Cloud Composer 3 | Cloud Composer 2 | Cloud Composer 1
이 튜토리얼에서는 Cloud Composer를 사용하여 Dataproc 클러스터에서 Apache Hadoop 워드카운트 작업을 실행하는 Apache Airflow DAG(방향성 비순환 그래프)를 만드는 방법을 보여줍니다.
목표
- Cloud Composer 환경에 액세스하여 Airflow UI를 사용하기
- Airflow 환경 변수를 만들고 열람하기
- 다음 작업이 포함된 DAG를 만들고 실행하기
- Dataproc 클러스터를 만듭니다.
- 클러스터에서 Apache Hadoop 워드카운트 작업을 실행합니다.
- 워드카운트 결과를 Cloud Storage 버킷에 출력합니다.
- 클러스터를 삭제합니다.
비용
이 문서에서는 비용이 청구될 수 있는 다음과 같은 Google Cloud 구성요소를 사용합니다.
- Cloud Composer
- Dataproc
- Cloud Storage
프로젝트 사용량을 기준으로 예상 비용을 산출하려면 가격 계산기를 사용하세요.
시작하기 전에
프로젝트에 다음 API가 사용 설정되어 있는지 확인합니다.
콘솔
Enable the Dataproc, Cloud Storage APIs.
gcloud
Enable the Dataproc, Cloud Storage APIs:
gcloud services enable dataproc.googleapis.com
storage-component.googleapis.com 프로젝트에서 스토리지 클래스와 리전에 상관없이 Hadoop 워드카운트 작업 결과를 저장할 Cloud Storage 버킷을 만듭니다.
만든 버킷의 경로를 기록합니다(예:
gs://example-bucket
). 이 경로에 Airflow 변수를 정의하고 이 튜토리얼 후반부의 DAG 예시에서 변수를 사용합니다.기본 매개변수로 Cloud Composer 환경을 만듭니다. 환경 생성이 완료될 때까지 기다립니다. 완료되면 환경 이름 왼쪽에 녹색 체크표시가 표시됩니다.
환경을 만든 리전을 기록합니다(예:
us-central
). 이 리전의 Airflow 변수를 정의하고 예시 DAG에서 사용하여 같은 리전에서 Dataproc 클러스터를 실행합니다.
Airflow 변수 설정
나중에 예시 DAG에서 사용할 Airflow 변수를 설정합니다. 예를 들어 Airflow UI에서 Airflow 변수를 설정할 수 있습니다.
Airflow 변수 | 값 |
---|---|
gcp_project
|
이 튜토리얼에서 사용하는 프로젝트의 프로젝트 ID입니다(예: example-project ). |
gcs_bucket
|
이 튜토리얼용으로 만든 URI Cloud Storage 버킷입니다(예: gs://example-bucket ). |
gce_region
|
환경을 만든 리전입니다(예: us-central1 ).
이 리전은 Dataproc 클러스터를 만들 리전입니다. |
예시 워크플로 보기
Airflow DAG는 예약 및 실행하려는 태스크가 구성된 모음입니다. DAG는 표준 Python 파일에서 정의됩니다. hadoop_tutorial.py
에 나와 있는 코드는 워크플로 코드입니다.
Airflow 2
Airflow 1
연산자
예시 워크플로에서 태스크 3개를 조정하기 위해 DAG는 다음 Airflow 연산자 3개를 가져옵니다.
DataprocClusterCreateOperator
: Dataproc 클러스터를 만듭니다.DataProcHadoopOperator
: Hadoop 워드카운트 작업을 제출하고 결과를 Cloud Storage 버킷에 기록합니다.DataprocClusterDeleteOperator
: Compute Engine 요금이 청구되지 않도록 클러스터를 삭제합니다.
종속 항목
실행할 태스크를 관계 및 종속 항목을 반영하는 방식으로 구성합니다. 이 DAG의 태스크는 순차적으로 실행됩니다.
Airflow 2
Airflow 1
예약
DAG 이름은 composer_hadoop_tutorial
이며 DAG는 매일 1회 실행됩니다. default_dag_args
로 전달된 start_date
가 yesterday
로 설정되어 있으므로 Cloud Composer는 DAG가 환경 버킷에 업로드된 직후에 워크플로가 시작되도록 예약합니다.
Airflow 2
Airflow 1
환경 버킷에 DAG 업로드
Cloud Composer는 환경 버킷의 /dags
폴더에 DAG를 저장합니다.
DAG를 업로드하려면 다음 안내를 따르세요.
로컬 머신에서
hadoop_tutorial.py
를 저장합니다.Google Cloud 콘솔에서 환경 페이지로 이동합니다.
환경 목록에 있는 환경의 DAG 폴더 열에서 DAG 링크를 클릭합니다.
파일 업로드를 클릭합니다.
로컬 머신에서
hadoop_tutorial.py
를 선택하고 열기를 클릭합니다.
Cloud Composer는 DAG를 Airflow에 추가하고 DAG를 자동으로 예약합니다. DAG 변경사항은 3~5분 이내에 적용됩니다.
DAG 실행 살펴보기
태스크 상태 보기
DAG 파일을 Cloud Storage의 dags/
폴더에 업로드하면 Cloud Composer가 파일을 파싱합니다. 작업이 성공적으로 완료되면 워크플로 이름이 DAG 목록에 나타나고 워크플로가 즉시 실행되도록 큐에 추가됩니다.
태스크 상태를 보려면 Airflow 웹 인터페이스로 이동하고 툴바에서 DAGs를 클릭합니다.
DAG 세부정보 페이지를 열려면
composer_hadoop_tutorial
을 클릭합니다. 이 페이지에서는 워크플로 태스크와 종속 항목이 그래픽으로 표시됩니다.각 태스크의 상태를 확인하려면 그래프 뷰를 클릭한 후 각 태스크의 그래픽에 마우스를 가져갑니다.
워크플로를 다시 큐에 추가
그래프 뷰에서 워크플로를 다시 실행하려면 다음 안내를 따르세요.
- Airflow UI 그래프 뷰에서
create_dataproc_cluster
그래픽을 클릭합니다. - 태스크 세 개를 재설정하려면 삭제를 클릭한 후 확인을 클릭하여 확인합니다.
- 그래프 뷰에서
create_dataproc_cluster
를 다시 클릭합니다. - 워크플로를 다시 큐에 추가하려면 실행을 클릭합니다.
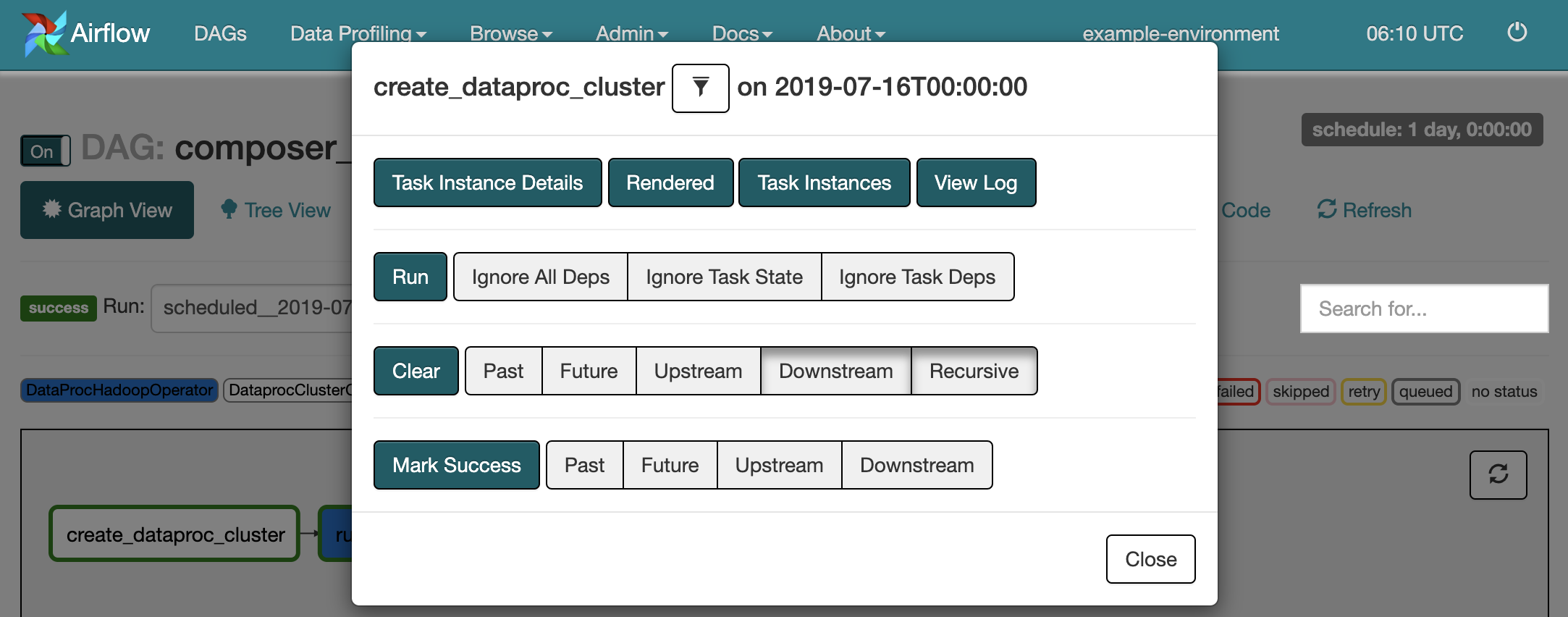
태스크 결과 보기
다음 Google Cloud 콘솔 페이지로 이동하여 composer_hadoop_tutorial
워크플로의 상태와 결과를 확인할 수도 있습니다.
Dataproc 클러스터: 클러스터 생성 및 삭제를 모니터링합니다. 워크플로에서 만든 클러스터는 수명이 짧습니다. 즉, 워크플로 지속시간 동안에만 존재하며 마지막 워크플로 태스크의 일부로 삭제됩니다.
Dataproc 작업: Apache Hadoop 워드카운트 작업을 보거나 모니터링합니다. 작업 ID를 클릭하여 작업 로그 출력을 확인합니다.
Cloud Storage 브라우저: 이 튜토리얼에서 만든 Cloud Storage 버킷의
wordcount
폴더에서 워드카운트 결과를 확인합니다.
삭제
이 튜토리얼에서 사용된 리소스를 삭제합니다.
환경 버킷을 수동으로 삭제하는 등 Cloud Composer 환경을 삭제합니다.
Hadoop 워드카운트 작업 결과를 저장하는 Cloud Storage 버킷을 삭제합니다.