10 tips for building long-running clusters using Cloud Dataproc
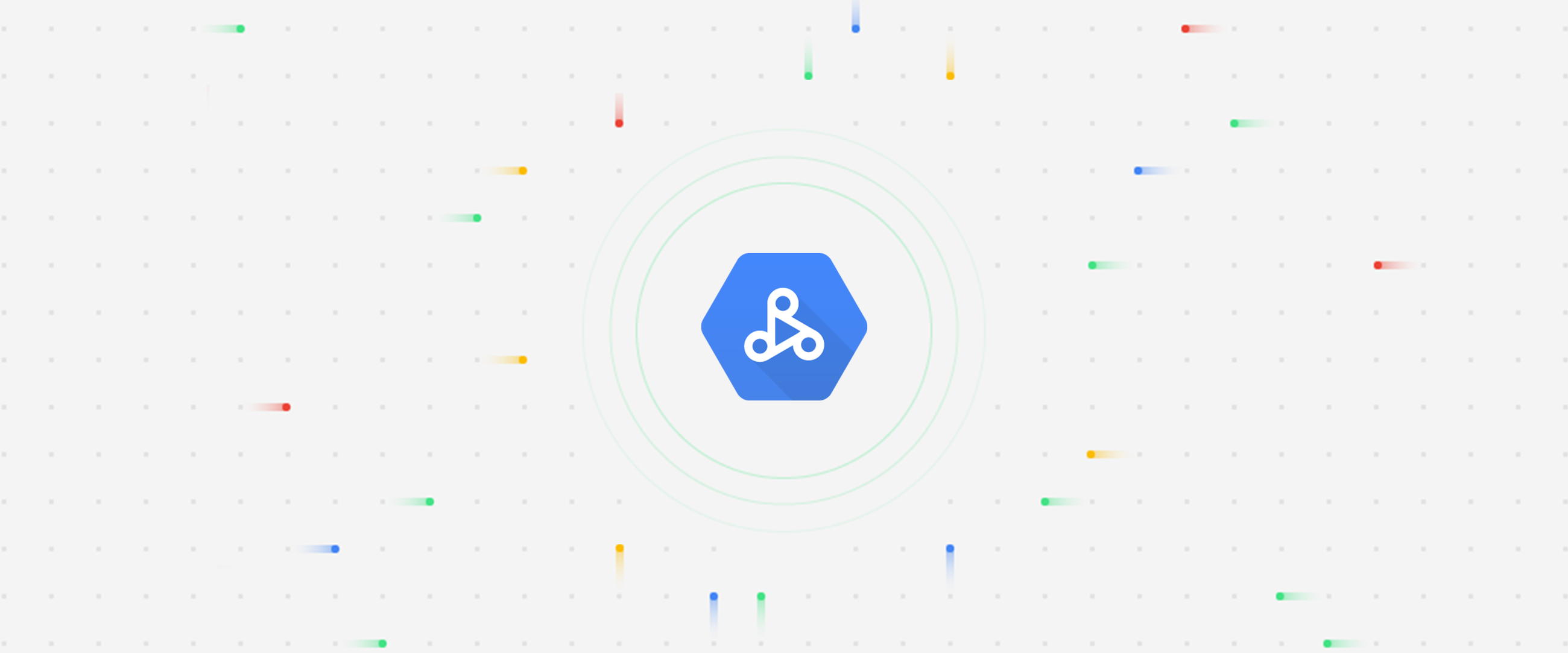
Christopher Crosbie
Group Product Manager, Google
Mikayla Konst
Software Engineer, Cloud Dataproc
Google’s Cloud Dataproc is a fast, easy-to-use, fully managed cloud service for running Apache Spark and Apache Hadoop clusters in a simple, cost-efficient way. Google Cloud Platform (GCP) customers like Pandora and Outbrain depend on Cloud Dataproc to run their Hadoop and Spark jobs.
A key differentiator for Cloud Dataproc is that it is optimized to create ephemeral job-scoped clusters in around 90 seconds. This speed of deployment means that a single job can have a dedicated cluster, containing just the resources needed to run the job, that is shut down upon job completion. On the Cloud Dataproc team, we’ve worked with countless customers who are creating clusters for their particular use cases. However, not all Hadoop and Spark workloads are appropriately served by an ephemeral job-scoped cluster model. Our goal on the Cloud Dataproc team is to make sure every customer’s use case can be addressed. To that end, we’re excited to share these tips and recommendations for using Cloud Dataproc in a non-ephemeral model.
How Cloud Dataproc clusters work
If you’re just getting started, here’s a quick primer on how Cloud Dataproc works. When you use Cloud Dataproc to create clusters, you can have a seemingly limitless amount of computation running in parallel, since you have access to GCP’s global fleet of virtual machines. As a result, you don’t need to manage YARN queues and isolate runaway jobs like you would with Hadoop or Spark clusters running on-premises. In the diagram below, you can see a representation of how, for each job, Cloud Dataproc can deploy a cluster sized to match that job’s requirements.
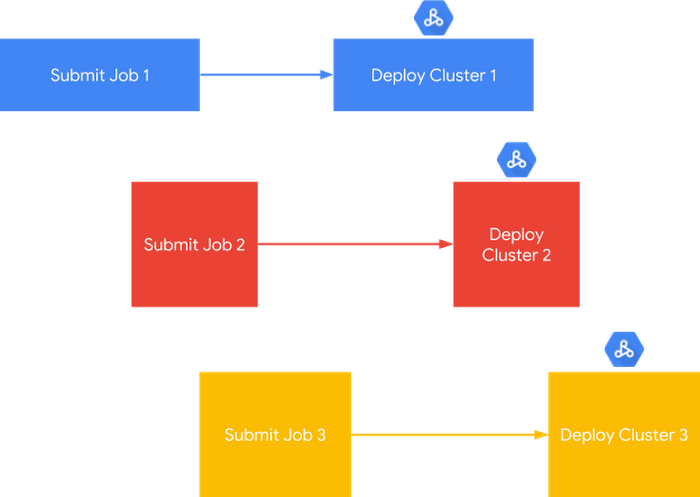
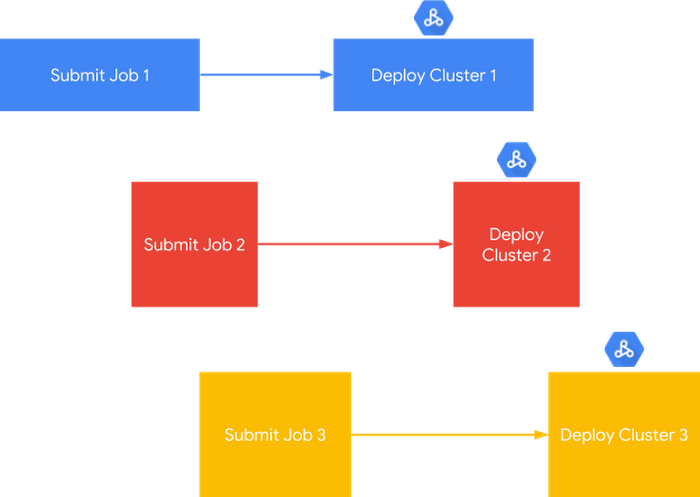
Specific features that make running job-scoped clusters in Cloud Dataproc fast, easy and cost-effective include the Jobs API, Workflow Templates API, Cluster Scheduled Deletion and Service Accounts.
While the job-scoped cluster model has been effective for our Cloud Dataproc customers with batch processing and ETL/ELT jobs, we’ve heard that there are a variety of other use cases where balancing semi-long-running clusters alongside cloud capabilities is critical.
Some scenarios for semi-long-running Cloud Dataproc clusters include:
- Interactive or ad hoc analysis, often through Cloud Dataproc web-based notebook optional components Jupyter and Zeppelin
- Reporting/dashboarding applications that expose cross-database queries in Presto
- Data and SQL exploration tools added to Cloud Dataproc with init actions (for example, HUE)
- Streaming jobs such as those found in Apache Spark DStreams architectures or Beam on Flink deployments
- Continually running jobs in workflow engines like Oozie
From these use cases and the conversations we have with customers, we see a pattern emerging for how teams want to shift long-running jobs to the cloud. There is a real desire to share the cluster among many users, which means 24/7 availability, while at the same time not being locked into the same confines that exist with on-premises Hadoop/Spark clusters. At its most basic, the long-running cloud cluster model that we hear customers want looks like this:
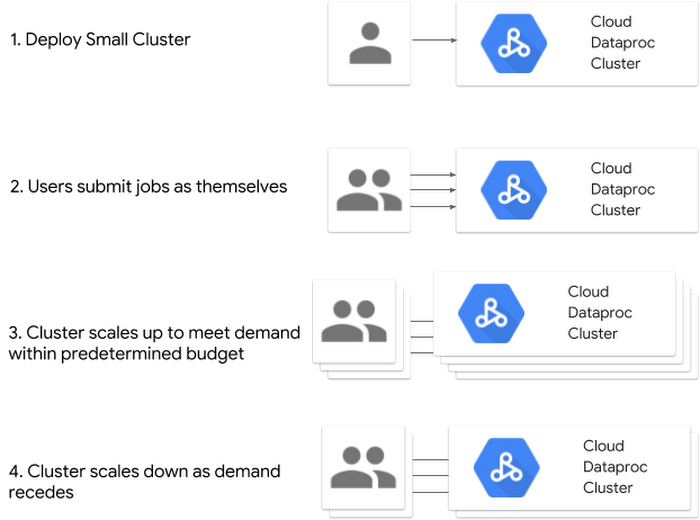
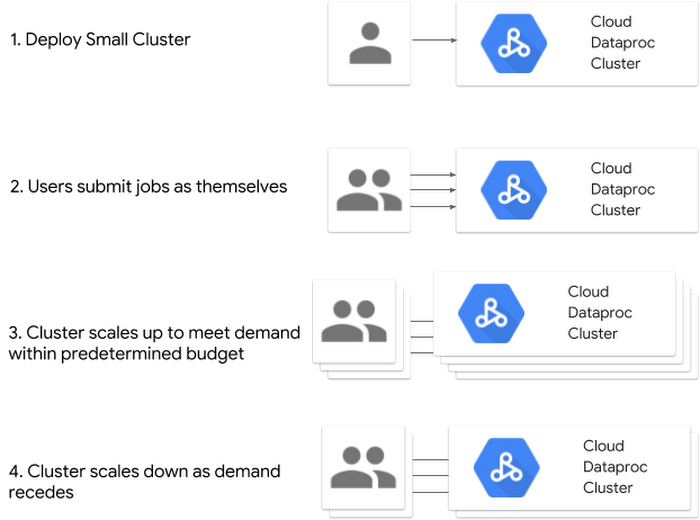
In this model, the goal is to deploy a small cluster, submit the jobs as the end user, and then have the cluster dynamically scale up and down to meet demand within a predetermined budget. To make this easier, Cloud Dataproc recently made a smart autoscaler available in alpha, which examines pending and available YARN memory averaged over a configurable period of time to determine an exact number of workers to add or remove from the cluster. In addition, to provide enhanced user-level security within the cluster itself, the Kerberos Optional Component for Cloud Dataproc includes and configures the MIT distribution of Kerberos to provide user authentication, isolation, and encryption. In this model, High Availability (HA) mode can also be useful in the rare event of a Google Compute Engine failure of the master node. In HA mode, HDFS High Availability and YARN High Availability are configured to allow uninterrupted YARN and HDFS operations despite any single-node failures/reboots.
Below, we have compiled a list of top 10 tips based on what we’ve learned from customers who have successfully built and continue to use semi-long-running Cloud Dataproc clusters. A common element to the success of these semi-long-running clusters is the practice of storing stateful data to GCP and then using Cloud Dataproc clusters for processing. The underlying theme behind all these tips is not building a single cluster that lives forever. Rather, use the automation and services of GCP to move the persistent data off the cluster. This is essential to letting you manage and scale compute resources independently of the data, apply the right tool to the job, and capture the value of the cloud, even for long-running clusters.
While the focus on these tips is for Cloud Dataproc, many of the same techniques and concepts can be applied to running the Hortonworks Data Platform on Google Cloud.
10 tips for building long-running Cloud Dataproc clusters
1. Use Google Cloud Storage as your primary data source and sink2. Persist information on how to build your clusters
3. Identify a source control mechanism
4. Externalize the Hive metastore database with Cloud SQL
5. Use cloud authentication and authorization policies
6. Know your way around Stackdriver
7. Transform YARN queues into workflow templates
8. Start small and enable autoscaling
9. Consolidate job history across multiple clusters
10. Take advantage of GCP services
Tip 1. Use Google Cloud Storage as your primary data source and sink
This tip is first because it is imperative to achieving “cloud freedom” for a cluster (i.e., severing dependencies between storage and compute). Luckily, this is often the easiest change to make, since converting from HDFS to Cloud Storage is usually as simple as a file prefix change (more on HDFS vs. Cloud Storage here).
The bottom line is that you can’t scale with HDFS because storage is still tied to compute. Not only does HDFS couple a very pricey resource—compute—with a relatively inexpensive resource—storage—but it also siloes the data into a single cluster instead of exposing it to all the possibilities offered by GCP.
There are still plenty of reasons to use a Cloud Dataproc storage device such as local SSDs, but the purposes should primarily be limited to ephemeral data such as scratch space, shuffle data, and LLAP cache.
Tip 2. Persist information on how to build your clusters
When creating a Cloud Dataproc cluster, there are several ways to represent the cluster itself as code. This lets you maintain a representation of the cluster even when it is not running.
The first method of storing a cluster as code is by specifying initialization actions, which are executables or scripts that Cloud Dataproc will run on all nodes in the cluster as soon as it’s set up. Initialization actions often set up job dependencies, such as installing Python packages, so that when you do need to tear down a long-running cluster or re-create a cluster to update it to the latest version of Cloud Dataproc, you can recreate the environment automatically with the initialization action. Check out the Cloud Dataproc Github repository of initialization actions for examples.
Alternatively, you can get started by creating a Cloud Dataproc custom image, which captures everything that’s installed on the disk. You can use that image across a variety of cluster shapes and sizes without having to write scripts to do the installation and configuration.
In addition, to simply capture the information in the cluster configuration files, you can export a running cluster configuration to a YAML file. This same YAML can then be used as an input to the import command, which can create a new cluster with the same configuration.
Tip 3. Identify a source control mechanism
Once data is securely stored in Cloud Storage and accessible to the cloud resources that you have identified, the next question we usually hear is “Where do I store my code?” A cloud migration is a great time to identify a source control mechanism that works for all the analytics users as well as the developers.
While most Java developers are experienced in source control, often, in analytics environments, the server’s local file system ends up morphing into a code repository for things like SQL queries, Python scripts and notebook files. Often the solution to this problem is not as simple as “just use Git,” because many of the SQL interfaces are not as well-integrated with source controls as integrated development environments (IDEs) are. Even popular notebooks like Jupyter require additional tools like nbviewer to properly render the notebook’s interactive features.
While a user’s folder on the cluster may have provided a way to get by in the past, users will get frustrated quickly, since the work left in these folders disappears as clusters become more ephemeral in the cloud. Cloud Dataproc has taken some steps to mitigate this. For example, when using the Jupyter optional component, Cloud Dataproc will automatically configure notebooks to be backed up with Cloud Storage, making the same notebook files accessible to other clusters. However, these mitigations should not be a substitute for a well-thought-out source control framework. Whatever framework you choose, be sure to include the scripts and code that you use to build your clusters as well.
For more on source control that is directly integrated with GCP, check out Cloud Source Repositories.
Tip 4. Externalize the Hive metastore database with Cloud SQL
The Hive metastore holds metadata about Hive tables, such as their schema and location, which in the cloud is usually an external table location of Cloud Storage. MySQL is commonly used as a back end for the Hive metastore. When you’re using GCP, Cloud SQL (our fully managed database service supporting MySQL or PostgreSQL) makes it easy to set up, maintain, manage, and administer that MySQL-based Hive backend. With Cloud Dataproc, you can use a Google-written and -maintained initialization action to set up and configure a Cloud Dataproc cluster to use an external Hive metastore database in Cloud SQL.
Using Cloud SQL as the Hive metastore database makes it easy to discover metadata and makes it possible to attach multiple clusters to the same source of Hive metadata. Additionally, Ranger and Atlas policy data can be stored in this database, persisted, and used across many clusters.
For a full tutorial on externalizing the Hive metastore database, see Using Apache Hive on Cloud Dataproc.
Tip 5. Use cloud authentication and authorization policies
Cloud authentication and authorization will vary greatly by company, and a full discussion is beyond the scope of this post. However, the principal point of this tip is that you should use the controls available in GCP’s Identity and Access Management as much as possible.
A common misstep we see some customers make is trying to bring only the security controls maintained in their clusters when moving Hadoop workloads to the cloud. This can be problematic when clusters become ephemeral. To properly secure clusters, you need to control the permissions of other cloud services (such as Cloud Storage) and control who has the ability to build the clusters themselves.
An important first step is understanding Cloud Dataproc permissions and IAM roles. Cloud Dataproc separates permissions into two categories: clusters and jobs. Cluster permissions are for administrators building the clusters and jobs are for the developers who submit code to the cluster. Granular IAM can also be used to limit which cluster(s) users can perform which actions on.
Using the OS Login feature of Compute Engine can also simplify how you manage SSH access to the Cloud Dataproc clusters. With OS Login enabled, the IAM permissions are automatically mapped to a Linux identity and there’s no longer a need to create SSH keys.
In addition to Cloud IAM, Cloud Dataproc has a Kerberos Optional Component, which customers often use to extend Cloud IAM into the cluster itself or to use existing Active Directory-based sources of identity, as shown here:
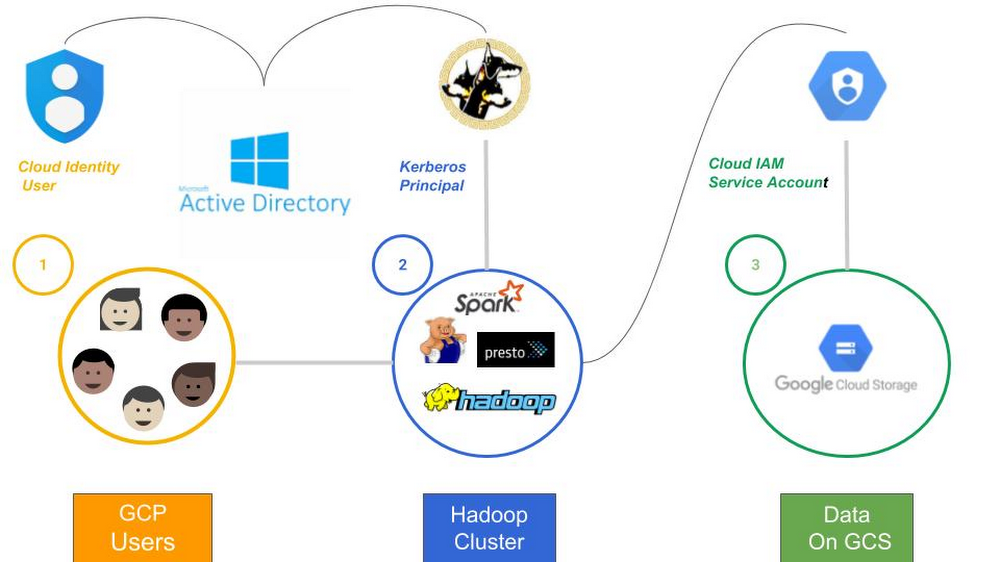
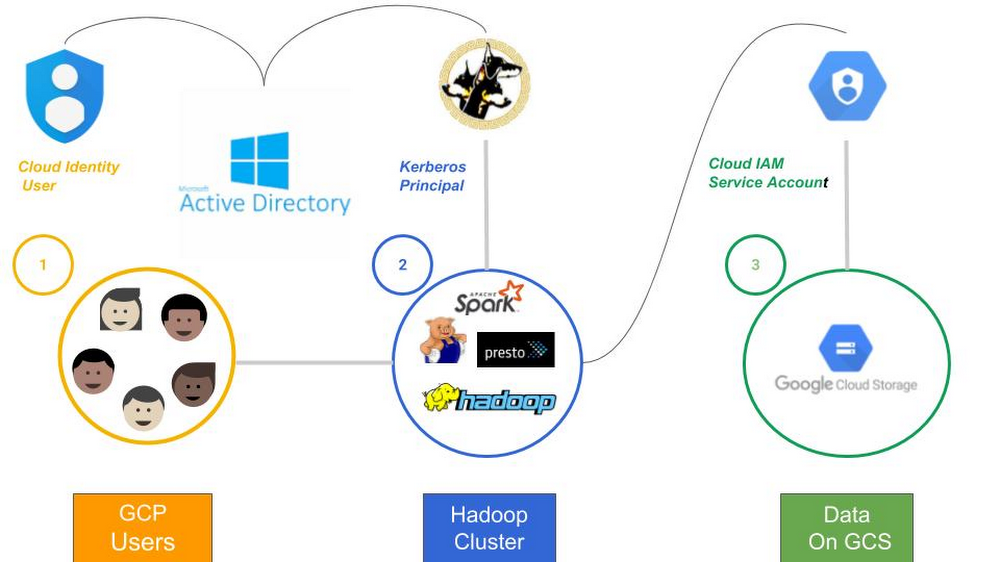
- Each GCP user is associated with a cloud identity. This authentication mechanism gives users the ability to SSH into a cluster, run jobs via the API and to create cloud resources (i.e., a Cloud Dataproc cluster).
- When a cloud user wants to use a Kerberized “Hadoop” application, a Kerberos principal must be obtained. Microsoft Active Directory is used as a cross-realm trust to users and groups that map into Cloud Dataproc Kerberos principals.
Note: This setup requires Active Directory to be source of truth for user identities. Cloud Identity is only a synchronized copy.
When the “Hadoop” application needs to obtain data from Cloud Storage, a Cloud Storage Connector is invoked. The Cloud Storage Connector allows “Hadoop” to access Cloud Storage data at the block level as if it were a native part of Hadoop. This connector relies on a Service Accounts to authenticate against Cloud Storage.
Tip 6. Know your way around Stackdriver
Stackdriver is the default way to persist an audit trail with ephemeral Cloud Dataproc resources.
Stackdriver logging is used capture the daemon logs and YARN container logs from Cloud Dataproc clusters. This is in addition to Stackdriver monitoring, which collects and ingests metrics, events, and metadata from Cloud Dataproc clusters to generate insights via dashboards and charts. You can use Stackdriver to understand the performance and health across all Cloud Dataproc clusters at once and examine HDFS, YARN, and Cloud Dataproc job and operation metrics. If an issue is identified, Stackdriver makes it easy to drill into a specific cluster’s metrics.
An easy way to get started is by going into Stackdriver Monitoring, opening Metrics Explorer, selecting the relevant resource for Cloud Dataproc and reviewing what is available.
In the rare situation you would like to send additional metrics, Cloud Dataproc customers can build a custom metric by enabling it through the Cloud Dataproc cluster property "dataproc:dataproc.monitoring.stackdriver.enable" during the cluster creation.
We’ve heard from customers that the Stackdriver integration is immensely helpful, and often that customers wish they had invested more up-front in building out their Stackdriver environment at the beginning of their cloud migration.
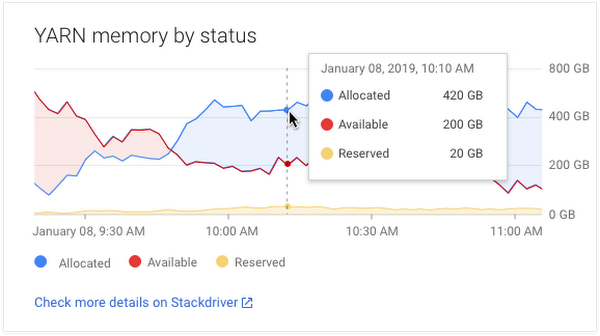
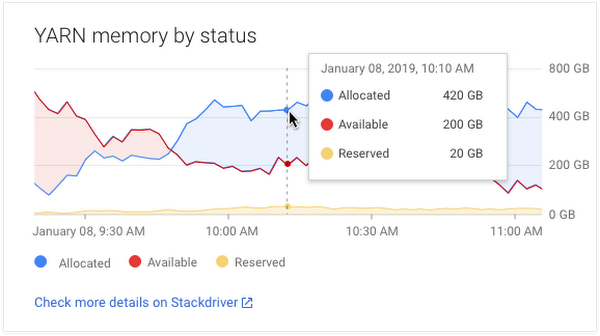
Tip 7. Transform YARN queues into workflow templates
Even with long-running clusters, the idea of managing YARN queues should begin to fade as you migrate to cloud-native architecture designs. For best results, transition YARN queues into separate clusters with unique cluster shapes and potentially different permissions. As long as you have persisted the storage of long-lived elements off the cluster, you can use the same data, metadata, and permissioning systems across the various clusters.
Workflow templates can make these right-sized clusters easier to configure, since they run in a single workload. A workflow template is a reusable workflow configuration. It defines a graph of jobs with information on where to run those jobs and ultimately allows you to iterate and right-size clusters by making lightweight tweaks.
Learn more about getting started with Cloud Dataproc workflow templates.
Tip 8. Start small and enable autoscaling
Don’t waste time trying to generate the perfect cluster configurations. Simply start with a small cluster that can autoscale to the needed (or allowable) size. The Cloud Dataproc autoscaler has a variety of settings to fine-tune how you would like the autoscaler to behave. An autoscaling cluster is a great way to have a small but available long-running cluster that can quickly become a large-scale data cruncher as soon as the workload requests it.
For more on the customizations exposed by the Cloud Dataproc autoscaler, check out Autoscaling Clusters.
Tip 9. Consolidate job history across multiple clusters
We hear that many customers want to persist job history information across multiple clusters. In Cloud Dataproc, you can achieve this by pointing the MapReduce and Spark job history servers at Cloud Storage. Override the MapReduce done directory and intermediate done directory and the Spark event log directory with Cloud Storage directories, like this:
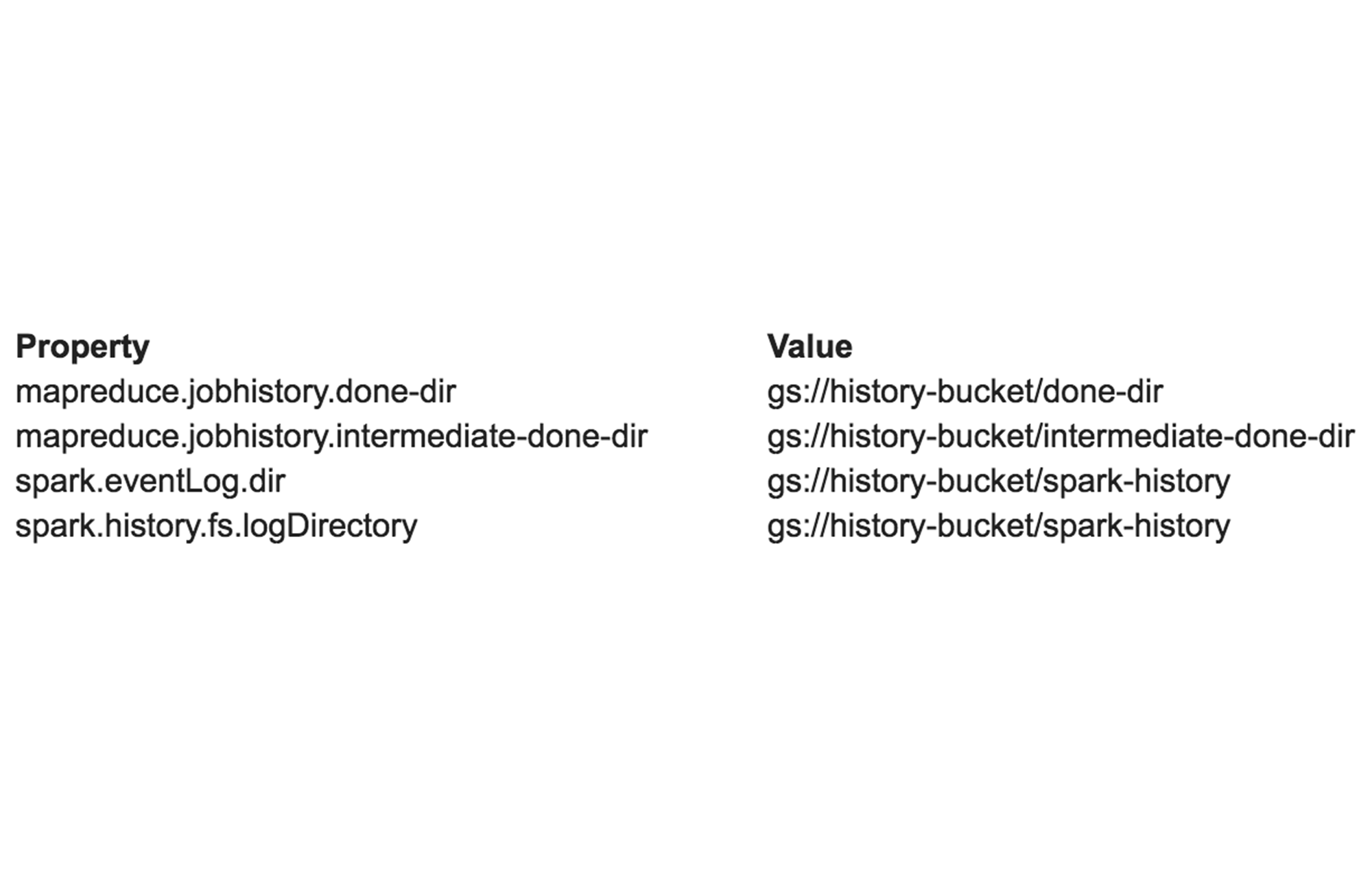
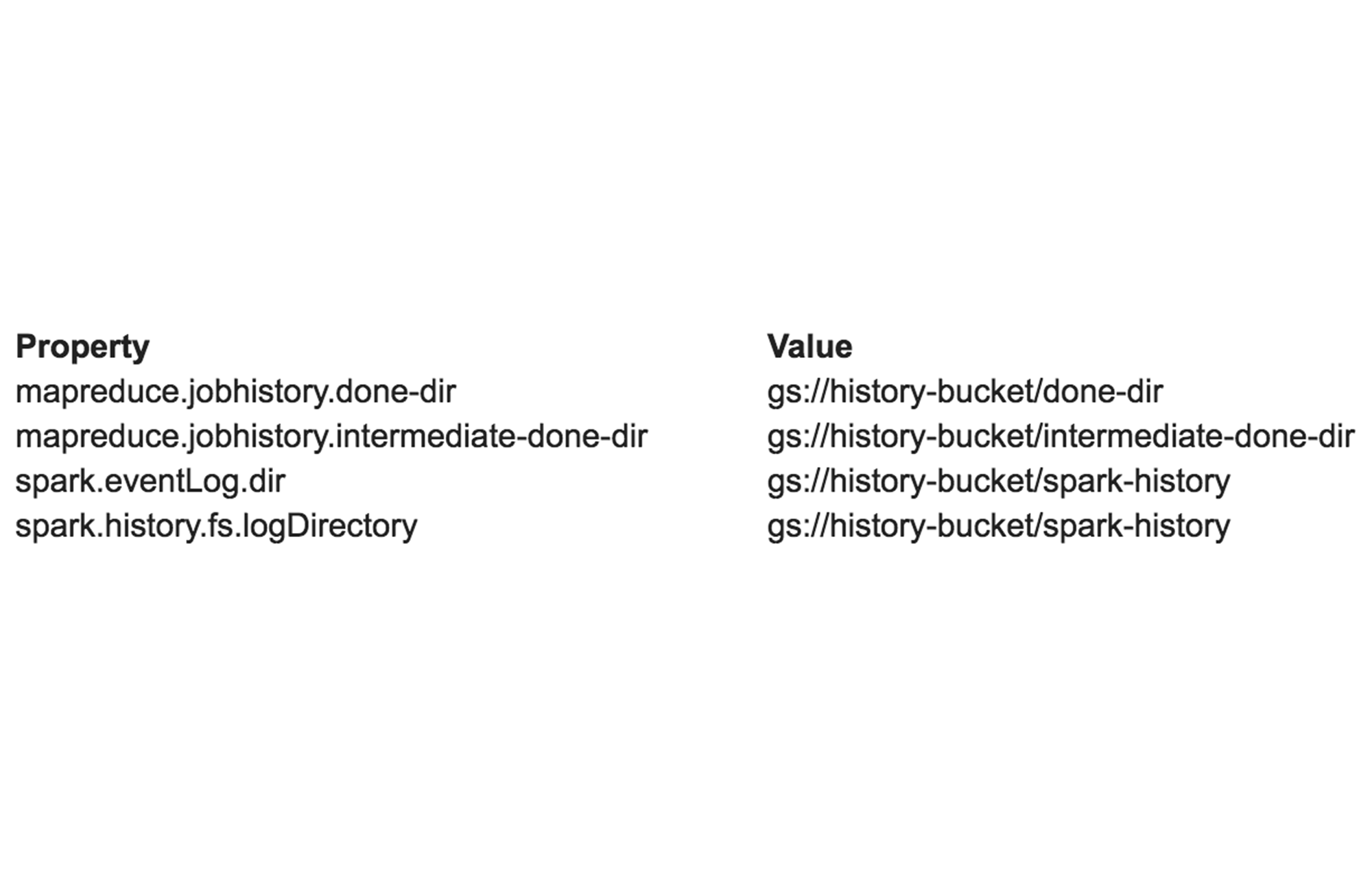
When specifying Cloud Storage directories, there are a few things to watch out for. The first is that you should ensure that all directories are a subdirectory within a bucket (gs://bucket/dir) as opposed to a top-level bucket (gs://bucket). The second is that the two Spark event log directories must match exactly. Also note that you must manually create an empty Spark event log directory before running any Spark jobs. Unlike the MapReduce job history directories, this directory is not created automatically. Finally, when creating a cluster, make sure that all properties include the appropriate prefix: mapred: for MapReduce properties, spark: for Spark properties.
This technique, while useful, does come with a couple of potential pitfalls. The first is that because the MapReduce job history server periodically moves files from the intermediate done directory to the done directory, a job may finish before its history files have been moved. Make sure that the history files for a job have actually been moved before terminating a cluster if you need to have the complete job history.
The second caveat is that the MapReduce job history server only reads history from Cloud Storage when it first starts up. From that point forward, the only new job history you will see in the UI is for the jobs that were run on that cluster; in other words, these are jobs whose history was moved by that cluster’s job history server. By contrast, the Spark job history server is completely stateless, so the previous caveats do not apply.
To avoid these pitfalls, try an architecture in which the job history servers are run on a single-node cluster. Create a single-node cluster that has the above four properties configured to point to Cloud Storage. Then, when creating additional clusters, point them at the job history servers on your single-node cluster by setting the above four properties along with the following additional two properties:
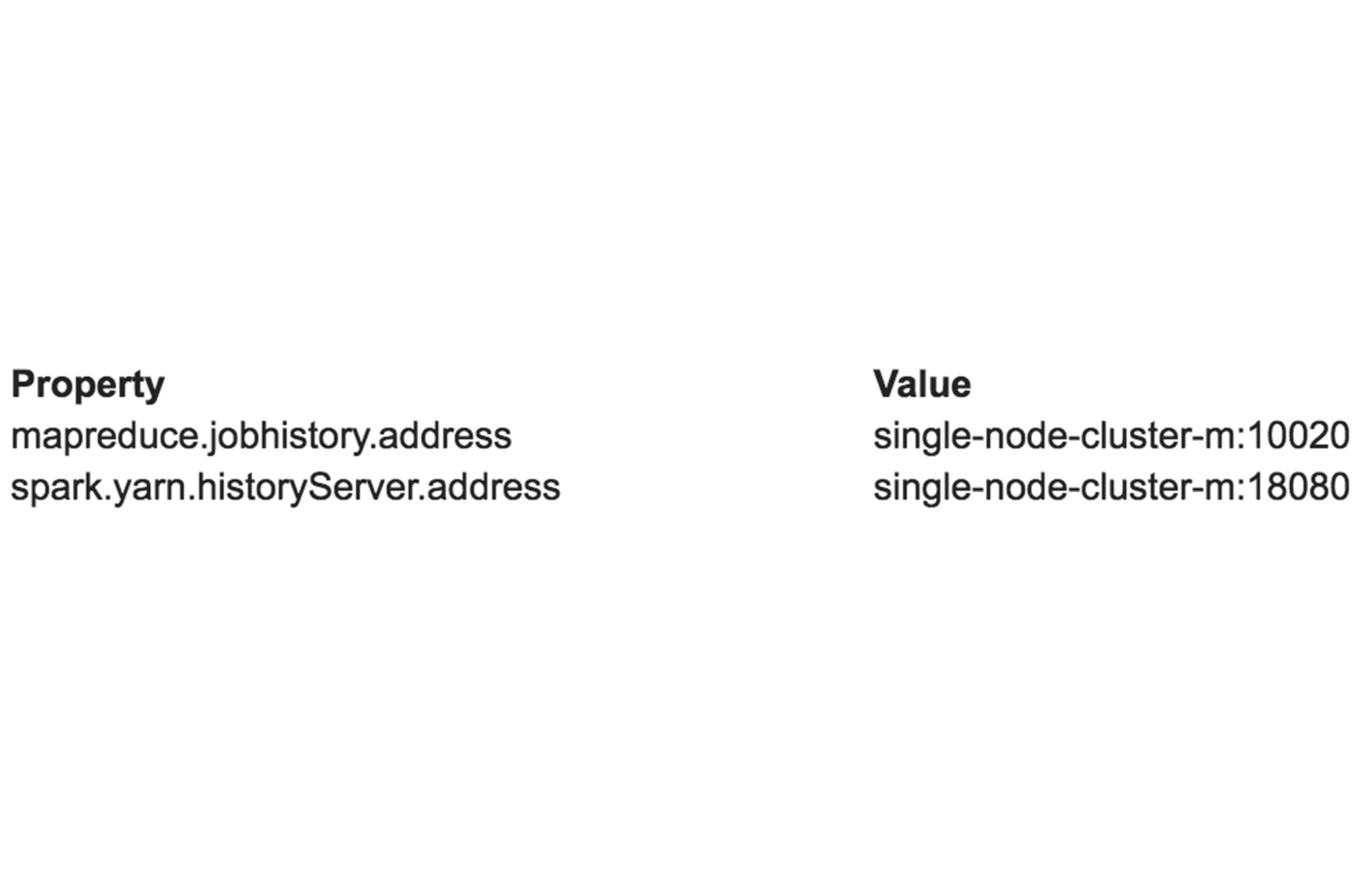
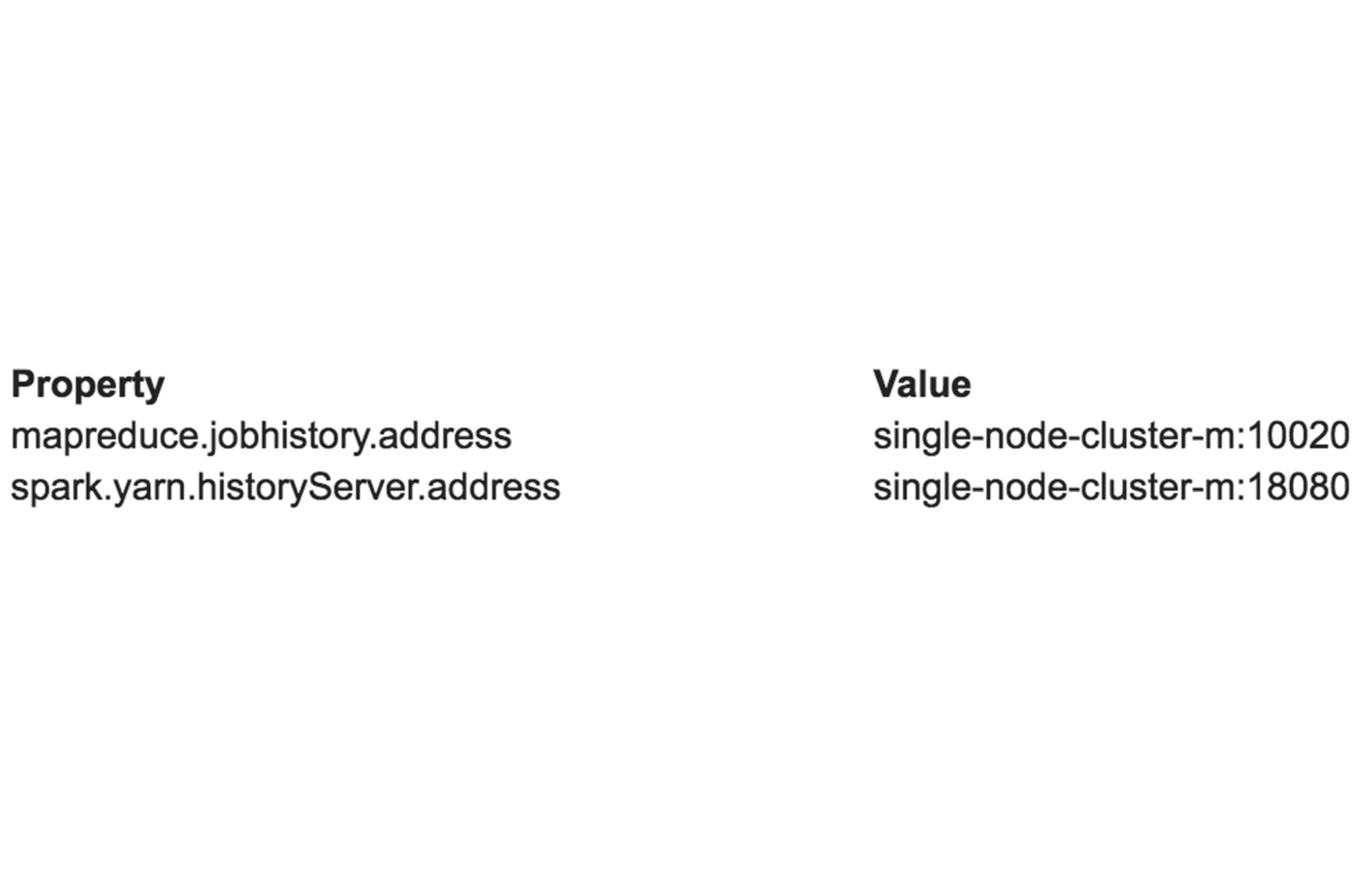
Note that if you take this approach, you should consider running an initialization action on your additional clusters to disable their job history servers, which should no longer be used. This might look something like:
systemctl stop hadoop-mapreduce-historyserver
systemctl stop spark-history-server
If you run MapReduce and Spark jobs on any of your clusters, you should be able to see the job history for all of your clusters in one place by accessing the web UIs of your single-node cluster (port 19888 for the MapReduce job history, port 18080 for the Spark job history). For more information on accessing web UIs, check out Cluster web interfaces.
Tip 10. Take advantage of GCP services
"Hadoop" has become a catch-all term for frameworks that run open source big data software in a somewhat standardized way. The phrase Hadoop has become synonymous with software such as Spark, Presto and Kafka (to name a few), despite having only loose ties to the Hadoop Distributed File System (HDFS) and MapReduce frameworks that were the original Hadoop applications. GCP is an alternative big data stack. So knowing how to properly map GCP data and analytics services to long-lived Hadoop workloads and applications is essential to extract value from the cloud.
For example, consider migrating HBase to Cloud Bigtable if you don’t require co-processors or the SQL capabilities of Apache Phoenix. If you have data analytics well-versed in SQL, consider BigQuery as an alternative to Hive. If you are using Spark with scikit-learn, consider Cloud Machine Learning Engine as a way to not only train your model but easily move it to production.
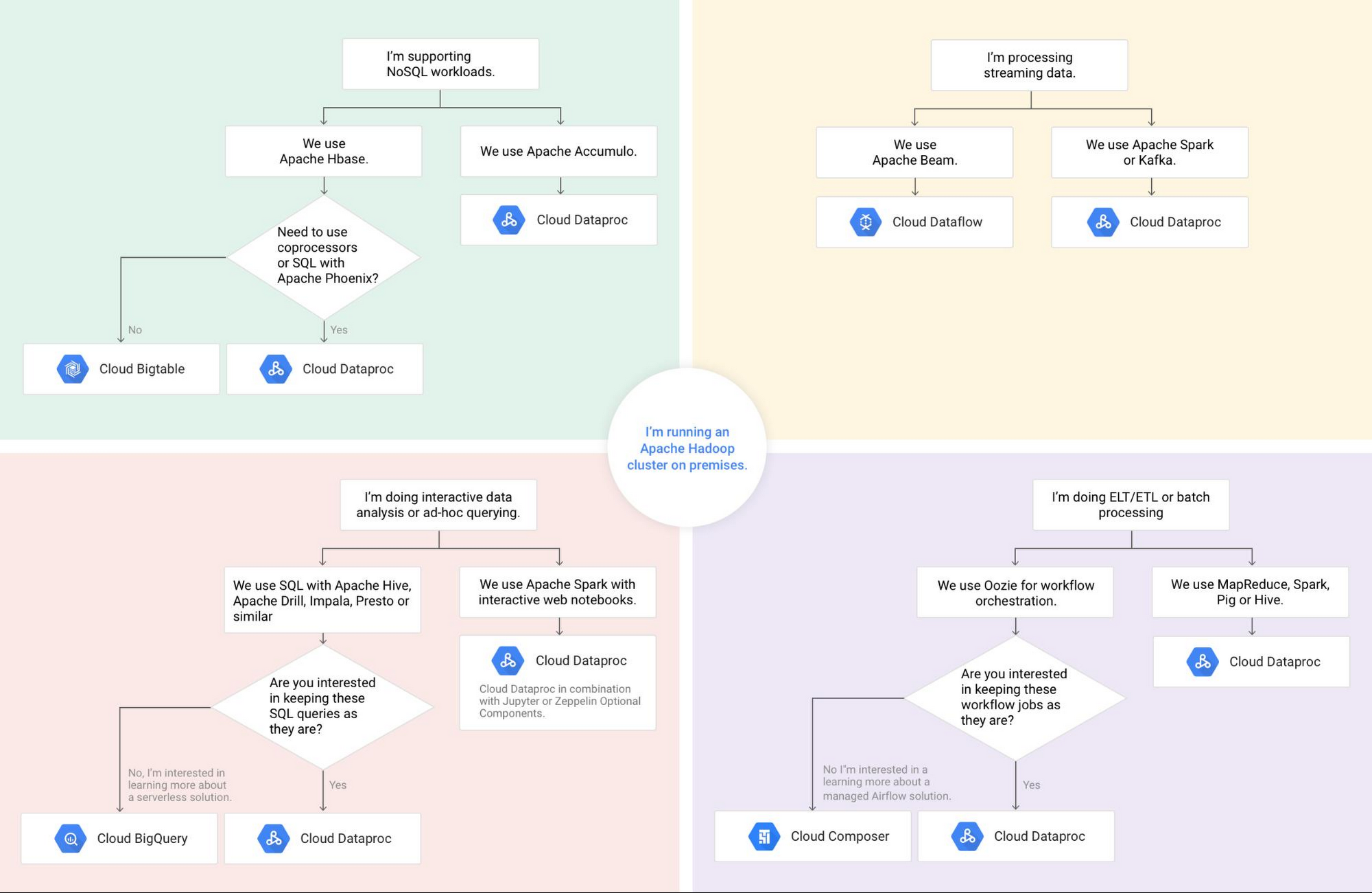
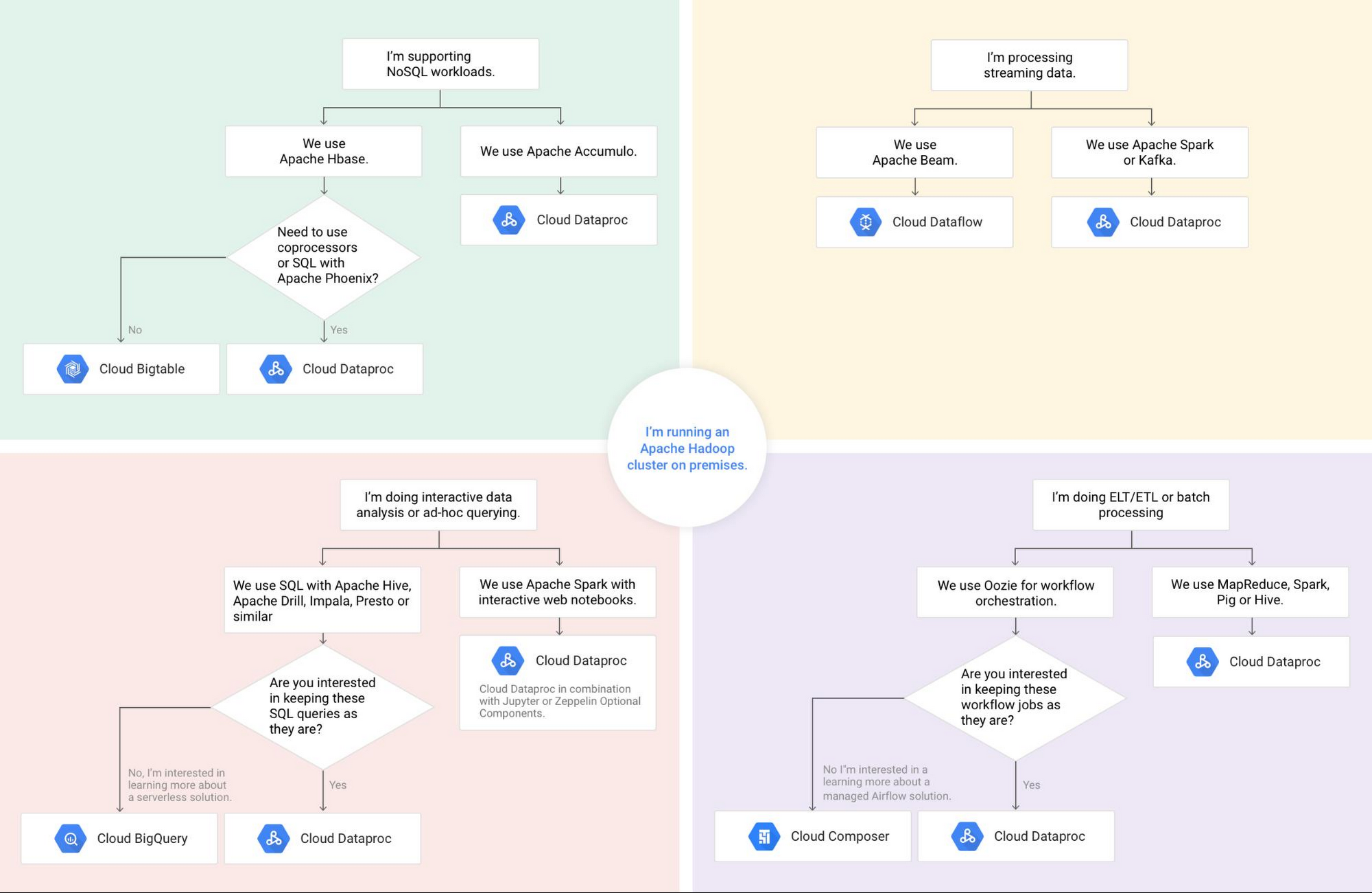
Get started with Cloud Dataproc today with a tutorial or for more information, get in touch.