I generatori utilizzano i più recenti modelli linguistici di grandi dimensioni (LLM) di Google e i messaggi forniti da te per generare il comportamento degli agenti e le risposte in fase di runtime. I modelli disponibili sono forniti da Vertex AI.
Un generatore consente di effettuare una chiamata a un LLM in modo nativo da Dialogflow CX senza dover creare un webhook esterno. Puoi configurare il generatore in modo che faccia tutto ciò che di solito chiedi a un LLM.
I generatori sono ottimi in attività come il riepilogo, l'estrazione di parametri, le trasformazioni dei dati e così via. Consulta gli esempi di seguito.
Limitazioni
Questa funzionalità è disponibile per gli agenti in qualsiasi linguaggio Dialogflow, anche se i modelli disponibili potrebbero avere limitazioni più restrittive relative alle lingue. Consulta Vertex AI per ulteriori informazioni.
Informazioni sui concetti del generatore
La documentazione di Vertex AI contiene informazioni importanti da comprendere durante la creazione di generatori per Dialogflow:
- Modelli (modelli di base di Google e versioni e ciclo di vita dei modelli)
- Prompt
- Controlli (chiamati "valori parametro" in Vertex AI)
Definisci un generatore
Per creare un generatore:
- Vai alla console Dialogflow CX.
- Selezionare il tuo progetto Google Cloud.
- Seleziona l'agente.
- Fai clic sulla scheda Gestisci.
- Fai clic su Generatori.
- Fai clic su Crea nuova.
- Inserisci un nome visualizzato descrittivo per il generatore.
- Inserisci il prompt di testo, il modello e i controlli come descritto nei concetti.
- Fai clic su Salva.
Il prompt di testo viene inviato al modello generativo durante il fulfillment in fase di runtime. Deve essere una domanda o una richiesta chiara affinché il modello possa generare una risposta soddisfacente.
Puoi rendere il prompt contestuale contrassegnando le parole come segnaposto aggiungendo un $
prima della parola. In un secondo momento puoi associare questi segnaposto dei prompt del generatore ai parametri di sessione nel fulfillment, che verranno sostituiti dai valori dei parametri di sessione durante l'esecuzione.
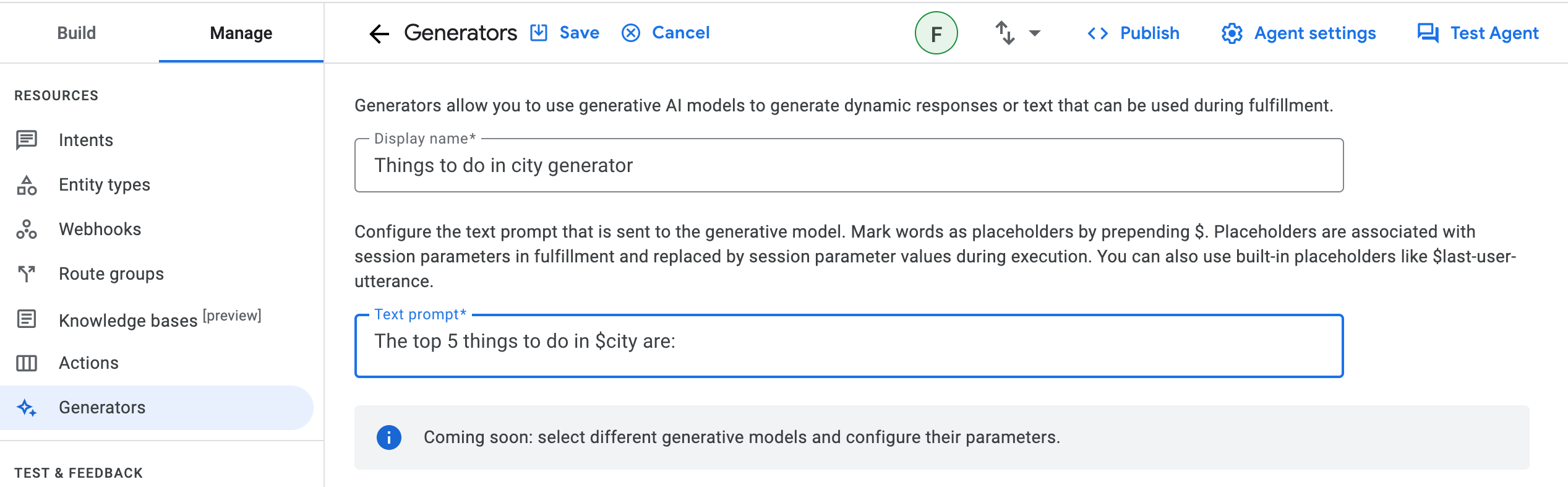
Esistono segnaposto dei prompt del generatore speciali che non devono essere associati ai parametri di sessione. Questi segnaposto dei prompt del generatore integrato sono
Termine | Definizione |
---|---|
$conversation |
La conversazione tra l'agente e l'utente, escluse l'ultima frase dell'utente e quelle successive. |
$last-user-utterance |
L'ultima frase dell'utente. |
Utilizza un generatore in fulfillment
Puoi utilizzare i generatori durante il fulfillment (in Route, Gestori di eventi, Parametri e altro ancora).
Vai alla sezione Generatori del riquadro Fulfillment ed espandila. Quindi, fai clic su Aggiungi generatore. Ora puoi selezionare un generatore predefinito o definirne uno nuovo.
Dopo aver selezionato un generatore, devi associare i segnaposto del prompt del generatore del prompt ai parametri di sessione. Inoltre, devi definire il parametro di output che conterrà il risultato del generatore dopo l'esecuzione.
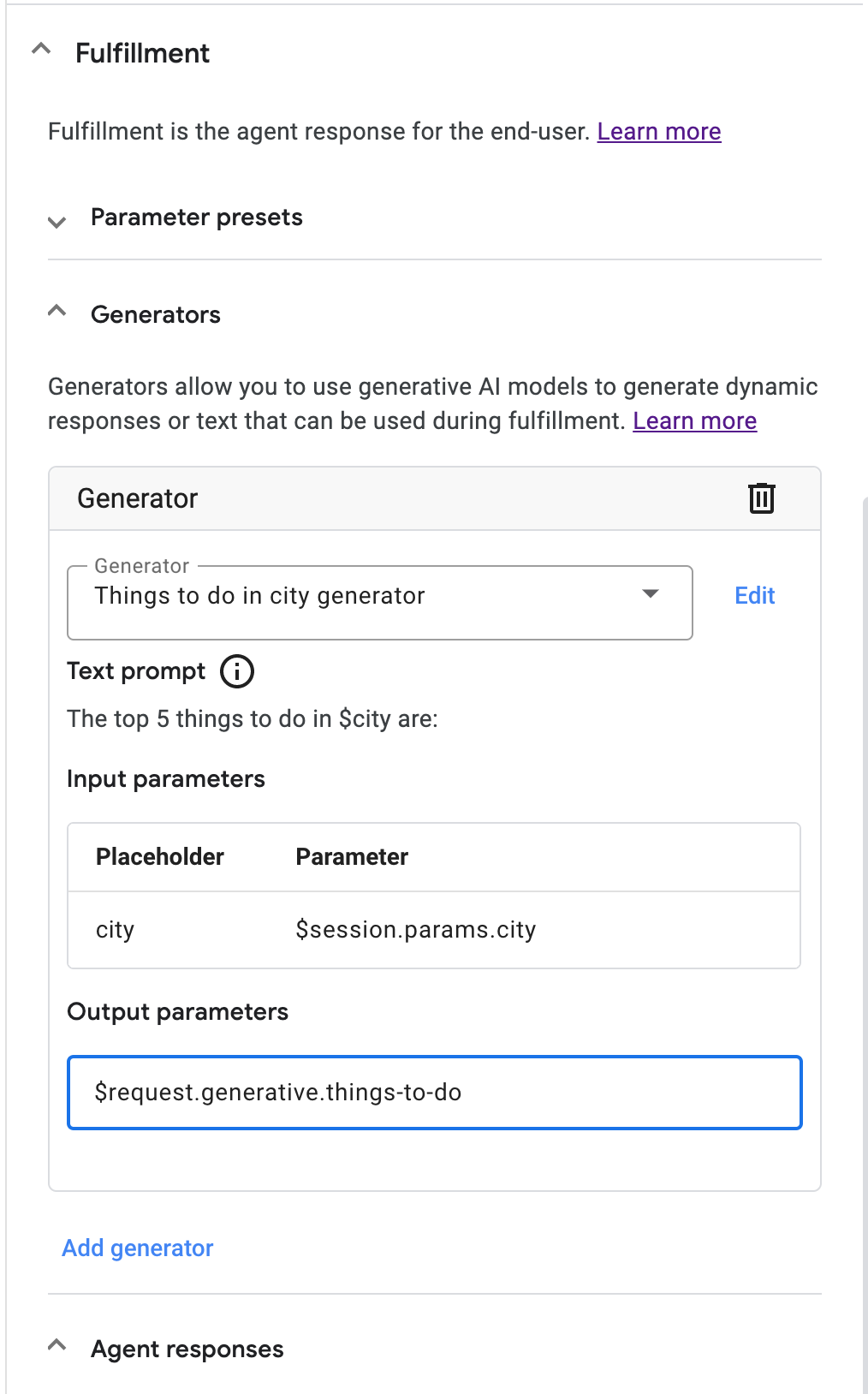
Tieni presente che in un singolo fulfillment puoi aggiungere diversi generatori, che vengono eseguiti in parallelo.
Il parametro di output può essere utilizzato in un secondo momento, ad esempio nella risposta dell'agente.
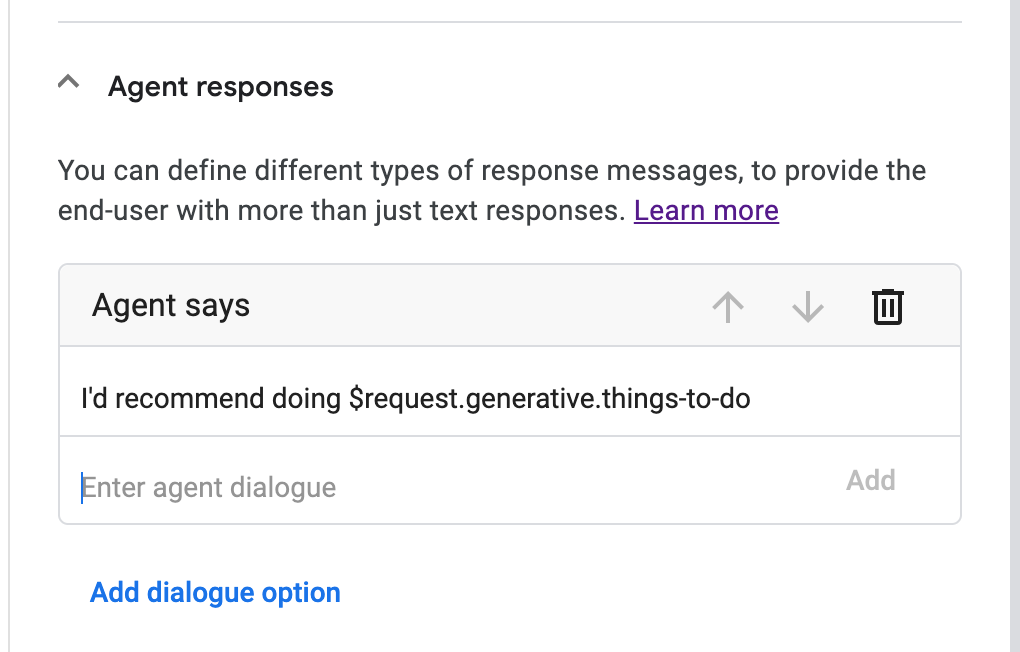
Testa un generatore
La funzione generatore può essere testata direttamente nel simulatore.
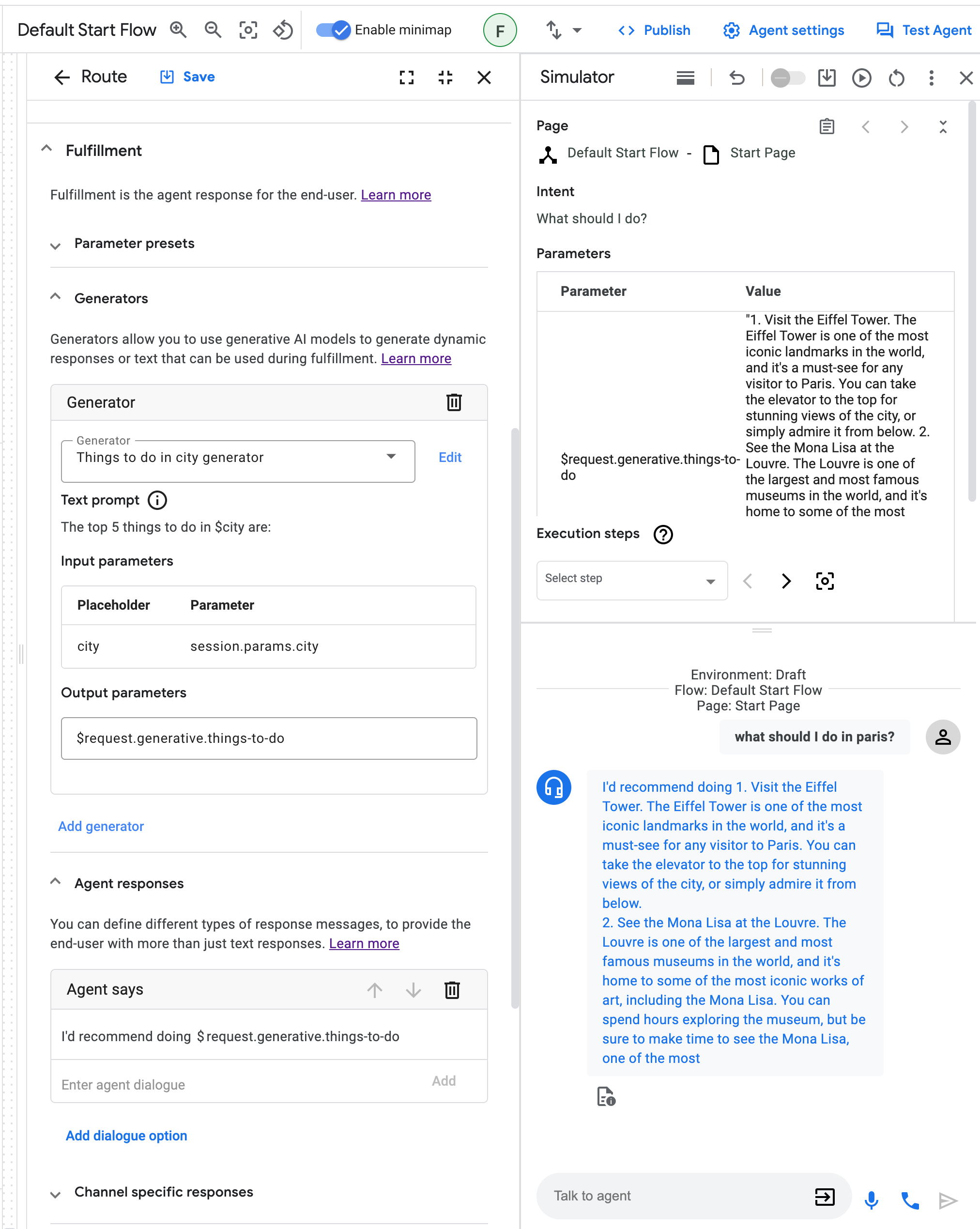
Esempi
Questa sezione fornisce casi d'uso di esempio per i generatori. Poiché i generatori sono una tecnologia generativa basata su modelli linguistici di grandi dimensioni (LLM), i singoli risultati ottenuti utilizzando i seguenti prompt di esempio potrebbero essere diversi dall'output qui documentato. Tutti i risultati dei prompt restituiti da Google si basano sul criterio del "best effort".
Riassunto dei contenuti
Questo esempio mostra come riassumere i contenuti.
Prompt:
Your goal is to summarize a given text.
Text:
$text
A concise summary of the text in 1 or 2 sentences is:
Riassunto delle conversazioni
Questo esempio mostra come fornire un riepilogo della conversazione.
Prompt:
You are an expert at summarizing conversations between a User and an Agent.
When providing the summary, always start with "Dear $email_address, the conversation summary is as follows:"
Provide a summary in a few bullet points.
Try to be as brief as possible with each bullet point,
only noting the key points of the conversation.
Output the summary in markdown format.
Conversation:
$conversation
Summary:
Prompt risolto:
Per una conversazione di esempio, il prompt risolto inviato al modello generativo potrebbe essere:
You are an expert at summarizing conversations between a User and an Agent.
When providing the summary, always start with "Dear joe@example.com conversation summary is as follows:"
Provide a summary in a few bullet points.
Try to be as brief as possible with each bullet point,
only noting the key points of the conversation.
Output the summary in markdown format.
Conversation:
Agent: Good day! What can I do for you today?
User: Hi, which models can I use in Dialogflow's generators?
Agent: You can use all models that Vertex AI provides!
User: Thanks, thats amazing!
Summary:
Formattazione di markdown
Questo esempio mostra come formattare il testo in markdown.
# Instructions
You are presented with a text and your goal is to apply markdown formatting to text.
**NOTE:** Do not change the meaning of the text, only the formatting.
# Example
## Text
Generators allow you to use Googles latest generative models to format text,
or to create a summaries, or even to write code. What an amazing feature.
## Text in Markdown
*Generators* allow you to use Google's latest generative models to
* format text
* create a summaries
* write code
What an amazing feature.
# Your current task
## Text
$text
## Text in Markdown
Question answering
Questa serie di esempi mostra come utilizzare i generatori per rispondere alle domande.
Innanzitutto, per rispondere alla domanda basta fare affidamento sulla conoscenza interna del modello generativo. Tuttavia, tieni presente che il modello fornirà semplicemente una risposta in base alle informazioni che erano parte dei suoi dati di addestramento. Non vi è alcuna garanzia che la risposta sia vera o aggiornata.
Chiedere di rispondere alle domande con autostima
Your goal is to politely reply to a human with an answer to their question.
The human asked:
$last-user-utterance
You answer:
Richiesta di risposta alle domande con le informazioni fornite
Tuttavia, se vuoi che il modello risponda in base alle informazioni fornite, puoi semplicemente aggiungerla al prompt. Questo metodo funziona se non ci sono troppe informazioni che vuoi fornire (ad es. un piccolo menù di ristorante o dati di contatto della tua azienda).
# Instructions
Your goal is to politely answer questions about the restaurant menu.
If you cannot answer the question because it's not related to the restaurant
menu or because relevant information is missing from the menu, you politely
decline to answer.
# Restaurant menu:
## Starters
Salat 5$
## Main dishes
Pizza 10$
## Deserts
Ice cream 2$
# Examples
Question: How much is the pizza?
Answer: The pizza is 10$.
Question: I want to order the ice cream.
Answer: We do have ice cream! However, I can only answer questions about the menu.
Question: Do you have spaghetti?
Answer: I'm sorry, we do not have spaghetti on the menu.
# Your current task
Question: $last-user-utterance
Answer:
Richiesta di risposta a domande con informazioni fornite dinamiche
Spesso, le informazioni su cui il modello deve basare la sua risposta sono troppe per poter essere semplicemente incollate nel prompt. In questo caso, puoi collegare il generatore a un sistema di recupero delle informazioni come un database o un motore di ricerca per recuperare dinamicamente le informazioni in base a una query. Puoi semplicemente salvare l'output del sistema in un parametro e collegarlo a un segnaposto nella richiesta.
# Instructions
Your goal is to politely answer questions based on the provided information.
If you cannot answer the question given the provided information, you plitely
decline to answer.
# Provided information:
$information
Question: $last-user-utterance
Answer:
Generazione del codice
Questo esempio mostra come utilizzare un generatore per scrivere codice. Nota che qui è utile usare un modello generativo addestrato specificatamente per generare codice.
Prompt
# Instructions:
Your goal is to write code in a given programming language solving a given problem.
Problem to solve:
$problem
Programming language:
$programming-language
# Solution:
Riassegnazione a un agente umano
Questo esempio mostra come gestire la riassegnazione a un agente umano. Le ultime due istruzioni nel prompt impediscono che il modello sia troppo dettagliato.
Prompt:
# Instructions:
You are a polite customer service agent that handles requests
from users to speak with an operator.
Based on the $last-user-utterance,
respond to the user appropriately about their request to speak with an operator.
Always be polite and assure the user that you
will do your best to help their situation.
Do not ask the user any questions.
Do not ask the user if there is anything you can do to help them.
# Answer:
Generazione di query di ricerca
Questo esempio mostra come ottimizzare una query della Ricerca Google fornita dall'utente.
Prompt:
# Instructions:
You are an expert at Google Search and using "Google Fu"
to build concise search terms that provide the highest quality results.
A user will provide an example query,
and you will attempt to optimize this to be the best Google Search query possible.
# Example:
User: when was covid-19 first started and where did it originated from?
Agent: covid-19 start origin
# Your task:
User: $text
Agent:
Recupero dei dati dei clienti
Questo esempio mostra come eseguire il recupero delle informazioni e la ricerca nei dati forniti in formato stringa o JSON. Questi formati sono comunemente utilizzati dai parametri di sessione Dialogflow.
Prompt:
You are a database engineer and specialize in extracting information
from both structured and unstructured data formats like CSV, SQL, JSON,
and also plain text.
Given a $user_db, extract the information requested
by the user from the $last-user-utterance
EXAMPLE:
user_db: {'customer_name': 'Patrick', 'balance': '100'}
User: What is my current account balance?
Agent: Your current balance is 100.
Begin!
user_db: $user_db
User: $last-user-utterance
Agent:
Aggiornamento di un oggetto JSON
Questo esempio mostra come accettare un oggetto JSON di input dall'utente (o webhook), quindi manipolare l'oggetto in base alla richiesta dell'utente.
Prompt:
You are an expert Software Engineer
that specializes in the JSON object data structure.
Given some user $update_request and existing $json_object,
you will modify the $json_object based on the user's $update_request.
EXAMPLE:
json_object = { "a": 1, "b": 123 }
User: Add a new key/value pair to my JSON
Agent: What do you want to add?
User: c: cat
Agent: { "a": 1, "b": 123, "c": "cat"}
json_object = {"accounts": [{"username": "user1", "account_number": 12345}, {"username": "user2", "account_number": 98765}], "timestamp": "2023-05-25", "version":"1.0"}
User: Add a new value for user1
Agent: What do you want to add?
User: birthday, 12/05/1982
Agent: {"accounts": [{"username": "user1", "account_number": 12345, "birthday": "12/05/1982"}, {"username": "user2", "account_number": 98765}], "timestamp": "2023-05-25", "version":"1.0"}
json_object = $json_object
User: Add a new key value to my db
Agent: What do you want to add?
User: $last-user-utterance
Agent:
Codelab
Consulta anche il Codelab dei generatori.
Risoluzione dei problemi
Se vuoi eseguire il debug della funzionalità, puoi ispezionare il prompt di input del modello linguistico di grandi dimensioni (LLM) risolto nel simulatore della console Dialogflow:
Fai clic sul pulsante risposta originale:
Individua il campo "Generators LLM Inputs". Leggi questi campi come testo normale e verifica se l'input LLM ha senso. Se una frase contiene
$
, esamina l'input del simulatore e chiarisci se$
nei prompt è intenzionale (ad esempio,$
inprice is $10
è probabilmente intenzionale, mentrevisit $city
probabilmente no e potrebbe sottintendere un uso improprio o un bug).Se non riesci a visualizzare il campo "Generative LLM Inputs " (Input LLM generativi), contatta l'assistenza.