생성기는 Google의 최신 생성 대규모 언어 모델(LLM) 및 개발자가 제공하는 프롬프트를 사용하여 런타임에 에이전트 응답을 생성합니다. 사용 가능한 모델은 Vertex AI에서 제공됩니다.
생성기를 사용하면 자체 외부 웹훅을 만들지 않고도 대화형 에이전트(Dialogflow CX)에서 기본적으로 LLM을 호출할 수 있습니다. 일반적으로 LLM에 요청하는 것과 같은 작업을 수행하도록 생성기를 구성할 수 있습니다.
생성기는 요약, 매개변수 추출, 데이터 변환 등의 태스크에 효과적입니다. 아래 예시를 참조하세요.
제한사항
이 기능을 모든 Dialogflow 언어의 에이전트에 사용할 수 있지만 사용 가능한 모델에 더 제한적인 언어 제한사항이 있을 수 있습니다. 자세한 내용은 Vertex AI를 참조하세요.
생성기 개념 이해
Vertex AI 문서에는 Dialogflow용 생성기를 만들 때 이해해야 하는 정보가 포함되어 있습니다.
생성기 정의
생성기를 만들려면 다음 안내를 따르세요.
- Dialogflow CX 콘솔로 이동합니다.
- Google Cloud 프로젝트를 선택합니다.
- 에이전트를 선택합니다.
- 관리 탭을 클릭합니다.
- 생성기를 클릭합니다.
- 새로 만들기를 클릭합니다.
- 생성기를 설명하는 표시 이름을 입력합니다.
- 개념에 설명된 대로 텍스트 프롬프트, 모델, 컨트롤을 입력합니다.
- 저장을 클릭합니다.
런타임 시 처리 중 텍스트 프롬프트가 생성 모델로 전송됩니다. 모델에서 만족스러운 응답을 얻으려면 명확한 질문 또는 요청이어야 합니다.
단어 앞에 $
를 추가하여 단어를 자리표시자로 표시하여 컨텍스트에 따라 프롬프트를 만들 수 있습니다. 나중에 처리 시 생성자 프롬프트 자리표시자를 세션 매개변수와 연결할 수 있으며, 실행 중에 세션 매개변수 값으로 대체됩니다.
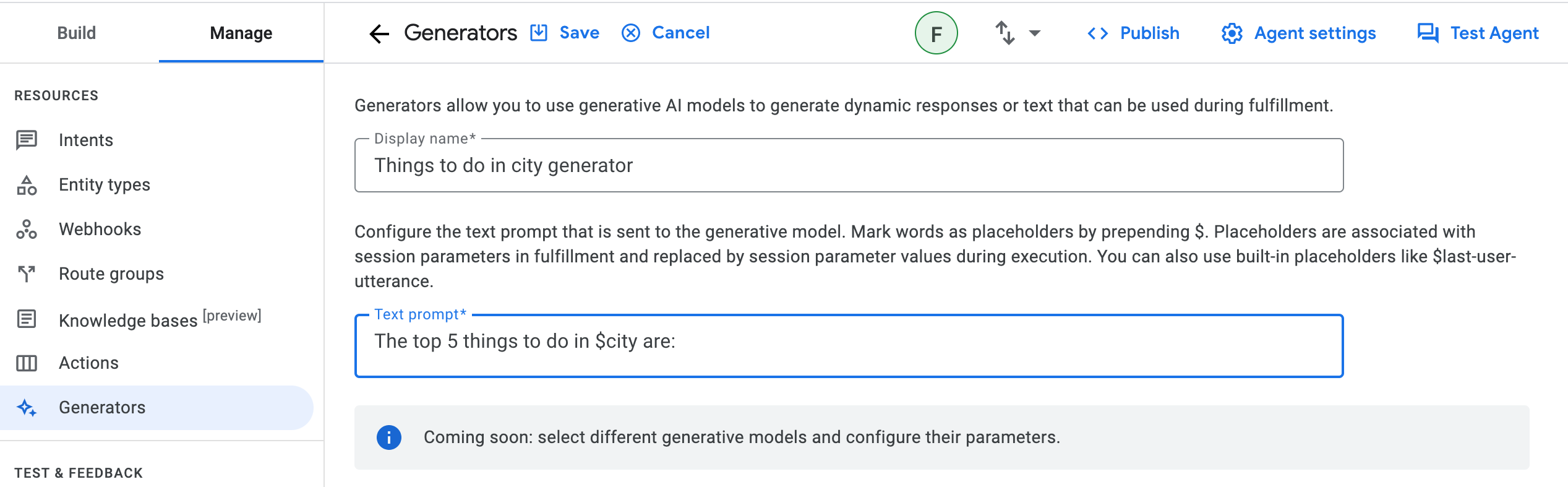
세션 파라미터와 연결할 필요가 없는 특수한 생성기 프롬프트 자리표시자가 있습니다. 이러한 기본 제공 생성기 프롬프트 자리표시자는 다음과 같습니다.
용어 | 정의 |
---|---|
$conversation |
마지막 사용자 발화와 이후 에이전트의 발화를 제외한 에이전트와 사용자 간의 대화입니다. |
$last-user-utterance |
마지막 사용자 발화입니다. |
fulfillment에서 생성기 사용
fulfillment 중에 생성기를 사용할 수 있습니다(경로, 이벤트 핸들러, 매개변수 등).
fulfillment 창의 생성기 섹션으로 이동하여 펼칩니다. 그런 다음 생성기 추가를 클릭합니다. 이제 사전 정의된 생성기를 선택하거나 새로운 생성기를 정의할 수 있습니다.
생성기를 선택한 후 프롬프트의 생성자 프롬프트 자리표시자를 세션 매개변수와 연결해야 합니다. 또한 실행 후 생성기의 결과를 포함할 출력 매개변수를 정의해야 합니다.
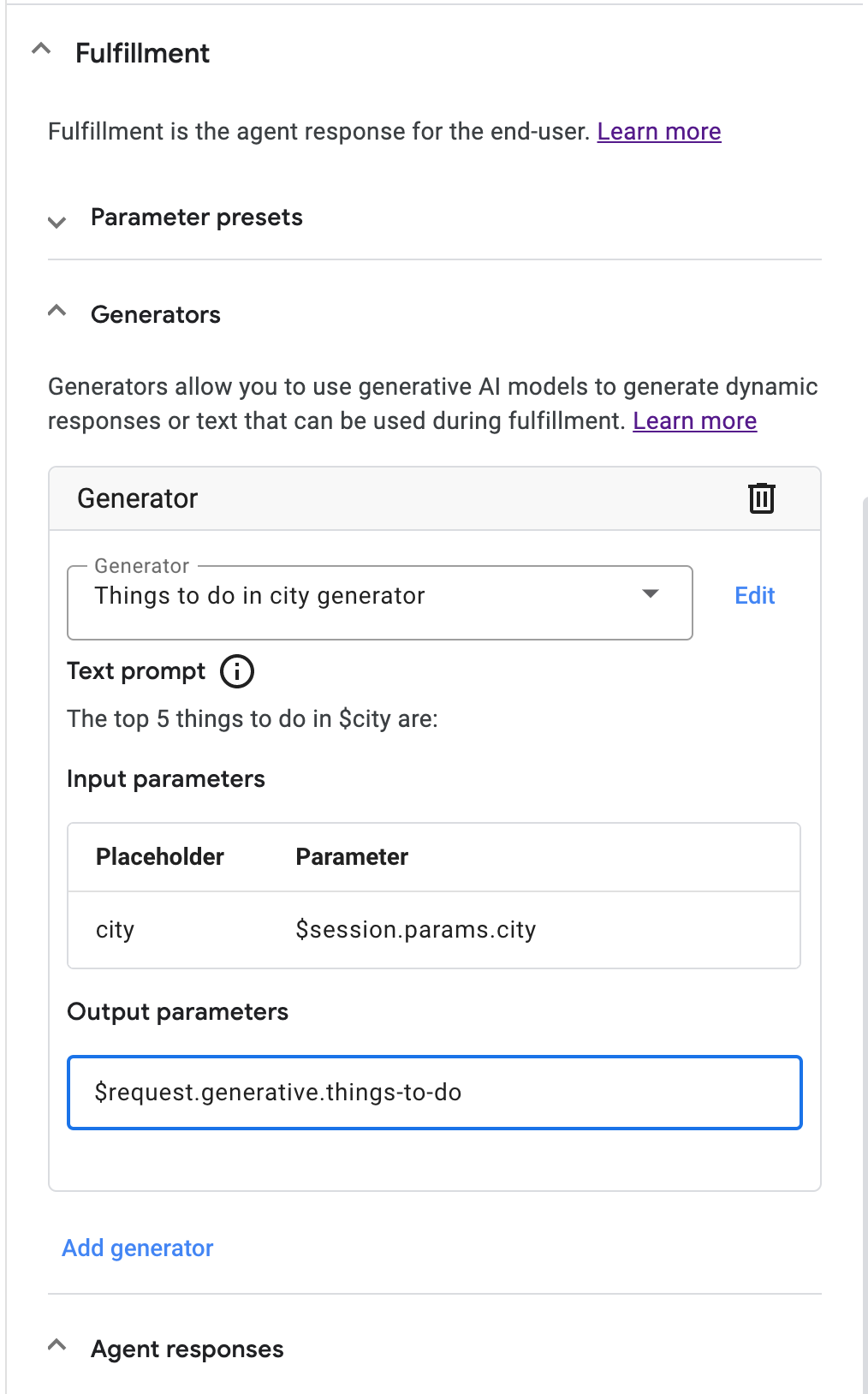
한 fulfillment에서 여러 생성기를 추가할 수 있으며 이는 동시에 실행됩니다.
그런 다음 출력 매개변수는 나중에 예를 들어 에이전트 응답에서 사용할 수 있습니다.
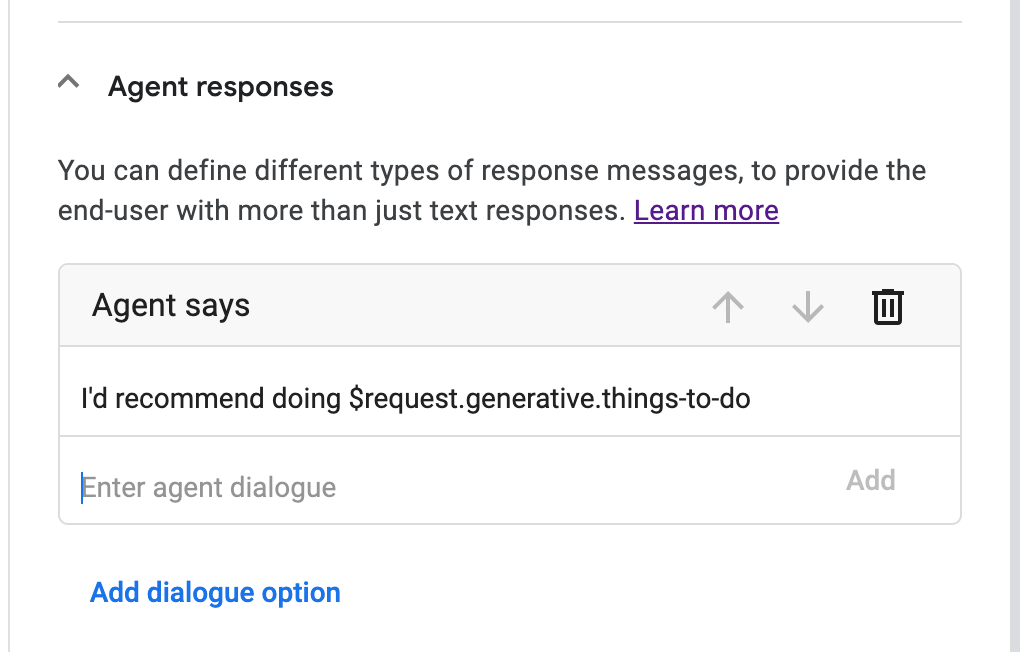
생성기 테스트
생성기 기능은 시뮬레이터에서 직접 테스트할 수 있습니다.
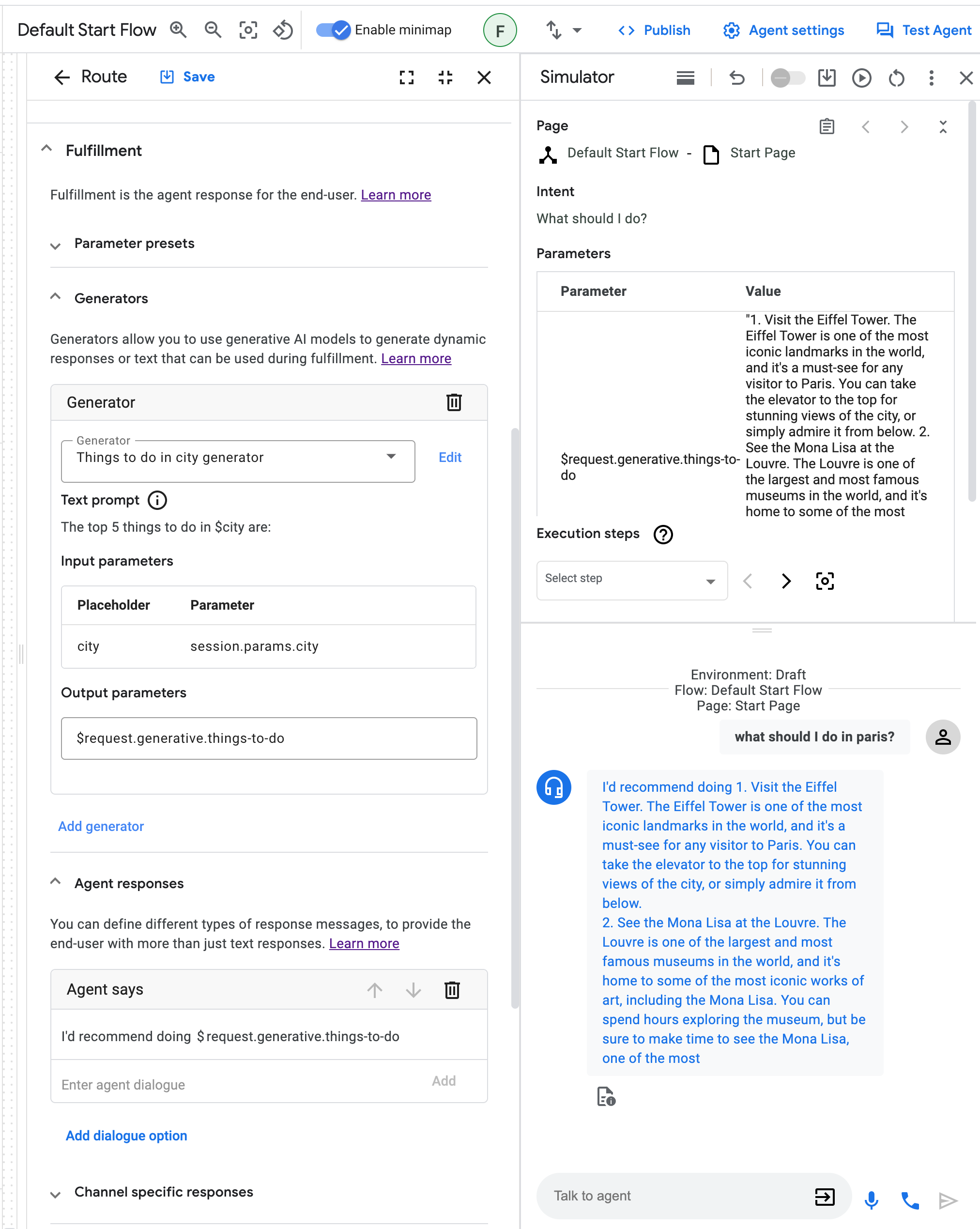
예시
이 섹션에서는 생성기의 사용 사례 예시를 제공합니다. 생성기는 대규모 언어 모델(LLM)을 기반으로 하는 생성 기술이므로 다음 예시 프롬프트를 사용한 개별 결과는 여기에 설명된 출력과 다를 수 있습니다. Google에서 반환하는 모든 프롬프트 결과는 최선의 노력입니다.
콘텐츠 요약
이 예시에서는 콘텐츠를 요약하는 방법을 보여줍니다.
프롬프트:
Your goal is to summarize a given text.
Text:
$text
A concise summary of the text in 1 or 2 sentences is:
대화 요약
이 예시에서는 대화 요약을 제공하는 방법을 보여줍니다.
프롬프트:
You are an expert at summarizing conversations between a User and an Agent.
When providing the summary, always start with "Dear $email_address, the conversation summary is as follows:"
Provide a summary in a few bullet points.
Try to be as brief as possible with each bullet point,
only noting the key points of the conversation.
Output the summary in markdown format.
Conversation:
$conversation
Summary:
변환된 프롬프트:
예시 대화에서 생성형 모델로 전송되는 변환된 프롬프트는 다음과 같을 수 있습니다.
You are an expert at summarizing conversations between a User and an Agent.
When providing the summary, always start with "Dear joe@example.com conversation summary is as follows:"
Provide a summary in a few bullet points.
Try to be as brief as possible with each bullet point,
only noting the key points of the conversation.
Output the summary in markdown format.
Conversation:
Agent: Good day! What can I do for you today?
User: Hi, which models can I use in Conversational Agents (Dialogflow CX)'s generators?
Agent: You can use all models that Vertex AI provides!
User: Thanks, thats amazing!
Summary:
마크다운 형식 지정
이 예시는 마크다운의 텍스트 형식을 지정하는 방법을 보여줍니다.
# Instructions
You are presented with a text and your goal is to apply markdown formatting to text.
**NOTE:** Do not change the meaning of the text, only the formatting.
# Example
## Text
Generators allow you to use Googles latest generative models to format text,
or to create a summaries, or even to write code. What an amazing feature.
## Text in Markdown
*Generators* allow you to use Google's latest generative models to
* format text
* create a summaries
* write code
What an amazing feature.
# Your current task
## Text
$text
## Text in Markdown
질의 응답
이 일련의 예시에서는 생성기를 사용하여 질문에 답변하는 방법을 보여줍니다.
먼저 단순히 생성형 모델의 내부 지식에 의존해서 질문에 답할 수 있습니다. 하지만 이 모델은 단순히 학습 데이터에 포함된 정보를 기반으로 답변을 제공합니다. 해당 답변이 사실이거나 최신 정보라는 보장은 없습니다.
자체 지식을 사용하는 질의 응답 프롬프트
Your goal is to politely reply to a human with an answer to their question.
The human asked:
$last-user-utterance
You answer:
제공된 정보를 사용하는 질의 응답 프롬프트
하지만 사용자가 제공한 정보를 기반으로 모델이 응답하도록 하려면 이를 단순히 프롬프트에 추가하면 됩니다. 이러한 방식은 소규모 레스토랑 메뉴나 회사의 연락처 정보와 같이 제공하려는 정보가 많지 않은 경우에 적합합니다.
# Instructions
Your goal is to politely answer questions about the restaurant menu.
If you cannot answer the question because it's not related to the restaurant
menu or because relevant information is missing from the menu, you politely
decline to answer.
# Restaurant menu:
## Starters
Salat 5$
## Main dishes
Pizza 10$
## Deserts
Ice cream 2$
# Examples
Question: How much is the pizza?
Answer: The pizza is 10$.
Question: I want to order the ice cream.
Answer: We do have ice cream! However, I can only answer questions about the menu.
Question: Do you have spaghetti?
Answer: I'm sorry, we do not have spaghetti on the menu.
# Your current task
Question: $last-user-utterance
Answer:
동적으로 제공된 정보를 사용하는 질의 응답 프롬프트
일반적으로는 모델 응답의 기준이 되는 정보가 너무 커서 단순히 이를 프롬프트에 붙여넣는 것이 어렵습니다. 이 경우에는 데이터베이스 또는 검색 엔진과 같은 정보 검색 시스템에 생성기를 연결하여 쿼리를 기반으로 정보를 동적으로 검색할 수 있습니다. 단순히 시스템 결과를 매개변수에 저장하고 이를 프롬프트의 자리표시자에 연결하면 됩니다.
# Instructions
Your goal is to politely answer questions based on the provided information.
If you can't answer the question given the provided information, you politely
decline to answer.
# Provided information:
$information
Question: $last-user-utterance
Answer:
코드 생성
이 예시에서는 생성기를 사용하여 코드를 작성하는 방법을 보여줍니다. 여기에서는 코드 생성을 위해 학습된 생성형 모델을 사용하는 것이 적합합니다.
프롬프트
# Instructions:
Your goal is to write code in a given programming language solving a given problem.
Problem to solve:
$problem
Programming language:
$programming-language
# Solution:
상담사에게 에스컬레이션
이 예시에서는 상담사에게 에스컬레이션하는 방법을 보여줍니다. 프롬프트의 마지막 두 명령어는 모델이 너무 상세하지 않도록 합니다.
프롬프트:
# Instructions:
You are a polite customer service agent that handles requests
from users to speak with an operator.
Based on the $last-user-utterance,
respond to the user appropriately about their request to speak with an operator.
Always be polite and assure the user that you
will do your best to help their situation.
Do not ask the user any questions.
Do not ask the user if there is anything you can do to help them.
# Answer:
검색어 생성
이 예시는 사용자가 제공한 Google 검색어를 최적화하는 방법을 보여줍니다.
프롬프트:
# Instructions:
You are an expert at Google Search and using "Google Fu"
to build concise search terms that provide the highest quality results.
A user will provide an example query,
and you will attempt to optimize this to be the best Google Search query possible.
# Example:
User: when was covid-19 first started and where did it originated from?
Agent: covid-19 start origin
# Your task:
User: $text
Agent:
고객 정보 검색
이 예시는 문자열 또는 JSON 형식으로 제공된 정보 검색 및 검색 데이터 수행 방법을 보여줍니다. 이러한 형식은 일반적으로 Dialogflow 세션 매개변수에서 사용됩니다.
프롬프트:
You are a database engineer and specialize in extracting information
from both structured and unstructured data formats like CSV, SQL, JSON,
and also plain text.
Given a $user_db, extract the information requested
by the user from the $last-user-utterance
EXAMPLE:
user_db: {'customer_name': 'Patrick', 'balance': '100'}
User: What is my current account balance?
Agent: Your current balance is 100.
Begin!
user_db: $user_db
User: $last-user-utterance
Agent:
JSON 객체 업데이트
이 예시에서는 사용자(또는 웹훅)의 입력 JSON 객체를 수락한 다음 사용자의 요청에 따라 객체를 조작하는 방법을 보여줍니다.
프롬프트:
You are an expert Software Engineer
that specializes in the JSON object data structure.
Given some user $update_request and existing $json_object,
you will modify the $json_object based on the user's $update_request.
EXAMPLE:
json_object = { "a": 1, "b": 123 }
User: Add a new key/value pair to my JSON
Agent: What do you want to add?
User: c: cat
Agent: { "a": 1, "b": 123, "c": "cat"}
json_object = {"accounts": [{"username": "user1", "account_number": 12345}, {"username": "user2", "account_number": 98765}], "timestamp": "2023-05-25", "version":"1.0"}
User: Add a new value for user1
Agent: What do you want to add?
User: birthday, 12/05/1982
Agent: {"accounts": [{"username": "user1", "account_number": 12345, "birthday": "12/05/1982"}, {"username": "user2", "account_number": 98765}], "timestamp": "2023-05-25", "version":"1.0"}
json_object = $json_object
User: Add a new key value to my db
Agent: What do you want to add?
User: $last-user-utterance
Agent:
Codelab
생성기 Codelab도 참조하세요.
문제 해결
기능을 디버깅하려면 Dialogflow 콘솔 시뮬레이터에서 확인된 대규모 언어 모델(LLM) 입력 프롬프트를 검사하면 됩니다.
원본 응답 버튼을 클릭합니다.
'생성기 LLM 입력' 필드를 찾습니다. 이러한 필드를 일반 텍스트로 읽고 LLM 입력이 적절한지 확인합니다. 구문에
$
가 포함된 경우 시뮬레이터 입력을 검사하고 프롬프트의$
가 의도적인지 확인합니다. 예를 들어price is $10
의$
는 의도적인 반면visit $city
에서는 그렇지 않을 가능성이 높으며 오용 또는 버그를 암시할 수 있습니다.'생성형 LLM 입력' 필드가 표시되지 않으면 지원팀에 문의하세요.