Konektor BigQuery untuk Apache Spark memungkinkan Data Scientist menggabungkan kecanggihan mesin SQL BigQuery yang skalabel dengan lancar dengan kemampuan Machine Learning Apache Spark. Dalam tutorial ini, kami menunjukkan cara menggunakan Dataproc, BigQuery, dan Apache Spark ML untuk melakukan machine learning pada set data.
Tujuan
Gunakan regresi linear untuk membuat model berat lahir sebagai fungsi dari lima faktor:- minggu masa gestasi
- usia ibu
- usia ayah
- penambahan berat ibu selama kehamilan
- Skor Apgar
Gunakan alat berikut:
- BigQuery, untuk menyiapkan tabel input regresi linear, yang ditulis ke project Google Cloud Anda
- Python, untuk membuat kueri dan mengelola data di BigQuery
- Apache Spark, untuk mengakses tabel regresi linear yang dihasilkan
- Spark ML, untuk membuat dan mengevaluasi model
- Tugas PySpark Dataproc, untuk memanggil fungsi Spark ML
Biaya
Dalam dokumen ini, Anda akan menggunakan komponen Google Cloudyang dapat ditagih berikut:
- Compute Engine
- Dataproc
- BigQuery
Untuk membuat perkiraan biaya berdasarkan proyeksi penggunaan Anda,
gunakan kalkulator harga.
Sebelum memulai
Cluster Dataproc telah menginstal komponen Spark, termasuk Spark ML. Untuk menyiapkan cluster Dataproc dan menjalankan kode dalam contoh ini, Anda harus melakukan (atau telah melakukan) hal berikut:
- Sign in to your Google Cloud account. If you're new to Google Cloud, create an account to evaluate how our products perform in real-world scenarios. New customers also get $300 in free credits to run, test, and deploy workloads.
-
In the Google Cloud console, on the project selector page, select or create a Google Cloud project.
-
Enable the Dataproc, BigQuery, Compute Engine APIs.
- Install the Google Cloud CLI.
-
To initialize the gcloud CLI, run the following command:
gcloud init
-
In the Google Cloud console, on the project selector page, select or create a Google Cloud project.
-
Enable the Dataproc, BigQuery, Compute Engine APIs.
- Install the Google Cloud CLI.
-
To initialize the gcloud CLI, run the following command:
gcloud init
- Buat cluster Dataproc di project Anda. Cluster Anda harus menjalankan versi Dataproc dengan Spark 2.0 atau yang lebih tinggi, (termasuk library machine learning).
Membuat Subkumpulan data natality
BigQuery
Di bagian ini, Anda akan membuat set data dalam project, lalu membuat tabel dalam set data tempat Anda menyalin subkumpulan data rasio kelahiran dari set data BigQuery natalitas yang tersedia untuk publik. Selanjutnya dalam tutorial ini, Anda akan menggunakan data subkumpulan dalam tabel ini untuk memprediksi berat lahir sebagai fungsi dari usia ibu, usia ayah, dan minggu masa gestasi.
Anda dapat membuat subset data menggunakan konsol Google Cloud atau menjalankan skrip Python di komputer lokal.
Konsol
Buat set data di project Anda.
- Buka UI Web BigQuery.
- Di panel navigasi sebelah kiri, klik nama project Anda, lalu klik BUAT SET DATA.
- Pada dialog Create dataset:
- Untuk Dataset ID, masukkan "natality_regression".
- Untuk Data location, Anda dapat memilih
location
untuk set data. Lokasi nilai defaultnya adalah
US multi-region
. Setelah set data dibuat, lokasinya tidak dapat diubah. - Untuk Default table expiration, pilih salah satu opsi berikut:
- Never (default): Anda harus menghapus tabel secara manual.
- Jumlah hari: Tabel akan dihapus setelah jumlah hari yang ditentukan sejak waktu pembuatannya.
- Untuk Enkripsi, pilih salah satu opsi berikut:
- Google-owned and Google-managed encryption key (default).
- Kunci yang dikelola pelanggan: Lihat Melindungi data dengan kunci Cloud KMS.
- Klik Create dataset.
Jalankan kueri terhadap set data kelahiran publik, lalu simpan hasil kueri dalam tabel baru di set data Anda.
- Salin dan tempel kueri berikut ke Editor Kueri, lalu
klik Run.
CREATE OR REPLACE TABLE natality_regression.regression_input as SELECT weight_pounds, mother_age, father_age, gestation_weeks, weight_gain_pounds, apgar_5min FROM `bigquery-public-data.samples.natality` WHERE weight_pounds IS NOT NULL AND mother_age IS NOT NULL AND father_age IS NOT NULL AND gestation_weeks IS NOT NULL AND weight_gain_pounds IS NOT NULL AND apgar_5min IS NOT NULL
- Setelah kueri selesai (sekitar satu menit), hasilnya
akan disimpan sebagai tabel BigQuery "regression_input"
dalam set data
natality_regression
di project Anda.
- Salin dan tempel kueri berikut ke Editor Kueri, lalu
klik Run.
Python
Sebelum mencoba contoh ini, ikuti petunjuk penyiapan Python di panduan memulai Dataproc menggunakan library klien. Untuk mengetahui informasi selengkapnya, lihat dokumentasi referensi API Python Dataproc.
Untuk melakukan autentikasi ke Dataproc, siapkan Kredensial Default Aplikasi. Untuk mengetahui informasi selengkapnya, baca Menyiapkan autentikasi untuk lingkungan pengembangan lokal.
Lihat Menyiapkan Lingkungan Pengembangan Python untuk mengetahui petunjuk cara menginstal Python dan Library Klien Google Cloud untuk Python (diperlukan untuk menjalankan kode). Sebaiknya instal dan gunakan
virtualenv
Python.Salin dan tempel kode
natality_tutorial.py
, di bawah, ke dalam shellpython
di komputer lokal Anda. Tekan tombol<return>
di shell untuk menjalankan kode guna membuat set data BigQuery "natality_regression" di projectGoogle Cloud default Anda dengan tabel "regression_input" yang diisi dengan subkumpulan datanatality
publik.Konfirmasi pembuatan set data
natality_regression
dan tabelregression_input
.
Menjalankan regresi linear
Di bagian ini, Anda akan menjalankan regresi linear PySpark dengan mengirimkan tugas ke layanan Dataproc menggunakan Konsol Google Cloud atau dengan menjalankan perintah gcloud
dari terminal lokal.
Konsol
Salin dan tempel kode berikut ke dalam file
natality_sparkml.py
baru di mesin lokal Anda."""Run a linear regression using Apache Spark ML. In the following PySpark (Spark Python API) code, we take the following actions: * Load a previously created linear regression (BigQuery) input table into our Cloud Dataproc Spark cluster as an RDD (Resilient Distributed Dataset) * Transform the RDD into a Spark Dataframe * Vectorize the features on which the model will be trained * Compute a linear regression using Spark ML """ from pyspark.context import SparkContext from pyspark.ml.linalg import Vectors from pyspark.ml.regression import LinearRegression from pyspark.sql.session import SparkSession # The imports, above, allow us to access SparkML features specific to linear # regression as well as the Vectors types. # Define a function that collects the features of interest # (mother_age, father_age, and gestation_weeks) into a vector. # Package the vector in a tuple containing the label (`weight_pounds`) for that # row. def vector_from_inputs(r): return (r["weight_pounds"], Vectors.dense(float(r["mother_age"]), float(r["father_age"]), float(r["gestation_weeks"]), float(r["weight_gain_pounds"]), float(r["apgar_5min"]))) sc = SparkContext() spark = SparkSession(sc) # Read the data from BigQuery as a Spark Dataframe. natality_data = spark.read.format("bigquery").option( "table", "natality_regression.regression_input").load() # Create a view so that Spark SQL queries can be run against the data. natality_data.createOrReplaceTempView("natality") # As a precaution, run a query in Spark SQL to ensure no NULL values exist. sql_query = """ SELECT * from natality where weight_pounds is not null and mother_age is not null and father_age is not null and gestation_weeks is not null """ clean_data = spark.sql(sql_query) # Create an input DataFrame for Spark ML using the above function. training_data = clean_data.rdd.map(vector_from_inputs).toDF(["label", "features"]) training_data.cache() # Construct a new LinearRegression object and fit the training data. lr = LinearRegression(maxIter=5, regParam=0.2, solver="normal") model = lr.fit(training_data) # Print the model summary. print("Coefficients:" + str(model.coefficients)) print("Intercept:" + str(model.intercept)) print("R^2:" + str(model.summary.r2)) model.summary.residuals.show()
Salin file
natality_sparkml.py
lokal ke bucket Cloud Storage di project Anda.gcloud storage cp natality_sparkml.py gs://bucket-name
Jalankan regresi dari halaman Kirim tugas Dataproc.
Di kolom Main python file, masukkan URI
gs://
dari bucket Cloud Storage tempat salinan filenatality_sparkml.py
Anda berada.Pilih
PySpark
sebagai Jenis tugas.Sisipkan
gs://spark-lib/bigquery/spark-bigquery-latest_2.12.jar
di kolom File jar. Hal ini membuat spark-bigquery-connector tersedia untuk aplikasi PySpark saat runtime agar dapat membaca data BigQuery ke dalam DataFrame Spark.Isi kolom ID Tugas, Wilayah, dan Cluster.
Klik Kirim untuk menjalankan tugas di cluster Anda.
Setelah tugas selesai, ringkasan model output regresi linear akan muncul di jendela detail Tugas Dataproc.
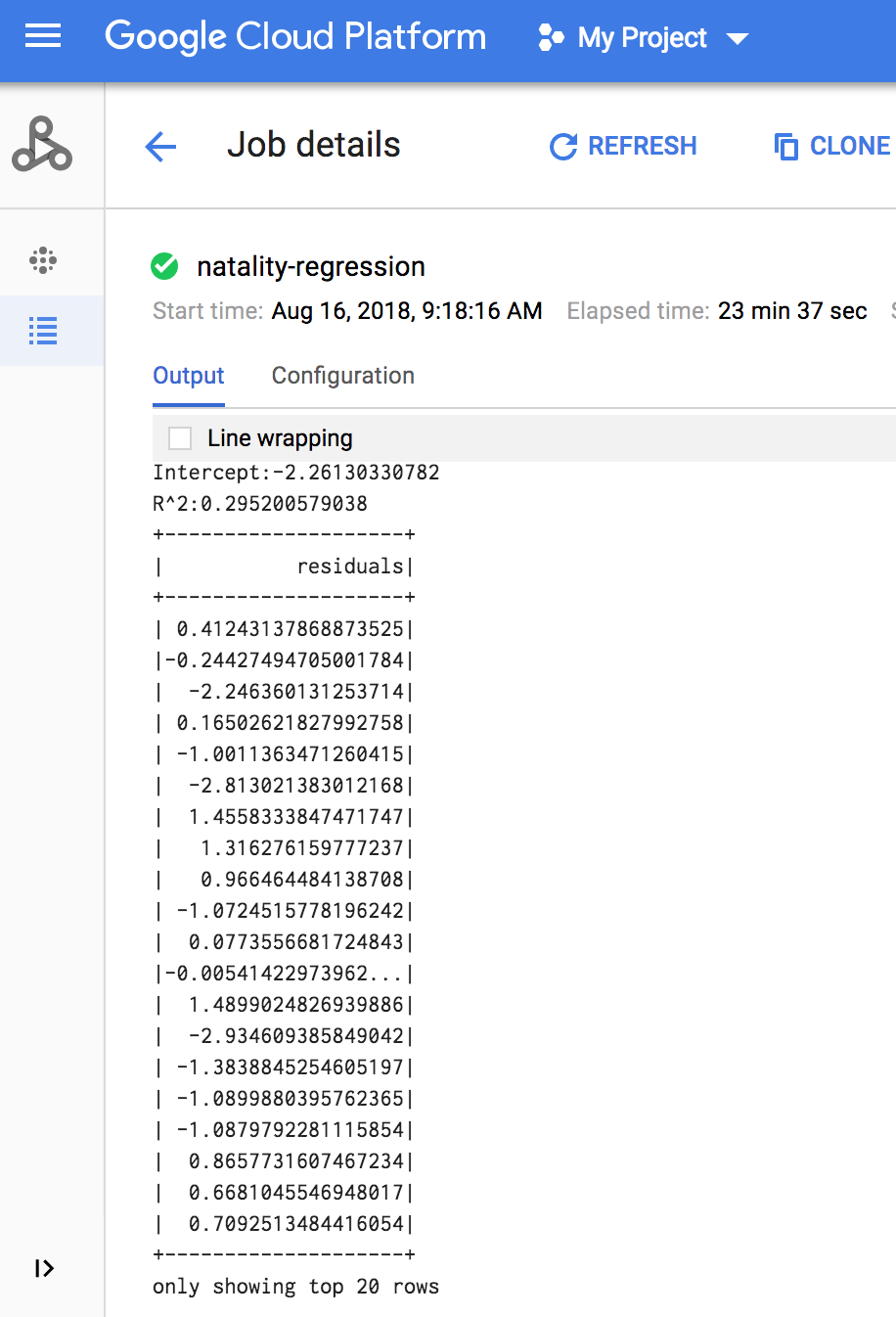
gcloud
Salin dan tempel kode berikut ke dalam file
natality_sparkml.py
baru di mesin lokal Anda."""Run a linear regression using Apache Spark ML. In the following PySpark (Spark Python API) code, we take the following actions: * Load a previously created linear regression (BigQuery) input table into our Cloud Dataproc Spark cluster as an RDD (Resilient Distributed Dataset) * Transform the RDD into a Spark Dataframe * Vectorize the features on which the model will be trained * Compute a linear regression using Spark ML """ from pyspark.context import SparkContext from pyspark.ml.linalg import Vectors from pyspark.ml.regression import LinearRegression from pyspark.sql.session import SparkSession # The imports, above, allow us to access SparkML features specific to linear # regression as well as the Vectors types. # Define a function that collects the features of interest # (mother_age, father_age, and gestation_weeks) into a vector. # Package the vector in a tuple containing the label (`weight_pounds`) for that # row. def vector_from_inputs(r): return (r["weight_pounds"], Vectors.dense(float(r["mother_age"]), float(r["father_age"]), float(r["gestation_weeks"]), float(r["weight_gain_pounds"]), float(r["apgar_5min"]))) sc = SparkContext() spark = SparkSession(sc) # Read the data from BigQuery as a Spark Dataframe. natality_data = spark.read.format("bigquery").option( "table", "natality_regression.regression_input").load() # Create a view so that Spark SQL queries can be run against the data. natality_data.createOrReplaceTempView("natality") # As a precaution, run a query in Spark SQL to ensure no NULL values exist. sql_query = """ SELECT * from natality where weight_pounds is not null and mother_age is not null and father_age is not null and gestation_weeks is not null """ clean_data = spark.sql(sql_query) # Create an input DataFrame for Spark ML using the above function. training_data = clean_data.rdd.map(vector_from_inputs).toDF(["label", "features"]) training_data.cache() # Construct a new LinearRegression object and fit the training data. lr = LinearRegression(maxIter=5, regParam=0.2, solver="normal") model = lr.fit(training_data) # Print the model summary. print("Coefficients:" + str(model.coefficients)) print("Intercept:" + str(model.intercept)) print("R^2:" + str(model.summary.r2)) model.summary.residuals.show()
Salin file
natality_sparkml.py
lokal ke bucket Cloud Storage di project Anda.gcloud storage cp natality_sparkml.py gs://bucket-name
Kirim tugas Pyspark ke layanan Dataproc dengan menjalankan perintah
gcloud
, yang ditampilkan di bawah, dari jendela terminal di komputer lokal Anda.- Nilai flag --jars membuat spark-bigquery-connector tersedia
untuk jobv PySpark saat runtime agar dapat membaca
data BigQuery ke dalam DataFrame Spark.
gcloud dataproc jobs submit pyspark \ gs://your-bucket/natality_sparkml.py \ --cluster=cluster-name \ --region=region \ --jars=gs://spark-lib/bigquery/spark-bigquery-with-dependencies_SCALA_VERSION-CONNECTOR_VERSION.jar
- Nilai flag --jars membuat spark-bigquery-connector tersedia
untuk jobv PySpark saat runtime agar dapat membaca
data BigQuery ke dalam DataFrame Spark.
Output regresi linear (ringkasan model) muncul di jendela terminal saat tugas selesai.
<<< # Cetak ringkasan model. ... print "Coefficients:" + str(model.coefficients) Coefficients:[0.0166657454602,-0.00296751984046,0.235714392936,0.00213002070133,-0.00048577251587] <<< print "Intercept:" + str(model.intercept) Intercept:-2.26130330748 <<< print "R^2:" + str(model.summary.r2) R^2:0.295200579035 <<< model.summary.residuals.show() +--------------------+ | residuals| +--------------------+ | -0.7234737533344147| | -0.985466980630501| | -0.6669710598385468| | 1.4162434829714794| |-0.09373154375186754| |-0.15461747949235072| | 0.32659061654192545| | 1.5053877697929803| | -0.640142797263989| | 1.229530260294963| |-0.03776160295256...| | -0.5160734239126814| | -1.5165972740062887| | 1.3269085258245008| | 1.7604670124710626| | 1.2348130901905972| | 2.318660276655887| | 1.0936947030883175| | 1.0169768511417363| | -1.7744915698181583| +--------------------+ hanya menampilkan 20 baris teratas.
Pembersihan
Setelah menyelesaikan tutorial, Anda dapat membersihkan resource yang dibuat agar resource tersebut berhenti menggunakan kuota dan dikenai biaya. Bagian berikut menjelaskan cara menghapus atau menonaktifkan resource ini.
Menghapus project
Cara termudah untuk menghilangkan penagihan adalah dengan menghapus project yang Anda buat untuk tutorial.
Untuk menghapus project:
- In the Google Cloud console, go to the Manage resources page.
- In the project list, select the project that you want to delete, and then click Delete.
- In the dialog, type the project ID, and then click Shut down to delete the project.
Menghapus cluster Dataproc
Lihat Menghapus cluster.