일반화는 특징적인 값을 취하여 더 일반적이고 덜 특징적인 값으로 추상화하는 프로세스입니다. 일반화는 데이터의 식별 가능성을 낮추면서 데이터의 유용성을 그대로 보존하기 위한 활동입니다.
데이터 유형에 따라 여러 일반화 레벨이 있습니다. 민감한 정보 보호의 위험 분석에 포함된 것과 같은 기술을 사용하여 데이터 세트 간에 또는 실제 세계의 모집단에서 일반화가 필요한 정도를 측정할 수 있습니다.
민감한 정보 보호에서 지원하는 일반적인 일반화 기술 중 하나는 버케팅입니다. 버케팅에서는 공격자가 민감한 정보를 식별 정보와 연결할 위험을 최소화하기 위해 레코드를 더 작은 버킷으로 그룹화합니다. 이렇게 하면 의미와 유용성을 보존하는 동시에 참여자 수가 지나치게 적은 개별 값을 모호화할 수 있습니다.
버케팅 시나리오 1
숫자 버케팅 시나리오를 살펴보겠습니다. 데이터베이스에 0부터 100까지의 사용자 만족도 점수가 저장되어 있습니다. 데이터베이스는 다음과 비슷합니다.
user_id |
score |
1 |
100 |
2 |
100 |
3 |
92 |
... |
... |
데이터를 스캔하다가 일부 값은 극히 소수의 사용자에 의해 사용된다는 점을 발견합니다.
실제로 몇 개의 값은 한 명의 사용자와 연결할 수 있습니다. 예를 들어 대다수 사용자는 0, 25, 50, 75, 100을 선택했습니다. 그런데 5명의 사용자가 95를 선택했고, 단 한 명의 사용자는 92를 선택했습니다. 이 경우 원시 데이터 그대로 두는 대신 이러한 값을 그룹으로 일반화하고 참여자 수가 지나치게 적은 그룹을 제거할 수 있습니다. 이 방법으로 데이터를 일반화하면 데이터가 사용되는 방법에 따라 재식별을 차단하는 데 도움이 될 수 있습니다.
이러한 이상점 데이터 행을 제거하는 방법이 있고, 버케팅을 사용하여 유용성이 보존되도록 시도할 수도 있습니다. 이 예시에서는 다음 기준에 따라 값을 버케팅하겠습니다.
- 0-25: '낮음'
- 26-75: '중간'
- 76-100: '높음'
민감한 정보 보호의 버케팅은 익명화에 사용할 수 있는 많은 기본 변환 중 하나입니다. 다음 JSON 구성은 DLP API에서 이 버케팅 시나리오를 구현하는 방법을 보여줍니다. 이 JSON은 content.deidentify
메서드에 대한 요청에 포함될 수 있습니다.
Go
Sensitive Data Protection의 클라이언트 라이브러리를 설치하고 사용하는 방법은 Sensitive Data Protection 클라이언트 라이브러리를 참조하세요.
Sensitive Data Protection에 인증하려면 애플리케이션 기본 사용자 인증 정보를 설정합니다.
자세한 내용은 로컬 개발 환경의 인증 설정을 참조하세요.
import (
"context"
"fmt"
"io"
dlp "cloud.google.com/go/dlp/apiv2"
"cloud.google.com/go/dlp/apiv2/dlppb"
)
// deIdentifyTablePrimitiveBucketing bucket sensitive data by grouping numerical values into
// predefined ranges to generalize and protect user information.
func deIdentifyTablePrimitiveBucketing(w io.Writer, projectID string) error {
// projectId := "your-project-id"
row1 := &dlppb.Table_Row{
Values: []*dlppb.Value{
{Type: &dlppb.Value_StringValue{StringValue: "22"}},
{Type: &dlppb.Value_StringValue{StringValue: "Jane Austen"}},
{Type: &dlppb.Value_StringValue{StringValue: "21"}},
},
}
row2 := &dlppb.Table_Row{
Values: []*dlppb.Value{
{Type: &dlppb.Value_StringValue{StringValue: "101"}},
{Type: &dlppb.Value_StringValue{StringValue: "Charles Dickens"}},
{Type: &dlppb.Value_StringValue{StringValue: "95"}},
},
}
row3 := &dlppb.Table_Row{
Values: []*dlppb.Value{
{Type: &dlppb.Value_StringValue{StringValue: "55"}},
{Type: &dlppb.Value_StringValue{StringValue: "Mark Twain"}},
{Type: &dlppb.Value_StringValue{StringValue: "75"}},
},
}
tableToDeidentify := &dlppb.Table{
Headers: []*dlppb.FieldId{
{Name: "AGE"},
{Name: "PATIENT"},
{Name: "HAPPINESS SCORE"},
},
Rows: []*dlppb.Table_Row{
{Values: row1.Values},
{Values: row2.Values},
{Values: row3.Values},
},
}
ctx := context.Background()
// Initialize a client once and reuse it to send multiple requests. Clients
// are safe to use across goroutines. When the client is no longer needed,
// call the Close method to cleanup its resources.
client, err := dlp.NewClient(ctx)
if err != nil {
return err
}
// Closing the client safely cleans up background resources.
defer client.Close()
// Specify what content you want the service to de-identify.
contentItem := &dlppb.ContentItem{
DataItem: &dlppb.ContentItem_Table{
Table: tableToDeidentify,
},
}
// Specify how the content should be de-identified.
buckets := []*dlppb.BucketingConfig_Bucket{
{
Min: &dlppb.Value{
Type: &dlppb.Value_IntegerValue{
IntegerValue: 0,
},
},
Max: &dlppb.Value{
Type: &dlppb.Value_IntegerValue{
IntegerValue: 25,
},
},
ReplacementValue: &dlppb.Value{
Type: &dlppb.Value_StringValue{
StringValue: "low",
},
},
},
{
Min: &dlppb.Value{
Type: &dlppb.Value_IntegerValue{
IntegerValue: 25,
},
},
Max: &dlppb.Value{
Type: &dlppb.Value_IntegerValue{
IntegerValue: 75,
},
},
ReplacementValue: &dlppb.Value{
Type: &dlppb.Value_StringValue{
StringValue: "Medium",
},
},
},
{
Min: &dlppb.Value{
Type: &dlppb.Value_IntegerValue{
IntegerValue: 75,
},
},
Max: &dlppb.Value{
Type: &dlppb.Value_IntegerValue{
IntegerValue: 100,
},
},
ReplacementValue: &dlppb.Value{
Type: &dlppb.Value_StringValue{
StringValue: "High",
},
},
},
}
// Specify the BucketingConfig in primitive transformation.
primitiveTransformation := &dlppb.PrimitiveTransformation_BucketingConfig{
BucketingConfig: &dlppb.BucketingConfig{
Buckets: buckets,
},
}
// Specify the field of the table to be de-identified
feildId := &dlppb.FieldId{
Name: "HAPPINESS SCORE",
}
// Specify the field transformation which apply to input field(s) on which you want to transform.
fieldTransformation := &dlppb.FieldTransformation{
Transformation: &dlppb.FieldTransformation_PrimitiveTransformation{
PrimitiveTransformation: &dlppb.PrimitiveTransformation{
Transformation: primitiveTransformation,
},
},
Fields: []*dlppb.FieldId{
feildId,
},
}
// Specify the record transformation to transform the record by applying various field transformations
transformation := &dlppb.RecordTransformations{
FieldTransformations: []*dlppb.FieldTransformation{
fieldTransformation,
},
}
// Specify the deidentification config.
deidentifyConfig := &dlppb.DeidentifyConfig{
Transformation: &dlppb.DeidentifyConfig_RecordTransformations{
RecordTransformations: transformation,
},
}
// Construct the de-identification request to be sent by the client.
req := &dlppb.DeidentifyContentRequest{
Parent: fmt.Sprintf("projects/%s/locations/global", projectID),
DeidentifyConfig: deidentifyConfig,
Item: contentItem,
}
// Send the request.
resp, err := client.DeidentifyContent(ctx, req)
if err != nil {
return err
}
// Print the results.
fmt.Fprintf(w, "Table after de-identification : %v", resp.GetItem().GetTable())
return nil
}
Node.js
Sensitive Data Protection의 클라이언트 라이브러리를 설치하고 사용하는 방법은 Sensitive Data Protection 클라이언트 라이브러리를 참조하세요.
Sensitive Data Protection에 인증하려면 애플리케이션 기본 사용자 인증 정보를 설정합니다.
자세한 내용은 로컬 개발 환경의 인증 설정을 참조하세요.
// Imports the Google Cloud client library
const DLP = require('@google-cloud/dlp');
// Instantiates a client
const dlp = new DLP.DlpServiceClient();
// The project ID to run the API call under
// const projectId = 'your-project-id';
// Construct the tabular data
const tablularData = {
headers: [{name: 'AGE'}, {name: 'PATIENT'}, {name: 'HAPPINESS SCORE'}],
rows: [
{
values: [
{stringValue: '101'},
{stringValue: 'Charles Dickens'},
{integerValue: 95},
],
},
{
values: [
{stringValue: '22'},
{stringValue: 'Jane Austen'},
{integerValue: 21},
],
},
{
values: [
{stringValue: '55'},
{stringValue: 'Mark Twain'},
{integerValue: 75},
],
},
],
};
async function deIdentifyTableBucketing() {
// Construct bucket confiugrations
const buckets = [
{
min: {integerValue: 0},
max: {integerValue: 25},
replacementValue: {stringValue: 'Low'},
},
{
min: {integerValue: 25},
max: {integerValue: 75},
replacementValue: {stringValue: 'Medium'},
},
{
min: {integerValue: 75},
max: {integerValue: 100},
replacementValue: {stringValue: 'High'},
},
];
const bucketingConfig = {
buckets: buckets,
};
// The list of fields to be transformed.
const fieldIds = [{name: 'HAPPINESS SCORE'}];
// Associate fields with bucketing configuration.
const fieldTransformations = [
{
primitiveTransformation: {bucketingConfig: bucketingConfig},
fields: fieldIds,
},
];
// Specify de-identify configuration using transformation object.
const deidentifyConfig = {
recordTransformations: {
fieldTransformations: fieldTransformations,
},
};
// Combine configurations into a request for the service.
const request = {
parent: `projects/${projectId}/locations/global`,
deidentifyConfig: deidentifyConfig,
item: {
table: tablularData,
},
};
// Send the request and receive response from the service.
const [response] = await dlp.deidentifyContent(request);
// Print the results.
console.log(
`Table after de-identification: ${JSON.stringify(
response.item.table,
null,
2
)}`
);
}
deIdentifyTableBucketing();
REST
...
{
"primitiveTransformation":
{
"bucketingConfig":
{
"buckets":
[
{
"min":
{
"integerValue": "0"
},
"max":
{
"integerValue": "25"
},
"replacementValue":
{
"stringValue": "Low"
}
},
{
"min":
{
"integerValue": "26"
},
"max":
{
"integerValue": "75"
},
"replacementValue":
{
"stringValue": "Medium"
}
},
{
"min":
{
"integerValue": "76"
},
"max":
{
"integerValue": "100"
},
"replacementValue":
{
"stringValue": "High"
}
}
]
}
}
}
...
버케팅 시나리오 2
문자열 또는 열거된 값에도 버케팅을 사용할 수 있습니다. 직책을 포함하여 급여 데이터를 공유하려는 경우를 가정해 보겠습니다. 그런데 CEO 또는 특별 엔지니어와 같은 일부 직책은 한 사람 또는 소수의 그룹과 연결할 수 있습니다. 이러한 직책은 그 직책을 보유한 직원과 손쉽게 연결됩니다.
이 경우에도 버케팅이 유용할 수 있습니다. 정확한 직책을 포함하는 대신 일반화 및 버케팅합니다. 예를 들어 '선임 엔지니어', '후임 엔지니어', '특별 엔지니어'는 일반화를 통해 단순히 '엔지니어'로 버케팅됩니다. 다음 표에서 특정 직책을 직책군으로 버케팅하는 방법을 볼 수 있습니다.
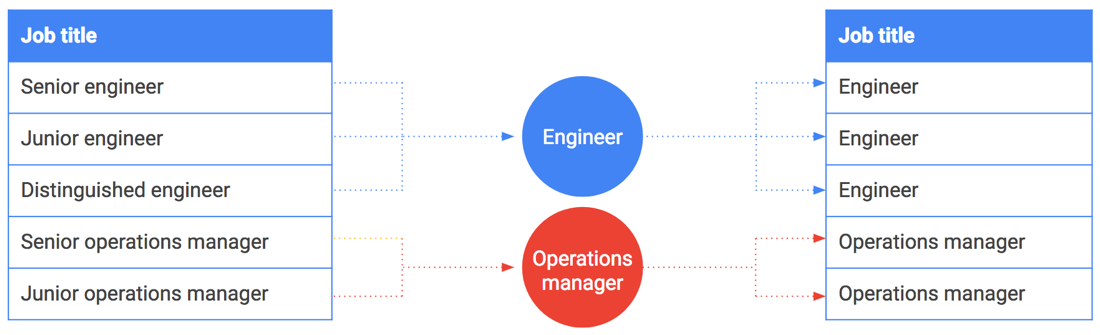
기타 시나리오
이러한 예에서는 구조화된 데이터에 변환을 적용했습니다. 사전 정의된 또는 커스텀 infoType으로 값을 분류할 수 있다면 구조화되지 않은 예에도 버케팅을 사용할 수 있습니다. 아래는 몇 가지 시나리오 예입니다.
- 날짜를 분류하고 연도 범위로 버케팅합니다.
- 이름을 분류하고 첫 문자(A-M, N-Z)를 기준으로 그룹으로 버케팅합니다.
리소스
일반화 및 버케팅에 대한 자세한 내용은 텍스트 콘텐츠의 민감한 정보 익명화를 참조하세요.
API 문서는 다음을 참조하세요.