Separation of storage and compute in BigQuery
Tino Tereshko
Product Manager, Google BigQuery
When Google BigQuery launched in 2012, it introduced several novel service designs: a fully managed “serverless” operational model, rapidly scalable and multi-tenant compute, pay-per-job pricing, in-place data sharing, and perhaps most significantly: separation of storage and compute.
Separation of storage and compute specifically offers a wide range of benefits to BigQuery users. By decoupling these components BigQuery provides:
- Inexpensive, virtually unlimited, and seamlessly scalable storage.
- Stateless, resilient compute.
- In-place, scale-invariant data sharing.
- ACID-compliant storage operations.
- A logical database storage model, rather than physical.
This blog post unpacks the what, the how, and the why behind BigQuery’s approach to data management.
BigQuery storage under the hood
Let’s start with the lowest level. Here’s a quick recap of the four major building blocks of BigQuery:- Compute is Dremel, a large multi-tenant cluster that executes Standard SQL queries.
- Storage is Colossus, Google’s global storage system.
- Compute and storage talk to each other through the petabit Jupiter network.
- BigQuery is orchestrated via Borg, Google’s precursor to Kubernetes.
We encourage you to read “BigQuery Under the Hood,” a detailed post on this subject. Additionally, “The 12 Components of BigQuery” lays out a more complete topological map of BigQuery.
The Colossus storage subservice
The concept of “platforms” is a key principle of technical infrastructure at Google, and Colossus is the unified global platform for much of storage, from Spanner to Bigtable, from internal object stores to Cloud Storage, and even Compute Engine Persistent Disks. Colossus helps address encryption, low-level security primitives, global scale, durability, performance, hot-spotting, cross-datacenter replication, maintenance, upgrades, and so on.BigQuery leverages Colossus for most of its storage needs. This approach is hugely beneficial:
- Very fast table scans enabled by Colossus’ powerful IO throughput. It’s important to note that this throughput is invariant of levels of query concurrency. In other words, IO performance is nearly constant when you run many jobs at once.
- Seamless unlimited scaling of storage.
- Low costs of storage.
- Out-of-the-box durability using Reed-Solomon encoding
- No need to rebalance or grow storage, and no need to define restrictive sort keys or distribution keys.
- Great interoperability with other Colossus-based storage systems like Cloud Storage and Bigtable.
- Performant, ACID compliant, and deterministic physical storage metadata operations, obsoleting storage race conditions, and table locks. There’s also no such thing as a halfway-complete operation in BigQuery, and nothing to clean up when a job fails.
The Capacitor file format
BigQuery storage takes care of compression, columnar file formats, logical metadata operations, caching, buffering, and general data management.Mosha Pasumansky in April of 2016 described Capacitor, BigQuery’s next generation columnar storage format, which replaced ColumnIO (on which Parquet and ORC are based). Capacitor encodes and compresses data in more efficient ways, and heavily leverages data preprocessing and statistics about the data shape to further optimize materialization of data in Colossus. Capacitor maintains rich metadata pertaining to datasets—metadata that Dremel uses for more efficient IO, as well as to inform of better parallelization decisions.
BigQuery as the logical storage system
BigQuery storage is a logical, rather than a physical, storage system. This is a key but often misunderstood aspect of BigQuery. When you load bits into BigQuery, the service takes on the full responsibility of managing that data, and only exposing the logical database primitives to you—datasets, tables, rows, columns, cells, partitions, views, permissions, and so on.This distinction is important. By taking care of everything except the very highest layer, BigQuery can do whatever gives users the best experience possible—changing compression ratios, caching, replicating, changing encoding and data formats, and so on. The logical layer is static and deterministic, but the behaviors may improve due to changing nature of how BigQuery stores and caches your data for you.
Automated DBA tasks
Over time, BigQuery built a number of automated smarts that change the physical structure of data storage and processing, while keeping the logical layer constant. BigQuery storage has learned to self-optimize and take strong opinions about how data is managed.Examples of what these background processes do include:
- Repairing inefficient file constructions.
- Automatically fixing the many small files problem common in many Big Data systems.
- Rectifying difficult data shapes and data skew, and caching data & metadata for performance reasons.
- Define various keys to get adequate performance.
- “Watch your storage space” and delete older data.
- Spend your weekends re-distributing data within your storage system.
- Constantly monitor and tinker with your storage files to achieve maximum performance.
How BigQuery executes automatic DBA tasks
In order to understand how this is possible, we should describe Dremel. Dremel, BigQuery’s execution engine, is a multi-tenant cluster powered by millions of cores. When you run a query in Dremel, you use up to tens of thousands of cores for the duration of the query only. When you rent Dremel/BigQuery, you rent a resource limit, rather than specific hardware.This operational model gives BigQuery some interesting options. Dremel is for SQL queries, yes. However, just like with any other sensibly-operated distributed service, there are times when idle capacity is available:
- This capacity is used to service batch data ingest at no cost.
- This capacity is also used to perform the above-mentioned self-optimizing tasks. It’s as if there’s a very busy DBA running around in the background tuning your database for you.
Dynamic query execution
How aggressively should you parallelize a particular stage of the query? What about the whole query? How does this affect performance? Should this parallelization process be aware of data skew, data cardinality, or data sizes? Should we be mindful of what happens in the query downstream, like a JOIN or a window function? What about multiple queries running at once? Traditional big data technologies offer manual configurations to help answer these questions.BigQuery’s philosophy is to minimize complexity and simplify the user experience. Therefore, BigQuery doesn’t try to offer users a broad suite of execution configs and knobs. Rather, aided by metadata, Dremel’s dynamic query execution aims to automatically configure each stage of a query, being mindful of data sizes, data shapes, query complexity, and so on. BigQuery users just observe faster query execution.
In-place data sharing with BigQuery
With BigQuery, you share data within your organization in-place, using IAM and Access Control Lists. Much like with Google Docs, you simply specify which users or groups get access to your datasets, and the sharing occurs in place.One key aspect of BigQuery’s data sharing capabilities is that you don’t need to “spin up a cluster” to leverage these datasets— functionality provided by the “serverless” aspects of BigQuery. (Excuse the buzzword.)
BigQuery’s powerful sharing features are used for:
- Public Datasets (like the recently released scan of all of The MET’s objects)
- Commercial Datasets
- Marketing Datasets
- Ads Datahub
Just say no to data silos
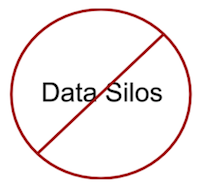
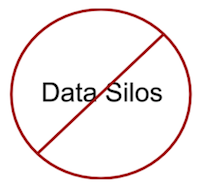
BigQuery’s data sharing model spares you from creating data silos, playing the game of telephone with data, creating multiple copies of the same data, increasing complexity and cost. Somehow, when the same report is ran on two supposedly identical data siloes, you rarely get the same results. Just say no to data silos: we mean it!
Conclusion
Over the past few years, separation of storage and compute has been broadly embraced by distributed analytics technologies. There are tradeoffs, but benefits of scale, performance, cost, ease of use, reliability, data sharing, and durability are attractive in a vast majority of use cases. However, having a separate storage system is not enough. BigQuery has continued to push the envelope by abstracting away complexity of operations typically thrust upon data engineers, automatically performing classic database administration tasks and dynamically configuring queries to maximize performance.But don’t take our word for it—we hope you arrive at the same conclusion find out on your own. The wonderful BigQuery Public Datasets program is a great jumping off point, especially with BigQuery’s free tier: you get 10GB of storage and 1TB of query every month!