Sie können einen Job über eine Dataproc API als HTTP- oder programmatische Anfrage (jobs.submit) an einen vorhandenen Dataproc-Cluster übergeben. Verwenden Sie dazu das gcloud
-Befehlszeilentool der Google Cloud CLI in einem lokalen Terminalfenster oder in Cloud Shell oder aus der Google Cloud -Konsole in einem lokalen Browser. Sie können auch eine SSH-Verbindung zur Masterinstanz in Ihrem Cluster herstellen und dann einen Job direkt über die Instanz ausführen, ohne den Dataproc-Dienst zu verwenden.
So reichen Sie einen Job ein
Console
Öffnen Sie die Dataproc-Seite Job senden in der Google Cloud -Konsole in Ihrem Browser.
Spark-Job – Beispiel
Zum Senden eines Spark-Beispieljobs füllen Sie die Felder auf der Seite Submit a job (Job senden) aus. Gehen Sie dabei so vor:
- Wählen Sie den Namen des Clusters aus der Clusterliste aus.
- Legen Sie für Job type (Jobtyp) den Wert
Spark
fest. - Legen Sie für Main class or jar (Hauptklasse oder JAR-Datei) den Wert
org.apache.spark.examples.SparkPi
fest. - Legen Sie für Arguments (Argumente) das einzelne Argument
1000
fest. - Fügen Sie
file:///usr/lib/spark/examples/jars/spark-examples.jar
zu Jar-Dateien hinzu:file:///
gibt ein Hadoop LocalFileSystem-Schema an./usr/lib/spark/examples/jars/spark-examples.jar
wurde von Dataproc auf dem Masterknoten des Clusters installiert, als der Cluster erstellt wurde.- Alternativ können Sie einen Cloud Storage-Pfad (
gs://your-bucket/your-jarfile.jar
) oder einen Hadoop Distributed File System-Pfad (hdfs://path-to-jar.jar
) zu einer Ihrer JAR-Dateien angeben.
Klicken Sie auf Submit (Senden), um den Job zu starten. Nach dem Start wird der Job der Jobliste hinzugefügt.
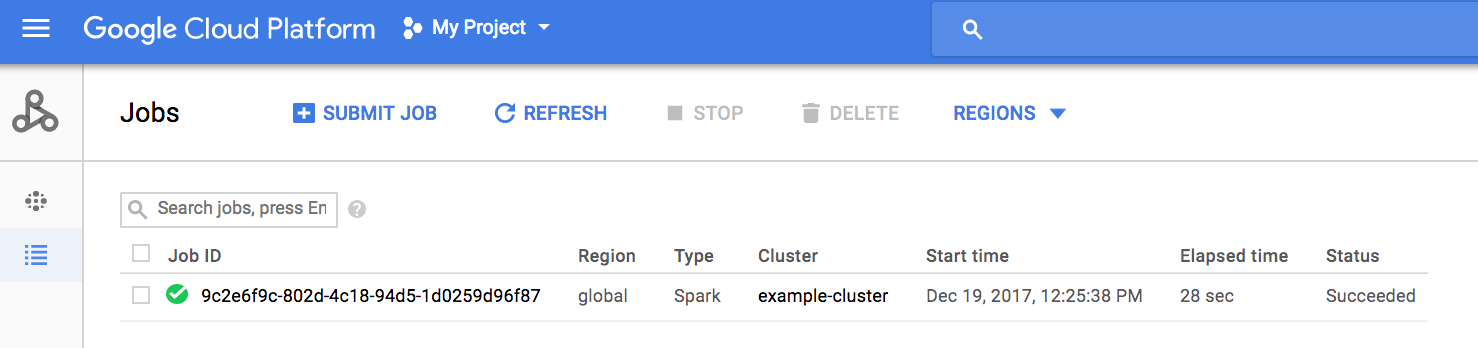
Klicken Sie auf die Job-ID, um die Seite Jobs zu öffnen, auf der Sie die Treiberausgabe des Jobs anzeigen können. Da die generierten Ausgabezeilen die Breite des Browserfensters überschreiten, klicken Sie auf das Kästchen Zeilenumbruch, um den gesamten Ausgabetext in der Ansicht darzustellen und das berechnete Ergebnis für pi
einzublenden.
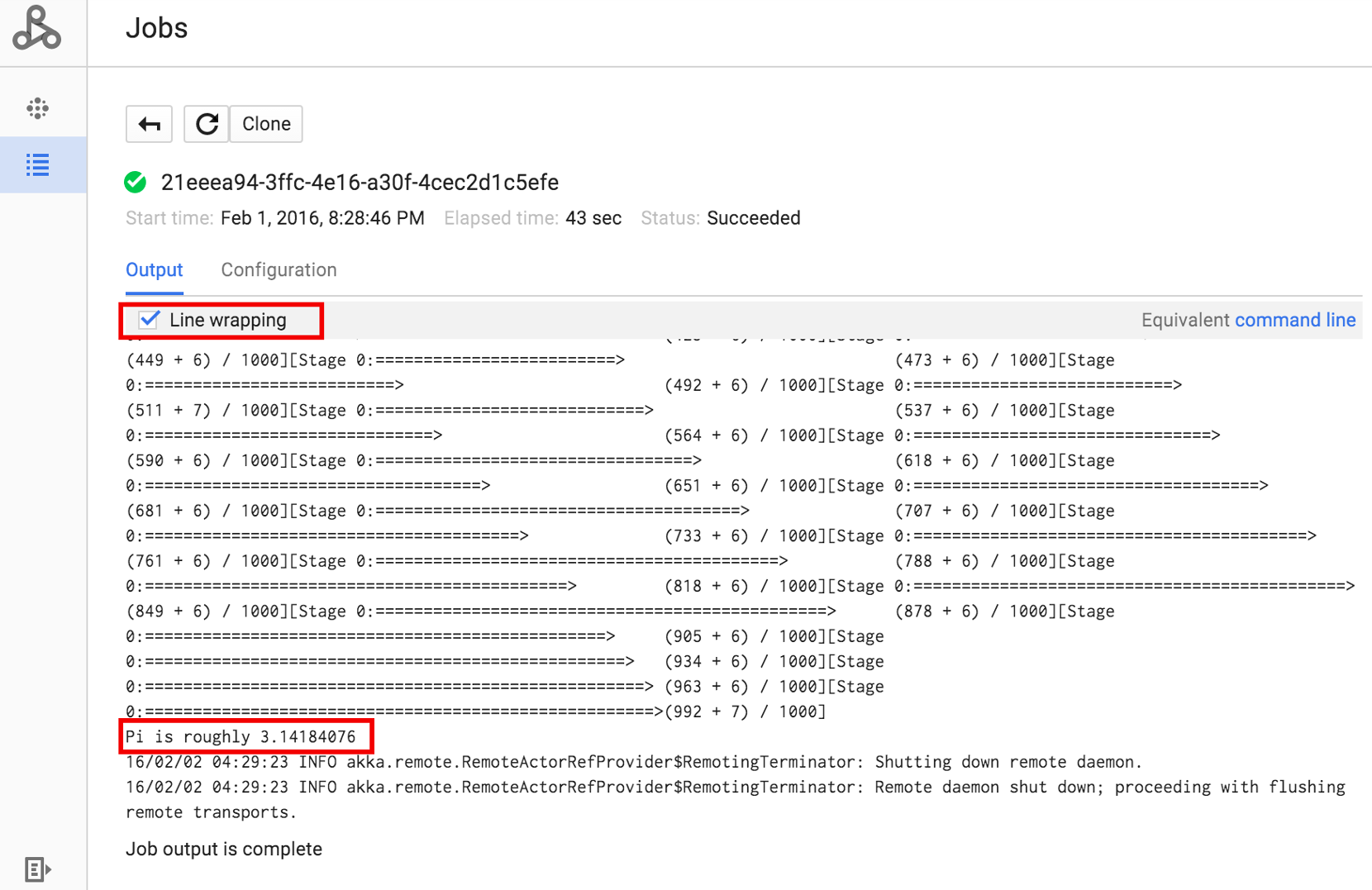
Sie können die Treiberausgabe des Jobs über die Befehlszeile mit dem unten gezeigten Befehl gcloud dataproc jobs wait aufrufen. Weitere Informationen finden Sie unter Jobausgabe ansehen – GCLOUD-BEFEHL.
Kopieren Sie die Projekt-ID und fügen Sie sie als den Wert für das Flag --project
ein. Kopieren Sie anschließend die Job-ID (in der Jobliste angezeigt) und fügen Sie sie als endgültiges Argument ein.
gcloud dataproc jobs wait job-id \ --project=project-id \ --region=region
Hier sind Snippets aus der Treiberausgabe für den oben gesendeten Beispieljob SparkPi
:
... 2015-06-25 23:27:23,810 INFO [dag-scheduler-event-loop] scheduler.DAGScheduler (Logging.scala:logInfo(59)) - Stage 0 (reduce at SparkPi.scala:35) finished in 21.169 s 2015-06-25 23:27:23,810 INFO [task-result-getter-3] cluster.YarnScheduler (Logging.scala:logInfo(59)) - Removed TaskSet 0.0, whose tasks have all completed, from pool 2015-06-25 23:27:23,819 INFO [main] scheduler.DAGScheduler (Logging.scala:logInfo(59)) - Job 0 finished: reduce at SparkPi.scala:35, took 21.674931 s Pi is roughly 3.14189648 ... Job [c556b47a-4b46-4a94-9ba2-2dcee31167b2] finished successfully. driverOutputUri: gs://sample-staging-bucket/google-cloud-dataproc-metainfo/cfeaa033-749e-48b9-... ...
gcloud
Zum Senden eines Jobs an einen Dataproc-Cluster führen Sie den Befehl gcloud dataproc jobs submit der gcloud CLI lokal in einem Terminalfenster oder in Cloud Shell aus.
gcloud dataproc jobs submit job-command \ --cluster=cluster-name \ --region=region \ other dataproc-flags \ -- job-args
- Listen Sie das öffentlich zugängliche
hello-world.py
in Cloud Storage auf. Dateiliste:gcloud storage cat gs://dataproc-examples/pyspark/hello-world/hello-world.py
#!/usr/bin/python import pyspark sc = pyspark.SparkContext() rdd = sc.parallelize(['Hello,', 'world!']) words = sorted(rdd.collect()) print(words)
- Senden Sie den Pyspark-Job an Dataproc.
Terminalausgabe:gcloud dataproc jobs submit pyspark \ gs://dataproc-examples/pyspark/hello-world/hello-world.py \ --cluster=cluster-name \ --region=region
Waiting for job output... … ['Hello,', 'world!'] Job finished successfully.
- Führen Sie das vorinstallierte SparkPi-Beispiel auf dem Masterknoten des Dataproc-Clusters aus.
Terminalausgabe:gcloud dataproc jobs submit spark \ --cluster=cluster-name \ --region=region \ --class=org.apache.spark.examples.SparkPi \ --jars=file:///usr/lib/spark/examples/jars/spark-examples.jar \ -- 1000
Job [54825071-ae28-4c5b-85a5-58fae6a597d6] submitted. Waiting for job output… … Pi is roughly 3.14177148 … Job finished successfully. …
REST
In diesem Abschnitt wird gezeigt, wie Sie einen Spark-Job senden, um den ungefähren Wert von pi
mithilfe der Dataproc-API jobs.submit zu berechnen.
Ersetzen Sie diese Werte in den folgenden Anfragedaten:
- project-id: Google Cloud Projekt-ID
- region: Cluster-Region
- clusterName: Clustername
HTTP-Methode und URL:
POST https://dataproc.googleapis.com/v1/projects/project-id/regions/region/jobs:submit
JSON-Text anfordern:
{ "job": { "placement": { "clusterName": "cluster-name" }, "sparkJob": { "args": [ "1000" ], "mainClass": "org.apache.spark.examples.SparkPi", "jarFileUris": [ "file:///usr/lib/spark/examples/jars/spark-examples.jar" ] } } }
Wenn Sie die Anfrage senden möchten, maximieren Sie eine der folgenden Optionen:
Sie sollten in etwa folgende JSON-Antwort erhalten:
{ "reference": { "projectId": "project-id", "jobId": "job-id" }, "placement": { "clusterName": "cluster-name", "clusterUuid": "cluster-Uuid" }, "sparkJob": { "mainClass": "org.apache.spark.examples.SparkPi", "args": [ "1000" ], "jarFileUris": [ "file:///usr/lib/spark/examples/jars/spark-examples.jar" ] }, "status": { "state": "PENDING", "stateStartTime": "2020-10-07T20:16:21.759Z" }, "jobUuid": "job-Uuid" }
Java
- Clientbibliothek installieren
- Standardanmeldedaten für Anwendungen einrichten
- Führen Sie den Code aus
Python
- Clientbibliothek installieren
- Standardanmeldedaten für Anwendungen einrichten
- Führen Sie den Code aus
Go
Node.js
- Clientbibliothek installieren
- Standardanmeldedaten für Anwendungen einrichten
- Führen Sie den Code aus.
Job direkt an Cluster senden
Wenn Sie einen Job direkt auf Ihrem Cluster ohne den Dataproc-Dienst ausführen möchten, stellen Sie eine SSH-Verbindung zum Masterknoten Ihres Clusters her und führen Sie den Job dann auf dem Masterknoten aus.
Nachdem Sie eine SSH-Verbindung zur VM-Masterinstanz hergestellt haben, führen Sie die folgenden Schritte in einem Terminalfenster im Masterknoten des Clusters aus:
- Öffnen Sie eine Spark-Shell.
- Führen Sie einen einfachen Spark-Job aus, um die Anzahl der Zeilen in einer (siebenzeiligen) Python-Datei "hello-world" zu zählen, die sich in einer öffentlich zugänglichen Cloud Storage-Datei befindet.
Beenden Sie die Shell.
user@cluster-name-m:~$ spark-shell ... scala> sc.textFile("gs://dataproc-examples" + "/pyspark/hello-world/hello-world.py").count ... res0: Long = 7 scala> :quit
Bash-Jobs auf Dataproc ausführen
Möglicherweise möchten Sie ein Bash-Skript als Dataproc-Job ausführen, weil die von Ihnen verwendeten Suchmaschinen nicht als Dataproc-Job auf oberster Ebene unterstützt werden oder weil Sie vor dem Start eines Jobs mit hadoop
oder spark-submit
aus dem Skript zusätzliche Einrichtungsschritte oder Berechnung von Argumenten vornehmen müssen.
Pig-Beispiel
Angenommen, Sie haben ein hello.sh-Bash-Skript in Cloud Storage kopiert:
gcloud storage cp hello.sh gs://${BUCKET}/hello.sh
Da der Befehl pig fs
Hadoop-Pfade verwendet, kopieren Sie das Skript aus Cloud Storage an ein Ziel als file:///
, damit es sich im lokalen Dateisystem statt in HDFS befindet. Die nachfolgenden sh
-Befehle verweisen automatisch auf das lokale Dateisystem und erfordern nicht das Präfix file:///
.
gcloud dataproc jobs submit pig --cluster=${CLUSTER} --region=${REGION} \
-e='fs -cp -f gs://${BUCKET}/hello.sh file:///tmp/hello.sh; sh chmod 750 /tmp/hello.sh; sh /tmp/hello.sh'
Alternativ können Sie das Cloud Storage-Shell-Skript als Argument --jars
angeben, da das Dataproc-Jobübermittlungsargument --jars
eine Datei in ein temporäres Verzeichnis stellt, das für die Lebensdauer des Jobs erstellt wurde:
gcloud dataproc jobs submit pig --cluster=${CLUSTER} --region=${REGION} \
--jars=gs://${BUCKET}/hello.sh \
-e='sh chmod 750 ${PWD}/hello.sh; sh ${PWD}/hello.sh'
Beachten Sie, dass das Argument --jars
auch auf ein lokales Skript verweisen kann:
gcloud dataproc jobs submit pig --cluster=${CLUSTER} --region=${REGION} \
--jars=hello.sh \
-e='sh chmod 750 ${PWD}/hello.sh; sh ${PWD}/hello.sh'