O Conector do BigQuery para Apache Spark permite que cientistas de dados mesclem a força do mecanismo SQL escalonável e confiável do BigQuery com os recursos de machine learning do Apache Spark. Neste tutorial, mostramos como usar o Dataproc, o BigQuery e o Apache Spark ML para realizar machine learning em um conjunto de dados.
Objetivos
Usar a regressão linear para criar um modelo de peso de nascimento como uma função de cinco fatores:- semanas de gestação
- idade da mãe
- idade do pai
- ganho de peso da mãe durante a gravidez
- escala de Apgar
Use as seguintes ferramentas:
- BigQuery, para preparar a tabela de entrada de regressão linear, que é gravada no projeto Google Cloud .
- Python, para consultar e gerenciar dados no BigQuery
- Apache Spark, para acessar a tabela de regressão linear resultante
- Spark ML, para criar e avaliar o modelo
- Job do Dataproc PySpark para invocar funções do Spark ML
Custos
Neste documento, você usará os seguintes componentes faturáveis do Google Cloud:
- Compute Engine
- Dataproc
- BigQuery
Para gerar uma estimativa de custo baseada na projeção de uso deste tutorial, use a calculadora de preços.
Antes de começar
Um cluster do Dataproc tem os componentes do Spark, inclusive o Spark ML, instalados. Para configurar um cluster do Dataproc e executar o código deste exemplo, você precisará fazer (ou ter feito) o seguinte:
- Sign in to your Google Cloud account. If you're new to Google Cloud, create an account to evaluate how our products perform in real-world scenarios. New customers also get $300 in free credits to run, test, and deploy workloads.
-
In the Google Cloud console, on the project selector page, select or create a Google Cloud project.
-
Enable the Dataproc, BigQuery, Compute Engine APIs.
- Install the Google Cloud CLI.
-
To initialize the gcloud CLI, run the following command:
gcloud init
-
In the Google Cloud console, on the project selector page, select or create a Google Cloud project.
-
Enable the Dataproc, BigQuery, Compute Engine APIs.
- Install the Google Cloud CLI.
-
To initialize the gcloud CLI, run the following command:
gcloud init
- Crie um cluster do Dataproc no projeto. Seu cluster precisa executar uma versão do Dataproc com Spark 2.0 ou superior, incluindo bibliotecas de machine learning.
Criar um subconjunto de dados do BigQuery natality
Nesta seção, você cria um conjunto de dados no projeto e uma tabela no conjunto de dados para que queira copiar um subconjunto de dados da taxa de natalidade do conjunto de dados natality do BigQuery disponível publicamente. Posteriormente, neste tutorial, você usará os dados do subconjunto nesta tabela para prever o peso de nascimento como uma função da idade materna, da idade paterna e das semanas de gestação.
É possível criar o subconjunto de dados usando o console do Google Cloud ou executando um script Python na máquina local.
Console
Crie um conjunto de dados no projeto.
- Acesse a IU da Web do BigQuery.
- No painel de navegação esquerdo, clique no nome do projeto e em CRIAR CONJUNTO DE DADOS.
- Na caixa de diálogo Criar conjunto de dados:
- Em ID do conjunto de dados, digite "natality_regression".
- Em Local de dados, escolha um
local
para o conjunto de dados. O local do valor padrão é
US multi-region
. Depois que um conjunto de dados é criado, o local não pode ser alterado. - Em Validade da tabela padrão, escolha uma das seguintes opções:
- Nunca (padrão): você deve excluir a tabela manualmente.
- Número de dias: a tabela será excluída após o número de dias especificado a partir da data de criação.
- Para Criptografia, escolha uma das seguintes opções:
- Google-owned and Google-managed encryption key (padrão).
- Chave gerenciada pelo cliente: consulte Como proteger dados com chaves do Cloud KMS.
- Clique em Criar conjunto de dados.
Execute uma consulta no conjunto de dados de natalidade pública e salve os resultados da consulta em uma nova tabela no conjunto de dados.
- Copie e cole a seguinte consulta no Editor de Consultas e
clique em Executar.
CREATE OR REPLACE TABLE natality_regression.regression_input as SELECT weight_pounds, mother_age, father_age, gestation_weeks, weight_gain_pounds, apgar_5min FROM `bigquery-public-data.samples.natality` WHERE weight_pounds IS NOT NULL AND mother_age IS NOT NULL AND father_age IS NOT NULL AND gestation_weeks IS NOT NULL AND weight_gain_pounds IS NOT NULL AND apgar_5min IS NOT NULL
- Depois que a consulta for concluída (em aproximadamente um minuto), os resultados
serão salvos como a tabela "regression_input" do tabela do BigQuery
no conjunto de dados
natality_regression
do seu projeto.
- Copie e cole a seguinte consulta no Editor de Consultas e
clique em Executar.
Python
Antes de testar este exemplo, siga as instruções de configuração do Python no Guia de início rápido do Dataproc: como usar bibliotecas de cliente. Para mais informações, consulte a documentação de referência da API Python do Dataproc.
Para autenticar no Dataproc, configure o Application Default Credentials. Para mais informações, consulte Configurar a autenticação para um ambiente de desenvolvimento local.
Consulte Como configurar um ambiente de desenvolvimento do Python para ver instruções sobre como instalar o Python e a biblioteca de cliente do Google Cloud para Python (necessária para executar o código). É recomendado instalar e usar um
virtualenv
do Python.Copie e cole o código
natality_tutorial.py
abaixo em um shellpython
na máquina local. Pressione o botão<return>
no shell para executar o código e criar um conjunto de dados "natality_regression" do BigQuery no seu projeto padrão Google Cloud com uma tabela "regression_input" preenchida com um subconjunto dos dadosnatality
públicos.Confirme a criação do conjunto de dados
natality_regression
e da tabelaregression_input
.
Executar uma regressão linear
Nesta seção, você vai executar uma regressão linear do PySpark enviando
o job ao serviço do Dataproc usando o console do Google Cloud
ou executando o comando gcloud
em um terminal local.
Console
Copie e cole o seguinte código em um novo arquivo
natality_sparkml.py
na máquina local."""Run a linear regression using Apache Spark ML. In the following PySpark (Spark Python API) code, we take the following actions: * Load a previously created linear regression (BigQuery) input table into our Cloud Dataproc Spark cluster as an RDD (Resilient Distributed Dataset) * Transform the RDD into a Spark Dataframe * Vectorize the features on which the model will be trained * Compute a linear regression using Spark ML """ from pyspark.context import SparkContext from pyspark.ml.linalg import Vectors from pyspark.ml.regression import LinearRegression from pyspark.sql.session import SparkSession # The imports, above, allow us to access SparkML features specific to linear # regression as well as the Vectors types. # Define a function that collects the features of interest # (mother_age, father_age, and gestation_weeks) into a vector. # Package the vector in a tuple containing the label (`weight_pounds`) for that # row. def vector_from_inputs(r): return (r["weight_pounds"], Vectors.dense(float(r["mother_age"]), float(r["father_age"]), float(r["gestation_weeks"]), float(r["weight_gain_pounds"]), float(r["apgar_5min"]))) sc = SparkContext() spark = SparkSession(sc) # Read the data from BigQuery as a Spark Dataframe. natality_data = spark.read.format("bigquery").option( "table", "natality_regression.regression_input").load() # Create a view so that Spark SQL queries can be run against the data. natality_data.createOrReplaceTempView("natality") # As a precaution, run a query in Spark SQL to ensure no NULL values exist. sql_query = """ SELECT * from natality where weight_pounds is not null and mother_age is not null and father_age is not null and gestation_weeks is not null """ clean_data = spark.sql(sql_query) # Create an input DataFrame for Spark ML using the above function. training_data = clean_data.rdd.map(vector_from_inputs).toDF(["label", "features"]) training_data.cache() # Construct a new LinearRegression object and fit the training data. lr = LinearRegression(maxIter=5, regParam=0.2, solver="normal") model = lr.fit(training_data) # Print the model summary. print("Coefficients:" + str(model.coefficients)) print("Intercept:" + str(model.intercept)) print("R^2:" + str(model.summary.r2)) model.summary.residuals.show()
Copie o arquivo
natality_sparkml.py
local para um bucket do Cloud Storage no seu projeto.gcloud storage cp natality_sparkml.py gs://bucket-name
Execute a regressão na página Enviar um job do Dataproc.
No campo Arquivo principal python, insira o URI
gs://
do bucket do Cloud Storage no qual sua cópia do arquivonatality_sparkml.py
está localizada.Selecione
PySpark
como o Tipo de job.Insira
gs://spark-lib/bigquery/spark-bigquery-latest_2.12.jar
no campo Arquivos Jar. Isso torna o conector spark-bigquery disponível para o aplicativo PySpark no ambiente de execução para permitir a leitura de dados do BigQuery em um Spark DataFrame.Preencha os campos ID do job, Região e Cluster.
Clique em Enviar para executar o job no cluster.
Quando o job é concluído, o resumo do modelo de saída de regressão linear é exibido na janela de detalhes Job do Dataproc.
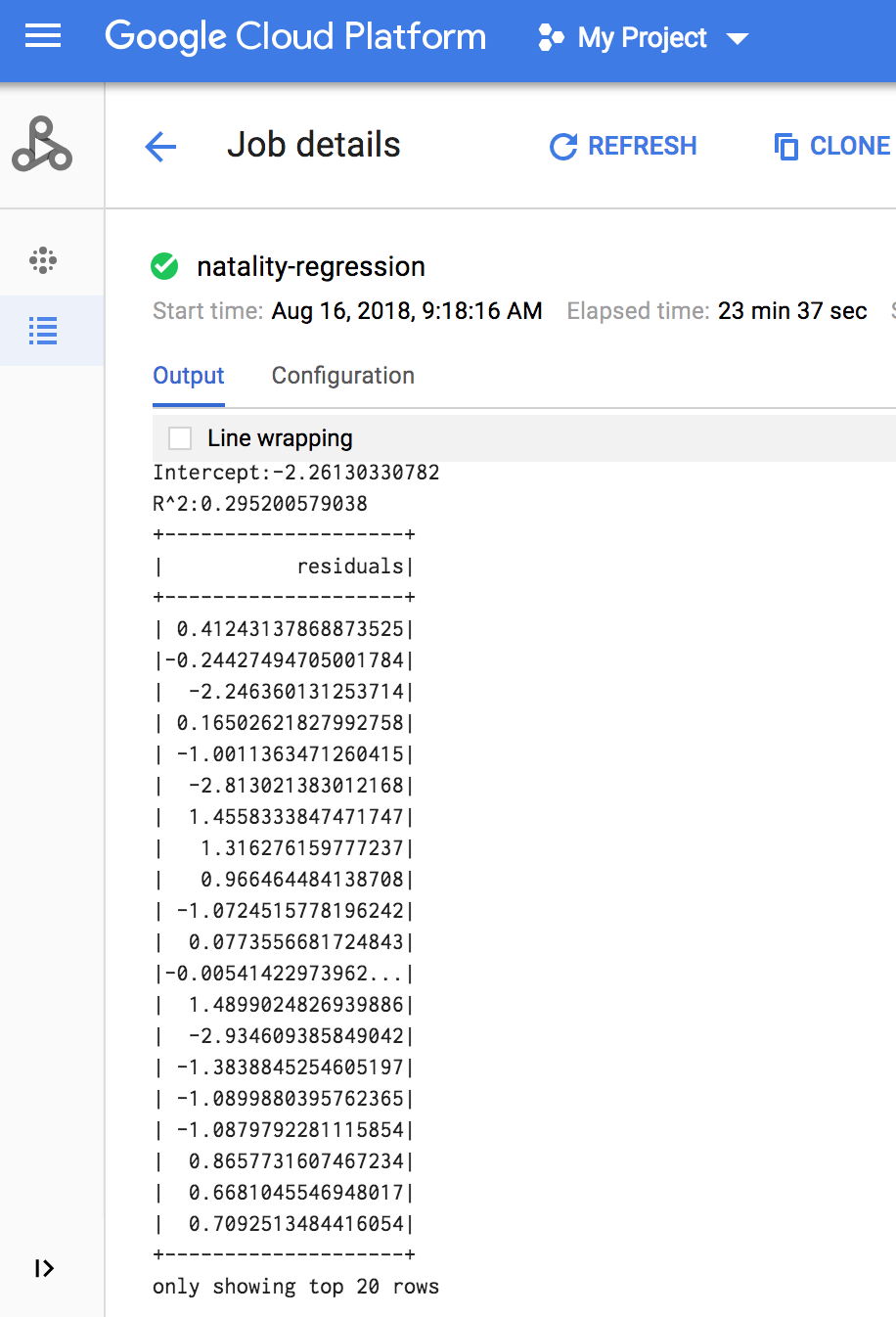
gcloud
Copie e cole o seguinte código em um novo arquivo
natality_sparkml.py
na máquina local."""Run a linear regression using Apache Spark ML. In the following PySpark (Spark Python API) code, we take the following actions: * Load a previously created linear regression (BigQuery) input table into our Cloud Dataproc Spark cluster as an RDD (Resilient Distributed Dataset) * Transform the RDD into a Spark Dataframe * Vectorize the features on which the model will be trained * Compute a linear regression using Spark ML """ from pyspark.context import SparkContext from pyspark.ml.linalg import Vectors from pyspark.ml.regression import LinearRegression from pyspark.sql.session import SparkSession # The imports, above, allow us to access SparkML features specific to linear # regression as well as the Vectors types. # Define a function that collects the features of interest # (mother_age, father_age, and gestation_weeks) into a vector. # Package the vector in a tuple containing the label (`weight_pounds`) for that # row. def vector_from_inputs(r): return (r["weight_pounds"], Vectors.dense(float(r["mother_age"]), float(r["father_age"]), float(r["gestation_weeks"]), float(r["weight_gain_pounds"]), float(r["apgar_5min"]))) sc = SparkContext() spark = SparkSession(sc) # Read the data from BigQuery as a Spark Dataframe. natality_data = spark.read.format("bigquery").option( "table", "natality_regression.regression_input").load() # Create a view so that Spark SQL queries can be run against the data. natality_data.createOrReplaceTempView("natality") # As a precaution, run a query in Spark SQL to ensure no NULL values exist. sql_query = """ SELECT * from natality where weight_pounds is not null and mother_age is not null and father_age is not null and gestation_weeks is not null """ clean_data = spark.sql(sql_query) # Create an input DataFrame for Spark ML using the above function. training_data = clean_data.rdd.map(vector_from_inputs).toDF(["label", "features"]) training_data.cache() # Construct a new LinearRegression object and fit the training data. lr = LinearRegression(maxIter=5, regParam=0.2, solver="normal") model = lr.fit(training_data) # Print the model summary. print("Coefficients:" + str(model.coefficients)) print("Intercept:" + str(model.intercept)) print("R^2:" + str(model.summary.r2)) model.summary.residuals.show()
Copie o arquivo
natality_sparkml.py
local para um bucket do Cloud Storage no seu projeto.gcloud storage cp natality_sparkml.py gs://bucket-name
Envie o job Pyspark para o serviço Dataproc executando o comando
gcloud
, mostrado abaixo, a partir de uma janela de terminal na máquina local.- O valor da sinalização --jars torna o conector spark-bigquery disponível
para o jobv do PySpark no ambiente de execução para permitir a leitura de
dados do BigQuery em um Spark DataFrame.
gcloud dataproc jobs submit pyspark \ gs://your-bucket/natality_sparkml.py \ --cluster=cluster-name \ --region=region \ --jars=gs://spark-lib/bigquery/spark-bigquery-with-dependencies_SCALA_VERSION-CONNECTOR_VERSION.jar
- O valor da sinalização --jars torna o conector spark-bigquery disponível
para o jobv do PySpark no ambiente de execução para permitir a leitura de
dados do BigQuery em um Spark DataFrame.
A saída de regressão linear (resumo do modelo) é exibida na janela do terminal quando o job é concluído.
<<< # Imprime o resumo do modelo. ... print "Coefficients:" + str(model.coefficients) Coefficients:[0.0166657454602,-0.00296751984046,0.235714392936,0.00213002070133,-0.00048577251587] <<< print "Intercept:" + str(model.intercept) Intercept:-2.26130330748 <<< print "R^2:" + str(model.summary.r2) R^2:0.295200579035 <<< model.summary.residuals.show() +--------------------+ | residuals| +--------------------+ | -0.7234737533344147| | -0.985466980630501| | -0.6669710598385468| | 1.4162434829714794| |-0.09373154375186754| |-0.15461747949235072| | 0.32659061654192545| | 1.5053877697929803| | -0.640142797263989| | 1.229530260294963| |-0.03776160295256...| | -0.5160734239126814| | -1.5165972740062887| | 1.3269085258245008| | 1.7604670124710626| | 1.2348130901905972| | 2.318660276655887| | 1.0936947030883175| | 1.0169768511417363| | -1.7744915698181583| +--------------------+ mostrando apenas as 20 primeiras linhas.
Limpar
Depois de concluir o tutorial, você pode limpar os recursos que criou para que eles parem de usar a cota e gerar cobranças. Nas seções a seguir, você aprenderá a excluir e desativar esses recursos.
Excluir o projeto
O jeito mais fácil de evitar cobranças é excluindo o projeto que você criou para o tutorial.
Para excluir o projeto:
- In the Google Cloud console, go to the Manage resources page.
- In the project list, select the project that you want to delete, and then click Delete.
- In the dialog, type the project ID, and then click Shut down to delete the project.
Como excluir o cluster do Dataproc
Consulte Excluir um cluster.