画像キャプションを使用すると、画像に関連する説明を生成できます。この情報は、以下のようにさまざまな用途で使用できます。
- 保存と検索のために、画像に関するより詳細なメタデータを取得する。
- ユーザー補助のユースケースをサポートする自動字幕起こしを生成する。
- プロダクトや画像アセットについて簡単な説明を受け取る。
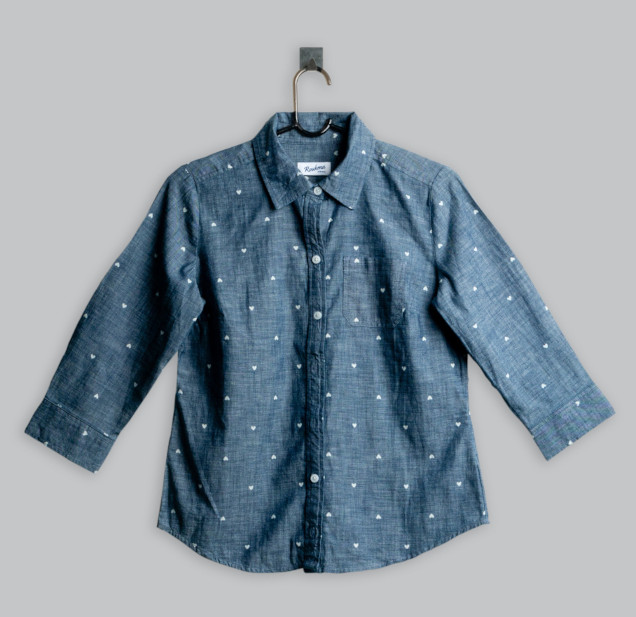
画像の出典: Unsplash の Santhosh Kumar より抜粋(切り抜き)
キャプション(短形式): 白い水玉模様の青いシャツがフックに掛けられている
サポートされている言語
画像キャプションは次の言語で利用できます。
- 英語(
en
) - フランス語(
fr
) - ドイツ語(
de
) - イタリア語(
it
) - スペイン語(
es
)
パフォーマンスと制限事項
このモデルを使用するときは次の上限が適用されます。
制限事項 | 値 |
---|---|
各プロジェクト 1 分あたりの最大 API リクエスト数(短形式) | 500 |
レスポンスで返されるトークンの最大数(短形式) | 64 トークン |
リクエストで受け入れられるトークンの最大数(VQA の短形式のみ) | 80 トークン |
このモデルを使用する場合は、次のサービス レイテンシの見積もりが適用されます。これらの値は例示を目的としたものであり、サービスを約束するものではありません。
レイテンシ | 値 |
---|---|
API リクエスト(短形式) | 1.5 秒 |
場所
ロケーションは、安静時にデータで保存される場所を制御するためにリクエストで指定できるリージョンです。使用可能なリージョンの一覧については、Vertex AI の生成 AI のロケーションをご覧ください。
責任ある AI の安全フィルタリング
画像キャプションと Visual Question Answering(VQA)の機能モデルは、ユーザーが構成可能な安全フィルタをサポートしていません。ただし、Imagen の全体的な安全フィルタリングは、次のデータに対して行われます。
- ユーザー入力
- モデル出力
その結果、Imagen がこれらの安全フィルタを適用すると、出力がサンプル出力と異なる場合があります。以下の例を考えてみましょう。
フィルタされた入力
入力がフィルタされている場合、レスポンスは次のようになります。
{
"error": {
"code": 400,
"message": "Media reasoning failed with the following error: The response is blocked, as it may violate our policies. If you believe this is an error, please send feedback to your account team. Error Code: 63429089, 72817394",
"status": "INVALID_ARGUMENT",
"details": [
{
"@type": "type.googleapis.com/google.rpc.DebugInfo",
"detail": "[ORIGINAL ERROR] generic::invalid_argument: Media reasoning failed with the following error: The response is blocked, as it may violate our policies. If you believe this is an error, please send feedback to your account team. Error Code: 63429089, 72817394 [google.rpc.error_details_ext] { message: \"Media reasoning failed with the following error: The response is blocked, as it may violate our policies. If you believe this is an error, please send feedback to your account team. Error Code: 63429089, 72817394\" }"
}
]
}
}
フィルタされた出力
返されたレスポンス数が指定したサンプル数より少ない場合は、欠落しているレスポンスが責任ある AI によってフィルタされていることを示しています。たとえば、"sampleCount": 2
を含むリクエストに対するレスポンスは次のようになりますが、レスポンスの一つは除外されます。
{
"predictions": [
"cappuccino"
]
}
すべての出力がフィルタされている場合、レスポンスは次のような空のオブジェクトになります。
{}
短い形式の画像キャプションを取得する
次のサンプルを使用して画像の短形式のキャプションを生成します。
REST
imagetext
モデル リクエストの詳細については、imagetext
モデル API リファレンスをご覧ください。
リクエストのデータを使用する前に、次のように置き換えます。
- PROJECT_ID: Google Cloud プロジェクト ID。
- LOCATION: プロジェクトのリージョン。 たとえば、
us-central1
、europe-west2
、またはasia-northeast3
です。使用可能なリージョンの一覧については、Vertex AI の 生成 AI のロケーション をご覧ください。 - B64_IMAGE: 字幕を取得する画像。画像は base64 でエンコードされたバイト文字列として指定する必要があります。サイズ制限: 10 MB。
- RESPONSE_COUNT: 生成する画像キャプションの数。指定できる整数値: 1~3。
- LANGUAGE_CODE: サポートされている言語コードのいずれか。サポートされている言語:
- 英語(
en
) - フランス語(
fr
) - ドイツ語(
de
) - イタリア語(
it
) - スペイン語(
es
)
- 英語(
HTTP メソッドと URL:
POST https://LOCATION-aiplatform.googleapis.com/v1/projects/PROJECT_ID/locations/LOCATION/publishers/google/models/imagetext:predict
リクエストの本文(JSON):
{ "instances": [ { "image": { "bytesBase64Encoded": "B64_IMAGE" } } ], "parameters": { "sampleCount": RESPONSE_COUNT, "language": "LANGUAGE_CODE" } }
リクエストを送信するには、次のいずれかのオプションを選択します。
curl
リクエスト本文を request.json
という名前のファイルに保存して、次のコマンドを実行します。
curl -X POST \
-H "Authorization: Bearer $(gcloud auth print-access-token)" \
-H "Content-Type: application/json; charset=utf-8" \
-d @request.json \
"https://LOCATION-aiplatform.googleapis.com/v1/projects/PROJECT_ID/locations/LOCATION/publishers/google/models/imagetext:predict"
PowerShell
リクエスト本文を request.json
という名前のファイルに保存して、次のコマンドを実行します。
$cred = gcloud auth print-access-token
$headers = @{ "Authorization" = "Bearer $cred" }
Invoke-WebRequest `
-Method POST `
-Headers $headers `
-ContentType: "application/json; charset=utf-8" `
-InFile request.json `
-Uri "https://LOCATION-aiplatform.googleapis.com/v1/projects/PROJECT_ID/locations/LOCATION/publishers/google/models/imagetext:predict" | Select-Object -Expand Content
"sampleCount": 2
を含むリクエストに対するものです。レスポンスは 2 つの予測文字列を返します。英語(en
):
{ "predictions": [ "a yellow mug with a sheep on it sits next to a slice of cake", "a cup of coffee with a heart shaped latte art next to a slice of cake" ], "deployedModelId": "DEPLOYED_MODEL_ID", "model": "projects/PROJECT_ID/locations/LOCATION/models/MODEL_ID", "modelDisplayName": "MODEL_DISPLAYNAME", "modelVersionId": "1" }
スペイン語(es
):
{ "predictions": [ "una taza de café junto a un plato de pastel de chocolate", "una taza de café con una forma de corazón en la espuma" ] }
Python
このサンプルを試す前に、Vertex AI クイックスタート: クライアント ライブラリの使用にある Python の設定手順を完了してください。詳細については、Vertex AI Python API のリファレンス ドキュメントをご覧ください。
Vertex AI に対する認証を行うには、アプリケーションのデフォルト認証情報を設定します。詳細については、ローカル開発環境の認証を設定するをご覧ください。
このサンプルでは、load_from_file
メソッドを使用して、字幕の取得対象となるベース Image
としてローカル ファイルを参照します。ベース画像を指定したら、ImageTextModel
で get_captions
メソッドを使用して、出力結果を表示します。
Node.js
このサンプルを試す前に、Vertex AI クイックスタート: クライアント ライブラリの使用にある Node.js の設定手順を完了してください。詳細については、Vertex AI Node.js API のリファレンス ドキュメントをご覧ください。
Vertex AI に対する認証を行うには、アプリケーションのデフォルト認証情報を設定します。詳細については、ローカル開発環境の認証を設定するをご覧ください。
このサンプルでは、PredictionServiceClient
で predict
メソッドを呼び出します。
サービスは、指定された画像の字幕を返します。
画像キャプションのパラメータを使用する
画像キャプションを取得するときに、ユースケースに応じていくつかのパラメータを設定できます。
検索結果の表示件数
[検索結果の表示件数] パラメータを使用して、送信するリクエストごとに返されるキャプションの数を制限できます。詳細については、imagetext
(画像キャプション)モデル API リファレンスをご覧ください。
シード番号
生成される説明文を確定するためリクエストに追加する数値。リクエストにシード番号を追加すると、毎回確実に同じ予測(説明文)できます。ただし、画像キャプションが同じ順序で返されるとは限りません。詳細については、imagetext
(画像キャプション)モデル API リファレンスをご覧ください。
次のステップ
Imagen や Vertex AI のその他の生成 AI プロダクトに関する次の記事を読む。
- Vertex AI で Imagen 3 を使い始めるためのデベロッパー ガイド
- クリエイターとともにクリエイターのために構築された、新しい生成メディアのモデルとツール
- Gemini の新機能: カスタム Gem と Imagen 3 による画像生成の改善
- Google DeepMind: Imagen 3 - 最も高い品質水準の Text-to-Image モデル