Cloud Composer 3 | Cloud Composer 2 | Cloud Composer 1
このチュートリアルでは、Cloud Composer を使用して Apache Airflow DAG(有向非巡回グラフ)を作成し、Dataproc クラスタで Apache Hadoop ワードカウント ジョブを実行する方法を説明します。
目標
- Cloud Composer 環境にアクセスし、Airflow UI を使用します。
- Airflow 環境変数を作成して表示します。
- 次のタスクを含む DAG を作成、実行します。
- Dataproc クラスタを作成します。
- クラスタ上で Apache Hadoop ワードカウント ジョブを実行します。
- ワードカウントの結果を Cloud Storage バケットに出力します。
- クラスタを削除します。
費用
このドキュメントでは、Google Cloud の次の課金対象のコンポーネントを使用します。
- Cloud Composer
- Dataproc
- Cloud Storage
料金計算ツールを使うと、予想使用量に基づいて費用の見積もりを生成できます。
始める前に
プロジェクトで次の API が有効になっていることを確認します。
コンソール
Enable the Dataproc, Cloud Storage APIs.
gcloud
Enable the Dataproc, Cloud Storage APIs:
gcloud services enable dataproc.googleapis.com
storage-component.googleapis.com プロジェクトで、Hadoop ワードカウント ジョブの結果を格納する任意のストレージ クラスとリージョンの Cloud Storage バケットを作成します。
作成したバケットのパスをメモします(
gs://example-bucket
など)。このパスの Airflow 変数を定義して、この変数をこのチュートリアルの後半の DAG の例で使用します。デフォルト パラメータを使用して Cloud Composer 環境を作成します。環境の作成が完了するまで待ちます。処理が完了すると、緑色のチェックマークが環境名の左側に表示されます。
環境を作成したリージョンをメモします(
us-central
など)。このリージョンに対して Airflow 変数を定義し、サンプルの DAG で同じリージョンに Dataproc クラスタを実行します。
Airflow 変数を設定する
後で例の DAG で使用する Airflow 変数を設定します。たとえば、Airflow UI で Airflow 変数を設定できます。
Airflow 変数 | 値 |
---|---|
gcp_project
|
このチュートリアルで使用しているプロジェクトのプロジェクト ID(example-project など)。 |
gcs_bucket
|
このチュートリアル用に作成した Cloud Storage バケット(gs://example-bucket など)。 |
gce_region
|
環境を作成したリージョン(us-central1 など)。
これは、Dataproc クラスタが作成されるリージョンです。 |
ワークフローの例を表示する
Airflow DAG は、スケジュールを設定して実行する体系的なタスクの集まりです。DAG は、標準の Python ファイルで定義されます。hadoop_tutorial.py
に含まれるコードは、ワークフロー コードです。
Airflow 2
Airflow 1
演算子
ワークフロー例の 3 つのタスクをオーケストレーションするため、DAG は次の 3 つの Airflow オペレーターをインポートします。
DataprocClusterCreateOperator
: Dataproc クラスタを作成します。DataProcHadoopOperator
: Hadoop のワードカウント ジョブを送信し、結果を Cloud Storage バケットに書き込みます。DataprocClusterDeleteOperator
: クラスタを削除して、現在使用中の Compute Engine の利用料金が発生しないようにします。
依存
実行するタスクを関係と依存状態が反映されるように編成してください。この DAG 内のタスクが順次実行されます。
Airflow 2
Airflow 1
スケジュール
DAG の名前は composer_hadoop_tutorial
です。この DAG は 1 日 1 回実行されます。
default_dag_args
に渡される start_date
が yesterday
に設定されているため、DAG が環境のバケットにアップロードされた直後に開始されるように、Cloud Composer によってワークフローのスケジュールが設定されます。
Airflow 2
Airflow 1
DAG を環境のバケットにアップロードする
Cloud Composer は、DAG を環境のバケット内の /dags
フォルダに保存します。
DAG をアップロードするには:
ローカルマシン上で
hadoop_tutorial.py
を保存します。Google Cloud コンソールで [環境] ページに移動します。
環境のリストにある環境の [DAG のフォルダ] 列で、[DAG] リンクをクリックします。
[ファイルをアップロード] をクリックします。
ローカルマシン上の
hadoop_tutorial.py
を選択して、[開く] をクリックします。
Cloud Composer により、DAG が Airflow に追加されて自動的にスケジュール設定されます。DAG の変更は 3~5 分以内に行われます。
DAG の実行状況を確認する
タスクのステータスを表示する
DAG ファイルを Cloud Storage の dags/
フォルダにアップロードすると、ファイルが Cloud Composer によって解析されます。正常に完了すれば、このワークフローの名前が DAG のリストに表示され、即時実行されるようキューに登録されます。
タスクのステータスを確認するには、Airflow ウェブ インターフェースに移動して、ツールバーの [DAG] をクリックします。
DAG の詳細ページを開くには、
composer_hadoop_tutorial
をクリックします。このページでは、ワークフローのタスクと依存関係が図で示されます。各タスクのステータスを確認するには、[Graph View] をクリックしてから、各タスクのグラフィックにカーソルを合わせます。
ワークフローをもう一度キューに入れる
グラフビューからワークフローをもう一度実行するには、次の手順を行います。
- Airflow UI のグラフビューで、
create_dataproc_cluster
グラフィックをクリックします。 - [Clear] をクリックしてから [OK] をクリックして、3 つのタスクをリセットします。
- グラフビューで
create_dataproc_cluster
をもう一度クリックします。 - [Run] をクリックして、ワークフローをもう一度キューに入れます。
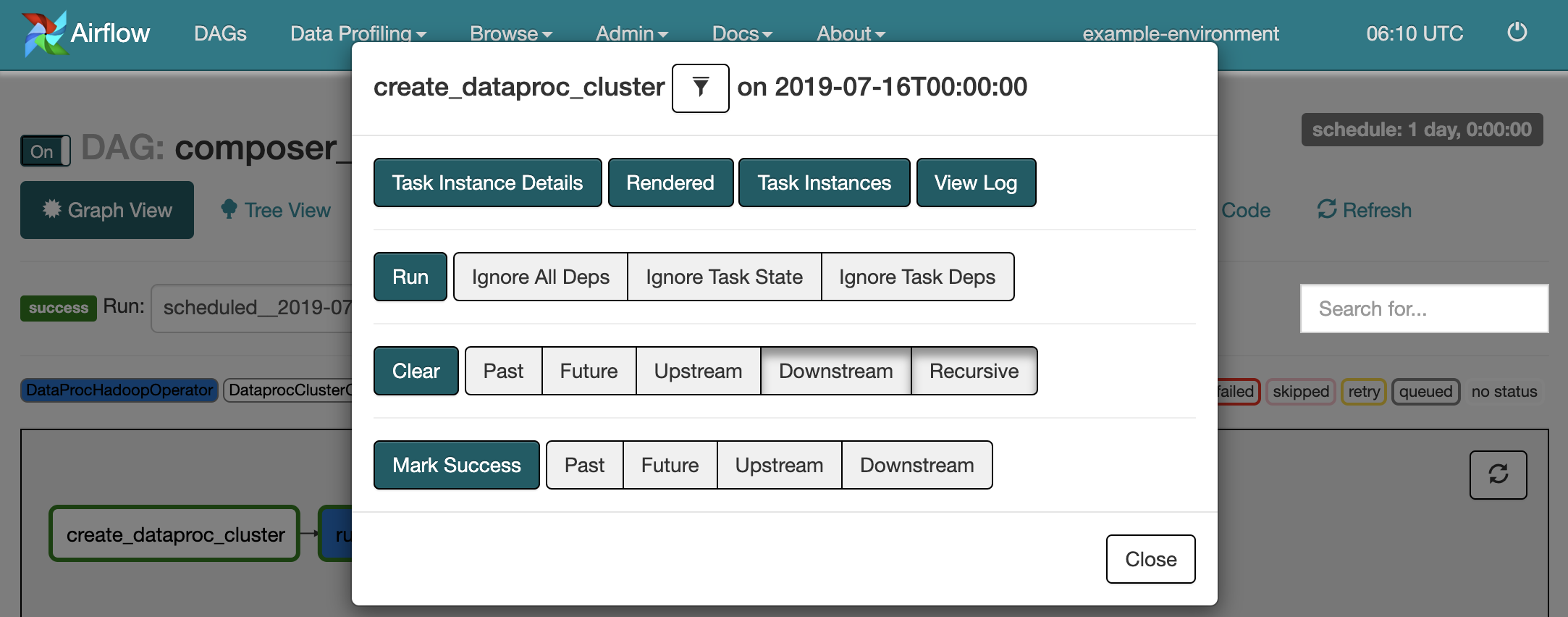
タスクの結果を表示する
次の Google Cloud コンソール ページに移動して、composer_hadoop_tutorial
ワークフローのステータスと結果を確認することもできます。
Dataproc クラスタ: クラスタの作成と削除をモニタリングします。ワークフローによって作成されるクラスタは、一時的なものです。ワークフローの実行中にのみ存在し、最後のワークフロー タスクの一部として削除されます。
Dataproc ジョブ: Apache Hadoop のワードカウント ジョブを表示またはモニタリングします。ジョブ ID をクリックすると、ジョブのログ出力を確認できます。
Cloud Storage ブラウザ: このチュートリアル用に作成した Cloud Storage バケット内の
wordcount
フォルダのワードカウントの結果を表示します。
クリーンアップ
このチュートリアルで使用したリソースを削除します。
Cloud Composer 環境を削除します(環境のバケットを手動で削除します)。
Hadoop ワードカウント ジョブの結果を格納する Cloud Storage バケットを削除します。