Cloud Composer 1 | Cloud Composer 2
En esta página, se describe cómo usar los operadores de Google Kubernetes Engine para crear clústeres en Google Kubernetes Engine y cómo iniciar Pods de Kubernetes en esos clústeres.
Los operadores de Google Kubernetes Engine ejecutan pods de Kubernetes en un clúster específico, que puede ser un clúster separado que no está relacionado con tu entorno.
En comparación, KubernetesPodOperator
ejecuta pods de Kubernetes en el clúster de tu entorno.
En esta página, se muestra un ejemplo de DAG que crea un clúster de Google Kubernetes Engine con GKECreateClusterOperator
, usa GKEStartPodOperator
con las siguientes configuraciones y, luego, lo borra con GKEDeleteClusterOperator
:
- Configuración mínima: establece solo los parámetros requeridos.
- Configuración de plantilla: utiliza parámetros que puedes agregar a una plantilla con Jinja.
- Configuración de afinidad de pods: Limita los nodos disponibles para programar pods.
- Configuración completa: incluye todas las configuraciones.
Antes de comenzar
Te recomendamos usar la versión más reciente de Cloud Composer. Como mínimo, esta versión debe ser compatible como parte de la política de baja y asistencia.
Configuración del operador de GKE
Para continuar con este ejemplo, coloca todo el archivo gke_operator.py
en la carpeta dags/
de tu entorno o agrega el código relevante a un DAG.
Crea un clúster
Mediante el código que se muestra aquí, se crea un clúster de Google Kubernetes Engine con dos grupos de nodos, pool-0
y pool-1
, cada uno de los cuales tiene un nodo. Si es necesario, puedes configurar otros parámetros de la API de Google Kubernetes Engine como parte de body
.
Antes del lanzamiento de la versión 5.1.0 de apache-airflow-providers-google
, no era posible pasar el objeto node_pools
en GKECreateClusterOperator
. Si usas Airflow 2, asegúrate de que tu entorno use la versión 5.1.0 o posterior de apache-airflow-providers-google
. Puedes instalar una versión más reciente de este paquete de PyPI si especificas apache-airflow-providers-google
y >=5.1.0
como las versiones requeridas.
Como solución alternativa para los usuarios de Airflow 1, usamos BashOperator
y gcloud
para crear estos grupos de nodos.
Airflow 2
# TODO(developer): update with your values
PROJECT_ID = "my-project-id"
# It is recommended to use regional clusters for increased reliability
# though passing a zone in the location parameter is also valid
CLUSTER_REGION = "us-west1"
CLUSTER_NAME = "example-cluster"
CLUSTER = {
"name": CLUSTER_NAME,
"node_pools": [
{"name": "pool-0", "initial_node_count": 1},
{"name": "pool-1", "initial_node_count": 1},
],
}
create_cluster = GKECreateClusterOperator(
task_id="create_cluster",
project_id=PROJECT_ID,
location=CLUSTER_REGION,
body=CLUSTER,
)
Airflow 1
# TODO(developer): update with your values
PROJECT_ID = "my-project-id"
CLUSTER_ZONE = "us-west1-a"
CLUSTER_NAME = "example-cluster"
CLUSTER = {"name": CLUSTER_NAME, "initial_node_count": 1}
create_cluster = GKECreateClusterOperator(
task_id="create_cluster",
project_id=PROJECT_ID,
location=CLUSTER_ZONE,
body=CLUSTER,
)
# Using the BashOperator to create node pools is a workaround
# In Airflow 2, because of https://github.com/apache/airflow/pull/17820
# Node pool creation can be done using the GKECreateClusterOperator
create_node_pools = BashOperator(
task_id="create_node_pools",
bash_command=f"gcloud container node-pools create pool-0 \
--cluster {CLUSTER_NAME} \
--num-nodes 1 \
--zone {CLUSTER_ZONE} \
&& gcloud container node-pools create pool-1 \
--cluster {CLUSTER_NAME} \
--num-nodes 1 \
--zone {CLUSTER_ZONE}",
)
Inicia cargas de trabajo en el clúster
Las siguientes secciones explican cada configuración de GKEStartPodOperator
en el ejemplo. Para obtener información sobre cada variable de configuración, consulta la referencia de Airflow para los operadores de GKE.
Airflow 2
from airflow import models
from airflow.providers.google.cloud.operators.kubernetes_engine import (
GKECreateClusterOperator,
GKEDeleteClusterOperator,
GKEStartPodOperator,
)
from airflow.utils.dates import days_ago
from kubernetes.client import models as k8s_models
with models.DAG(
"example_gcp_gke",
schedule_interval=None, # Override to match your needs
start_date=days_ago(1),
tags=["example"],
) as dag:
# TODO(developer): update with your values
PROJECT_ID = "my-project-id"
# It is recommended to use regional clusters for increased reliability
# though passing a zone in the location parameter is also valid
CLUSTER_REGION = "us-west1"
CLUSTER_NAME = "example-cluster"
CLUSTER = {
"name": CLUSTER_NAME,
"node_pools": [
{"name": "pool-0", "initial_node_count": 1},
{"name": "pool-1", "initial_node_count": 1},
],
}
create_cluster = GKECreateClusterOperator(
task_id="create_cluster",
project_id=PROJECT_ID,
location=CLUSTER_REGION,
body=CLUSTER,
)
kubernetes_min_pod = GKEStartPodOperator(
# The ID specified for the task.
task_id="pod-ex-minimum",
# Name of task you want to run, used to generate Pod ID.
name="pod-ex-minimum",
project_id=PROJECT_ID,
location=CLUSTER_REGION,
cluster_name=CLUSTER_NAME,
# Entrypoint of the container, if not specified the Docker container's
# entrypoint is used. The cmds parameter is templated.
cmds=["echo"],
# The namespace to run within Kubernetes, default namespace is
# `default`.
namespace="default",
# Docker image specified. Defaults to hub.docker.com, but any fully
# qualified URLs will point to a custom repository. Supports private
# gcr.io images if the Composer Environment is under the same
# project-id as the gcr.io images and the service account that Composer
# uses has permission to access the Google Container Registry
# (the default service account has permission)
image="gcr.io/gcp-runtimes/ubuntu_18_0_4",
)
kubenetes_template_ex = GKEStartPodOperator(
task_id="ex-kube-templates",
name="ex-kube-templates",
project_id=PROJECT_ID,
location=CLUSTER_REGION,
cluster_name=CLUSTER_NAME,
namespace="default",
image="bash",
# All parameters below are able to be templated with jinja -- cmds,
# arguments, env_vars, and config_file. For more information visit:
# https://airflow.apache.org/docs/apache-airflow/stable/macros-ref.html
# Entrypoint of the container, if not specified the Docker container's
# entrypoint is used. The cmds parameter is templated.
cmds=["echo"],
# DS in jinja is the execution date as YYYY-MM-DD, this docker image
# will echo the execution date. Arguments to the entrypoint. The docker
# image's CMD is used if this is not provided. The arguments parameter
# is templated.
arguments=["{{ ds }}"],
# The var template variable allows you to access variables defined in
# Airflow UI. In this case we are getting the value of my_value and
# setting the environment variable `MY_VALUE`. The pod will fail if
# `my_value` is not set in the Airflow UI.
env_vars={"MY_VALUE": "{{ var.value.my_value }}"},
)
kubernetes_affinity_ex = GKEStartPodOperator(
task_id="ex-pod-affinity",
project_id=PROJECT_ID,
location=CLUSTER_REGION,
cluster_name=CLUSTER_NAME,
name="ex-pod-affinity",
namespace="default",
image="perl",
cmds=["perl"],
arguments=["-Mbignum=bpi", "-wle", "print bpi(2000)"],
# affinity allows you to constrain which nodes your pod is eligible to
# be scheduled on, based on labels on the node. In this case, if the
# label 'cloud.google.com/gke-nodepool' with value
# 'nodepool-label-value' or 'nodepool-label-value2' is not found on any
# nodes, it will fail to schedule.
affinity={
"nodeAffinity": {
# requiredDuringSchedulingIgnoredDuringExecution means in order
# for a pod to be scheduled on a node, the node must have the
# specified labels. However, if labels on a node change at
# runtime such that the affinity rules on a pod are no longer
# met, the pod will still continue to run on the node.
"requiredDuringSchedulingIgnoredDuringExecution": {
"nodeSelectorTerms": [
{
"matchExpressions": [
{
# When nodepools are created in Google Kubernetes
# Engine, the nodes inside of that nodepool are
# automatically assigned the label
# 'cloud.google.com/gke-nodepool' with the value of
# the nodepool's name.
"key": "cloud.google.com/gke-nodepool",
"operator": "In",
# The label key's value that pods can be scheduled
# on.
"values": [
"pool-1",
],
}
]
}
]
}
}
},
)
kubernetes_full_pod = GKEStartPodOperator(
task_id="ex-all-configs",
name="full",
project_id=PROJECT_ID,
location=CLUSTER_REGION,
cluster_name=CLUSTER_NAME,
namespace="default",
image="perl:5.34.0",
# Entrypoint of the container, if not specified the Docker container's
# entrypoint is used. The cmds parameter is templated.
cmds=["perl"],
# Arguments to the entrypoint. The docker image's CMD is used if this
# is not provided. The arguments parameter is templated.
arguments=["-Mbignum=bpi", "-wle", "print bpi(2000)"],
# The secrets to pass to Pod, the Pod will fail to create if the
# secrets you specify in a Secret object do not exist in Kubernetes.
secrets=[],
# Labels to apply to the Pod.
labels={"pod-label": "label-name"},
# Timeout to start up the Pod, default is 120.
startup_timeout_seconds=120,
# The environment variables to be initialized in the container
# env_vars are templated.
env_vars={"EXAMPLE_VAR": "/example/value"},
# If true, logs stdout output of container. Defaults to True.
get_logs=True,
# Determines when to pull a fresh image, if 'IfNotPresent' will cause
# the Kubelet to skip pulling an image if it already exists. If you
# want to always pull a new image, set it to 'Always'.
image_pull_policy="Always",
# Annotations are non-identifying metadata you can attach to the Pod.
# Can be a large range of data, and can include characters that are not
# permitted by labels.
annotations={"key1": "value1"},
# Optional resource specifications for Pod, this will allow you to
# set both cpu and memory limits and requirements.
# Prior to Airflow 2.3 and the cncf providers package 5.0.0
# resources were passed as a dictionary. This change was made in
# https://github.com/apache/airflow/pull/27197
# Additionally, "memory" and "cpu" were previously named
# "limit_memory" and "limit_cpu"
# resources={'limit_memory': "250M", 'limit_cpu': "100m"},
container_resources=k8s_models.V1ResourceRequirements(
limits={"memory": "250M", "cpu": "100m"},
),
# If true, the content of /airflow/xcom/return.json from container will
# also be pushed to an XCom when the container ends.
do_xcom_push=False,
# List of Volume objects to pass to the Pod.
volumes=[],
# List of VolumeMount objects to pass to the Pod.
volume_mounts=[],
# Affinity determines which nodes the Pod can run on based on the
# config. For more information see:
# https://kubernetes.io/docs/concepts/configuration/assign-pod-node/
affinity={},
)
delete_cluster = GKEDeleteClusterOperator(
task_id="delete_cluster",
name=CLUSTER_NAME,
project_id=PROJECT_ID,
location=CLUSTER_REGION,
)
create_cluster >> kubernetes_min_pod >> delete_cluster
create_cluster >> kubernetes_full_pod >> delete_cluster
create_cluster >> kubernetes_affinity_ex >> delete_cluster
create_cluster >> kubenetes_template_ex >> delete_cluster
Airflow 1
from airflow import models
from airflow.operators.bash_operator import BashOperator
from airflow.providers.google.cloud.operators.kubernetes_engine import (
GKECreateClusterOperator,
GKEDeleteClusterOperator,
GKEStartPodOperator,
)
from airflow.utils.dates import days_ago
with models.DAG(
"example_gcp_gke",
schedule_interval=None, # Override to match your needs
start_date=days_ago(1),
tags=["example"],
) as dag:
# TODO(developer): update with your values
PROJECT_ID = "my-project-id"
CLUSTER_ZONE = "us-west1-a"
CLUSTER_NAME = "example-cluster"
CLUSTER = {"name": CLUSTER_NAME, "initial_node_count": 1}
create_cluster = GKECreateClusterOperator(
task_id="create_cluster",
project_id=PROJECT_ID,
location=CLUSTER_ZONE,
body=CLUSTER,
)
# Using the BashOperator to create node pools is a workaround
# In Airflow 2, because of https://github.com/apache/airflow/pull/17820
# Node pool creation can be done using the GKECreateClusterOperator
create_node_pools = BashOperator(
task_id="create_node_pools",
bash_command=f"gcloud container node-pools create pool-0 \
--cluster {CLUSTER_NAME} \
--num-nodes 1 \
--zone {CLUSTER_ZONE} \
&& gcloud container node-pools create pool-1 \
--cluster {CLUSTER_NAME} \
--num-nodes 1 \
--zone {CLUSTER_ZONE}",
)
kubernetes_min_pod = GKEStartPodOperator(
# The ID specified for the task.
task_id="pod-ex-minimum",
# Name of task you want to run, used to generate Pod ID.
name="pod-ex-minimum",
project_id=PROJECT_ID,
location=CLUSTER_ZONE,
cluster_name=CLUSTER_NAME,
# Entrypoint of the container, if not specified the Docker container's
# entrypoint is used. The cmds parameter is templated.
cmds=["echo"],
# The namespace to run within Kubernetes, default namespace is
# `default`.
namespace="default",
# Docker image specified. Defaults to hub.docker.com, but any fully
# qualified URLs will point to a custom repository. Supports private
# gcr.io images if the Composer Environment is under the same
# project-id as the gcr.io images and the service account that Composer
# uses has permission to access the Google Container Registry
# (the default service account has permission)
image="gcr.io/gcp-runtimes/ubuntu_18_0_4",
)
kubenetes_template_ex = GKEStartPodOperator(
task_id="ex-kube-templates",
name="ex-kube-templates",
project_id=PROJECT_ID,
location=CLUSTER_ZONE,
cluster_name=CLUSTER_NAME,
namespace="default",
image="bash",
# All parameters below are able to be templated with jinja -- cmds,
# arguments, env_vars, and config_file. For more information visit:
# https://airflow.apache.org/docs/apache-airflow/stable/macros-ref.html
# Entrypoint of the container, if not specified the Docker container's
# entrypoint is used. The cmds parameter is templated.
cmds=["echo"],
# DS in jinja is the execution date as YYYY-MM-DD, this docker image
# will echo the execution date. Arguments to the entrypoint. The docker
# image's CMD is used if this is not provided. The arguments parameter
# is templated.
arguments=["{{ ds }}"],
# The var template variable allows you to access variables defined in
# Airflow UI. In this case we are getting the value of my_value and
# setting the environment variable `MY_VALUE`. The pod will fail if
# `my_value` is not set in the Airflow UI.
env_vars={"MY_VALUE": "{{ var.value.my_value }}"},
)
kubernetes_affinity_ex = GKEStartPodOperator(
task_id="ex-pod-affinity",
project_id=PROJECT_ID,
location=CLUSTER_ZONE,
cluster_name=CLUSTER_NAME,
name="ex-pod-affinity",
namespace="default",
image="perl",
cmds=["perl"],
arguments=["-Mbignum=bpi", "-wle", "print bpi(2000)"],
# affinity allows you to constrain which nodes your pod is eligible to
# be scheduled on, based on labels on the node. In this case, if the
# label 'cloud.google.com/gke-nodepool' with value
# 'nodepool-label-value' or 'nodepool-label-value2' is not found on any
# nodes, it will fail to schedule.
affinity={
"nodeAffinity": {
# requiredDuringSchedulingIgnoredDuringExecution means in order
# for a pod to be scheduled on a node, the node must have the
# specified labels. However, if labels on a node change at
# runtime such that the affinity rules on a pod are no longer
# met, the pod will still continue to run on the node.
"requiredDuringSchedulingIgnoredDuringExecution": {
"nodeSelectorTerms": [
{
"matchExpressions": [
{
# When nodepools are created in Google Kubernetes
# Engine, the nodes inside of that nodepool are
# automatically assigned the label
# 'cloud.google.com/gke-nodepool' with the value of
# the nodepool's name.
"key": "cloud.google.com/gke-nodepool",
"operator": "In",
# The label key's value that pods can be scheduled
# on.
"values": [
"pool-1",
],
}
]
}
]
}
}
},
)
kubernetes_full_pod = GKEStartPodOperator(
task_id="ex-all-configs",
name="full",
project_id=PROJECT_ID,
location=CLUSTER_ZONE,
cluster_name=CLUSTER_NAME,
namespace="default",
image="perl",
# Entrypoint of the container, if not specified the Docker container's
# entrypoint is used. The cmds parameter is templated.
cmds=["perl"],
# Arguments to the entrypoint. The docker image's CMD is used if this
# is not provided. The arguments parameter is templated.
arguments=["-Mbignum=bpi", "-wle", "print bpi(2000)"],
# The secrets to pass to Pod, the Pod will fail to create if the
# secrets you specify in a Secret object do not exist in Kubernetes.
secrets=[],
# Labels to apply to the Pod.
labels={"pod-label": "label-name"},
# Timeout to start up the Pod, default is 120.
startup_timeout_seconds=120,
# The environment variables to be initialized in the container
# env_vars are templated.
env_vars={"EXAMPLE_VAR": "/example/value"},
# If true, logs stdout output of container. Defaults to True.
get_logs=True,
# Determines when to pull a fresh image, if 'IfNotPresent' will cause
# the Kubelet to skip pulling an image if it already exists. If you
# want to always pull a new image, set it to 'Always'.
image_pull_policy="Always",
# Annotations are non-identifying metadata you can attach to the Pod.
# Can be a large range of data, and can include characters that are not
# permitted by labels.
annotations={"key1": "value1"},
# Resource specifications for Pod, this will allow you to set both cpu
# and memory limits and requirements.
# Prior to Airflow 1.10.4, resource specifications were
# passed as a Pod Resources Class object,
# If using this example on a version of Airflow prior to 1.10.4,
# import the "pod" package from airflow.contrib.kubernetes and use
# resources = pod.Resources() instead passing a dict
# For more info see:
# https://github.com/apache/airflow/pull/4551
resources={"limit_memory": "250M", "limit_cpu": "100m"},
# If true, the content of /airflow/xcom/return.json from container will
# also be pushed to an XCom when the container ends.
do_xcom_push=False,
# List of Volume objects to pass to the Pod.
volumes=[],
# List of VolumeMount objects to pass to the Pod.
volume_mounts=[],
# Affinity determines which nodes the Pod can run on based on the
# config. For more information see:
# https://kubernetes.io/docs/concepts/configuration/assign-pod-node/
affinity={},
)
delete_cluster = GKEDeleteClusterOperator(
task_id="delete_cluster",
name=CLUSTER_NAME,
project_id=PROJECT_ID,
location=CLUSTER_ZONE,
)
create_cluster >> create_node_pools >> kubernetes_min_pod >> delete_cluster
create_cluster >> create_node_pools >> kubernetes_full_pod >> delete_cluster
create_cluster >> create_node_pools >> kubernetes_affinity_ex >> delete_cluster
create_cluster >> create_node_pools >> kubenetes_template_ex >> delete_cluster
Configuración mínima
Para iniciar un pod en tu clúster de GKE con GKEStartPodOperator
, solo las opciones project_id
, location
, cluster_name
, name
, namespace
, image
y task_id
son obligatorias.
Cuando colocas el siguiente fragmento de código en un DAG, la tarea pod-ex-minimum
funciona siempre que los parámetros enumerados antes estén definidos y sean válidos.
Airflow 2
# TODO(developer): update with your values
PROJECT_ID = "my-project-id"
# It is recommended to use regional clusters for increased reliability
# though passing a zone in the location parameter is also valid
CLUSTER_REGION = "us-west1"
CLUSTER_NAME = "example-cluster"
kubernetes_min_pod = GKEStartPodOperator(
# The ID specified for the task.
task_id="pod-ex-minimum",
# Name of task you want to run, used to generate Pod ID.
name="pod-ex-minimum",
project_id=PROJECT_ID,
location=CLUSTER_REGION,
cluster_name=CLUSTER_NAME,
# Entrypoint of the container, if not specified the Docker container's
# entrypoint is used. The cmds parameter is templated.
cmds=["echo"],
# The namespace to run within Kubernetes, default namespace is
# `default`.
namespace="default",
# Docker image specified. Defaults to hub.docker.com, but any fully
# qualified URLs will point to a custom repository. Supports private
# gcr.io images if the Composer Environment is under the same
# project-id as the gcr.io images and the service account that Composer
# uses has permission to access the Google Container Registry
# (the default service account has permission)
image="gcr.io/gcp-runtimes/ubuntu_18_0_4",
)
Airflow 1
# TODO(developer): update with your values
PROJECT_ID = "my-project-id"
CLUSTER_ZONE = "us-west1-a"
CLUSTER_NAME = "example-cluster"
kubernetes_min_pod = GKEStartPodOperator(
# The ID specified for the task.
task_id="pod-ex-minimum",
# Name of task you want to run, used to generate Pod ID.
name="pod-ex-minimum",
project_id=PROJECT_ID,
location=CLUSTER_ZONE,
cluster_name=CLUSTER_NAME,
# Entrypoint of the container, if not specified the Docker container's
# entrypoint is used. The cmds parameter is templated.
cmds=["echo"],
# The namespace to run within Kubernetes, default namespace is
# `default`.
namespace="default",
# Docker image specified. Defaults to hub.docker.com, but any fully
# qualified URLs will point to a custom repository. Supports private
# gcr.io images if the Composer Environment is under the same
# project-id as the gcr.io images and the service account that Composer
# uses has permission to access the Google Container Registry
# (the default service account has permission)
image="gcr.io/gcp-runtimes/ubuntu_18_0_4",
)
Configuración de la plantilla
Airflow admite el uso de plantillas de Jinja.
Debes declarar las variables obligatorias (task_id
, name
, namespace
y image
) con el operador. Como se muestra en el siguiente ejemplo, puedes crear plantillas de todos los demás parámetros con Jinja, incluidos cmds
, arguments
y env_vars
.
Si no cambias el DAG ni tu entorno, la tarea ex-kube-templates
falla. Configura una variable de Airflow llamada my_value
para que este DAG se realice con éxito.
Para configurar my_value
con gcloud
o la IU de Airflow:
gcloud
Para Airflow 2, ingresa el siguiente comando:
gcloud composer environments run ENVIRONMENT \
--location LOCATION \
variables set -- \
my_value example_value
En Airflow 1, ingresa el siguiente comando:
gcloud composer environments run ENVIRONMENT \
--location LOCATION \
variables -- \
--set my_value example_value
Reemplaza lo siguiente:
ENVIRONMENT
por el nombre del entorno.LOCATION
por la región en la que se encuentra el entorno
IU de Airflow
En la IU de Airflow 2, haz lo siguiente:
En la barra de herramientas, selecciona Administrador > Variables.
En la página Variable de lista, haz clic en Agregar un registro nuevo.
En la página Agregar variable, ingresa la siguiente información:
- Key:
my_value
- Val:
example_value
- Key:
Haz clic en Guardar.
En la IU de Airflow 1, haz lo siguiente:
En la barra de herramientas, selecciona Administrador > Variables.
En la página Variables, haz clic en la pestaña Crear.
En la página Variable, ingresa la siguiente información:
- Key:
my_value
- Val:
example_value
- Key:
Haz clic en Guardar.
Configuración de plantilla:
Airflow 2
# TODO(developer): update with your values
PROJECT_ID = "my-project-id"
# It is recommended to use regional clusters for increased reliability
# though passing a zone in the location parameter is also valid
CLUSTER_REGION = "us-west1"
CLUSTER_NAME = "example-cluster"
kubenetes_template_ex = GKEStartPodOperator(
task_id="ex-kube-templates",
name="ex-kube-templates",
project_id=PROJECT_ID,
location=CLUSTER_REGION,
cluster_name=CLUSTER_NAME,
namespace="default",
image="bash",
# All parameters below are able to be templated with jinja -- cmds,
# arguments, env_vars, and config_file. For more information visit:
# https://airflow.apache.org/docs/apache-airflow/stable/macros-ref.html
# Entrypoint of the container, if not specified the Docker container's
# entrypoint is used. The cmds parameter is templated.
cmds=["echo"],
# DS in jinja is the execution date as YYYY-MM-DD, this docker image
# will echo the execution date. Arguments to the entrypoint. The docker
# image's CMD is used if this is not provided. The arguments parameter
# is templated.
arguments=["{{ ds }}"],
# The var template variable allows you to access variables defined in
# Airflow UI. In this case we are getting the value of my_value and
# setting the environment variable `MY_VALUE`. The pod will fail if
# `my_value` is not set in the Airflow UI.
env_vars={"MY_VALUE": "{{ var.value.my_value }}"},
)
Airflow 1
# TODO(developer): update with your values
PROJECT_ID = "my-project-id"
CLUSTER_ZONE = "us-west1-a"
CLUSTER_NAME = "example-cluster"
kubenetes_template_ex = GKEStartPodOperator(
task_id="ex-kube-templates",
name="ex-kube-templates",
project_id=PROJECT_ID,
location=CLUSTER_ZONE,
cluster_name=CLUSTER_NAME,
namespace="default",
image="bash",
# All parameters below are able to be templated with jinja -- cmds,
# arguments, env_vars, and config_file. For more information visit:
# https://airflow.apache.org/docs/apache-airflow/stable/macros-ref.html
# Entrypoint of the container, if not specified the Docker container's
# entrypoint is used. The cmds parameter is templated.
cmds=["echo"],
# DS in jinja is the execution date as YYYY-MM-DD, this docker image
# will echo the execution date. Arguments to the entrypoint. The docker
# image's CMD is used if this is not provided. The arguments parameter
# is templated.
arguments=["{{ ds }}"],
# The var template variable allows you to access variables defined in
# Airflow UI. In this case we are getting the value of my_value and
# setting the environment variable `MY_VALUE`. The pod will fail if
# `my_value` is not set in the Airflow UI.
env_vars={"MY_VALUE": "{{ var.value.my_value }}"},
)
Configuración de afinidad de los pods
Si configuras el parámetro affinity
en el GKEStartPodOperator
, puedes controlar en qué nodos se programan los pods (por ejemplo, puedes especificar un grupo de nodos en particular). Cuando creaste el clúster, creaste dos grupos de nodos llamados pool-0
y pool-1
. Este operador determina que los pods deben ejecutarse solo en pool-1
.
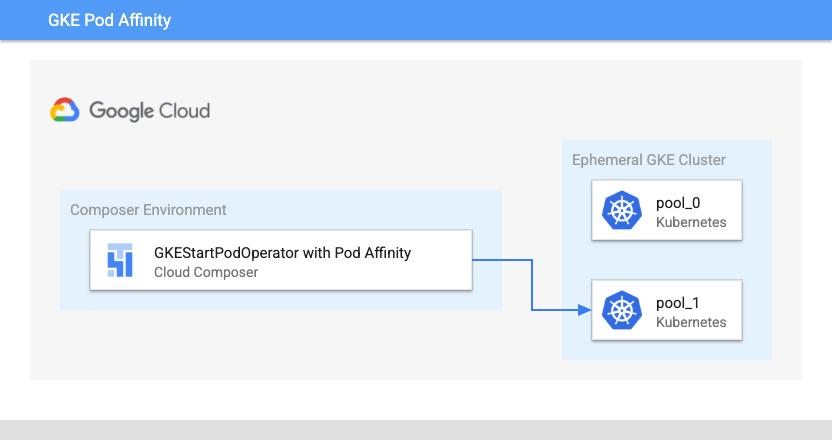
Airflow 2
# TODO(developer): update with your values
PROJECT_ID = "my-project-id"
# It is recommended to use regional clusters for increased reliability
# though passing a zone in the location parameter is also valid
CLUSTER_REGION = "us-west1"
CLUSTER_NAME = "example-cluster"
kubernetes_affinity_ex = GKEStartPodOperator(
task_id="ex-pod-affinity",
project_id=PROJECT_ID,
location=CLUSTER_REGION,
cluster_name=CLUSTER_NAME,
name="ex-pod-affinity",
namespace="default",
image="perl",
cmds=["perl"],
arguments=["-Mbignum=bpi", "-wle", "print bpi(2000)"],
# affinity allows you to constrain which nodes your pod is eligible to
# be scheduled on, based on labels on the node. In this case, if the
# label 'cloud.google.com/gke-nodepool' with value
# 'nodepool-label-value' or 'nodepool-label-value2' is not found on any
# nodes, it will fail to schedule.
affinity={
"nodeAffinity": {
# requiredDuringSchedulingIgnoredDuringExecution means in order
# for a pod to be scheduled on a node, the node must have the
# specified labels. However, if labels on a node change at
# runtime such that the affinity rules on a pod are no longer
# met, the pod will still continue to run on the node.
"requiredDuringSchedulingIgnoredDuringExecution": {
"nodeSelectorTerms": [
{
"matchExpressions": [
{
# When nodepools are created in Google Kubernetes
# Engine, the nodes inside of that nodepool are
# automatically assigned the label
# 'cloud.google.com/gke-nodepool' with the value of
# the nodepool's name.
"key": "cloud.google.com/gke-nodepool",
"operator": "In",
# The label key's value that pods can be scheduled
# on.
"values": [
"pool-1",
],
}
]
}
]
}
}
},
)
Airflow 1
# TODO(developer): update with your values
PROJECT_ID = "my-project-id"
CLUSTER_ZONE = "us-west1-a"
CLUSTER_NAME = "example-cluster"
kubernetes_affinity_ex = GKEStartPodOperator(
task_id="ex-pod-affinity",
project_id=PROJECT_ID,
location=CLUSTER_ZONE,
cluster_name=CLUSTER_NAME,
name="ex-pod-affinity",
namespace="default",
image="perl",
cmds=["perl"],
arguments=["-Mbignum=bpi", "-wle", "print bpi(2000)"],
# affinity allows you to constrain which nodes your pod is eligible to
# be scheduled on, based on labels on the node. In this case, if the
# label 'cloud.google.com/gke-nodepool' with value
# 'nodepool-label-value' or 'nodepool-label-value2' is not found on any
# nodes, it will fail to schedule.
affinity={
"nodeAffinity": {
# requiredDuringSchedulingIgnoredDuringExecution means in order
# for a pod to be scheduled on a node, the node must have the
# specified labels. However, if labels on a node change at
# runtime such that the affinity rules on a pod are no longer
# met, the pod will still continue to run on the node.
"requiredDuringSchedulingIgnoredDuringExecution": {
"nodeSelectorTerms": [
{
"matchExpressions": [
{
# When nodepools are created in Google Kubernetes
# Engine, the nodes inside of that nodepool are
# automatically assigned the label
# 'cloud.google.com/gke-nodepool' with the value of
# the nodepool's name.
"key": "cloud.google.com/gke-nodepool",
"operator": "In",
# The label key's value that pods can be scheduled
# on.
"values": [
"pool-1",
],
}
]
}
]
}
}
},
)
Configuración completa
En este ejemplo, se muestran todas las variables que puedes configurar en GKEStartPodOperator
. No es necesario modificar el código para que la tarea ex-all-configs
se realice con éxito.
Para obtener detalles sobre cada variable, consulta la referencia de Airflow para los operadores de GKE.
Airflow 2
# TODO(developer): update with your values
PROJECT_ID = "my-project-id"
# It is recommended to use regional clusters for increased reliability
# though passing a zone in the location parameter is also valid
CLUSTER_REGION = "us-west1"
CLUSTER_NAME = "example-cluster"
kubernetes_full_pod = GKEStartPodOperator(
task_id="ex-all-configs",
name="full",
project_id=PROJECT_ID,
location=CLUSTER_REGION,
cluster_name=CLUSTER_NAME,
namespace="default",
image="perl:5.34.0",
# Entrypoint of the container, if not specified the Docker container's
# entrypoint is used. The cmds parameter is templated.
cmds=["perl"],
# Arguments to the entrypoint. The docker image's CMD is used if this
# is not provided. The arguments parameter is templated.
arguments=["-Mbignum=bpi", "-wle", "print bpi(2000)"],
# The secrets to pass to Pod, the Pod will fail to create if the
# secrets you specify in a Secret object do not exist in Kubernetes.
secrets=[],
# Labels to apply to the Pod.
labels={"pod-label": "label-name"},
# Timeout to start up the Pod, default is 120.
startup_timeout_seconds=120,
# The environment variables to be initialized in the container
# env_vars are templated.
env_vars={"EXAMPLE_VAR": "/example/value"},
# If true, logs stdout output of container. Defaults to True.
get_logs=True,
# Determines when to pull a fresh image, if 'IfNotPresent' will cause
# the Kubelet to skip pulling an image if it already exists. If you
# want to always pull a new image, set it to 'Always'.
image_pull_policy="Always",
# Annotations are non-identifying metadata you can attach to the Pod.
# Can be a large range of data, and can include characters that are not
# permitted by labels.
annotations={"key1": "value1"},
# Optional resource specifications for Pod, this will allow you to
# set both cpu and memory limits and requirements.
# Prior to Airflow 2.3 and the cncf providers package 5.0.0
# resources were passed as a dictionary. This change was made in
# https://github.com/apache/airflow/pull/27197
# Additionally, "memory" and "cpu" were previously named
# "limit_memory" and "limit_cpu"
# resources={'limit_memory': "250M", 'limit_cpu': "100m"},
container_resources=k8s_models.V1ResourceRequirements(
limits={"memory": "250M", "cpu": "100m"},
),
# If true, the content of /airflow/xcom/return.json from container will
# also be pushed to an XCom when the container ends.
do_xcom_push=False,
# List of Volume objects to pass to the Pod.
volumes=[],
# List of VolumeMount objects to pass to the Pod.
volume_mounts=[],
# Affinity determines which nodes the Pod can run on based on the
# config. For more information see:
# https://kubernetes.io/docs/concepts/configuration/assign-pod-node/
affinity={},
)
Airflow 1
# TODO(developer): update with your values
PROJECT_ID = "my-project-id"
CLUSTER_ZONE = "us-west1-a"
CLUSTER_NAME = "example-cluster"
kubernetes_full_pod = GKEStartPodOperator(
task_id="ex-all-configs",
name="full",
project_id=PROJECT_ID,
location=CLUSTER_ZONE,
cluster_name=CLUSTER_NAME,
namespace="default",
image="perl",
# Entrypoint of the container, if not specified the Docker container's
# entrypoint is used. The cmds parameter is templated.
cmds=["perl"],
# Arguments to the entrypoint. The docker image's CMD is used if this
# is not provided. The arguments parameter is templated.
arguments=["-Mbignum=bpi", "-wle", "print bpi(2000)"],
# The secrets to pass to Pod, the Pod will fail to create if the
# secrets you specify in a Secret object do not exist in Kubernetes.
secrets=[],
# Labels to apply to the Pod.
labels={"pod-label": "label-name"},
# Timeout to start up the Pod, default is 120.
startup_timeout_seconds=120,
# The environment variables to be initialized in the container
# env_vars are templated.
env_vars={"EXAMPLE_VAR": "/example/value"},
# If true, logs stdout output of container. Defaults to True.
get_logs=True,
# Determines when to pull a fresh image, if 'IfNotPresent' will cause
# the Kubelet to skip pulling an image if it already exists. If you
# want to always pull a new image, set it to 'Always'.
image_pull_policy="Always",
# Annotations are non-identifying metadata you can attach to the Pod.
# Can be a large range of data, and can include characters that are not
# permitted by labels.
annotations={"key1": "value1"},
# Resource specifications for Pod, this will allow you to set both cpu
# and memory limits and requirements.
# Prior to Airflow 1.10.4, resource specifications were
# passed as a Pod Resources Class object,
# If using this example on a version of Airflow prior to 1.10.4,
# import the "pod" package from airflow.contrib.kubernetes and use
# resources = pod.Resources() instead passing a dict
# For more info see:
# https://github.com/apache/airflow/pull/4551
resources={"limit_memory": "250M", "limit_cpu": "100m"},
# If true, the content of /airflow/xcom/return.json from container will
# also be pushed to an XCom when the container ends.
do_xcom_push=False,
# List of Volume objects to pass to the Pod.
volumes=[],
# List of VolumeMount objects to pass to the Pod.
volume_mounts=[],
# Affinity determines which nodes the Pod can run on based on the
# config. For more information see:
# https://kubernetes.io/docs/concepts/configuration/assign-pod-node/
affinity={},
)
Borre el clúster
El código que se muestra aquí borra el clúster que se creó al comienzo de la guía.
Airflow 2
delete_cluster = GKEDeleteClusterOperator(
task_id="delete_cluster",
name=CLUSTER_NAME,
project_id=PROJECT_ID,
location=CLUSTER_REGION,
)
Airflow 1
delete_cluster = GKEDeleteClusterOperator(
task_id="delete_cluster",
name=CLUSTER_NAME,
project_id=PROJECT_ID,
location=CLUSTER_ZONE,
)