Il connettore BigQuery per Apache Spark consente ai data scientist di unire la potenza del motore SQL scalabile e senza interruzioni di BigQuery con le funzionalità di machine learning di Apache Spark. In questo tutorial, mostriamo come utilizzare Dataproc, BigQuery e Apache Spark ML per eseguire il machine learning su un set di dati.
Obiettivi
Utilizzare la regressione lineare per creare un modello di peso alla nascita in funzione di cinque fattori:- settimane di gestazione
- età della mamma
- età del padre
- aumento di peso di madre durante gravidanza
- Punteggio Apgar
Utilizza i seguenti strumenti:
- BigQuery, per preparare la tabella di input di regressione lineare, che è scritta nel tuo progetto Google Cloud
- Python, per eseguire query e gestire i dati in BigQuery
- Apache Spark, per accedere alla tabella di regressione lineare risultante
- Spark ML, per creare e valutare il modello
- Job Dataproc PySpark, per richiamare funzioni Spark ML
Costi
In questo documento, utilizzi i seguenti componenti fatturabili di Google Cloud:
- Compute Engine
- Dataproc
- BigQuery
Per generare una stima dei costi in base all'utilizzo previsto, utilizza il Calcolatore prezzi.
Prima di iniziare
In un cluster Dataproc sono installati i componenti Spark, tra cui Spark ML. Per configurare un cluster Dataproc ed eseguire il codice in questo esempio, devi eseguire (o aver eseguito) le seguenti operazioni:
- Accedi al tuo account Google Cloud. Se non conosci Google Cloud, crea un account per valutare le prestazioni dei nostri prodotti in scenari reali. I nuovi clienti ricevono anche 300 $di crediti gratuiti per l'esecuzione, il test e il deployment dei carichi di lavoro.
-
In the Google Cloud console, on the project selector page, select or create a Google Cloud project.
-
Abilita le API Dataproc, BigQuery, Compute Engine.
- Installa Google Cloud CLI.
-
Per initialize gcloud CLI, esegui questo comando:
gcloud init
-
In the Google Cloud console, on the project selector page, select or create a Google Cloud project.
-
Abilita le API Dataproc, BigQuery, Compute Engine.
- Installa Google Cloud CLI.
-
Per initialize gcloud CLI, esegui questo comando:
gcloud init
- Crea un cluster Dataproc nel progetto. Il cluster deve eseguire una versione Dataproc con Spark 2.0 o versioni successive (incluse le librerie di machine learning).
Crea un sottoinsieme di dati natality
BigQuery
In questa sezione, creerai un set di dati nel tuo progetto, quindi creerai una tabella nel set di dati in cui copierai un sottoinsieme di dati sul tasso di natalità dal set di dati BigQuery nazionalità disponibile pubblicamente. Più avanti in questo tutorial, utilizzerai i dati dei sottoinsiemi in questa tabella per prevedere il peso alla nascita in funzione dell'età materna, dell'età paterna e delle settimane di gestazione.
Puoi creare il sottoinsieme di dati utilizzando Google Cloud Console o eseguendo uno script Python sulla tua macchina locale.
Console
Creare un set di dati nel progetto.
- Vai all'interfaccia utente web di BigQuery.
- Nel pannello di navigazione a sinistra, fai clic sul nome del progetto e poi su CREA SET DI DATI.
- Nella finestra di dialogo Crea set di dati:
- In ID set di dati, inserisci "natality_regression".
- In Località dei dati, puoi scegliere una
località
per il set di dati. La posizione del valore predefinito è
US multi-region
. Dopo la creazione di un set di dati, la località non può essere modificata. - In Scadenza tabella predefinita, scegli una delle opzioni seguenti:
- Mai (impostazione predefinita): devi eliminare la tabella manualmente.
- Numero di giorni: la tabella verrà eliminata dopo il numero di giorni specificato dall'ora di creazione.
- Per Crittografia, scegli una delle seguenti opzioni:
- Chiave gestita da Google (impostazione predefinita).
- Chiave gestita dal cliente: consulta la pagina relativa alla protezione dei dati con le chiavi Cloud KMS.
- Fai clic su Crea set di dati.
Esegui una query sul set di dati di nudità pubblica, quindi salva i risultati in una nuova tabella del set di dati.
- Copia e incolla la query seguente nell'Editor query, quindi fai clic su Esegui.
SELECT weight_pounds, mother_age, father_age, gestation_weeks, weight_gain_pounds, apgar_5min FROM `bigquery-public-data.samples.natality` WHERE weight_pounds IS NOT NULL AND mother_age IS NOT NULL AND father_age IS NOT NULL AND gestation_weeks IS NOT NULL AND weight_gain_pounds IS NOT NULL AND apgar_5min IS NOT NULL
- Una volta completata la query (dopo circa un minuto), fai clic su SALVA RISULTATI, quindi seleziona le opzioni di salvataggio per salvare i risultati come "regression_input" tabella BigQuery nel
set di dati
natality_regression
nel tuo progetto.
- Copia e incolla la query seguente nell'Editor query, quindi fai clic su Esegui.
Python
Consulta la pagina relativa alla configurazione di un ambiente di sviluppo Python per le istruzioni sull'installazione di Python e della libreria client di Google Cloud per Python (necessaria per eseguire il codice). È consigliata l'installazione e l'utilizzo di un Python
virtualenv
.Copia e incolla il codice
natality_tutorial.py
riportato di seguito in una shellpython
sulla tua macchina locale. Premi la chiave<return>
nella shell per eseguire il codice per creare un set di dati BigQuery"natality_regression"nel tuo progetto predefinito di Google Cloud con una tabella "&grest_regression_input"completata con un sottoinsieme di datinatality
pubblici.Conferma la creazione del set di dati
natality_regression
e della tabellaregression_input
.
Eseguire una regressione lineare
In questa sezione eseguirai una regressione lineare PySpark inviando il job al servizio Dataproc utilizzando la console Google Cloud o eseguendo il comando gcloud
da un terminale locale.
Console
Copia e incolla il codice seguente in un nuovo file
natality_sparkml.py
sulla tua macchina locale."""Run a linear regression using Apache Spark ML. In the following PySpark (Spark Python API) code, we take the following actions: * Load a previously created linear regression (BigQuery) input table into our Cloud Dataproc Spark cluster as an RDD (Resilient Distributed Dataset) * Transform the RDD into a Spark Dataframe * Vectorize the features on which the model will be trained * Compute a linear regression using Spark ML """ from pyspark.context import SparkContext from pyspark.ml.linalg import Vectors from pyspark.ml.regression import LinearRegression from pyspark.sql.session import SparkSession # The imports, above, allow us to access SparkML features specific to linear # regression as well as the Vectors types. # Define a function that collects the features of interest # (mother_age, father_age, and gestation_weeks) into a vector. # Package the vector in a tuple containing the label (`weight_pounds`) for that # row. def vector_from_inputs(r): return (r["weight_pounds"], Vectors.dense(float(r["mother_age"]), float(r["father_age"]), float(r["gestation_weeks"]), float(r["weight_gain_pounds"]), float(r["apgar_5min"]))) sc = SparkContext() spark = SparkSession(sc) # Read the data from BigQuery as a Spark Dataframe. natality_data = spark.read.format("bigquery").option( "table", "natality_regression.regression_input").load() # Create a view so that Spark SQL queries can be run against the data. natality_data.createOrReplaceTempView("natality") # As a precaution, run a query in Spark SQL to ensure no NULL values exist. sql_query = """ SELECT * from natality where weight_pounds is not null and mother_age is not null and father_age is not null and gestation_weeks is not null """ clean_data = spark.sql(sql_query) # Create an input DataFrame for Spark ML using the above function. training_data = clean_data.rdd.map(vector_from_inputs).toDF(["label", "features"]) training_data.cache() # Construct a new LinearRegression object and fit the training data. lr = LinearRegression(maxIter=5, regParam=0.2, solver="normal") model = lr.fit(training_data) # Print the model summary. print("Coefficients:" + str(model.coefficients)) print("Intercept:" + str(model.intercept)) print("R^2:" + str(model.summary.r2)) model.summary.residuals.show()
Copia il file
natality_sparkml.py
locale in un bucket Cloud Storage nel tuo progetto.gsutil cp natality_sparkml.py gs://bucket-name
Esegui la regressione dalla pagina Invia un job di Dataproc.
Nel campo File Python principale, inserisci l'URI
gs://
del bucket Cloud Storage in cui si trova la copia del filenatality_sparkml.py
.Seleziona
PySpark
come Tipo di prestazione.Inserisci
gs://spark-lib/bigquery/spark-bigquery-latest_2.12.jar
nel campo File jar. Questo rende il connettore spark-bigquery disponibile per l'applicazione PySpark in esecuzione per consentire la lettura dei dati BigQuery in un DataFrame Spark.Compila i campi ID job, Regione e Cluster.
Fai clic su Invia per eseguire il job sul cluster.
Al termine del job, il riepilogo del modello di output di regressione lineare viene visualizzato nella finestra dei dettagli del job Dataproc.
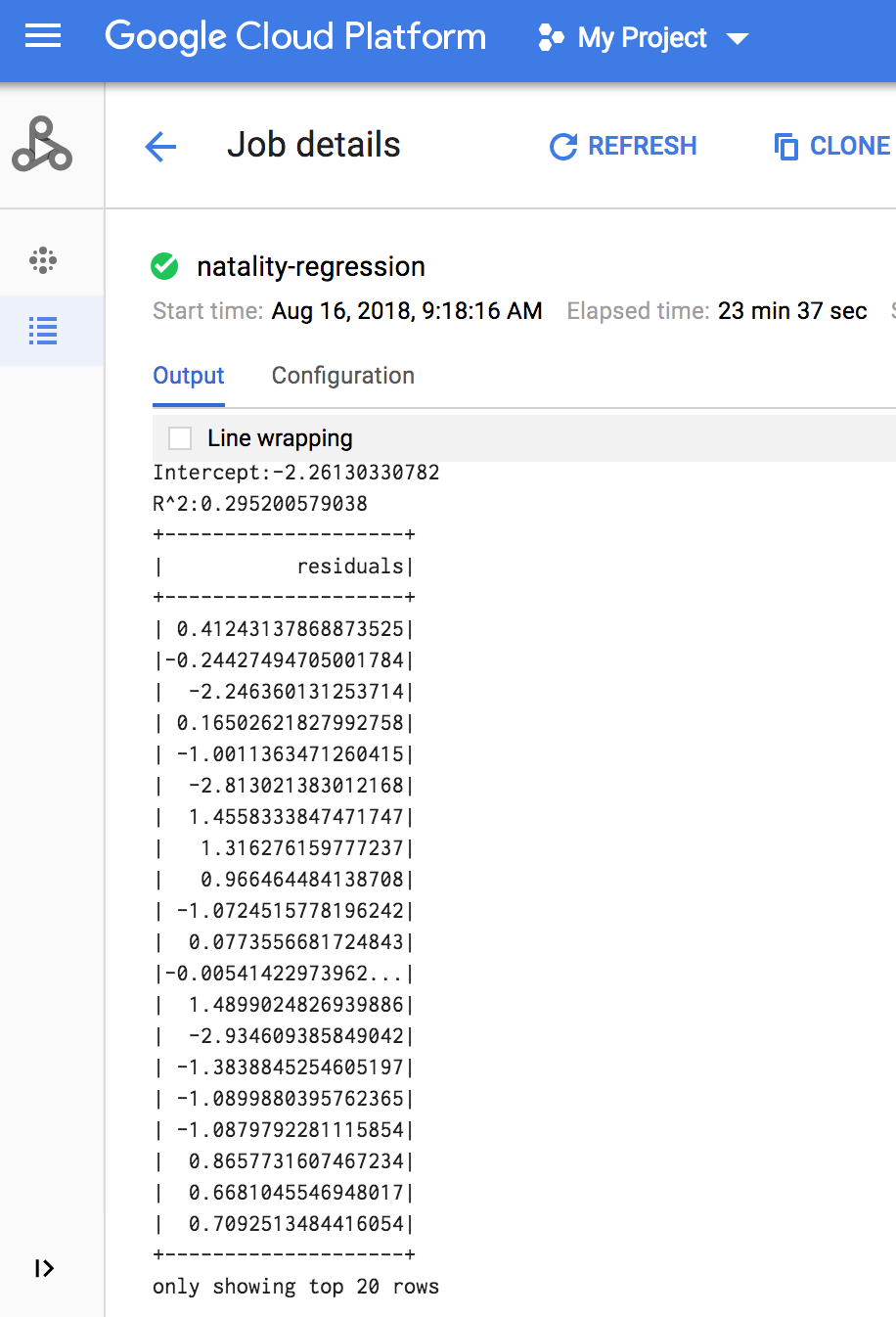
gcloud
Copia e incolla il codice seguente in un nuovo file
natality_sparkml.py
sulla tua macchina locale."""Run a linear regression using Apache Spark ML. In the following PySpark (Spark Python API) code, we take the following actions: * Load a previously created linear regression (BigQuery) input table into our Cloud Dataproc Spark cluster as an RDD (Resilient Distributed Dataset) * Transform the RDD into a Spark Dataframe * Vectorize the features on which the model will be trained * Compute a linear regression using Spark ML """ from pyspark.context import SparkContext from pyspark.ml.linalg import Vectors from pyspark.ml.regression import LinearRegression from pyspark.sql.session import SparkSession # The imports, above, allow us to access SparkML features specific to linear # regression as well as the Vectors types. # Define a function that collects the features of interest # (mother_age, father_age, and gestation_weeks) into a vector. # Package the vector in a tuple containing the label (`weight_pounds`) for that # row. def vector_from_inputs(r): return (r["weight_pounds"], Vectors.dense(float(r["mother_age"]), float(r["father_age"]), float(r["gestation_weeks"]), float(r["weight_gain_pounds"]), float(r["apgar_5min"]))) sc = SparkContext() spark = SparkSession(sc) # Read the data from BigQuery as a Spark Dataframe. natality_data = spark.read.format("bigquery").option( "table", "natality_regression.regression_input").load() # Create a view so that Spark SQL queries can be run against the data. natality_data.createOrReplaceTempView("natality") # As a precaution, run a query in Spark SQL to ensure no NULL values exist. sql_query = """ SELECT * from natality where weight_pounds is not null and mother_age is not null and father_age is not null and gestation_weeks is not null """ clean_data = spark.sql(sql_query) # Create an input DataFrame for Spark ML using the above function. training_data = clean_data.rdd.map(vector_from_inputs).toDF(["label", "features"]) training_data.cache() # Construct a new LinearRegression object and fit the training data. lr = LinearRegression(maxIter=5, regParam=0.2, solver="normal") model = lr.fit(training_data) # Print the model summary. print("Coefficients:" + str(model.coefficients)) print("Intercept:" + str(model.intercept)) print("R^2:" + str(model.summary.r2)) model.summary.residuals.show()
Copia il file
natality_sparkml.py
locale in un bucket Cloud Storage nel tuo progetto.gsutil cp natality_sparkml.py gs://bucket-name
Invia il job Pyspark al servizio Dataproc eseguendo il comando
gcloud
, mostrato di seguito, da una finestra del terminale sulla tua macchina locale.- Il valore del flag --jars rende disponibile il connettore spark-bigquery nella jobv PySpark in fase di runtime per consentire la lettura dei dati BigQuery in un DataFrame Spark.
gcloud dataproc jobs submit pyspark \ gs://your-bucket/natality_sparkml.py \ --cluster=cluster-name \ --region=region \ --jars=gs://spark-lib/bigquery/spark-bigquery-with-dependencies_SCALA_VERSION-CONNECTOR_VERSION.jar
- Il valore del flag --jars rende disponibile il connettore spark-bigquery nella jobv PySpark in fase di runtime per consentire la lettura dei dati BigQuery in un DataFrame Spark.
L'output di regressione lineare (riepilogo del modello) viene visualizzato nella finestra del terminale al completamento del job.
<<< # Print the model summary. ... print "Coefficients:" + str(model.coefficients) Coefficients:[0.0166657454602,-0.00296751984046,0.235714392936,0.00213002070133,-0.00048577251587] <<< print "Intercept:" + str(model.intercept) Intercept:-2.26130330748 <<< print "R^2:" + str(model.summary.r2) R^2:0.295200579035 <<< model.summary.residuals.show() +--------------------+ | residuals| +--------------------+ | -0.7234737533344147| | -0.985466980630501| | -0.6669710598385468| | 1.4162434829714794| |-0.09373154375186754| |-0.15461747949235072| | 0.32659061654192545| | 1.5053877697929803| | -0.640142797263989| | 1.229530260294963| |-0.03776160295256...| | -0.5160734239126814| | -1.5165972740062887| | 1.3269085258245008| | 1.7604670124710626| | 1.2348130901905972| | 2.318660276655887| | 1.0936947030883175| | 1.0169768511417363| | -1.7744915698181583| +--------------------+ only showing top 20 rows.
Esegui la pulizia
Una volta completato il tutorial, puoi eseguire la pulizia delle risorse che hai creato in modo che interrompano l'utilizzo della quota e che vengano addebitati dei costi. Le sezioni seguenti descrivono come eliminare o disattivare queste risorse.
Elimina il progetto
Il modo più semplice per eliminare la fatturazione è eliminare il progetto che hai creato per il tutorial.
Per eliminare il progetto:
- Nella console Google Cloud, vai alla pagina Gestisci risorse.
- Nell'elenco dei progetti, seleziona il progetto che vuoi eliminare, quindi fai clic su Elimina.
- Nella finestra di dialogo, digita l'ID del progetto e fai clic su Chiudi per eliminare il progetto.
Eliminazione del cluster Dataproc
Vedi Eliminare un cluster.