Cloud Composer 1 | Cloud Composer 2
Questa pagina descrive come utilizzare gli operatori di Google Kubernetes Engine per creare cluster in Google Kubernetes Engine e avviare i pod Kubernetes in questi cluster.
Gli operatori di Google Kubernetes Engine eseguono i pod Kubernetes in un cluster specificato, che può essere un cluster separato non correlato al tuo ambiente.
In confronto, KubernetesPodOperator
esegue i pod Kubernetes
nel cluster del tuo ambiente.
Questa pagina illustra un esempio di DAG che crea un cluster Google Kubernetes Engine con GKECreateClusterOperator
, utilizza GKEStartPodOperator
con le seguenti configurazioni, quindi lo elimina con GKEDeleteClusterOperator
in seguito:
- Configurazione minima: imposta solo i parametri richiesti.
- Configurazione modello: utilizza i parametri che puoi modellare con Jinja.
- Configurazione di affinità pod: limita i nodi disponibili su cui pianificare i pod.
- Configurazione completa: include tutte le configurazioni.
Prima di iniziare
Ti consigliamo di utilizzare l'ultima versione di Cloud Composer. Come minima, questa versione deve essere supportata nell'ambito dei criteri relativi a ritiro e assistenza.
Configurazione degli operatori GKE
Per continuare con questo esempio, inserisci l'intero file gke_operator.py
nella cartella dags/
del tuo ambiente o aggiungi il codice pertinente a un DAG.
Crea un cluster
Il codice mostrato qui crea un cluster Google Kubernetes Engine con due pool di nodi, pool-0
e pool-1
, ciascuno dei quali ha un nodo. Se necessario, puoi impostare altri parametri dall'API Google Kubernetes Engine come parte dell'body
.
Prima del rilascio della versione 5.1.0 di apache-airflow-providers-google
, non era possibile passare l'oggetto node_pools
in GKECreateClusterOperator
. Se utilizzi Airflow 2, assicurati che il tuo ambiente utilizzi apache-airflow-providers-google
versione 5.1.0 o successiva. Puoi
installare una versione più recente di questo pacchetto PyPI
specificando apache-airflow-providers-google
e >=5.1.0
come
versione richiesta.
Come soluzione alternativa per gli utenti di Airflow 1, utilizziamo BashOperator
e gcloud
per creare questi pool di nodi.
Airflow 2
# TODO(developer): update with your values
PROJECT_ID = "my-project-id"
# It is recommended to use regional clusters for increased reliability
# though passing a zone in the location parameter is also valid
CLUSTER_REGION = "us-west1"
CLUSTER_NAME = "example-cluster"
CLUSTER = {
"name": CLUSTER_NAME,
"node_pools": [
{"name": "pool-0", "initial_node_count": 1},
{"name": "pool-1", "initial_node_count": 1},
],
}
create_cluster = GKECreateClusterOperator(
task_id="create_cluster",
project_id=PROJECT_ID,
location=CLUSTER_REGION,
body=CLUSTER,
)
Airflow 1
# TODO(developer): update with your values
PROJECT_ID = "my-project-id"
CLUSTER_ZONE = "us-west1-a"
CLUSTER_NAME = "example-cluster"
CLUSTER = {"name": CLUSTER_NAME, "initial_node_count": 1}
create_cluster = GKECreateClusterOperator(
task_id="create_cluster",
project_id=PROJECT_ID,
location=CLUSTER_ZONE,
body=CLUSTER,
)
# Using the BashOperator to create node pools is a workaround
# In Airflow 2, because of https://github.com/apache/airflow/pull/17820
# Node pool creation can be done using the GKECreateClusterOperator
create_node_pools = BashOperator(
task_id="create_node_pools",
bash_command=f"gcloud container node-pools create pool-0 \
--cluster {CLUSTER_NAME} \
--num-nodes 1 \
--zone {CLUSTER_ZONE} \
&& gcloud container node-pools create pool-1 \
--cluster {CLUSTER_NAME} \
--num-nodes 1 \
--zone {CLUSTER_ZONE}",
)
Avvia carichi di lavoro nel cluster
Le sezioni seguenti spiegano ogni configurazione GKEStartPodOperator
nell'esempio. Per informazioni su ogni variabile di configurazione, consulta
il riferimento Airflow per gli operatori GKE.
Airflow 2
from airflow import models
from airflow.providers.google.cloud.operators.kubernetes_engine import (
GKECreateClusterOperator,
GKEDeleteClusterOperator,
GKEStartPodOperator,
)
from airflow.utils.dates import days_ago
from kubernetes.client import models as k8s_models
with models.DAG(
"example_gcp_gke",
schedule_interval=None, # Override to match your needs
start_date=days_ago(1),
tags=["example"],
) as dag:
# TODO(developer): update with your values
PROJECT_ID = "my-project-id"
# It is recommended to use regional clusters for increased reliability
# though passing a zone in the location parameter is also valid
CLUSTER_REGION = "us-west1"
CLUSTER_NAME = "example-cluster"
CLUSTER = {
"name": CLUSTER_NAME,
"node_pools": [
{"name": "pool-0", "initial_node_count": 1},
{"name": "pool-1", "initial_node_count": 1},
],
}
create_cluster = GKECreateClusterOperator(
task_id="create_cluster",
project_id=PROJECT_ID,
location=CLUSTER_REGION,
body=CLUSTER,
)
kubernetes_min_pod = GKEStartPodOperator(
# The ID specified for the task.
task_id="pod-ex-minimum",
# Name of task you want to run, used to generate Pod ID.
name="pod-ex-minimum",
project_id=PROJECT_ID,
location=CLUSTER_REGION,
cluster_name=CLUSTER_NAME,
# Entrypoint of the container, if not specified the Docker container's
# entrypoint is used. The cmds parameter is templated.
cmds=["echo"],
# The namespace to run within Kubernetes, default namespace is
# `default`.
namespace="default",
# Docker image specified. Defaults to hub.docker.com, but any fully
# qualified URLs will point to a custom repository. Supports private
# gcr.io images if the Composer Environment is under the same
# project-id as the gcr.io images and the service account that Composer
# uses has permission to access the Google Container Registry
# (the default service account has permission)
image="gcr.io/gcp-runtimes/ubuntu_18_0_4",
)
kubenetes_template_ex = GKEStartPodOperator(
task_id="ex-kube-templates",
name="ex-kube-templates",
project_id=PROJECT_ID,
location=CLUSTER_REGION,
cluster_name=CLUSTER_NAME,
namespace="default",
image="bash",
# All parameters below are able to be templated with jinja -- cmds,
# arguments, env_vars, and config_file. For more information visit:
# https://airflow.apache.org/docs/apache-airflow/stable/macros-ref.html
# Entrypoint of the container, if not specified the Docker container's
# entrypoint is used. The cmds parameter is templated.
cmds=["echo"],
# DS in jinja is the execution date as YYYY-MM-DD, this docker image
# will echo the execution date. Arguments to the entrypoint. The docker
# image's CMD is used if this is not provided. The arguments parameter
# is templated.
arguments=["{{ ds }}"],
# The var template variable allows you to access variables defined in
# Airflow UI. In this case we are getting the value of my_value and
# setting the environment variable `MY_VALUE`. The pod will fail if
# `my_value` is not set in the Airflow UI.
env_vars={"MY_VALUE": "{{ var.value.my_value }}"},
)
kubernetes_affinity_ex = GKEStartPodOperator(
task_id="ex-pod-affinity",
project_id=PROJECT_ID,
location=CLUSTER_REGION,
cluster_name=CLUSTER_NAME,
name="ex-pod-affinity",
namespace="default",
image="perl",
cmds=["perl"],
arguments=["-Mbignum=bpi", "-wle", "print bpi(2000)"],
# affinity allows you to constrain which nodes your pod is eligible to
# be scheduled on, based on labels on the node. In this case, if the
# label 'cloud.google.com/gke-nodepool' with value
# 'nodepool-label-value' or 'nodepool-label-value2' is not found on any
# nodes, it will fail to schedule.
affinity={
"nodeAffinity": {
# requiredDuringSchedulingIgnoredDuringExecution means in order
# for a pod to be scheduled on a node, the node must have the
# specified labels. However, if labels on a node change at
# runtime such that the affinity rules on a pod are no longer
# met, the pod will still continue to run on the node.
"requiredDuringSchedulingIgnoredDuringExecution": {
"nodeSelectorTerms": [
{
"matchExpressions": [
{
# When nodepools are created in Google Kubernetes
# Engine, the nodes inside of that nodepool are
# automatically assigned the label
# 'cloud.google.com/gke-nodepool' with the value of
# the nodepool's name.
"key": "cloud.google.com/gke-nodepool",
"operator": "In",
# The label key's value that pods can be scheduled
# on.
"values": [
"pool-1",
],
}
]
}
]
}
}
},
)
kubernetes_full_pod = GKEStartPodOperator(
task_id="ex-all-configs",
name="full",
project_id=PROJECT_ID,
location=CLUSTER_REGION,
cluster_name=CLUSTER_NAME,
namespace="default",
image="perl:5.34.0",
# Entrypoint of the container, if not specified the Docker container's
# entrypoint is used. The cmds parameter is templated.
cmds=["perl"],
# Arguments to the entrypoint. The docker image's CMD is used if this
# is not provided. The arguments parameter is templated.
arguments=["-Mbignum=bpi", "-wle", "print bpi(2000)"],
# The secrets to pass to Pod, the Pod will fail to create if the
# secrets you specify in a Secret object do not exist in Kubernetes.
secrets=[],
# Labels to apply to the Pod.
labels={"pod-label": "label-name"},
# Timeout to start up the Pod, default is 120.
startup_timeout_seconds=120,
# The environment variables to be initialized in the container
# env_vars are templated.
env_vars={"EXAMPLE_VAR": "/example/value"},
# If true, logs stdout output of container. Defaults to True.
get_logs=True,
# Determines when to pull a fresh image, if 'IfNotPresent' will cause
# the Kubelet to skip pulling an image if it already exists. If you
# want to always pull a new image, set it to 'Always'.
image_pull_policy="Always",
# Annotations are non-identifying metadata you can attach to the Pod.
# Can be a large range of data, and can include characters that are not
# permitted by labels.
annotations={"key1": "value1"},
# Optional resource specifications for Pod, this will allow you to
# set both cpu and memory limits and requirements.
# Prior to Airflow 2.3 and the cncf providers package 5.0.0
# resources were passed as a dictionary. This change was made in
# https://github.com/apache/airflow/pull/27197
# Additionally, "memory" and "cpu" were previously named
# "limit_memory" and "limit_cpu"
# resources={'limit_memory': "250M", 'limit_cpu': "100m"},
container_resources=k8s_models.V1ResourceRequirements(
limits={"memory": "250M", "cpu": "100m"},
),
# If true, the content of /airflow/xcom/return.json from container will
# also be pushed to an XCom when the container ends.
do_xcom_push=False,
# List of Volume objects to pass to the Pod.
volumes=[],
# List of VolumeMount objects to pass to the Pod.
volume_mounts=[],
# Affinity determines which nodes the Pod can run on based on the
# config. For more information see:
# https://kubernetes.io/docs/concepts/configuration/assign-pod-node/
affinity={},
)
delete_cluster = GKEDeleteClusterOperator(
task_id="delete_cluster",
name=CLUSTER_NAME,
project_id=PROJECT_ID,
location=CLUSTER_REGION,
)
create_cluster >> kubernetes_min_pod >> delete_cluster
create_cluster >> kubernetes_full_pod >> delete_cluster
create_cluster >> kubernetes_affinity_ex >> delete_cluster
create_cluster >> kubenetes_template_ex >> delete_cluster
Airflow 1
from airflow import models
from airflow.operators.bash_operator import BashOperator
from airflow.providers.google.cloud.operators.kubernetes_engine import (
GKECreateClusterOperator,
GKEDeleteClusterOperator,
GKEStartPodOperator,
)
from airflow.utils.dates import days_ago
with models.DAG(
"example_gcp_gke",
schedule_interval=None, # Override to match your needs
start_date=days_ago(1),
tags=["example"],
) as dag:
# TODO(developer): update with your values
PROJECT_ID = "my-project-id"
CLUSTER_ZONE = "us-west1-a"
CLUSTER_NAME = "example-cluster"
CLUSTER = {"name": CLUSTER_NAME, "initial_node_count": 1}
create_cluster = GKECreateClusterOperator(
task_id="create_cluster",
project_id=PROJECT_ID,
location=CLUSTER_ZONE,
body=CLUSTER,
)
# Using the BashOperator to create node pools is a workaround
# In Airflow 2, because of https://github.com/apache/airflow/pull/17820
# Node pool creation can be done using the GKECreateClusterOperator
create_node_pools = BashOperator(
task_id="create_node_pools",
bash_command=f"gcloud container node-pools create pool-0 \
--cluster {CLUSTER_NAME} \
--num-nodes 1 \
--zone {CLUSTER_ZONE} \
&& gcloud container node-pools create pool-1 \
--cluster {CLUSTER_NAME} \
--num-nodes 1 \
--zone {CLUSTER_ZONE}",
)
kubernetes_min_pod = GKEStartPodOperator(
# The ID specified for the task.
task_id="pod-ex-minimum",
# Name of task you want to run, used to generate Pod ID.
name="pod-ex-minimum",
project_id=PROJECT_ID,
location=CLUSTER_ZONE,
cluster_name=CLUSTER_NAME,
# Entrypoint of the container, if not specified the Docker container's
# entrypoint is used. The cmds parameter is templated.
cmds=["echo"],
# The namespace to run within Kubernetes, default namespace is
# `default`.
namespace="default",
# Docker image specified. Defaults to hub.docker.com, but any fully
# qualified URLs will point to a custom repository. Supports private
# gcr.io images if the Composer Environment is under the same
# project-id as the gcr.io images and the service account that Composer
# uses has permission to access the Google Container Registry
# (the default service account has permission)
image="gcr.io/gcp-runtimes/ubuntu_18_0_4",
)
kubenetes_template_ex = GKEStartPodOperator(
task_id="ex-kube-templates",
name="ex-kube-templates",
project_id=PROJECT_ID,
location=CLUSTER_ZONE,
cluster_name=CLUSTER_NAME,
namespace="default",
image="bash",
# All parameters below are able to be templated with jinja -- cmds,
# arguments, env_vars, and config_file. For more information visit:
# https://airflow.apache.org/docs/apache-airflow/stable/macros-ref.html
# Entrypoint of the container, if not specified the Docker container's
# entrypoint is used. The cmds parameter is templated.
cmds=["echo"],
# DS in jinja is the execution date as YYYY-MM-DD, this docker image
# will echo the execution date. Arguments to the entrypoint. The docker
# image's CMD is used if this is not provided. The arguments parameter
# is templated.
arguments=["{{ ds }}"],
# The var template variable allows you to access variables defined in
# Airflow UI. In this case we are getting the value of my_value and
# setting the environment variable `MY_VALUE`. The pod will fail if
# `my_value` is not set in the Airflow UI.
env_vars={"MY_VALUE": "{{ var.value.my_value }}"},
)
kubernetes_affinity_ex = GKEStartPodOperator(
task_id="ex-pod-affinity",
project_id=PROJECT_ID,
location=CLUSTER_ZONE,
cluster_name=CLUSTER_NAME,
name="ex-pod-affinity",
namespace="default",
image="perl",
cmds=["perl"],
arguments=["-Mbignum=bpi", "-wle", "print bpi(2000)"],
# affinity allows you to constrain which nodes your pod is eligible to
# be scheduled on, based on labels on the node. In this case, if the
# label 'cloud.google.com/gke-nodepool' with value
# 'nodepool-label-value' or 'nodepool-label-value2' is not found on any
# nodes, it will fail to schedule.
affinity={
"nodeAffinity": {
# requiredDuringSchedulingIgnoredDuringExecution means in order
# for a pod to be scheduled on a node, the node must have the
# specified labels. However, if labels on a node change at
# runtime such that the affinity rules on a pod are no longer
# met, the pod will still continue to run on the node.
"requiredDuringSchedulingIgnoredDuringExecution": {
"nodeSelectorTerms": [
{
"matchExpressions": [
{
# When nodepools are created in Google Kubernetes
# Engine, the nodes inside of that nodepool are
# automatically assigned the label
# 'cloud.google.com/gke-nodepool' with the value of
# the nodepool's name.
"key": "cloud.google.com/gke-nodepool",
"operator": "In",
# The label key's value that pods can be scheduled
# on.
"values": [
"pool-1",
],
}
]
}
]
}
}
},
)
kubernetes_full_pod = GKEStartPodOperator(
task_id="ex-all-configs",
name="full",
project_id=PROJECT_ID,
location=CLUSTER_ZONE,
cluster_name=CLUSTER_NAME,
namespace="default",
image="perl",
# Entrypoint of the container, if not specified the Docker container's
# entrypoint is used. The cmds parameter is templated.
cmds=["perl"],
# Arguments to the entrypoint. The docker image's CMD is used if this
# is not provided. The arguments parameter is templated.
arguments=["-Mbignum=bpi", "-wle", "print bpi(2000)"],
# The secrets to pass to Pod, the Pod will fail to create if the
# secrets you specify in a Secret object do not exist in Kubernetes.
secrets=[],
# Labels to apply to the Pod.
labels={"pod-label": "label-name"},
# Timeout to start up the Pod, default is 120.
startup_timeout_seconds=120,
# The environment variables to be initialized in the container
# env_vars are templated.
env_vars={"EXAMPLE_VAR": "/example/value"},
# If true, logs stdout output of container. Defaults to True.
get_logs=True,
# Determines when to pull a fresh image, if 'IfNotPresent' will cause
# the Kubelet to skip pulling an image if it already exists. If you
# want to always pull a new image, set it to 'Always'.
image_pull_policy="Always",
# Annotations are non-identifying metadata you can attach to the Pod.
# Can be a large range of data, and can include characters that are not
# permitted by labels.
annotations={"key1": "value1"},
# Resource specifications for Pod, this will allow you to set both cpu
# and memory limits and requirements.
# Prior to Airflow 1.10.4, resource specifications were
# passed as a Pod Resources Class object,
# If using this example on a version of Airflow prior to 1.10.4,
# import the "pod" package from airflow.contrib.kubernetes and use
# resources = pod.Resources() instead passing a dict
# For more info see:
# https://github.com/apache/airflow/pull/4551
resources={"limit_memory": "250M", "limit_cpu": "100m"},
# If true, the content of /airflow/xcom/return.json from container will
# also be pushed to an XCom when the container ends.
do_xcom_push=False,
# List of Volume objects to pass to the Pod.
volumes=[],
# List of VolumeMount objects to pass to the Pod.
volume_mounts=[],
# Affinity determines which nodes the Pod can run on based on the
# config. For more information see:
# https://kubernetes.io/docs/concepts/configuration/assign-pod-node/
affinity={},
)
delete_cluster = GKEDeleteClusterOperator(
task_id="delete_cluster",
name=CLUSTER_NAME,
project_id=PROJECT_ID,
location=CLUSTER_ZONE,
)
create_cluster >> create_node_pools >> kubernetes_min_pod >> delete_cluster
create_cluster >> create_node_pools >> kubernetes_full_pod >> delete_cluster
create_cluster >> create_node_pools >> kubernetes_affinity_ex >> delete_cluster
create_cluster >> create_node_pools >> kubenetes_template_ex >> delete_cluster
Configurazione minima
Per avviare un pod nel tuo cluster GKE con GKEStartPodOperator
, sono necessarie solo le opzioni project_id
, location
, cluster_name
, name
, namespace
, image
e task_id
.
Quando inserisci il seguente snippet di codice in un DAG, l'attività pod-ex-minimum
ha esito positivo a condizione che i parametri elencati in precedenza siano definiti e validi.
Airflow 2
# TODO(developer): update with your values
PROJECT_ID = "my-project-id"
# It is recommended to use regional clusters for increased reliability
# though passing a zone in the location parameter is also valid
CLUSTER_REGION = "us-west1"
CLUSTER_NAME = "example-cluster"
kubernetes_min_pod = GKEStartPodOperator(
# The ID specified for the task.
task_id="pod-ex-minimum",
# Name of task you want to run, used to generate Pod ID.
name="pod-ex-minimum",
project_id=PROJECT_ID,
location=CLUSTER_REGION,
cluster_name=CLUSTER_NAME,
# Entrypoint of the container, if not specified the Docker container's
# entrypoint is used. The cmds parameter is templated.
cmds=["echo"],
# The namespace to run within Kubernetes, default namespace is
# `default`.
namespace="default",
# Docker image specified. Defaults to hub.docker.com, but any fully
# qualified URLs will point to a custom repository. Supports private
# gcr.io images if the Composer Environment is under the same
# project-id as the gcr.io images and the service account that Composer
# uses has permission to access the Google Container Registry
# (the default service account has permission)
image="gcr.io/gcp-runtimes/ubuntu_18_0_4",
)
Airflow 1
# TODO(developer): update with your values
PROJECT_ID = "my-project-id"
CLUSTER_ZONE = "us-west1-a"
CLUSTER_NAME = "example-cluster"
kubernetes_min_pod = GKEStartPodOperator(
# The ID specified for the task.
task_id="pod-ex-minimum",
# Name of task you want to run, used to generate Pod ID.
name="pod-ex-minimum",
project_id=PROJECT_ID,
location=CLUSTER_ZONE,
cluster_name=CLUSTER_NAME,
# Entrypoint of the container, if not specified the Docker container's
# entrypoint is used. The cmds parameter is templated.
cmds=["echo"],
# The namespace to run within Kubernetes, default namespace is
# `default`.
namespace="default",
# Docker image specified. Defaults to hub.docker.com, but any fully
# qualified URLs will point to a custom repository. Supports private
# gcr.io images if the Composer Environment is under the same
# project-id as the gcr.io images and the service account that Composer
# uses has permission to access the Google Container Registry
# (the default service account has permission)
image="gcr.io/gcp-runtimes/ubuntu_18_0_4",
)
Configurazione modello
Airflow supporta l'utilizzo di Jinja Templating.
Devi dichiarare le variabili richieste (task_id
, name
, namespace
e image
) con l'operatore. Come mostrato nell'esempio seguente, puoi modello tutti gli altri parametri con Jinja, tra cui cmds
, arguments
e env_vars
.
Se non modifichi il DAG o l'ambiente, l'attività ex-kube-templates
non va a buon fine. Imposta una variabile Airflow denominata my_value
per consentire l'esito positivo di questo DAG.
Per impostare my_value
con gcloud
o la UI di Airflow:
gcloud
Per Airflow 2, inserisci il seguente comando:
gcloud composer environments run ENVIRONMENT \
--location LOCATION \
variables set -- \
my_value example_value
Per Airflow 1, inserisci il seguente comando:
gcloud composer environments run ENVIRONMENT \
--location LOCATION \
variables -- \
--set my_value example_value
Sostituisci:
ENVIRONMENT
con il nome dell'ambiente.LOCATION
con la regione in cui si trova l'ambiente.
UI di Airflow
Nell'interfaccia utente di Airflow 2:
Nella barra degli strumenti, seleziona Amministrazione > Variabili.
Nella pagina Variabile elenco, fai clic su Aggiungi un nuovo record.
Nella pagina Aggiungi variabile, inserisci le seguenti informazioni:
- Chiave:
my_value
- Valore:
example_value
- Chiave:
Fai clic su Salva.
Nell'interfaccia utente di Airflow 1:
Nella barra degli strumenti, seleziona Amministrazione > Variabili.
Nella pagina Variabili, fai clic sulla scheda Crea.
Nella pagina Variabile, inserisci le seguenti informazioni:
- Chiave:
my_value
- Valore:
example_value
- Chiave:
Fai clic su Salva.
Configurazione modello:
Airflow 2
# TODO(developer): update with your values
PROJECT_ID = "my-project-id"
# It is recommended to use regional clusters for increased reliability
# though passing a zone in the location parameter is also valid
CLUSTER_REGION = "us-west1"
CLUSTER_NAME = "example-cluster"
kubenetes_template_ex = GKEStartPodOperator(
task_id="ex-kube-templates",
name="ex-kube-templates",
project_id=PROJECT_ID,
location=CLUSTER_REGION,
cluster_name=CLUSTER_NAME,
namespace="default",
image="bash",
# All parameters below are able to be templated with jinja -- cmds,
# arguments, env_vars, and config_file. For more information visit:
# https://airflow.apache.org/docs/apache-airflow/stable/macros-ref.html
# Entrypoint of the container, if not specified the Docker container's
# entrypoint is used. The cmds parameter is templated.
cmds=["echo"],
# DS in jinja is the execution date as YYYY-MM-DD, this docker image
# will echo the execution date. Arguments to the entrypoint. The docker
# image's CMD is used if this is not provided. The arguments parameter
# is templated.
arguments=["{{ ds }}"],
# The var template variable allows you to access variables defined in
# Airflow UI. In this case we are getting the value of my_value and
# setting the environment variable `MY_VALUE`. The pod will fail if
# `my_value` is not set in the Airflow UI.
env_vars={"MY_VALUE": "{{ var.value.my_value }}"},
)
Airflow 1
# TODO(developer): update with your values
PROJECT_ID = "my-project-id"
CLUSTER_ZONE = "us-west1-a"
CLUSTER_NAME = "example-cluster"
kubenetes_template_ex = GKEStartPodOperator(
task_id="ex-kube-templates",
name="ex-kube-templates",
project_id=PROJECT_ID,
location=CLUSTER_ZONE,
cluster_name=CLUSTER_NAME,
namespace="default",
image="bash",
# All parameters below are able to be templated with jinja -- cmds,
# arguments, env_vars, and config_file. For more information visit:
# https://airflow.apache.org/docs/apache-airflow/stable/macros-ref.html
# Entrypoint of the container, if not specified the Docker container's
# entrypoint is used. The cmds parameter is templated.
cmds=["echo"],
# DS in jinja is the execution date as YYYY-MM-DD, this docker image
# will echo the execution date. Arguments to the entrypoint. The docker
# image's CMD is used if this is not provided. The arguments parameter
# is templated.
arguments=["{{ ds }}"],
# The var template variable allows you to access variables defined in
# Airflow UI. In this case we are getting the value of my_value and
# setting the environment variable `MY_VALUE`. The pod will fail if
# `my_value` is not set in the Airflow UI.
env_vars={"MY_VALUE": "{{ var.value.my_value }}"},
)
Configurazione affinità pod
Quando configuri il parametro affinity
in GKEStartPodOperator
, puoi controllare su quali nodi pianificare i pod, ad esempio i nodi solo in un determinato pool di nodi. Quando hai creato il cluster, hai creato due pool di nodi denominati
pool-0
e pool-1
. Questo operatore indica che i pod devono essere eseguiti solo in
pool-1
.
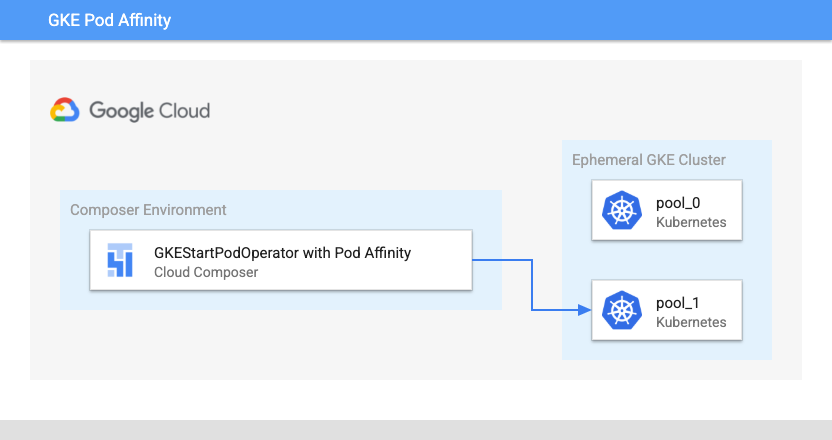
Airflow 2
# TODO(developer): update with your values
PROJECT_ID = "my-project-id"
# It is recommended to use regional clusters for increased reliability
# though passing a zone in the location parameter is also valid
CLUSTER_REGION = "us-west1"
CLUSTER_NAME = "example-cluster"
kubernetes_affinity_ex = GKEStartPodOperator(
task_id="ex-pod-affinity",
project_id=PROJECT_ID,
location=CLUSTER_REGION,
cluster_name=CLUSTER_NAME,
name="ex-pod-affinity",
namespace="default",
image="perl",
cmds=["perl"],
arguments=["-Mbignum=bpi", "-wle", "print bpi(2000)"],
# affinity allows you to constrain which nodes your pod is eligible to
# be scheduled on, based on labels on the node. In this case, if the
# label 'cloud.google.com/gke-nodepool' with value
# 'nodepool-label-value' or 'nodepool-label-value2' is not found on any
# nodes, it will fail to schedule.
affinity={
"nodeAffinity": {
# requiredDuringSchedulingIgnoredDuringExecution means in order
# for a pod to be scheduled on a node, the node must have the
# specified labels. However, if labels on a node change at
# runtime such that the affinity rules on a pod are no longer
# met, the pod will still continue to run on the node.
"requiredDuringSchedulingIgnoredDuringExecution": {
"nodeSelectorTerms": [
{
"matchExpressions": [
{
# When nodepools are created in Google Kubernetes
# Engine, the nodes inside of that nodepool are
# automatically assigned the label
# 'cloud.google.com/gke-nodepool' with the value of
# the nodepool's name.
"key": "cloud.google.com/gke-nodepool",
"operator": "In",
# The label key's value that pods can be scheduled
# on.
"values": [
"pool-1",
],
}
]
}
]
}
}
},
)
Airflow 1
# TODO(developer): update with your values
PROJECT_ID = "my-project-id"
CLUSTER_ZONE = "us-west1-a"
CLUSTER_NAME = "example-cluster"
kubernetes_affinity_ex = GKEStartPodOperator(
task_id="ex-pod-affinity",
project_id=PROJECT_ID,
location=CLUSTER_ZONE,
cluster_name=CLUSTER_NAME,
name="ex-pod-affinity",
namespace="default",
image="perl",
cmds=["perl"],
arguments=["-Mbignum=bpi", "-wle", "print bpi(2000)"],
# affinity allows you to constrain which nodes your pod is eligible to
# be scheduled on, based on labels on the node. In this case, if the
# label 'cloud.google.com/gke-nodepool' with value
# 'nodepool-label-value' or 'nodepool-label-value2' is not found on any
# nodes, it will fail to schedule.
affinity={
"nodeAffinity": {
# requiredDuringSchedulingIgnoredDuringExecution means in order
# for a pod to be scheduled on a node, the node must have the
# specified labels. However, if labels on a node change at
# runtime such that the affinity rules on a pod are no longer
# met, the pod will still continue to run on the node.
"requiredDuringSchedulingIgnoredDuringExecution": {
"nodeSelectorTerms": [
{
"matchExpressions": [
{
# When nodepools are created in Google Kubernetes
# Engine, the nodes inside of that nodepool are
# automatically assigned the label
# 'cloud.google.com/gke-nodepool' with the value of
# the nodepool's name.
"key": "cloud.google.com/gke-nodepool",
"operator": "In",
# The label key's value that pods can be scheduled
# on.
"values": [
"pool-1",
],
}
]
}
]
}
}
},
)
Configurazione completa
Questo esempio mostra tutte le variabili che puoi configurare in GKEStartPodOperator
. Non è necessario modificare il codice per completare l'attività ex-all-configs
.
Per i dettagli su ogni variabile, consulta il riferimento Airflow per gli operatori GKE.
Airflow 2
# TODO(developer): update with your values
PROJECT_ID = "my-project-id"
# It is recommended to use regional clusters for increased reliability
# though passing a zone in the location parameter is also valid
CLUSTER_REGION = "us-west1"
CLUSTER_NAME = "example-cluster"
kubernetes_full_pod = GKEStartPodOperator(
task_id="ex-all-configs",
name="full",
project_id=PROJECT_ID,
location=CLUSTER_REGION,
cluster_name=CLUSTER_NAME,
namespace="default",
image="perl:5.34.0",
# Entrypoint of the container, if not specified the Docker container's
# entrypoint is used. The cmds parameter is templated.
cmds=["perl"],
# Arguments to the entrypoint. The docker image's CMD is used if this
# is not provided. The arguments parameter is templated.
arguments=["-Mbignum=bpi", "-wle", "print bpi(2000)"],
# The secrets to pass to Pod, the Pod will fail to create if the
# secrets you specify in a Secret object do not exist in Kubernetes.
secrets=[],
# Labels to apply to the Pod.
labels={"pod-label": "label-name"},
# Timeout to start up the Pod, default is 120.
startup_timeout_seconds=120,
# The environment variables to be initialized in the container
# env_vars are templated.
env_vars={"EXAMPLE_VAR": "/example/value"},
# If true, logs stdout output of container. Defaults to True.
get_logs=True,
# Determines when to pull a fresh image, if 'IfNotPresent' will cause
# the Kubelet to skip pulling an image if it already exists. If you
# want to always pull a new image, set it to 'Always'.
image_pull_policy="Always",
# Annotations are non-identifying metadata you can attach to the Pod.
# Can be a large range of data, and can include characters that are not
# permitted by labels.
annotations={"key1": "value1"},
# Optional resource specifications for Pod, this will allow you to
# set both cpu and memory limits and requirements.
# Prior to Airflow 2.3 and the cncf providers package 5.0.0
# resources were passed as a dictionary. This change was made in
# https://github.com/apache/airflow/pull/27197
# Additionally, "memory" and "cpu" were previously named
# "limit_memory" and "limit_cpu"
# resources={'limit_memory': "250M", 'limit_cpu': "100m"},
container_resources=k8s_models.V1ResourceRequirements(
limits={"memory": "250M", "cpu": "100m"},
),
# If true, the content of /airflow/xcom/return.json from container will
# also be pushed to an XCom when the container ends.
do_xcom_push=False,
# List of Volume objects to pass to the Pod.
volumes=[],
# List of VolumeMount objects to pass to the Pod.
volume_mounts=[],
# Affinity determines which nodes the Pod can run on based on the
# config. For more information see:
# https://kubernetes.io/docs/concepts/configuration/assign-pod-node/
affinity={},
)
Airflow 1
# TODO(developer): update with your values
PROJECT_ID = "my-project-id"
CLUSTER_ZONE = "us-west1-a"
CLUSTER_NAME = "example-cluster"
kubernetes_full_pod = GKEStartPodOperator(
task_id="ex-all-configs",
name="full",
project_id=PROJECT_ID,
location=CLUSTER_ZONE,
cluster_name=CLUSTER_NAME,
namespace="default",
image="perl",
# Entrypoint of the container, if not specified the Docker container's
# entrypoint is used. The cmds parameter is templated.
cmds=["perl"],
# Arguments to the entrypoint. The docker image's CMD is used if this
# is not provided. The arguments parameter is templated.
arguments=["-Mbignum=bpi", "-wle", "print bpi(2000)"],
# The secrets to pass to Pod, the Pod will fail to create if the
# secrets you specify in a Secret object do not exist in Kubernetes.
secrets=[],
# Labels to apply to the Pod.
labels={"pod-label": "label-name"},
# Timeout to start up the Pod, default is 120.
startup_timeout_seconds=120,
# The environment variables to be initialized in the container
# env_vars are templated.
env_vars={"EXAMPLE_VAR": "/example/value"},
# If true, logs stdout output of container. Defaults to True.
get_logs=True,
# Determines when to pull a fresh image, if 'IfNotPresent' will cause
# the Kubelet to skip pulling an image if it already exists. If you
# want to always pull a new image, set it to 'Always'.
image_pull_policy="Always",
# Annotations are non-identifying metadata you can attach to the Pod.
# Can be a large range of data, and can include characters that are not
# permitted by labels.
annotations={"key1": "value1"},
# Resource specifications for Pod, this will allow you to set both cpu
# and memory limits and requirements.
# Prior to Airflow 1.10.4, resource specifications were
# passed as a Pod Resources Class object,
# If using this example on a version of Airflow prior to 1.10.4,
# import the "pod" package from airflow.contrib.kubernetes and use
# resources = pod.Resources() instead passing a dict
# For more info see:
# https://github.com/apache/airflow/pull/4551
resources={"limit_memory": "250M", "limit_cpu": "100m"},
# If true, the content of /airflow/xcom/return.json from container will
# also be pushed to an XCom when the container ends.
do_xcom_push=False,
# List of Volume objects to pass to the Pod.
volumes=[],
# List of VolumeMount objects to pass to the Pod.
volume_mounts=[],
# Affinity determines which nodes the Pod can run on based on the
# config. For more information see:
# https://kubernetes.io/docs/concepts/configuration/assign-pod-node/
affinity={},
)
Eliminare il cluster
Il codice mostrato qui elimina il cluster creato all'inizio della guida.
Airflow 2
delete_cluster = GKEDeleteClusterOperator(
task_id="delete_cluster",
name=CLUSTER_NAME,
project_id=PROJECT_ID,
location=CLUSTER_REGION,
)
Airflow 1
delete_cluster = GKEDeleteClusterOperator(
task_id="delete_cluster",
name=CLUSTER_NAME,
project_id=PROJECT_ID,
location=CLUSTER_ZONE,
)