Data lineage is a Dataplex feature that lets you track how data moves through your systems: where it comes from, where it is passed to, and what transformations are applied to it.
Why do you need data lineage?
Dealing with big datasets often involves transforming data into entities tailored to the needs of a specific project: text files, tables, reports, dashboards, models.
For example, imagine you have an online store where you record every purchase in a single SQL table. To make it easier for your analysts to work with the data, you start running jobs that extract information from this single table and produce smaller tables by region, brand, or sale price. Your analysts then start doing the same: they perform further transformations, merging these smaller tables with other data sources to produce even more tables.
This can become a big challenge for your stakeholders:
- Data consumers can't use a self-service tool to understand whether data comes from an authoritative source.
- Data engineers can't root cause issues due to a lack of reliable way to track all the data transformations.
- Data engineers and analysts can't fully assess possible impact before modifying or deleting tables.
- Data governors can't understand how sensitive data is used throughout the organization and ensure adherence to regulatory requirements.
Data lineage is a solution that provides a practical way to do the following:
- Understand how data is sourced and transformed with the help of lineage graphs.
- Trace errors related to entries and data operations back to their root causes.
- Enable better change management through impact analysis: avoid downtime or unexpected errors, understand dependent entries and collaborate with relevant stakeholders.
Data lineage information model
In its basic form, lineage is a record of data being transformed from sources to targets. Data Lineage API collects that information and organizes it into a hierarchical data model using the concepts of processes, runs, and events.
Process
A process is the definition of a data transformation operation supported for
a specific system. In the context of BigQuery lineage,
a process
is one of the supported job types.
Run
A run is an execution of a process. Processes can have multiple runs.
Runs contain details such as start and end times, state, or additional attributes.
For more information, see the
run
resource reference.
Event
An event represents a point in time when a data transformation operation took place and resulted in data moving between a source and a target entity.
Events contain a list of links that define which entry was the source and which was the target in a particular event. While events are used to compute lineage graphs, they are not directly exposed on the Google Cloud console. You can create, read, and delete (but not update) them using Data Lineage API.
Example
Consider the following example where data is copied between BigQuery tables:
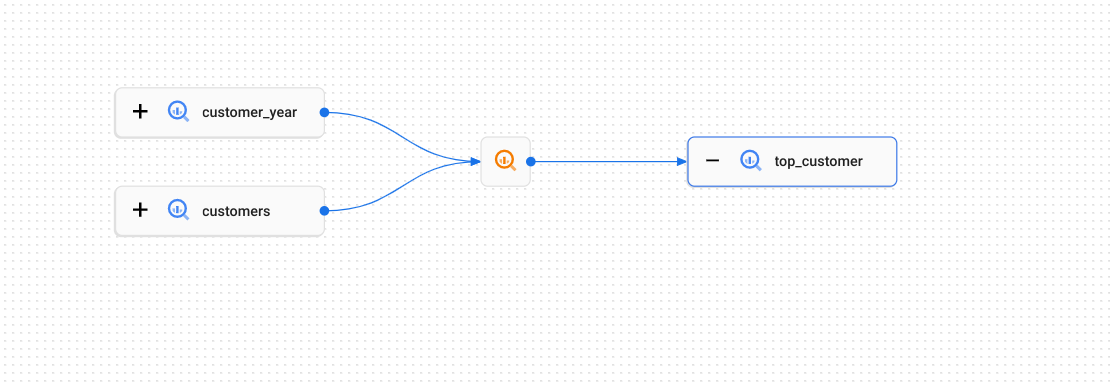
How data moves between the tables is described by the lineage process
(represented on the graph by the
icon): it could be a SQL
CREATE TABLE AS SELECT
query or an INSERT
statement.
Each execution of that SQL statement would constitute an individual run.
Runs contain events that record which tables were used as the sources and
which as the targets. In this example, the tables
customer_year
and customers
are both the source
for the target top_customer
table.
Lineage graph
Lineage graphs represent information gathered by Data Lineage API for a particular Data Catalog entry. A lineage graph shows the lineage that is upstream or downstream of a single root entry. Root refers to the entry you're viewing lineage for.

Dataplex works with the Data Lineage API to identify entries whose fully qualified name matches entities recognized by data lineage. For matched Dataplex entries, you can access the Lineage tab on their details page and view the graph.
Lineage graphs display two types of elements:
Wide, rectangular buttons that represent entities involved in constructing lineage information as sources or targets of a lineage event.
Smaller, square buttons representing processes responsible for creating or updating the source or target entities. The process buttons use icons specific to the source system that reported them to Data Lineage API. For example, BigQuery jobs use the
icon.
Lineage path visualization
Lineage path visualizations help you to understand the lineage links between two selected resources. (Contrast this with the lineage graph, which shows the lineage that is upstream or downstream of a single root entry, potentially for multiple sources or targets.)
You choose the root resource and a target resource, and the Google Cloud console displays the lineage links between the two resources. Other resources and processes that don't lay on a path between the two resources are hidden from the path visualization.
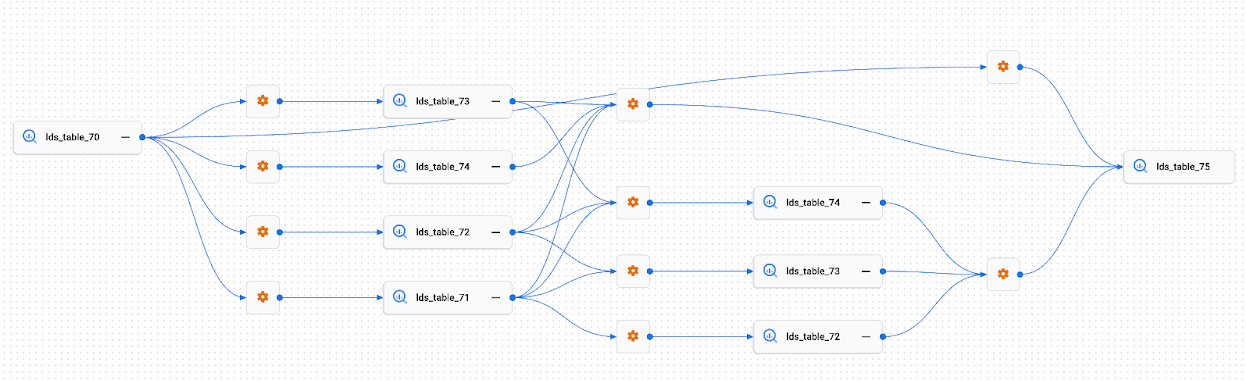
Lineage list view
The lineage list view displays detailed lineage information for entities in a single table.
Compared to the lineage graph, which is better for viewing relatively small lineage graphs, the lineage list view lets you view lineage information for entities with many connections.
The following image shows an example of the lineage list view in the Google Cloud console. The list that follows describes the image in more detail.
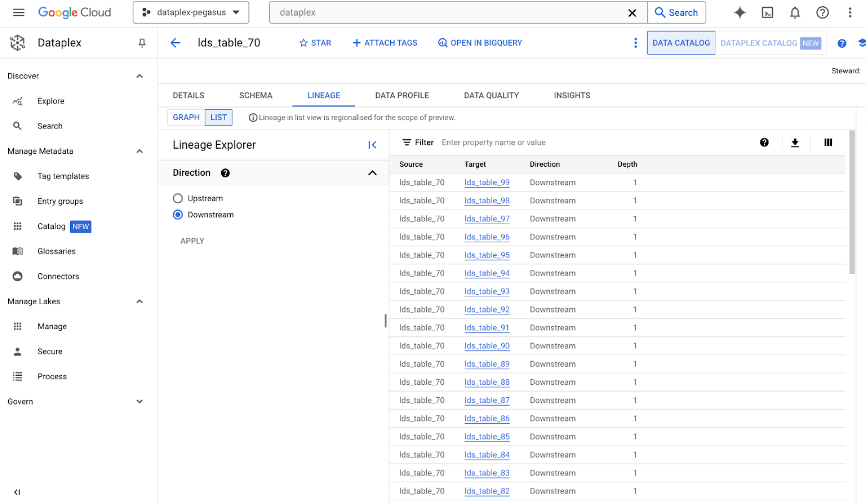
Each row in the table represents a single lineage link between two entries. In the graph, these names are portrayed as the lineage links between two entries, including any process nodes in between. For example,
Source
andTarget
are asset nodes, with possibly multiple process nodes in between.The Direction option specifies the part of the data flow to display in the list, in relation to the root asset:
Upstream: displays lineage information for entries that are data sources for the selected entry. On the lineage graph, these entries are the entries that appear to the left of the selected entry.
Downstream: displays lineage information for entries that use or are derived from the selected entry. On the lineage graph, these entries are the entries that appear to the right of the selected entry.
The Time Range option lets you filter lineage information based on the time that the lineage occurred:
Start Time: displays lineage that occurred after the start time.
End Time: displays lineage that occurred before the end time.
Depth refers to how far removed from the root resource, a source or derivative resource is. The list view displays up to 1,000 lineage links, with the maximum depth from the root as 10 lineage links. If there is any lineage outside this range, you are notified. You can see lineage outside this range by selecting the name of a different entity in the list view.
The Details panel shows information for the source of the link, the target of the link, and for all processes that created this link.
You can customize the columns that are displayed in the table and filter the results. You can also export the results to a CSV file.
Automated data lineage tracking
When you enable Data Lineage API, Google Cloud systems that support data lineage start reporting their data movement. Each integrated system can submit lineage information for a different range of data sources. For more information on every supported product, see the following sections.
BigQuery
Enabling data lineage in your BigQuery project causes Dataplex to automatically record lineage information for:
New tables as a result of the following BigQuery jobs:
- Copy jobs
- Load jobs that use the Cloud Storage URI to load data in any allowed format from Cloud Storage*
- Query jobs that use the following data definition language (DDL) in GoogleSQL:
Existing tables as a result of using following data manipulation language (DML) statements in GoogleSQL:
- SELECT in relation to any of the listed table types:
- INSERT SELECT
- MERGE
- UPDATE
- DELETE
BigQuery copy, query, and load jobs are represented
as processes. To view the process details,
on the lineage graph, click
.
Each process contains the BigQuery job_id
in the
attributes
list for the most recent BigQuery job.
Other services
Data lineage supports integration with the following Google Cloud services:
Data lineage for custom data sources
You can use the Data Lineage API in Dataplex to record lineage information manually for any data source that isn't supported by the integrated systems.
Dataplex can create lineage graphs for manually recorded
lineage if you use a
fullyQualifiedNames
that match the fully
qualified names of existing Data Catalog entries. If you want to record
lineage for a custom data source, first create a
custom Data Catalog entry.
Each process for custom data source may contain sql
key in the attributes
list. The value of such key will be used to render code highlight in details
panel of the data lineage graph. SQL statement will be displayed as it was
provided. The user is responsible for filtering out sensitive information. The
key name sql
is case-sensitive.
OpenLineage
If you're already using OpenLineage to collect lineage information from other data sources, you can import OpenLineage events into Dataplex and display these events in the Google Cloud console. For details, see Integrate with OpenLineage.
Limitations
- All lineage information is retained in the system for 30 days only.
- Lineage information persists after you remove its related data source. That is, if you remove a BigQuery table and its Data Catalog entry, you can still read the lineage for that table using the API for up to 30 days.
Access data lineage
You can access data lineage functionalities using the following:
- Entry details page in the Dataplex web interface in the Google Cloud console. See View lineage graphs.
- Table details page in the BigQuery web interface in the Google Cloud console. See View lineage graphs.
- Dataset and Model Registry pages in the Vertex AI web interface in the Google Cloud console. See View lineage graphs.
- Data Lineage API
Pricing
Dataplex uses the premium processing SKU to charge for data lineage. For more information, see Pricing.
To separate data lineage charges from other charges in the Dataplex premium processing SKU, on the Cloud Billing report, use the label
goog-dataplex-workload-type
with valueLINEAGE
.If you call the Data Lineage API
Origin
sourceType
with a value other thanCUSTOM
, it causes additional costs.
What's next
Learn to track data lineage for a BigQuery table copy and query jobs.
For administrative information, see updated IAM sections, lineage considerations, and data lineage audit logging.