Choose models and infrastructure for your generative AI application
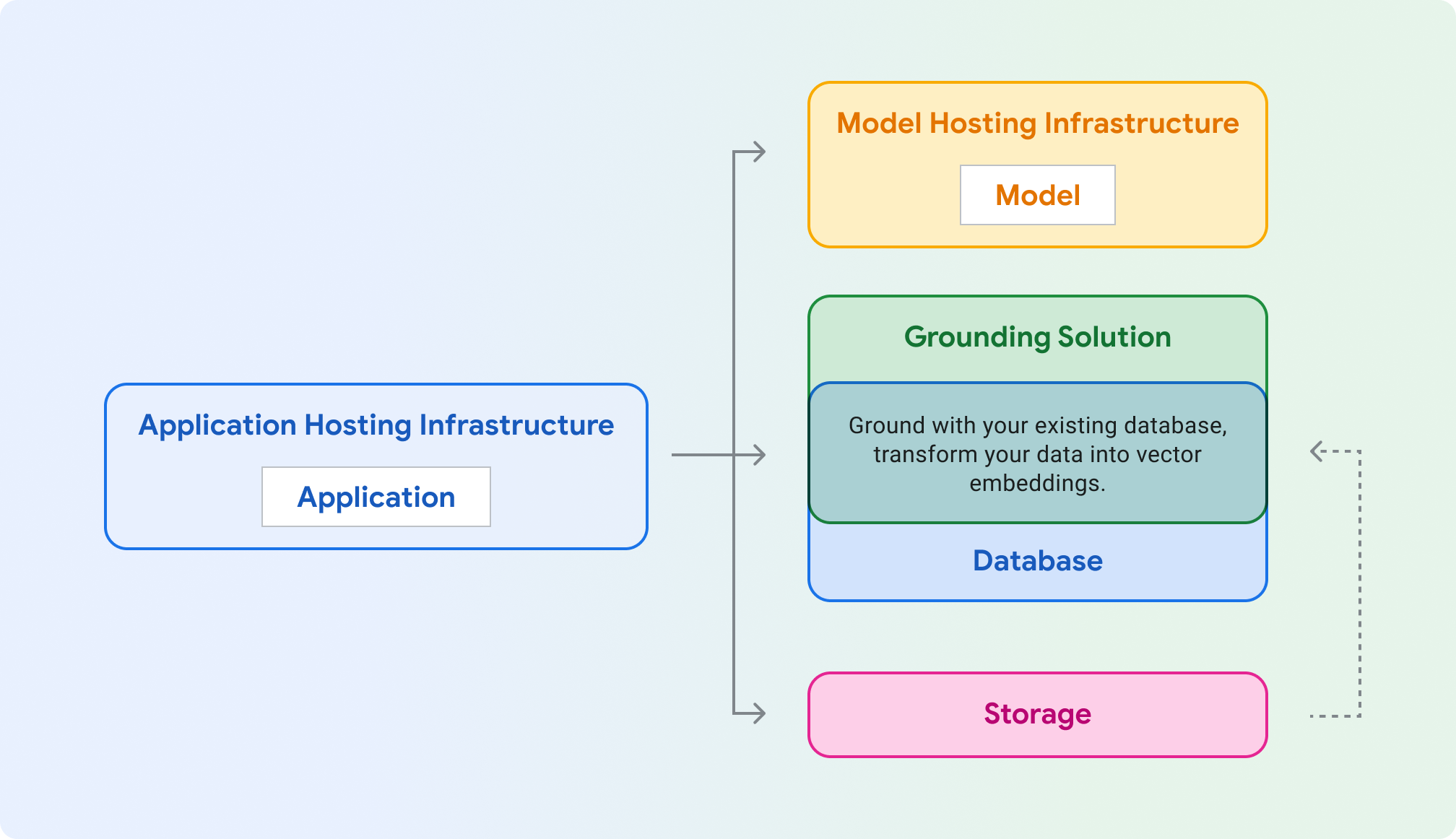
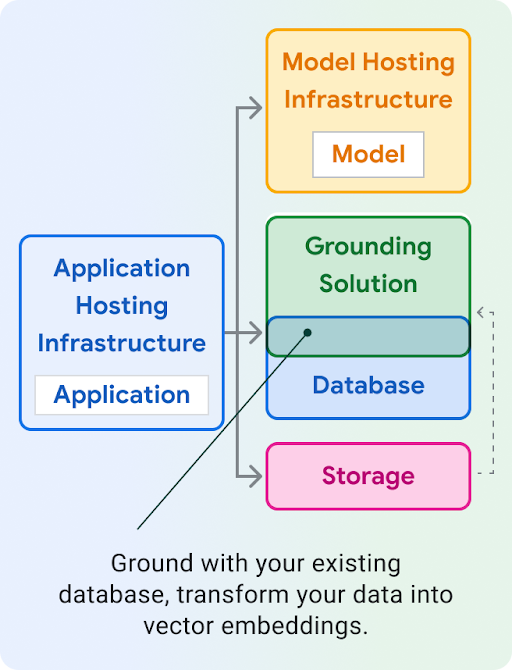
Application hosting: Compute to host your application. Your application can use Google Cloud's client libraries and SDKs to talk to different Cloud products.
Model hosting: Scalable and secure hosting for a generative model.
Model: Generative model for text, chat, images, code, embeddings, and multimodal.
Grounding solution: Anchor model output to verifiable, updated sources of information.
Database: Store your application's data. You might reuse your existing database as your grounding solution, by augmenting prompts via SQL query, and/or storing your data as vector embeddings using an extension like pgvector.
Storage: Store files such as images, videos, or static web frontends. You might also use Storage for the raw grounding data (eg. PDFs) that you later convert into embeddings and store in a vector database.
The sections below walk through each of those components, helping you choose which Google Cloud products to try.
Application hosting infrastructure
Choose a product to host and serve your application workload, which makes calls out to the generative model.
Model hosting infrastructure
Google Cloud provides multiple ways to host a generative model, from the flagship Vertex AI platform, to customizable and portable hosting on Google Kubernetes Engine.
Using Gemini and need enterprise features like scaling, security, data privacy, and observability
Want fully managed infrastructure, with first-class generative AI tools and APIs?
Does your model require a specialized kernel, legacy OS, or have special licensing terms?
Model
Google Cloud provides a set of state-of-the-art foundation models through Vertex AI , including Gemini. You can also deploy a third-party model to either Vertex AI Model Garden or self-host on GKE , Cloud Run, or Compute Engine.
Generating embeddings for search, classification, or clustering?
Ok, you want to generate text. Would you like to include images or video in your text prompts? (multi-modal)
Ok, just text prompts. Want to leverage Google's most capable flagship model?
Deploy an open-source model to: Vertex AI (Model Garden) GKE (HuggingFace)
Grounding and RAG
To ensure informed and accurate model responses, ground your generative AI application with real-time data. This is called retrieval-augmented generation (RAG).
If you want to generate content that’s grounded on up-to-date information from the internet, then Gemini models can evaluate whether the model's knowledge is sufficient or whether grounding with Google Search is required.
You can implement grounding using an index of your data with a search engine. Many search engines now store embeddings in a vector database, which is an optimal format for operations like similarity search. Google Cloud offers multiple vector database solutions, for different use cases.
Note: You can ground using non-vector databases by querying an existing database like Cloud SQL or Firestore, and you can use the result of the query in your model prompt.
Do you want a fully-managed optimized solution that supports most data sources and prevents direct access to the underlying embeddings?
Do you want to build a search engine for RAG using a managed orchestrator with a LlamaIndex-like interface?
Do you need a low-latency vector search, large-scale serving, or a specialized and optimized vector database?
Is your data accessed programmatically (OLTP)? Already using a SQL database?
Want to use Google AI models directly from your database? Require low latency?
Have a large analytical dataset (OLAP)? Require batch processing, and frequent SQL table access by humans or scripts (data science)?
Grounding with APIs
Vertex AI Extensions (Private Preview)
LangChain Components
Grounding in Vertex AI
Start building
Set up your development environment for Google Cloud
Set up LangChain
LangChain is an open source framework for generative AI apps that allows you to build context into your prompts, and take action based on the model's response.
View code samples and deploy sample applications
View code samples for popular use cases and deploy examples of generative AI applications that are secure, efficient, resilient, high-performing, and cost-effective.