Cloud Composer 3 | Cloud Composer 2 | Cloud Composer 1
This page provides troubleshooting steps and information for common workflow issues.
Many DAG execution issues are caused by non-optimal environment performance. You can optimize your environment by following the Optimize environment performance and costs guide.
Some DAG executions issues might be caused by the Airflow scheduler not working correctly or optimally. Follow Scheduler troubleshooting instructions to solve these issues.
Troubleshooting workflow
To begin troubleshooting:
Check the Airflow logs.
You can increase the logging level of Airflow by overriding the following Airflow configuration option.
Section Key Value logging
logging_level
The default value is INFO
. Set toDEBUG
to get more verbosity in log messages.Check the Monitoring Dashboard.
Review the Cloud Monitoring.
In Google Cloud console, check for errors on the pages for the components of your environment.
In the Airflow web interface, check in the DAG's Graph View for failed task instances.
Section Key Value webserver
dag_orientation
LR
,TB
,RL
, orBT
Debugging operator failures
To debug an operator failure:
- Check for task-specific errors.
- Check the Airflow logs.
- Review the Cloud Monitoring.
- Check operator-specific logs.
- Fix the errors.
- Upload the DAG to the
/dags
folder. - In the Airflow web interface, clear the past states for the DAG.
- Resume or run the DAG.
Troubleshooting task execution
Airflow is a distributed system with many entities like scheduler, executor, workers that communicate to each other through a task queue and the Airflow database and send signals (like SIGTERM). The following diagram shows an overview of interconnections between Airflow components.
In a distributed system like Airflow there might be some network connectivity issues, or the underlying infrastructure might experience intermittent issues; this can lead to situations when tasks can fail and be rescheduled for execution, or tasks might not be successfully completed (for example, Zombie tasks, or tasks that got stuck in execution). Airflow has mechanisms to deal with such situations and automatically resume the normal functioning. Following sections explain common problems that occur during task execution by Airflow: Zombie tasks, Terminating instance and SIGTERM signals.
Troubleshooting Zombie tasks
Airflow detects two kinds of mismatch between a task and a process that executes the task:
Zombie tasks are tasks that are supposed to be running but are not running. This might happen if the task's process was terminated or is not responding, if the Airflow worker didn't report a task status in time because it is overloaded, or if VM where the task is executed was shut down. Airflow finds such tasks periodically, and either fails or retries the task, depending on the task's settings.
Discover zombie tasks
resource.type="cloud_composer_environment" resource.labels.environment_name="ENVIRONMENT_NAME" log_id("airflow-scheduler") textPayload:"Detected zombie job"
Undead tasks are tasks that are not supposed to be running. Airflow finds such tasks periodically and terminates them.
The following sections describe most common reasons and solutions for Zombie tasks.
Airflow worker ran out of memory
Each Airflow worker can run up to [celery]worker_concurrency
task instances
simultaneously. If a cumulative memory consumption of those task instances
exceeds the memory limit for an Airflow worker, a random process on it is
terminated to free up resources.
Sometimes, the shortage of memory on an Airflow worker can lead to malformed packets being sent during an SQL Alchemy session to the database, to a DNS server or to any other service called by a DAG. In this case the other end of the connection might reject or drop connections from the Airflow worker. For example:
"UNKNOWN:Error received from peer
{created_time:"2024-11-31T10:09:52.217738071+00:00", grpc_status:14,
grpc_message:"failed to connect to all addresses; last error: UNKNOWN:
ipv4:<ip address>:443: handshaker shutdown"}"
Solutions:
Optimize tasks to use less memory, for example, by avoiding top level code.
Decrease
[celery]worker_concurrency
.Increase memory for Airflow workers to accommodate
[celery]worker_concurrency
changes.
Airflow worker was evicted
Pod evictions are a normal part of running workloads on Kubernetes. GKE evicts pods if they ran out of storage or to free up resources for workloads with a higher priority.
Solutions:
- If an eviction is caused by the lack of storage, you can reduce the storage
usage or remove temporary files as soon as they aren't needed.
As an alternative, you can
increase available storage or run
workloads in a dedicated pod with
KubernetesPodOperator
.
Airflow worker was terminated
Airflow workers might be removed externally. If currently running tasks don't finish during a graceful termination period, they are interrupted and might end up being detected as zombies.
Possible scenarios and solutions:
Airflow workers are restarted during environment modifications, such as upgrades or package installation:
Discover Composer environment modifications
resource.type="cloud_composer_environment" resource.labels.environment_name="ENVIRONMENT_NAME" log_id("cloudaudit.googleapis.com%2Factivity")
You can perform such operations when no critical tasks are running or enable task retries.
Various components might be temporarily unavailable during maintenance operations.
You can specify maintenance windows to minimizeoverlaps with the critical tasks execution.
Airflow worker was under heavy load
The amount of CPU and memory resources available to an Airflow worker is limited by the environment's configuration. If the resource utilization gets closer to the limits, it might cause a resource contention and unnecessary delays during the task execution. In the extreme situations, when resources lack during longer periods of time, this might cause zombie tasks.
Solutions:
- Monitor workers CPU and memory usage and adjust it to avoid exceeding 80%.
Airflow database was under heavy load
A database is used by various Airflow components to communicate to each other and, in particular, to store heartbeats of task instances. Resource shortage on the database leads to longer query times and might affect task execution.
Sometimes, the following errors are present in an Airflow worker's logs:
(psycopg2.OperationalError) connection to server at <IP address>,
port 3306 failed: server closed the connection unexpectedly
This probably means the server terminated abnormally before or while
processing the request.
Solutions:
- Avoid using a lot of
Variables.get
instructions in your top-level DAG code. Instead, use Jijna templates for retrieving values of Airflow variables. - Optimize (reduce) the usage of xcom_push and xcom_pull instructions in Jinja Templates in top-level DAG code.
- Consider upgrading to a larger environment size (Medium or Large).
- Lower the number of schedulers
- Lower the frequency of DAG parsing.
- Monitor the database CPU and memory usage.
Airflow database was temporarily unavailable
An Airflow worker might take time to detect and gracefully handle intermittent errors, such as temporary connectivity issues. It might exceed the default zombie detection threshold.
Discover Airflow heartbeat timeouts
resource.type="cloud_composer_environment" resource.labels.environment_name="ENVIRONMENT_NAME" log_id("airflow-worker") textPayload:"Heartbeat time limit exceeded"
Solutions:
Increase the timeout for zombie tasks and override the value of the
[scheduler]scheduler_zombie_task_threshold
Airflow configuration option:Section Key Value Notes scheduler
scheduler_zombie_task_threshold
New timeout (in seconds) The default value is 300
Troubleshooting Terminating instance
Airflow uses the terminating instance mechanism to shut down Airflow tasks. This mechanism is used in the following situations:
- When a scheduler terminates a task that did not complete on time.
- When a task times out or is executed for too long.
When Airflow terminates task instances, you can see the following log entries in the logs of an Airflow worker that executed the task:
INFO - Subtask ... WARNING - State of this instance has been externally set
to success. Terminating instance.
INFO - Subtask ... INFO - Sending Signals.SIGTERM to GPID <X>
INFO - Subtask ... ERROR - Received SIGTERM. Terminating subprocesses.
Possible solutions:
Check the task code for errors that might cause it to run for too long.
Increase the CPU and memory for Airflow workers, so that tasks execute faster.
Increase the value of the
[celery_broker_transport_options]visibility-timeout
Airflow configuration option.As a result, the scheduler waits longer for a task to be finished, before considering the task to be a Zombie task. This option is especially useful for time-consuming tasks that last many hours. If the value is too low (for example, 3 hours), then the scheduler considers tasks that run for 5 or 6 hours as "hanged" (Zombie tasks).
Increase the value of the
[core]killed_task_cleanup_time
Airflow configuration option.A longer value provides more time to Airflow workers to finish their tasks gracefully. If the value is too low, Airflow tasks might be interrupted abruptly, without enough time to finish their work gracefully.
Troubleshooting SIGTERM signals
SIGTERM signals are used by Linux, Kubernetes, Airflow scheduler and Celery to terminate processes responsible for running Airflow workers or Airflow tasks.
There might be several reasons why SIGTERM signals are sent in an environment:
A task became a Zombie task and must be stopped.
The scheduler discovered a duplicate of a task and sends Terminating instance and SIGTERM signals to the task to stop it.
In Horizontal Pod Autoscaling, the GKE Control Plane sends SIGTERM signals to remove Pods that are no longer needed.
The scheduler can send SIGTERM signals to DagFileProcessorManager process. Such SIGTERM signals are used by the Scheduler to manage DagFileProcessorManager process lifecycle and can be safely ignored.
Example:
Launched DagFileProcessorManager with pid: 353002 Sending Signals.SIGTERM to group 353002. PIDs of all processes in the group: [] Sending the signal Signals.SIGTERM to group 353002 Sending the signal Signals.SIGTERM to process 353002 as process group is missing.
Race condition between the heartbeat callback and exit callbacks in the local_task_job, which monitors the execution of the task. If the heartbeat detects that a task was marked as success, it cannot distinguish whether the task itself succeeded or that Airflow was told to consider the task successful. Nonetheless, it will terminate a task runner, without waiting for it to exit.
Such SIGTERM signals can be safely ignored. The task is already in the successful state and the execution of the DAG run as a whole will not be affected.
The log entry
Received SIGTERM.
is the only difference between the regular exit and the termination of task in the successful state.Figure 2. Race condition between the heartbeat and exit callbacks (click to enlarge) An Airflow component uses more resources (CPU, memory) than permitted by the cluster node.
GKE service performs maintenance operations and sends SIGTERM signals to Pods that run on a node that is about to be upgraded.
When a task instance is terminated with SIGTERM, you can see the following log entries in the logs of an Airflow worker that executed the task:
{local_task_job.py:211} WARNING - State of this instance has been externally set to queued. Terminating instance. {taskinstance.py:1411} ERROR - Received SIGTERM. Terminating subprocesses. {taskinstance.py:1703} ERROR - Task failed with exception
Possible solutions:
This issue happens when a VM that runs the task is out of memory. This is not related to Airflow configurations but to the amount of memory available to the VM.
In Cloud Composer 3, you can assign more CPU and memory resources to Airflow workers.
You can lower the value of the
[celery]worker_concurrency
concurrency Airflow configuration option. This option determines how many tasks are executed concurrently by a given Airflow worker.
For more information about optimizing your environment, see Optimize environment performance and costs.
Impact of update or upgrade operations on Airflow task executions
Update or upgrade operations interrupt currently executing Airflow tasks, unless a task is executed in the deferrable mode.
We recommend to perform these operations when you expect minimal impact on Airflow task executions and set up appropriate retry mechanisms in your DAGs and tasks.
Troubleshooting KubernetesExecutor tasks
CeleryKubernetesExecutor is a type of executor in Cloud Composer 3 that can use CeleryExecutor and KubernetesExecutor at the same time.
See the Use CeleryKubernetesExecutor page for more information about troubleshooting tasks executed with KubernetesExecutor.
Common issues
The following sections describe symptoms and potential fixes for some common DAG issues.
Airflow task was interrupted by Negsignal.SIGKILL
Sometimes your task might be using more memory than Airflow worker is allocated.
In such a situation it might be interrupted by Negsignal.SIGKILL
. The system
sends this signal to avoid further memory consumption which might impact
the execution of other Airflow tasks. In the Airflow worker's log you might see
the following log entry:
{local_task_job.py:102} INFO - Task exited with return code Negsignal.SIGKILL
Negsignal.SIGKILL
might also appear as code -9
.
Possible solutions:
Lower
worker_concurrency
of Airflow workers.Increase the amount of memory available to Airflow workers.
Manage resource-intensive tasks in Cloud Composer by using KubernetesPodOperator or GKEStartPodOperator for task isolation and customized resource allocation.
Optimize your tasks to use less memory.
Task fails without emitting logs because of DAG parsing errors
Sometimes there might be subtle DAG errors that lead to a situation where
the Airflow scheduler can schedule tasks for execution, the DAG processor can
parse the DAG file, but then the Airflow worker fails to execute tasks
from the DAG because there are programming errors in the DAG file. This might
lead to a situation where an Airflow task is marked as Failed
and there is no
log from its execution.
Solutions:
Verify in Airflow worker logs that there are no errors raised by Airflow worker that are related to a missing DAG or DAG parsing errors.
Increase parameters related to DAG parsing:
Increase dagbag-import-timeout to at least 120 seconds (or more, if required).
Increase dag-file-processor-timeout to at least 180 seconds (or more, if required). This value must be higher than
dagbag-import-timeout
.
See also Inspecting DAG Processor logs.
Task fails without emitting logs because of the resource pressure
Symptom: during execution of a task, Airflow worker's subprocess responsible for Airflow task execution is interrupted abruptly. The error visible in Airflow worker's log might look similar to the one below:
...
File "/opt/python3.8/lib/python3.8/site-packages/celery/app/trace.py", line 412, in trace_task R = retval = fun(*args, **kwargs) File "/opt/python3.8/lib/python3.8/site-packages/celery/app/trace.py", line 704, in __protected_call__ return self.run(*args, **kwargs) File "/opt/python3.8/lib/python3.8/site-packages/airflow/executors/celery_executor.py", line 88, in execute_command _execute_in_fork(command_to_exec) File "/opt/python3.8/lib/python3.8/site-packages/airflow/executors/celery_executor.py", line 99, in _execute_in_fork
raise AirflowException('Celery command failed on host: ' + get_hostname())airflow.exceptions.AirflowException: Celery command failed on host: airflow-worker-9qg9x
...
Solution:
In Cloud Composer 3, increase memory limits for Airflow workers.
If your environment also generates zombie tasks, see Troubleshooting Zombie tasks.
For a tutorial on debugging out of memory issues, see Debug out of memory and out of storage DAG issues.
Task fails without emitting logs because of Pod eviction
Google Kubernetes Engine Pods are subject to the Kubernetes Pod Lifecycle and Pod eviction. Task spikes is the most common cause for Pod eviction in Cloud Composer.
Pod eviction can occur when a particular Pod overuses resources of a node, relative to the configured resource consumption expectations for the node. For example, eviction might happen when several memory-heavy tasks run in a Pod, and their combined load causes the node where this Pod runs to exceed the memory consumption limit.
If an Airflow worker Pod is evicted, all task instances running on that Pod are interrupted, and later are marked as failed by Airflow.
Logs are buffered. If a worker Pod is evicted before the buffer flushes, logs are not emitted. Task failure without logs is an indication that the Airflow workers are restarted due to out-of-memory (OOM). Some logs might be present in Cloud Logging even though the Airflow logs were not emitted.
To view logs:
In Google Cloud console, go to the Environments page.
In the list of environments, click the name of your environment. The Environment details page opens.
Go to the Logs tab.
View logs of individual Airflow workers under All logs > Airflow logs > Workers.
Solution:
Increase memory limits for Airflow workers.
Make sure that the tasks in the DAG are idempotent and retriable.
Avoid downloading unnecessary files to the local file system of Airflow workers.
Airflow workers have limited local file system capacity. An Airflow worker can have from 1 GB to 10 GB of storage. When the storage space runs out, the Airflow worker Pod is evicted by the GKE Control Plane. This fails all tasks that the evicted worker was executing.
Examples, of problematic operations:
- Downloading files or objects and storing them locally in an Airflow worker. Instead, store these objects directly in a suitable service such as a Cloud Storage bucket.
- Accessing big objects in the
/data
folder from an Airflow worker. The Airflow worker downloads the object into its local filesystem. Instead, implement your DAGs so that large files are processed outside of the Airflow worker Pod.
DAG load import timeout
Symptom:
- In the Airflow web interface, at the top of the DAGs list page, a red alert
box shows
Broken DAG: [/path/to/dagfile] Timeout
. In Cloud Monitoring: The
airflow-scheduler
logs contain entries similar to:ERROR - Process timed out
ERROR - Failed to import: /path/to/dagfile
AirflowTaskTimeout: Timeout
Fix:
Override the dag_file_processor_timeout
Airflow
configuration option and allow more time for DAG parsing:
Section | Key | Value |
---|---|---|
core |
dag_file_processor_timeout |
New timeout value |
DAG execution does not end within expected time
Symptom:
Sometimes a DAG run does not end because Airflow tasks get stuck and DAG run lasts longer than expected. Under normal conditions, Airflow tasks do not stay indefinitely in the queued or running state, because Airflow has timeout and cleanup procedures that help avoid this situation.
Fix:
Use the
dagrun_timeout
parameter for the DAGs. For example:dagrun_timeout=timedelta(minutes=120)
. As a result, each DAG run must be finished within the DAG run timeout. Not finished tasks are marked asFailed
orUpstream Failed
. For more information about Airflow task states, see Apache Airflow documentation.Use the task execution timeout parameter to define a default timeout for tasks that run based on Apache Airflow operators.
DAG runs are not executed
Symptom:
When a schedule date for a DAG is set dynamically, this can lead to various unexpected side effects. For example:
A DAG execution is always in the future, and the DAG is never executed.
Past DAG runs are marked as executed and successful despite not being executed.
More information is available in the Apache Airflow documentation.
Possible solutions:
Follow the recommendations in the Apache Airflow documentation.
Set static
start_date
for DAGs. As an option, you can usecatchup=False
to disable running the DAG for past dates.Avoid using
datetime.now()
ordays_ago(<number of days>)
unless you are aware of the side effects of this approach.
Increased network traffic to and from the Airflow database
The amount of traffic network between your environment's GKE cluster and the Airflow database depends on the number of DAGs, number of tasks in DAGs, and the way DAGs access data in the Airflow database. The following factors might influence the network usage:
Queries to the Airflow database. If your DAGs do a lot of queries, they generate large amounts of traffic. Examples: checking the status of tasks before proceeding with other tasks, querying the XCom table, dumping Airflow database content.
Large number of tasks. The more tasks are there to schedule, the more network traffic is generated. This consideration applies both to the total number of tasks in your DAGs and to the scheduling frequency. When the Airflow scheduler schedules DAG runs, it makes queries to the Airflow database and generates traffic.
Airflow web interface generates network traffic because it makes queries to the Airflow database. Intensively using pages with graphs, tasks, and diagrams can generate large volumes of network traffic.
DAG crashes the Airflow web server or causes it to return a '502 gateway timeout' error
Web server failures can occur for several different reasons. Check
the airflow-webserver logs in
Cloud Logging to determine the cause of the
502 gateway timeout
error.
Handling a large number of DAGs and plugins in dags and plugins folders
Contents of /dags
and /plugins
folders are synchronized from
your environment's bucket to local file systems of Airflow workers and
schedulers.
The more data stored in these folders, the longer it takes to perform the synchronization. To address such situations:
Limit the number of files in
/dags
and/plugins
folders. Store only the minimum of required files.Increase the disk space available to Airflow schedulers and workers.
Increase CPU and memory of Airflow schedulers and workers, so that the sync operation is performed faster.
In case of a very large number of DAGs, divide DAGs into batches, compress them into zip archives and deploy these archives into the
/dags
folder. This approach speeds up the DAGs syncing process. Airflow components uncompress zip archives before processing DAGs.Generating DAGs in a programmatic way might also be a method for limiting the number of DAG files stored in the
/dags
folder. See the section about Programmatic DAGs to avoid problems with scheduling and executing DAGs generated programmatically.
Do not schedule programmatically generated DAGs at the same time
Generating DAG objects programmatically from a DAG file is an efficient method to author many similar DAGs that only have small differences.
It's important to not schedule all such DAGs for execution immediately. There is a high chance that Airflow workers do not have enough CPU and memory resources to execute all tasks that scheduled at the same time.
To avoid issues with scheduling programmatic DAGs:
- Increase worker concurrency and scale up your environment, so that it can execute more tasks simultaneously.
- Generate DAGs in a way to distribute their schedules evenly over time, to avoid scheduling hundreds of tasks at the same time, so that Airflow workers have time to execute all scheduled tasks.
Error 504 when accessing the Airflow web server
See Error 504 when accessing the Airflow UI.
Lost connection to Postgres server during query exception is thrown during the task execution or right after it
Lost connection to Postgres server during query
exceptions
often happen when the following conditions are met:
- Your DAG uses
PythonOperator
or a custom operator. - Your DAG makes queries to the Airflow database.
If several queries are made from a callable function, tracebacks might
incorrectly point to self.refresh_from_db(lock_for_update=True)
line in the
Airflow code; it is the first database query after the task execution. The
actual cause of the exception happens before this, when an SQLAlchemy session
is not properly closed.
SQLAlchemy sessions are scoped to a thread and created in a callable function session can be later continued inside the Airflow code. If there are significant delays between queries within one session, the connection might be already closed by the Postgres server. The connection timeout in Cloud Composer environments is set to approximately 10 minutes.
Solution:
- Use the
airflow.utils.db.provide_session
decorator. This decorator provides a valid session to the Airflow database in thesession
parameter and correctly closes the session at the end of the function. - Do not use a single long-running function. Instead, move all database
queries to separate functions, so that there are multiple functions with
the
airflow.utils.db.provide_session
decorator. In this case, sessions are automatically closed after retrieving query results.
Controlling execution time of DAGs, tasks and parallel executions of the same DAG
If you want to control how long a single DAG execution for a particular DAG
lasts, then you can use the dagrun_timeout
DAG
parameter to do so. For example, if you expect that a single DAG run (
irrespective, whether execution finishes with success or failure) must not last
longer than 1 hour, then set this parameter to 3600 seconds.
You can also control how long you allow for a single Airflow task to last. To do
so, you can use execution_timeout
.
If you want to control how many active DAG runs you want to have for a
particular DAG then you can use the [core]max-active-runs-per-dag
Airflow configuration option to do so.
If you want to have only a single instance of a DAG run in a given moment, set
max-active-runs-per-dag
parameter to 1
.
Issues impacting DAGs and plugins syncing to schedulers, workers and web servers
Cloud Composer synchronizes the content of /dags
and /plugins
folders to schedulers and workers. Certain objects in /dags
and /plugins
folders might prevent this synchronization to work correctly or slow it down.
The
/dags
folder is synchronized to schedulers and workers.This folder is not synchronized to the web server.
The
/plugins
folder is synchronized to schedulers, workers and web servers.
You might encounter the following issues:
You uploaded gzip-compressed files that use compression transcoding to
/dags
and/plugins
folders. It usually happens if you use the--gzip-local-all
flag in agcloud storage cp
command to upload data to the bucket.Solution: Delete the object that used compression transcoding and re-upload it to the bucket.
One of the objects is named '.'—such an object is not synchronized to schedulers and workers, and it might stop synchronizing at all.
Solution: Rename the object.
A folder and a DAG Python file have the same names, for example
a.py
. In this case, the DAG file is not properly synchronized to Airflow components.Solution: Remove the folder that has the same name as the DAG Python file.
One of the objects in
/dags
or/plugins
folders contains a/
symbol at the end of the object's name. Such objects can interfere with the synchronization process because the/
symbol means that an object is a folder, not a file.Solution: Remove the
/
symbol from the name of the problematic object.Don't store unnecessary files in
/dags
and/plugins
folders.Sometimes DAGs and plugins that you implement come with additional files, such as files that store tests for these components. These files are synchronized to workers and schedulers and impact the time needed to copy these files to schedulers, workers and web servers.
Solution: Don't store any additional and unnecessary files in
/dags
and/plugins
folders.
Done [Errno 21] Is a directory: '/home/airflow/gcs/dags/...' error is generated by schedulers and workers
This problem happens because objects can have overlapping namespace in Cloud Storage, while at the same time schedulers and workers use traditional file systems. For example, it is possible to add both a folder and an object with the same name to an environment's bucket. When the bucket is synced to the environment's schedulers and workers, this error is generated, which can lead to task failures.
To fix this problem, make sure that there are no overlapping namespaces in the
environment's bucket. For example, if both /dags/misc
(a file) and
/dags/misc/example_file.txt
(another file) are in a bucket, an error is
generated by the scheduler.
Transient interruptions when connecting to Airflow Metadata DB
Cloud Composer runs on top of a distributed infrastructure. It means that from time to time some transient issues may appear and they might interrupt execution of your Airflow tasks.
In such situations you might see the following error messages in Airflow workers' logs:
"Can't connect to Postgres server on 'airflow-sqlproxy-service.default.svc.cluster.local' (111)"
or
"Can't connect to Postgres server on 'airflow-sqlproxy-service.default.svc.cluster.local' (104)"
Such intermittent issues might be also caused by maintenance operations performed for your Cloud Composer environments.
Usually such errors are intermittent and if your Airflow tasks are idempotent and you have retries configured, they do not affect you. You can also consider defining maintenance windows.
One additional reason for such errors might be the lack of resources in your environment's cluster. In such cases, you might scale up or optimize your environment as described in Scaling environments or Optimizing your environment instructions.
A DAG run is marked as successful but has no executed tasks
If a DAG run execution_date
is earlier than the DAG's start_date
then
you might see DAG runs that don't have any task runs, but are still marked as successful.
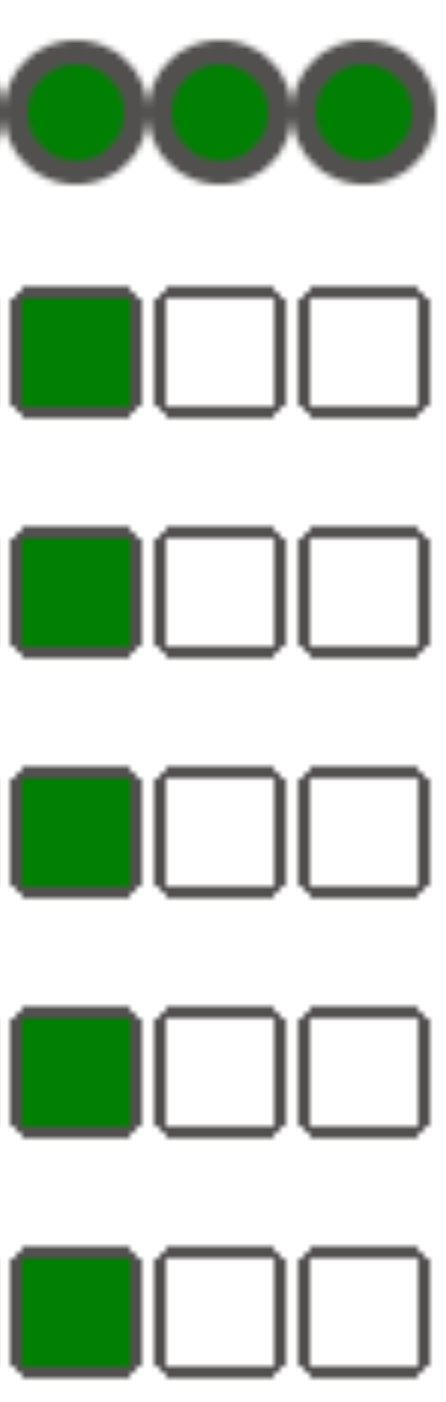
Cause
This situation might happen in one of the following cases:
A mismatch is caused by the timezone difference between the DAG's
execution_date
andstart_date
. It might happen, for example, when usingpendulum.parse(...)
to setstart_date
.The DAG's
start_date
is set to a dynamic value, for exampleairflow.utils.dates.days_ago(1)
Solution
Make sure that
execution_date
andstart_date
are using the same timezone.Specify a static
start_date
and combine it withcatchup=False
to avoid running DAGs with past start dates.
A DAG is not visible in Airflow UI or DAG UI and the scheduler does not schedule it
The DAG processor parses each DAG before it can be scheduled by the scheduler and before a DAG becomes visible in the Airflow UI or DAG UI.
The following Airflow configuration options define timeouts for parsing DAGs:
[core]dagrun_import_timeout
defines how much time the DAG processor has to parse a single DAG.[core]dag_file_processor_timeout
defines the total amount of time the DAG processor can spend on parsing all DAGs.
If a DAG is not visible in the Airflow UI or DAG UI:
Check DAG processor logs if the DAG processor is able to correctly process your DAG. In case of problems, you might see the following log entries in the DAG processor or scheduler logs:
[2020-12-03 03:06:45,672] {dag_processing.py:1334} ERROR - Processor for /usr/local/airflow/dags/example_dag.py with PID 21903 started at 2020-12-03T03:05:55.442709+00:00 has timed out, killing it.
Check scheduler logs to see if the scheduler works correctly. In case of problems, you might see the following log entries in scheduler logs:
DagFileProcessorManager (PID=732) last sent a heartbeat 240.09 seconds ago! Restarting it Process timed out, PID: 68496
Solutions:
Fix all DAG parsing errors. The DAG processor parses multiple DAGs, and in rare cases parsing errors of one DAG can negatively impact the parsing of other DAGs.
If the parsing of your DAG takes more than the amount of seconds defined in
[core]dagrun_import_timeout
, then increase this timeout.If the parsing of all your DAGs takes more than the amount of seconds defined in
[core]dag_file_processor_timeout
, then increase this timeout.If your DAG takes a long time to parse, it can also mean that it is not implemented in an optimal way. For example, if it reads read many environment variables, or performs calls to external services or Airflow database. To the extent possible, avoid performing such operations in global sections of DAGs.
Increase CPU and memory resources for the DAG processor so it can work faster.
Symptoms of Airflow database being under heavy load
For more information, see Symptoms of Airflow Database being under load pressure.