Vous pouvez envoyer une tâche à un cluster Dataproc existant via une requête HTTP ou automatisée jobs.submit de l'API Dataproc, à l'aide de l'outil de ligne de commande gcloud
du Google Cloud CLI dans une fenêtre de terminal locale ou dans Cloud Shell, ou encore à partir de la console Google Cloud ouverte dans un navigateur local. Vous pouvez également vous connecter en SSH à l'instance maître de votre cluster, puis exécuter une tâche directement depuis l'instance sans utiliser le service Dataproc.
Envoyer un job
Console
Ouvrez la page Dataproc Submit a job (Envoyer une tâche) dans la console Google Cloud de votre navigateur.
Exemple de tâche Spark
Pour envoyer un exemple de tâche Spark, remplissez les champs de la page Submit a job (Envoyer une tâche) comme suit:
- Sélectionnez le nom du cluster dans la liste des clusters.
- Définissez le champ Job Type (Type de tâche) sur
Spark
. - Définissez le champ Main class or jar (Classe principale ou fichier JAR) sur
org.apache.spark.examples.SparkPi
. - Définissez le champ Arguments sur l'argument unique
1000
. - Ajoutez des fichiers JAR
file:///usr/lib/spark/examples/jars/spark-examples.jar
dans le champ Jar files (Fichiers JAR) :file:///
désigne un schéma LocalFileSystem Hadoop. Dataproc a installé/usr/lib/spark/examples/jars/spark-examples.jar
sur le nœud maître du cluster lors de la création de celui-ci.- Vous pouvez également spécifier un chemin d'accès à Cloud Storage (
gs://your-bucket/your-jarfile.jar
) ou un chemin d'accès au système de fichiers distribué Hadoop (hdfs://path-to-jar.jar
) vers l'un de vos fichiers JAR.
Cliquez sur Envoyer pour démarrer la tâche. Une fois la tâche démarrée, elle est ajoutée à la liste des Tâches.
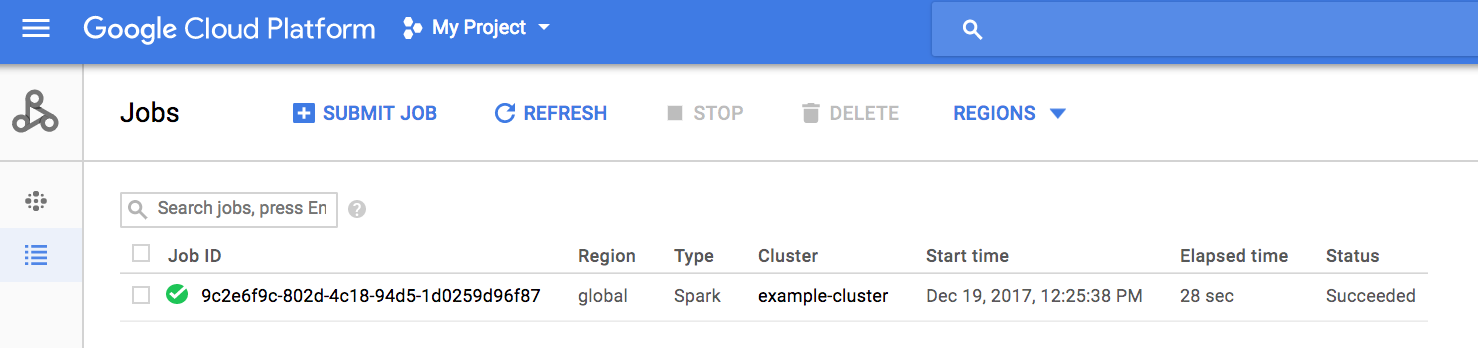
Cliquez sur l'ID de la tâche pour ouvrir la page Jobs (Tâches) qui affiche les résultats du pilote de la tâche. Étant donné que cette tâche génère de longues lignes de résultats dépassant la largeur de la fenêtre du navigateur, vous pouvez cocher la case Line wrapping (Renvoi à la ligne automatique) pour afficher tout le texte de résultats dans la vue et consulter le résultat de calcul pour pi
.
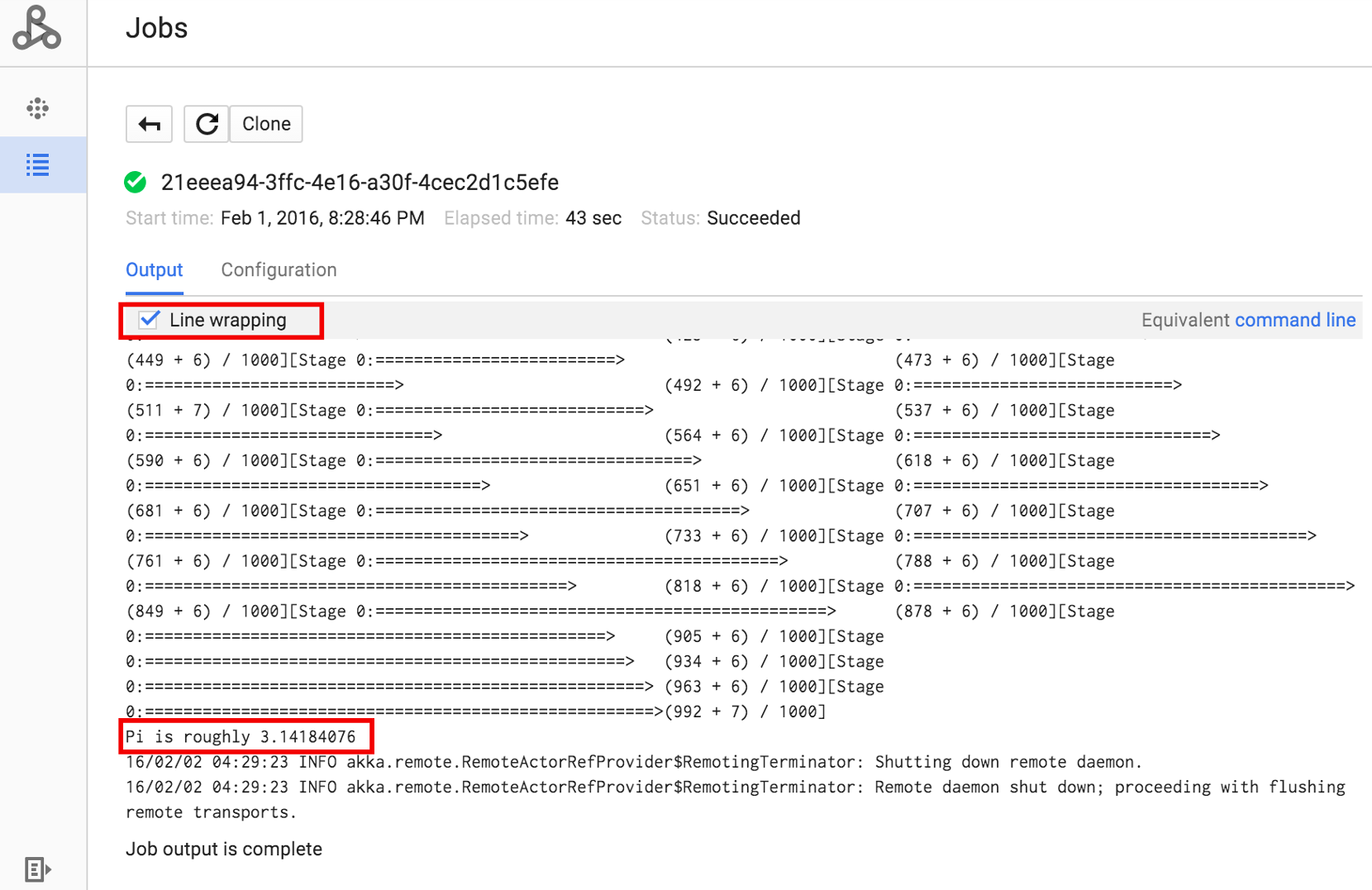
Vous pouvez afficher les résultats du pilote de la tâche depuis la ligne de commande à l'aide de la commande gcloud dataproc jobs wait présentée ci-dessous (pour en savoir plus, consultez la section Afficher la sortie de la tâche – Commande gcloud).
Copiez et collez votre ID de projet en tant que valeur de l'option --project
et votre ID de tâche (affiché dans la liste des tâches) en tant qu'argument final.
gcloud dataproc jobs wait job-id \ --project=project-id \ --region=region
Voici des extraits de résultats de pilotes pour l'exemple de tâche SparkPi
, envoyée ci-dessus :
... 2015-06-25 23:27:23,810 INFO [dag-scheduler-event-loop] scheduler.DAGScheduler (Logging.scala:logInfo(59)) - Stage 0 (reduce at SparkPi.scala:35) finished in 21.169 s 2015-06-25 23:27:23,810 INFO [task-result-getter-3] cluster.YarnScheduler (Logging.scala:logInfo(59)) - Removed TaskSet 0.0, whose tasks have all completed, from pool 2015-06-25 23:27:23,819 INFO [main] scheduler.DAGScheduler (Logging.scala:logInfo(59)) - Job 0 finished: reduce at SparkPi.scala:35, took 21.674931 s Pi is roughly 3.14189648 ... Job [c556b47a-4b46-4a94-9ba2-2dcee31167b2] finished successfully. driverOutputUri: gs://sample-staging-bucket/google-cloud-dataproc-metainfo/cfeaa033-749e-48b9-... ...
gcloud
Pour envoyer une tâche à un cluster Dataproc, exécutez la commande de gcloud CLI gcloud dataproc jobs submit en local dans une fenêtre de terminal ou dans Cloud Shell.
gcloud dataproc jobs submit job-command \ --cluster=cluster-name \ --region=region \ other dataproc-flags \ -- job-args
- Répertoriez l'élément accessible au public
hello-world.py
, situé dans Cloud Storage. Fichiers répertoriés:gcloud storage cat gs://dataproc-examples/pyspark/hello-world/hello-world.py
#!/usr/bin/python import pyspark sc = pyspark.SparkContext() rdd = sc.parallelize(['Hello,', 'world!']) words = sorted(rdd.collect()) print(words)
- Envoyez la tâche Pyspark à Dataproc.
Résultat du terminal:gcloud dataproc jobs submit pyspark \ gs://dataproc-examples/pyspark/hello-world/hello-world.py \ --cluster=cluster-name \ --region=region
Waiting for job output... … ['Hello,', 'world!'] Job finished successfully.
- Exécutez l'exemple SparkPi pré-installé sur le nœud maître du cluster Dataproc.
Résultat du terminal:gcloud dataproc jobs submit spark \ --cluster=cluster-name \ --region=region \ --class=org.apache.spark.examples.SparkPi \ --jars=file:///usr/lib/spark/examples/jars/spark-examples.jar \ -- 1000
Job [54825071-ae28-4c5b-85a5-58fae6a597d6] submitted. Waiting for job output… … Pi is roughly 3.14177148 … Job finished successfully. …
REST
Cette section explique comment envoyer une tâche Spark pour calculer la valeur approximative de pi
à l'aide de l'API jobs.submit de Dataproc.
Avant d'utiliser les données de requête ci-dessous, effectuez les remplacements suivants :
- project-id: Google Cloud ID du projet
- region : région du cluster
- clusterName : nom du cluster
Méthode HTTP et URL :
POST https://dataproc.googleapis.com/v1/projects/project-id/regions/region/jobs:submit
Corps JSON de la requête :
{ "job": { "placement": { "clusterName": "cluster-name" }, "sparkJob": { "args": [ "1000" ], "mainClass": "org.apache.spark.examples.SparkPi", "jarFileUris": [ "file:///usr/lib/spark/examples/jars/spark-examples.jar" ] } } }
Pour envoyer votre requête, développez l'une des options suivantes :
Vous devriez recevoir une réponse JSON de ce type :
{ "reference": { "projectId": "project-id", "jobId": "job-id" }, "placement": { "clusterName": "cluster-name", "clusterUuid": "cluster-Uuid" }, "sparkJob": { "mainClass": "org.apache.spark.examples.SparkPi", "args": [ "1000" ], "jarFileUris": [ "file:///usr/lib/spark/examples/jars/spark-examples.jar" ] }, "status": { "state": "PENDING", "stateStartTime": "2020-10-07T20:16:21.759Z" }, "jobUuid": "job-Uuid" }
Java
Python
Go
Node.js
Envoyer une tâche directement sur votre cluster
Si vous souhaitez exécuter une tâche directement sur votre cluster sans utiliser le service Dataproc, connectez-vous en SSH au nœud maître de votre cluster, puis exécutez la tâche sur le nœud maître.
Une fois la connexion SSH établie avec l'instance maître de la VM, exécutez des commandes dans une fenêtre de terminal sur le nœud maître du cluster pour effectuer les opérations suivantes :
- Ouvrir une interface système Spark.
- Exécuter une tâche Spark simple permettant de compter le nombre de lignes dans un fichier "hello-world" Python (à sept lignes), situé dans un fichier Cloud Storage accessible au public.
Fermer l'interface système.
user@cluster-name-m:~$ spark-shell ... scala> sc.textFile("gs://dataproc-examples" + "/pyspark/hello-world/hello-world.py").count ... res0: Long = 7 scala> :quit
Exécuter des tâches bash sur Dataproc
Vous souhaiterez peut-être exécuter un script bash en tant que tâche Dataproc, soit parce que les moteurs que vous utilisez ne sont pas acceptés en tant que type de tâche Dataproc de premier niveau, soit parce qu'une configuration ou un calcul d'arguments supplémentaires doit être effectué avant de lancer une tâche à l'aide de hadoop
ou spark-submit
à partir de votre script.
Exemple d'instruction Pig
Supposons que vous ayez copié un script bash "hello.sh" dans Cloud Storage :
gcloud storage cp hello.sh gs://${BUCKET}/hello.sh
Comme la commande pig fs
utilise des chemins Hadoop, copiez le script de Cloud Storage vers une destination spécifiée en tant que file:///
pour vous assurer qu'il se trouve sur le système de fichiers local plutôt que sur HDFS. Les commandes sh
suivantes font automatiquement référence au système de fichiers local et ne nécessitent pas le préfixe file:///
.
gcloud dataproc jobs submit pig --cluster=${CLUSTER} --region=${REGION} \
-e='fs -cp -f gs://${BUCKET}/hello.sh file:///tmp/hello.sh; sh chmod 750 /tmp/hello.sh; sh /tmp/hello.sh'
Étant donné que les tâches Dataproc envoient l'argument --jars
, un fichier est créé dans un répertoire temporaire créé pendant la durée de vie de la tâche, vous pouvez spécifier votre script shell Cloud Storage en tant qu'argument --jars
:
gcloud dataproc jobs submit pig --cluster=${CLUSTER} --region=${REGION} \
--jars=gs://${BUCKET}/hello.sh \
-e='sh chmod 750 ${PWD}/hello.sh; sh ${PWD}/hello.sh'
Notez que l'argument --jars
peut également faire référence à un script local :
gcloud dataproc jobs submit pig --cluster=${CLUSTER} --region=${REGION} \
--jars=hello.sh \
-e='sh chmod 750 ${PWD}/hello.sh; sh ${PWD}/hello.sh'