 준비되면 머신러닝 모델을 만들 준비가 된 것입니다. 같은 데이터 세트에서 다양한 모델 목표에 대한 주석 세트를 만들 수 있습니다. 주석 세트 만들기를 참조하세요.
Vertex AI의 이점 중 하나는 기본 매개변수로 신뢰할 수 있는 머신러닝 모델을 만들 수 있다는 점입니다. 그러나 데이터 품질과 원하는 결과에 따라 매개변수를 조정해야 할 수 있습니다. 예를 들면 다음과 같습니다.
- 예측 유형은 동영상이 처리되는 세분화 수준입니다.
- 프레임 속도는 동작 인식과 같이 분류하려는 라벨이 모션 변화에서 민감할 경우 중요합니다. 예를 들어 달리기 및 걷기을 적용합니다. 낮은 초당 프레임 수(FPS) 걷기 클립은 달리기처럼 보일 수 있습니다. 객체 추적의 경우 프레임 속도 역시 민감합니다. 기본적으로 추적 대상 객체는 인접한 프레임 간에 충분히 중첩되어야 합니다.
- 객체 추적의 해상도는 동작 인식 또는 동영상 분류보다 중요합니다. 객체가 작은 경우 고해상도 동영상을 업로드해야 합니다. 현재 파이프라인은 일반 학습에 256x256을 사용하고 사용자 데이터에 작은 객체(이미지 영역의 1% 미만)가 너무 적은 경우 512x512를 사용합니다. 최소 256p 이상의 동영상을 사용하는 것이 좋습니다. 내부적으로 동영상 프레임이 다운샘플링되어 학습 및 추론 속도를 높이기 때문에 해상도가 높은 동영상을 사용하면 모델 성능을 향상시키는 데 도움이 되지 않을 수 있습니다.
모델 평가, 테스트, 배포
모델 평가
이미지
모델이 학습되면 모델 성능에 대한 요약을 받게 됩니다. 평가 또는 전체 평가 보기를 클릭하여 자세한 분석을 보세요.
모델 디버깅은 모델 자체보다 데이터 디버깅에 관한 것입니다. 프로덕션으로 진행하기 전후에 성능을 평가할 때 어느 시점에서 모델이 예상하지 못한 방식으로 작동하기 시작하면 되돌아가서 데이터를 점검하여 어느 부분을 개선할 수 있는지 확인해야 합니다.
Vertex AI에서 어떤 종류의 분석을 수행할 수 있나요?
Vertex AI 평가 섹션에서는 테스트 예시를 사용한 모델 출력과 머신러닝 일반 측정항목을 사용해 커스텀 모델의 성능을 평가할 수 있습니다. 이 섹션에서는 각 개념의 의미를 설명합니다.
- 모델 출력
- 점수 임곗값
- 참양성, 참음성, 거짓양성, 거짓음성
- 정밀도와 재현율
- 정밀도/재현율 곡선
- 평균 정밀도
모델 출력은 어떻게 해석하나요?
Vertex AI는 테스트 데이터의 예시를 가져와서 모델에 완전히 새로운 과제를 제시합니다. 각 예시에서 모델은 각 라벨이 해당 예시와 얼마나 강한 연관성이 있는지 나타내는 일련의 숫자를 출력합니다. 출력된 숫자가 높을수록 해당 라벨이 문서에 적용되어야 하는 것으로 모델이 확신한다는 뜻입니다.
점수 임곗값이란 무엇인가요?
점수 임곗값을 설정하여 이러한 확률을 'on'/'off'라는 이진값으로 변환할 수 있습니다.
점수 임곗값은 테스트 항목에 카테고리를 지정하기 위해 모델이 가져야 하는 신뢰도 수준을 의미합니다. Google Cloud 콘솔에 있는 점수 임곗값 슬라이더는 데이터세트의 모든 카테고리와 개별 카테고리에서 다양한 임곗값의 영향을 테스트하기 위한 시각적 도구입니다.
점수 임곗값이 낮으면 모델이 더 많은 이미지를 분류하지만 프로세스에서 일부 이미지를 잘못 분류할 위험이 있습니다. 점수 임곗값이 높으면 모델이 분류하는 이미지 수는 적지만 이미지를 잘못 분류할 위험이 더 낮아집니다. Google Cloud 콘솔에서 카테고리별로 임곗값을 조정하면서 시험해볼 수 있습니다. 하지만 프로덕션에서 모델을 사용할 때는 자신이 최적이라고 판단한 임곗값을 적용해야 합니다.
참양성, 참음성, 거짓양성, 거짓음성이란 무엇인가요?
점수 임곗값을 적용한 후에 모델에 의해 작성된 예측은 다음 네 가지 카테고리 중 하나에 해당합니다.
자신이 최적이라고 판단한 임곗값입니다.
이러한 카테고리를 사용해 모델의 효과를 평가할 수 있는 측정항목인 정밀도와 재현율을 계산할 수 있습니다.
정밀도와 재현율이란 무엇인가요?
정밀도와 재현율은 모델이 정보를 얼마나 잘 포착하고 있는지와 정보를 얼마나 놓치고 있는지를 이해하는 데 도움을 줍니다. 정밀도는 라벨이 지정된 전체 테스트 예시 중에서 실제로 해당 라벨로 카테고리가 지정되어야 했던 예시가 몇 개인지를 알려줍니다. 재현율은 라벨이 지정되어야 했던 전체 테스트 예시 중에서 실제로 해당 라벨이 지정된 예시가 몇 개인지를 알려줍니다.
정밀도나 재현율 중에서 무엇을 기준으로 최적화해야 하나요?
정밀도나 재현율 중에서 무엇을 기준으로 최적화할지는 사용 사례에 따라 달라집니다. 가장 적합한 접근 방식을 결정할 때는 다음 두 가지 사용 사례를 고려합니다.
사용 사례: 이미지의 개인정보 보호
예를 들어 민감한 정보를 자동으로 감지하여 흐리게 처리하는 시스템을 만든다고 가정해 보겠습니다.
이 경우 거짓 양성은 흐리게 처리될 필요가 없는 대상이 흐리게 처리되는 것으로, 성가시기는 하지만 문제가 되지는 않습니다.
이 경우 거짓음성은 신용카드와 같이 흐리게 처리되어야 하는 대상이 흐리게 처리되지 않는 것으로, 신원 도용으로 이어질 수 있습니다.
이러한 경우 재현율을 기준으로 최적화하는 것이 좋습니다. 이 측정항목은 예측 전체에서 얼마나 누락되었는지를 측정합니다. 고재현율 모델은 관련성이 적은 예시에 라벨을 지정할 가능성이 높습니다. 이 방법은 카테고리에 학습 데이터가 별로 없는 경우에 유용합니다.
사용 사례: 스톡 사진 검색
특정 키워드에 가장 적합한 스톡 사진을 검색하는 시스템을 만들려는 경우를 가정해 보겠습니다.
이 경우 거짓양성은 관련이 없는 이미지를 반환하는 것입니다. 가장 일치하는 이미지만 반환하는 제품을 만들려고 하므로 이는 심각한 오류입니다.
이 경우 거짓음성은 키워드 검색과 관련 있는 이미지를 반환하지 못하는 것입니다.
일치 가능성이 높은 사진이 수천 장에 달하는 검색어가 많으므로, 이러한 문제는 괜찮습니다.
이 경우 정밀도 최적화가 좋습니다. 이 측정항목은 예측이 전체적으로 얼마나 정확한지를 측정합니다. 고정밀도 모델은 가장 관련성이 높은 예시만 라벨을 지정할 가능성이 높으므로, 해당 클래스가 학습 데이터에서 일반적인 경우에 유용합니다.
혼동 행렬은 어떻게 사용하나요?
정밀도-재현율 곡선은 어떻게 해석하나요?
점수 임곗값 도구를 사용하면 선택한 점수 임곗값이 정밀도와 재현율에 어떤 영향을 주는지 살펴볼 수 있습니다. 점수 임곗값 바의 슬라이더를 드래그하면 임곗값에 따라 변하는 정밀도-재현율 곡선 상의 위치뿐만 아니라 임곗값이 정밀도와 재현율에 개별적으로 미치는 영향을 확인할 수 있습니다(다중 클래스 모델의 경우, 이 그래프의 정밀도와 재현율은 반환되는 라벨 집합에서 점수가 가장 높은 라벨이 정밀도 및 재현율 측정항목 계산에 유일하게 사용되는 라벨임을 말해 줍니다.) 이렇게 하면 거짓양성과 거짓음성의 균형을 잘 잡을 수 있습니다.
모델 전체에 적용할 수 있는 임곗값을 선택한 후 각 라벨을 클릭하여 해당 임곗값이 라벨별 정밀도-재현율 곡선에서 어느 위치에 있는지 확인합니다. 몇몇 라벨에만 잘못된 예측을 많이 하도록 임곗값이 정해지는 경우가 있습니다. 이럴 때 라벨별로 곡선을 보면 이런 라벨에 맞춰 클래스별 임곗값을 선택하는 데 도움이 될 수 있습니다. 예를 들어 주택 데이터 세트에서 임곗값 0.5가 모든 이미지 유형에 적정한 정밀도와 재현율을 나타내며 예외적으로 '튜더'는 아주 일반적인 카테고리이므로 그렇지 않다고 가정해 보겠습니다. 이 카테고리에는 거짓양성이 많이 있습니다. 이 경우 예측을 위한 분류 기준을 호출할 때 '튜더' 카테고리에만 임곗값 0.8을 사용할 수 있습니다.
평균 정밀도란 무엇인가요?
모델 정확도를 측정하는 유용한 항목은 정밀도-재현율 곡선 아래에 있는 면적으로, 점수 임곗값 전체에 대비 모델 성능을 나타냅니다. Vertex AI에서는 이 측정항목은 평균 정밀도라고 합니다. 이 점수가 1.0에 가까울수록 테스트 세트에 대한 모델의 성능이 높습니다. 각 라벨에 대해 무작위로 추측하는 모델의 평균 정밀도는 약 0.5 수준입니다.
테이블 형식
모델 학습이 끝나면 성능에 대한 요약을 받게 됩니다. 모델 평가 측정항목은 데이터 세트의 일부(검증 데이터 세트)에 대한 모델의 성능을 기반으로 합니다. 실제 데이터에서 모델을 사용할 준비가 되었는지 결정할 때는 몇 가지 주요 측정항목과 개념을 고려해야 합니다.
분류 측정항목
점수 임곗값
고객의 내년 재킷 구매를 예측하는 머신러닝 모델을 생각해 보세요. 특정 고객의 재킷 구매를 예측하려면 모델이 얼마나 확실해야 할까요? 분류 모델에서는 각 예측에 신뢰도 점수가 할당됩니다. 이 점수는 예측한 클래스가 올바른지에 대한 모델의 확실성을 숫자로 평가한 것입니다. 점수 임곗값은 해당 점수를 예 또는 아니요로 판단하는 기준을 결정하는 숫자입니다. 즉, 모델이 '예, 이 신뢰도 점수는 고객이 내년에 외투를 구매한다고 결론을 내릴 정도로 충분히 높습니다.'라고 예측하는 값입니다.
점수 임곗값이 낮으면 모델이 분류를 잘못할 위험이 있습니다. 따라서 점수 임곗값은 해당 사용 사례를 기반으로 해야 합니다.
예측 결과
점수 임곗값을 적용한 후에 모델의 예측은 4가지 카테고리 중 하나에 해당합니다. 이러한 카테고리를 이해하려면 재킷 이진 분류 모델을 다시 떠올려 보세요. 이 예시에서는 포지티브 클래스(모델이 예측하려 하는 클래스)가 '고객이 내년에 재킷을 구매할 것이다'가 됩니다.
- 참양성: 모델이 포지티브 클래스를 정확하게 예측합니다. 모델이 고객이 재킷을 구매한다고 정확하게 예측했다는 뜻입니다.
- 거짓양성: 모델이 포지티브 클래스를 잘못 예측합니다. 모델이 고객이 재킷을 구매한다고 예측했지만 실제로는 그렇지 않았다는 뜻입니다.
- 참음성: 모델이 네거티브 클래스를 정확하게 예측합니다. 모델이 고객이 재킷을 구매하지 않는다고 정확하게 예측했다는 뜻입니다.
- 거짓음성: 모델이 네거티브 클래스를 잘못 예측합니다. 모델이 고객이 재킷을 구매하지 않는다고 예측했지만 실제로는 그렇지 않았다는 뜻입니다.
정밀도와 재현율
정밀도와 재현율 측정항목은 모델이 정보를 얼마나 잘 포착하고 있는지와 정보를 얼마나 놓치고 있는지를 이해하는 데 도움을 줍니다. 정밀도와 재현율 자세히 알아보기
- 정밀도는 정확한 포지티브 예측의 비율입니다. 전체 고객 구매 예측 중에서 실제 구매 사례가 차지하는 비율을 말합니다.
- 재현율은 모델이 올바르게 예측한 이 라벨이 있는 행의 비율입니다. 식별 가능한 전체 고객 구매 중 실제로 식별된 비율을 말합니다.
사용 사례에 따라 정밀도 또는 재현율을 기준으로 최적화할 수 있습니다.
기타 분류 측정항목
- AUC PR: 정밀도-재현율(PR) 곡선 아래의 영역입니다. 이 값의 범위는 0부터 1까지이며 값이 클수록 모델의 품질이 높습니다.
- AUC ROC: 수신자 조작 특성(ROC) 곡선 아래의 영역입니다. 이 범위는 0부터 1까지이며 값이 클수록 모델의 품질이 높다는 것을 나타냅니다.
- 정확도: 모델로 생성한 올바른 분류 예측의 비율입니다.
- 로그 손실: 모델 예측과 타겟 값 사이의 교차 엔트로피입니다. 이 범위는 0부터 무한대이며 값이 작을수록 모델의 품질이 높다는 것을 나타냅니다.
- F1 점수: 정밀도와 재현율의 조화 평균입니다. F1은 정밀도와 재현율 사이의 균형을 찾고 있고 클래스 분포가 균등하지 않을 때 유용한 측정항목입니다.
예측 및 회귀 측정항목
모델이 빌드된 후 Vertex AI에서 검토할 수 있는 다양한 표준 회귀 측정항목을 제공합니다. 모델 평가 방법에 정답은 없습니다. 평가 측정항목은 문제 유형 및 모델 사용 목표라는 틀 안에서 고려하세요. 다음 목록은 Vertex AI에서 제공할 수 있는 일부 측정항목의 개요입니다.
평균 절대 오차(MAE)
MAE는 타겟 값과 예측 값의 절대차 평균입니다. 일련의 예측에서 나타난 오차, 즉 타겟 값과 예측 값 차이의 평균 크기를 측정합니다. MAE는 절대값을 사용하기 때문에 관계의 방향은 고려하지 않으며, 과소성능이나 과대성능을 나타내지도 않습니다. MAE를 평가할 경우 값이 작을수록 모델 품질이 높음을 의미합니다(0은 완벽한 예측자를 나타냄).
평균 제곱근 오차(RMSE)
RMSE는 타겟 값과 예측 값 간의 평균 제곱 차이의 제곱근입니다. RMSE는 MAE보다 이상점에 더 민감하므로 큰 오차가 염려되는 경우 RMSE를 평가하는 쪽이 더 유용합니다. MAE와 유사하게 값이 작을수록 모델 품질이 높음을 의미합니다(0은 완벽한 예측자를 나타냄).
평균 제곱근 대수 오차(RMSLE)
RMSLE는 대수 눈금에서의 RMSE를 말합니다. RMSLE는 절대 오차와 비교하면 상대 오차에 더 민감하며, 과대성능보다는 과소성능을 더 신경 씁니다.
관찰된 분위수(예측만 해당)
특정 타겟 분위수에서 관찰된 분위수는 지정된 분위수 예측 값보다 낮은 관찰된 값의 실제 비율을 보여줍니다. 관찰된 분위수는 모델이 타겟 분위수에 얼마나 가까운지를 보여줍니다. 두 값의 차이가 작을수록 모델 품질이 높음을 의미합니다.
확장 핀볼 손실(예측만 해당)
특정 타겟 분위수에서 모델의 품질을 측정합니다. 값이 작을수록 모델 품질이 높음을 의미합니다. 여러 분위수에서 확장 핀볼 손실 측정항목을 비교하여 각기 다른 분위수에서 모델의 상대적 정확성을 확인할 수 있습니다.
텍스트
모델 학습이 끝나면 모델 성능에 대한 요약을 받습니다. 자세한 분석을 보려면 평가 또는 전체 평가 보기를 클릭합니다.
모델을 평가하기 전에 기억해야 할 사항은 무엇인가요?
모델 디버깅은 모델 자체보다 데이터 디버깅에 관한 것입니다. 프로덕션 진행 전후 성능을 평가할 때 모델이 예상하지 못한 방식으로 작동하면 다시 데이터를 확인하여 어느 부분을 개선할 수 있는지 파악해야 합니다.
Vertex AI에서 어떤 종류의 분석을 수행할 수 있나요?
Vertex AI 평가 섹션에서는 테스트 예시를 사용한 모델 출력과 머신러닝 일반 측정항목을 사용해 커스텀 모델의 성능을 평가할 수 있습니다. 이 섹션에서는 다음 각 개념의 의미를 설명합니다.
- 모델 출력
- 점수 임곗값
- 참양성, 참음성, 거짓양성, 거짓음성
- 정밀도와 재현율
- 정밀도/재현율 곡선
- 평균 정밀도
모델 출력은 어떻게 해석하나요?
Vertex AI는 테스트 데이터의 예시를 가져와서 모델에 새로운 과제를 제시합니다. 각 예시에서 모델은 각 라벨이 해당 예시와 얼마나 강한 연관성이 있는지 나타내는 일련의 숫자를 출력합니다. 출력된 숫자가 높을수록 해당 라벨이 문서에 적용되어야 하는 것으로 모델이 확신한다는 뜻입니다.
점수 임곗값이란 무엇인가요?
Vertex AI는 점수 임곗값을 통해 확률을 'on'/'off'라는 이진값으로 변환할 수 있습니다. 점수 임곗값은 테스트 항목에 카테고리를 지정하기 위해 모델이 가져야 하는 신뢰도 수준을 의미합니다. UI에 있는 점수 임곗값 슬라이더는 데이터 세트에서 다양한 임곗값의 영향을 테스트하기 위한 시각적 도구입니다. 위 예시에서 모든 카테고리에 점수 임곗값을 0.8로 설정하면 '훌륭한 서비스'와 '제안'이 할당되지만 '정보 요청'은 할당되지 않습니다. 점수 임곗값이 낮으면 모델이 분류하는 텍스트 항목 수가 늘어나지만 그 과정에서 더 많은 텍스트 항목을 잘못 분류할 위험이 있습니다. 점수 임곗값이 높으면 모델이 분류하는 텍스트 항목 수가 줄어들지만 텍스트 항목을 잘못 분류할 위험이 낮아집니다. Google Cloud 콘솔에서 카테고리별로 임곗값을 조정하면서 시험해볼 수 있습니다.
하지만 프로덕션에서 모델을 사용할 때는 자신이 최적이라고 판단한 임곗값을 적용해야 합니다.
참양성, 참음성, 거짓양성, 거짓음성이란 무엇인가요?
점수 임곗값을 적용한 후에 모델에서 실행한 예측 결과는 다음 네 가지 카테고리 중 하나에 해당합니다.
이러한 카테고리를 사용해 모델의 효과를 평가할 수 있는 측정항목인 정밀도와 재현율을 계산할 수 있습니다.
정밀도와 재현율이란 무엇인가요?
정밀도와 재현율은 모델이 정보를 얼마나 잘 포착하고 있는지와 정보를 얼마나 놓치고 있는지를 이해하는 데 도움을 줍니다. 정밀도는 라벨이 지정된 전체 테스트 예시 중에서 실제로 해당 라벨로 카테고리가 지정되어야 했던 예시가 몇 개인지를 알려줍니다. 재현율은 라벨이 지정되어야 했던 전체 테스트 예시 중에서 실제로 해당 라벨이 지정된 예시가 몇 개인지를 알려줍니다.
정밀도나 재현율 중에서 무엇을 기준으로 최적화해야 하나요?
정밀도나 재현율 중에서 무엇을 기준으로 최적화할지는 사용 사례에 따라 달라집니다. 가장 적합한 접근 방식을 결정할 때는 다음 두 가지 사용 사례를 고려합니다.
사용 사례: 긴급 문서
긴급 문서와 긴급하지 않은 문서의 우선순위를 지정할 수 있는 시스템을 만든다고 가정해 보겠습니다.
이 경우 거짓양성은 긴급하지 않지만 긴급한 것으로 표시되는 문서입니다.
사용자는 이를 긴급하지 않은 것으로 간주하고 다음 문서로 이동할 수 있습니다.
거짓음성은 긴급한 문서를 시스템에서 긴급한 것으로 표시하지 못하는 경우입니다. 이로 인해 문제가 발생할 수 있습니다.
이러한 경우 재현율을 기준으로 최적화하는 것이 좋습니다. 이 측정항목은 예측 전체에서 얼마나 누락되었는지를 측정합니다. 고재현율 모델은 관련성이 적은 예시에 라벨을 지정할 가능성이 높습니다. 이 방법은 카테고리에 학습 데이터가 별로 없는 경우에 유용합니다.
사용 사례: 스팸 필터링
스팸 이메일 메시지를 스팸이 아닌 이메일 메시지로부터 자동으로 필터링하는 시스템을 만든다고 가정해 보겠습니다.
이 경우 거짓음성은 필터링하지 못하고 받은 편지함에서 보게 되는 스팸 메일입니다. 보통은 조금 성가실 뿐입니다.
이 경우 거짓양성은 스팸으로 잘못 표시되어 받은 편지함에서 삭제되는 이메일입니다. 중요한 이메일의 경우에는 사용자에게 부정적인 영향을 미칠 수 있습니다.
이 경우 정밀도 최적화가 좋습니다. 이 측정항목은 예측이 전체적으로 얼마나 정확한지를 측정합니다. 고정밀도 모델은 가장 관련성이 높은 예시만 라벨을 지정할 가능성이 높으므로, 해당 카테고리가 학습 데이터에서 일반적인 경우에 유용합니다.
혼동 행렬은 어떻게 사용하나요?
혼동 행렬을 사용하여 각 라벨에서의 모델 성능을 비교할 수 있습니다. 이상적인 모델에서는 대각선상의 모든 값이 높고 다른 모든 값이 낮습니다. 이는 원하는 카테고리가 정확하게 식별되었음을 나타냅니다. 다른 값이 높으면 이 값을 통해 모델이 테스트 항목을 어떻게 잘못 분류하고 있는지 알 수 있습니다.
정밀도-재현율 곡선은 어떻게 해석하나요?
점수 임곗값 도구를 사용하면 선택한 점수 임곗값이 정밀도와 재현율에 어떤 영향을 주는지 살펴볼 수 있습니다. 점수 임곗값 바의 슬라이더를 드래그하면 임곗값에 따라 변하는 정밀도-재현율 곡선 상의 위치뿐만 아니라 임곗값이 정밀도와 재현율에 개별적으로 미치는 영향을 확인할 수 있습니다(다중 클래스 모델의 경우, 이 그래프의 정밀도와 재현율은 반환되는 라벨 집합에서 점수가 가장 높은 라벨이 정밀도 및 재현율 측정항목 계산에 유일하게 사용되는 라벨임을 말해 줍니다.) 이렇게 하면 거짓양성과 거짓음성의 균형을 잘 잡을 수 있습니다.
모델 전체에 적용할 수 있는 임곗값을 선택한 후 각 라벨을 클릭하여 해당 임곗값이 라벨별 정밀도-재현율 곡선에서 어느 위치에 있는지 확인할 수 있습니다. 몇몇 라벨에만 잘못된 예측을 많이 하도록 임곗값이 정해지는 경우가 있습니다. 이럴 때 라벨별로 곡선을 보면 이런 라벨에 맞춰 클래스별 임곗값을 선택하는 데 도움이 될 수 있습니다. 예를 들어 고객 의견 데이터 세트에서 임곗값 0.5가 모든 의견 유형에 적정한 정밀도와 재현율을 나타내며 예외적으로 '제안'은 아주 일반적인 카테고리이므로 그렇지 않다고 가정해 보겠습니다. 이 카테고리에는 거짓양성이 많이 있습니다. 이 경우 예측을 위한 분류 기준을 호출할 때 '제안' 카테고리에만 임곗값 0.8을 사용할 수 있습니다.
평균 정밀도란 무엇인가요?
모델 정확도를 측정하는 유용한 항목은 정밀도-재현율 곡선 아래에 있는 면적으로, 점수 임곗값 전체에 대비 모델 성능을 나타냅니다. Vertex AI에서는 이 측정항목은 평균 정밀도라고 합니다. 이 점수가 1.0에 가까울수록 테스트 세트에 대한 모델의 성능이 높습니다. 각 라벨에 대해 무작위로 추측하는 모델의 평균 정밀도는 약 0.5 수준입니다.
동영상
모델 학습이 끝나면 성능에 대한 요약을 받게 됩니다. 모델 평가 측정항목은 데이터 세트의 일부(검증 데이터 세트)에 대한 모델의 성능을 기반으로 합니다. 새로운 데이터에서 모델을 사용할 준비가 되었는지 결정할 때는 몇 가지 주요 측정항목과 개념을 고려해야 합니다.
점수 임곗값
머신러닝 모델은 축구 골이 실제 골인지를 어떻게 알 수 있나요? 각 예측에는 신뢰도 점수가 할당됩니다. 신뢰도 점수는 해당 동영상 세그먼트에 클래스가 포함되어 있다는 모델의 확실성에 대한 수치 평가입니다. 점수 임곗값은 해당 점수가 예 또는 아니요로 판단하는 기준을 결정하는 숫자입니다. 즉, 모델이 '예, 이 신뢰도 숫자는 이 동영상 세그먼트에 골이 포함되어 있다고 결론을 내릴 정도로 충분히 높습니다.'라고 예측하는 값입니다.
점수 임곗값이 낮으면 모델이 동영상 세그먼트의 라벨을 잘못 지정할 위험이 있습니다. 따라서 점수 임곗값은 해당 사용 사례를 기반으로 해야 합니다. 암 검출과 같은 의료 사용 사례를 상상해보세요. 라벨을 잘못 지정할 경우 스포츠 동영상 라벨을 잘못 지정할 때보다 그 결과가 더 심각해집니다. 암 검출에서는 높은 점수 임곗값이 적절합니다.
예측 결과
점수 임곗값을 적용한 후에 모델의 예측은 4가지 카테고리 중 하나에 해당합니다. 이러한 카테고리를 이해하기 위해 특정 세그먼트에 축구 골의 포함 여부를 감지하는 모델을 빌드한다고 가정해 보겠습니다. 이 예에서는 골이 포지티브 클래스입니다(모델이 예측하려고 시도하는 클래스).
- 참양성: 모델이 포지티브 클래스를 정확하게 예측합니다. 모델이 동영상 세그먼트에서 골을 정확하게 예측했습니다.
- 거짓양성: 모델이 포지티브 클래스를 잘못 예측합니다. 모델이 세그먼트에서 골을 예측했지만 실제로 하나도 존재하지 않았습니다.
- 참음성: 모델이 네거티브 클래스를 정확하게 예측합니다. 모델이 세그먼트에 골이 없음을 정확하게 예측했습니다.
- 거짓음성: 모델이 네거티브 클래스를 잘못 예측합니다. 모델이 세그먼트에 골이 없음을 예측했지만 실제로 존재했습니다.
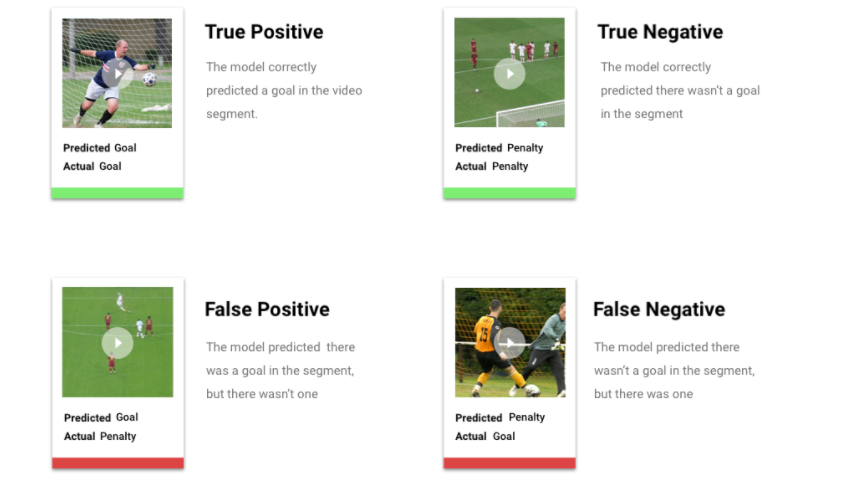
정밀도와 재현율
정밀도와 재현율 측정항목은 모델이 정보를 얼마나 잘 포착하고 있는지와 정보를 얼마나 놓치고 있는지를 이해하는 데 도움을 줍니다. 정밀도와 재현율 자세히 알아보기
- 정밀도는 정확한 포지티브 예측의 비율입니다. '골'이라고 라벨이 표시된 모든 예측 중에서 실제로 골이 포함된 비율은 얼마나 되나요?
- 재현율은 실제로 식별된 모든 포지티브 예측의 비율입니다. 식별할 수 있었던 모든 축구 골 중 그 비율은 얼마나 되나요?
사용 사례에 따라 정밀도 또는 재현율을 기준으로 최적화할 수 있습니다. 다음 사용 사례를 참조하세요.
사용 사례: 동영상의 비공개 정보
동영상에서 민감한 정보를 자동으로 감지하여 흐리게 처리하는 소프트웨어를 만든다고 가정하겠습니다. 거짓 결과의 영향에는 다음이 포함될 수 있습니다.
- 거짓양성은 검열할 필요가 없지만 검열되는 정보를 식별합니다. 이는 성가시기는 하지만 문제가 되지는 않습니다.
- 거짓음성은 신용카드 번호와 같은 검열해야 하는 정보를 식별하지 못합니다. 이는 비공개 정보가 유출되는 최악의 시나리오입니다.
이 사용 사례에서는 모델이 모든 관련 사례를 찾을 수 있도록 재현율을 기준으로 최적화하는 것이 중요합니다. 재현율을 기준으로 최적화된 모델은 조금이라도 관련성이 있는 예에 라벨을 지정할 가능성이 높지만 잘못된 예에 라벨을 지정할 가능성이 더 높습니다(필요한 것보다 더 많이 흐리게 처리).
사용 사례: 동영상 검색
사용자가 키워드를 기준으로 동영상 라이브러리를 검색할 수 있는 소프트웨어를 만든다고 가정해 보겠습니다. 다음과 같은 잘못된 결과를 고려하세요.
- 거짓양성은 관련이 없는 동영상을 반환합니다. 시스템은 관련된 동영상만 제공하려고 시도하므로 소프트웨어가 실제로 빌드한 목적을 수행하지 않습니다.
- 거짓음성은 관련된 동영상을 반환하지 못합니다. 많은 키워드에 수백 개의 동영상이 있으므로 이 문제는 관련 없는 동영상을 반환하는 것만큼 심각하지는 않습니다.
이 예시에서는 모델이 관련성이 높은 올바른 결과를 제공하도록 정밀도를 기준으로 최적화해야 합니다. 고정밀도 모델은 가장 관련성이 높은 예만 라벨을 지정할 가능성이 높지만 일부 예를 놓칠 수 있습니다. 모델 평가 측정항목 자세히 알아보기
모델 테스트
이미지
Vertex AI는 모델을 테스트하는 데 자동으로 데이터의 10%(또는 수동 데이터 분할을 선택한 경우에는 사용자가 선택한 비율)를 사용하며, 이 테스트 데이터에 대한 모델의 결과는 '평가' 페이지에 나타납니다. 하지만 모델의 신뢰도를 확인하려면 여러 가지 방법으로 확인할 수 있습니다. 가장 쉬운 방법은 '배포 및 테스트' 페이지에 몇 개의 이미지를 업로드하고 모델이 예시에 선택하는 라벨을 살펴보는 것입니다. 그 결과가 예상과 같기를 바랍니다. 받을 것으로 예상되는 이미지의 각 유형별로 몇 가지 예시를 시도해 보세요.
자동화된 자체 테스트에서 모델을 사용하려는 경우에는 '배포 및 테스트' 페이지에 프로그래매틱 방식으로 모델을 호출하는 방법도 표시됩니다.
테이블 형식
모델 측정항목 평가는 모델의 배포 준비 여부를 판단하는 기본적인 방법이지만, 새 데이터를 이용해 모델을 테스트할 수도 있습니다. 새 데이터를 업로드해 모델의 예측이 예상과 일치하는지 확인해 보세요. 평가 측정항목을 바탕으로, 또는 새 데이터를 이용한 테스트를 바탕으로 모델의 성능을 지속적으로 개선해야 할 수도 있습니다.
텍스트
Vertex AI는 자동으로 데이터의 10%(또는 데이터 분할을 선택한 경우에는 선택한 비율)를 모델 테스트에 사용하며, 이 테스트 데이터에 대한 모델의 결과는 평가 페이지에 나타납니다. 하지만 모델을 확인하려면 여러 가지 방법으로 확인할 수 있습니다. 모델을 배포한 후 배포 및 테스트 페이지의 필드에 텍스트 예시를 입력하면 모델이 예시에 선택하는 라벨을 확인할 수 있습니다. 그 결과가 예상과 같기를 바랍니다. 받을 것으로 예상되는 의견의 각 유형별로 몇 가지 예를 시도해 보세요.
자동화된 자체 테스트에서 모델을 사용하려는 경우에는 배포 및 테스트 페이지에 프로그래매틱 방식으로 모델을 호출하는 방법을 보여주는 샘플 API 요청을 제공합니다.
자동화된 자체 테스트에서 모델을 사용하려는 경우에는 배포 및 테스트 페이지에 프로그래매틱 방식으로 모델을 호출하는 방법을 보여주는 샘플 API 요청을 제공합니다.
일괄 예측 페이지에서 여러 예측 요청을 한 번에 묶는 일괄 예측을 만들 수 있습니다. 일괄 예측은 비동기식이므로 결과를 반환하기 전에 모든 예측 요청을 처리할 때까지 대기합니다.
동영상
Vertex AI 동영상은 데이터의 20%를 자동으로 사용하거나, 데이터를 직접 분할하기로 선택한 경우 선택한 비율의 데이터를 사용해 모델을 테스트합니다. 콘솔의 평가 탭에 모델의 테스트 데이터 결과가 표시됩니다. 하지만 모델을 확인하려면 여러 가지 방법으로 확인할 수 있습니다. 한 가지 방법은 '테스트 및 사용' 탭에 테스트용 동영상 데이터가 있는 CSV 파일을 제공하고 모델이 동영상에 대해 예측하는 라벨을 확인하는 것입니다. 그 결과가 예상과 같기를 바랍니다.
예측 시각화의 임곗값을 조정할 수 있을 뿐만 아니라 3개의 시계열 척도(1초 간격, 자동화된 장면 경계 감지 후 동영상 카메라 장면, 전체 동영상 세그먼트)에서 예측을 볼 수 있습니다.
모델 배포
이미지
모델의 성능이 만족스럽다면 이제 모델을 사용합니다. 이때는 프로덕션 규모의 사용일 수도 있고, 일회성 일괄 요청일 수도 있습니다. 사용 사례에 맞게 모델을 다양한 방식으로 사용하세요.
일괄 예측
일괄 예측은 한 번에 여러 예측 요청을 할 때 유용합니다. 일괄 예측은 비동기식입니다. 즉, 모델은 예측 요청을 모두 처리한 후에 예측 값이 포함된 JSON Lines 파일을 반환합니다.
온라인 예측
REST API를 사용해 예측 요청을 할 수 있도록 모델을 배포하세요. 온라인 예측은 동기식(실시간)이며, 따라서 예측을 빠르게 반환하지만 API 호출별로 예측 요청을 하나만 허용합니다. 온라인 예측은 모델이 애플리케이션의 일부이며 시스템 구성요소가 신속한 예측을 필요로 할 때 유용합니다.
테이블 형식
모델의 성능이 만족스럽다면 이제 모델을 사용합니다. 이때는 프로덕션 규모의 사용일 수도 있고, 일회성 일괄 요청일 수도 있습니다. 자신의 사용 사례에 맞게 모델을 다양한 방식으로 사용하세요.
일괄 예측
일괄 예측은 한 번에 여러 예측 요청을 할 때 유용합니다. 일괄 예측은 비동기식입니다. 즉, 모델은 예측 요청을 모두 처리한 후에 예측 답변이 포함된 CSV 또는 BigQuery 테이블을 반환합니다.
온라인 예측
REST API를 사용해 예측 요청을 할 수 있도록 모델을 배포하세요. 온라인 예측은 동기식(실시간)이며, 따라서 예측을 빠르게 반환하지만 API 호출별로 예측 요청을 하나만 허용합니다. 온라인 예측은 모델이 애플리케이션의 일부이며 시스템 구성요소가 신속한 예측을 필요로 할 때 유용합니다.
동영상
모델의 성능이 만족스럽다면 이제 모델을 사용합니다. Vertex AI는 일괄 예측을 사용하므로 Cloud Storage에서 호스팅되는 동영상의 경로가 포함된 CSV 파일을 업로드할 수 있습니다. 모델은 각 동영상을 처리하고 다른 CSV 파일에 예측을 출력합니다. 일괄 예측은 비동기식입니다. 즉, 모델은 모든 예측 요청을 먼저 처리한 후에 결과를 출력합니다.
삭제
원치않는 요금이 청구되지 않도록 사용하지 않는 모델의 배포를 취소해야 합니다.
모델 사용을 마치면 계정에 원치 않는 요금이 청구되지 않도록 만든 리소스를 삭제합니다.