From turnkey to custom: Tailor your AI risk governance to help build confidence
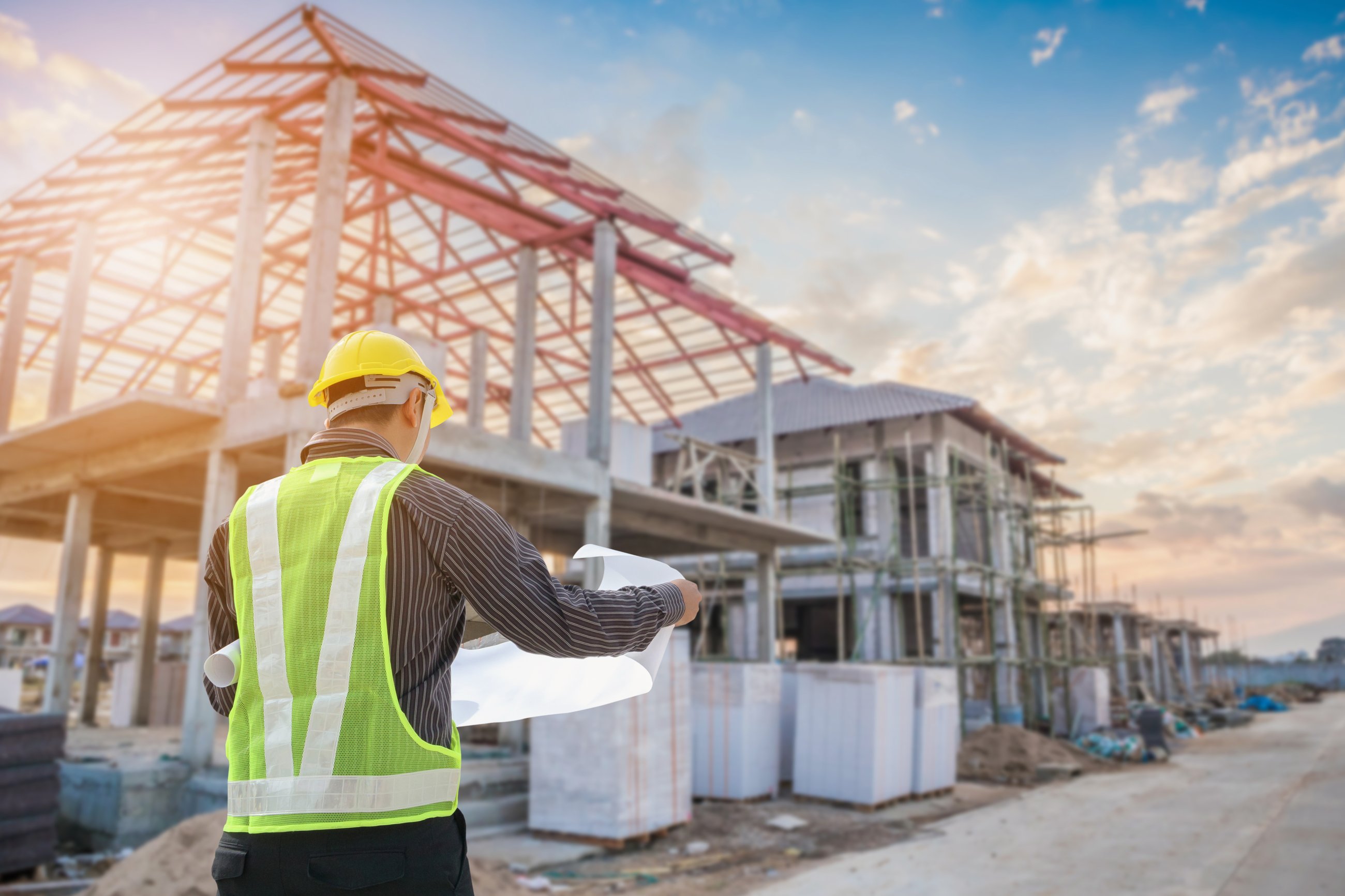
Mark Schadler
Tech Lead for Compliance and Security, Cloud AI
Tanya Popova-Jones
Head of Trusted Cloud Services, Office of the CISO
Almost everything you wanted to know about AI risk mitigation (but were afraid to ask)
Hear monthly from our Cloud CISO in your inbox
Get the latest on security from Cloud CISO Phil Venables.
SubscribeIn barely less than a year, generative AI has grown from a technological curiosity to a developmental force that Google Cloud customers and others have only just begun to explore. As is appropriate for any emergent technology, many business and security leaders want to know how generative AI models affect their risk-management strategies.
At Google Cloud, we are committed to helping enterprises develop effective AI risk management strategies to be able to use the full potential of generative AI. For example, at Google Cloud Next, we made many announcements on how we’re improving security with AI to address pervasive and fundamental security challenges, and we recently clarified and expanded our terms of service to provide additional protections to customers with generative AI indemnifications.
While risk profiles are often complex, this is especially true for generative AI because of how intricate the models can be. The specific risks and mitigations depend on the business use of generative AI and the data sensitivity. An AI foundation model can generate outputs for many downstream tasks, including replying to questions, summarization, and content or code creation.
Importantly, risk management can vary depending on how the organization has chosen to use AI: Is it developing its own AI applications, using AI applications developed by a third party (including those developed by Google Cloud), or a mix of both? How enterprise ready those services are is also a factor.
While that may sound like a lot to take on for a technology still in its infancy, we have structures in place that can help guide our approaches. One of the most important is shared fate, which goes beyond shared responsibility and emphasizes the continuous partnership between cloud providers and customers to help achieve stronger security outcomes.
“As we continue to develop our AI platform, systems, and foundational models, our belief in shared fate and our experience in using these technologies guides us to invest in end-to-end governance tools, opinionated guidance, and best practices to help our customers keep their data and AI models safe,” said Phil Venables, vice president and CISO, Google Cloud, in a recent podcast.
Looking through the lens of how a customer might participate in the AI ecosystem, we see four basic scenarios that require different risk management strategies. A key difference between these four scenarios is the level of direct control an organization has over the AI model, as compared to what is outsourced to an external provider, such as Google Cloud.
Customers can rely on Google Cloud to uphold our strong AI privacy commitments and to protect customer’s data, enabling them to pursue data-rich use cases while complying with relevant regulations and laws.
We can think of the four scenarios as similar to building a house. The four scenarios are:
- Build it yourself: Similar to how house builders can design and build a house from scratch, customers can design and build their own AI models and services, using Google Cloud Vertex AI Platform.
- Customize to your needs: A scalable approach for house builders is to use existing designs and customize to their needs. Similarly, customers can adapt models from Google Cloud to their specific use case, or apply powerful domain and use-case specific services. This can rely on using customer’s proprietary data, and we can see examples of it in Anti Money Laundering AI, Vertex AI Search, and Document AI Workbench.
- Integrate as-is: Building a house either from scratch or from existing designs is not for everybody. One can simply take a ready-built house and furnish it to make it a home. Similarly, customers can integrate Google Cloud AI models or pre-built solutions without further adaptation into their custom applications, such as using Translation API as part of Translation AI and PaLM API as part of Vertex AI Model Garden.
- Consume out-of-the-box: In the house analogy, this is the turnkey, fully furnished scenario. Customers can use Google Cloud applications with fully integrated AI capabilities, as we offer with Duet AI in Chronicle and Duet AI in Google Workspace.
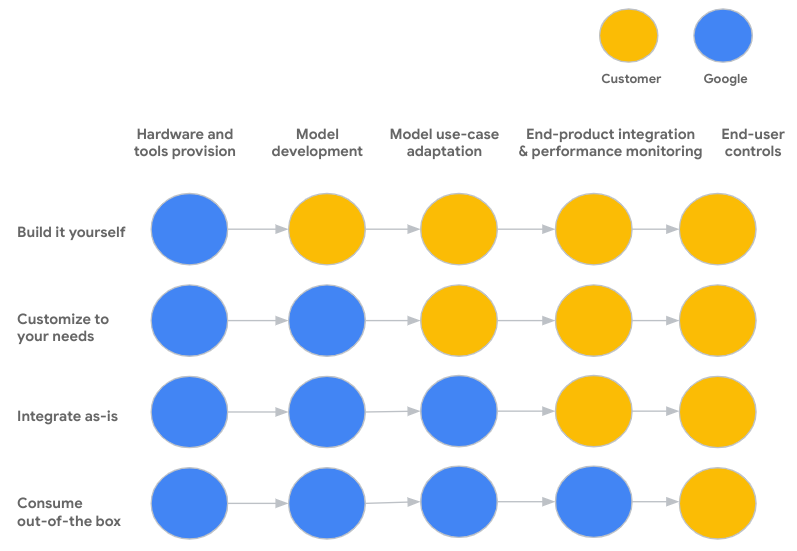
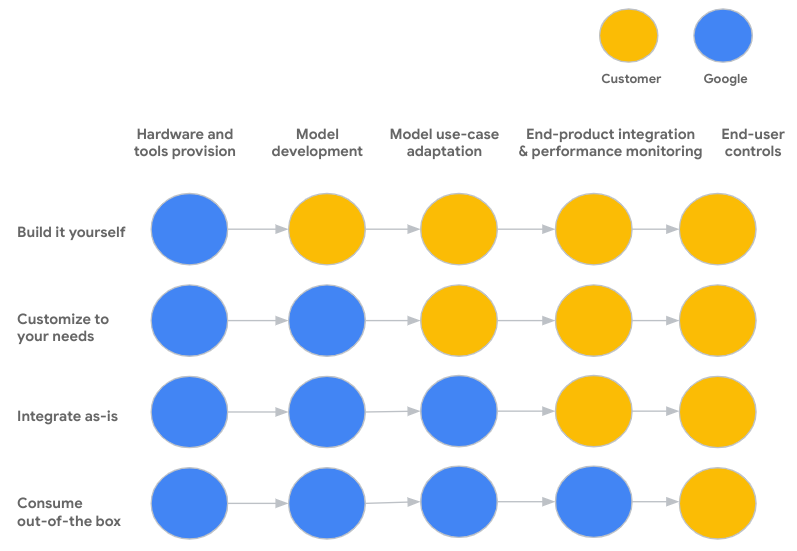
Across all four scenarios, customers can rely on Google Cloud to uphold our strong AI privacy commitments and to protect customer’s data, enabling them to pursue data-rich use cases while complying with relevant regulations and laws.
Here are some specifics of how Google Cloud supports customers to mitigate risks in each scenario.
1. Build it yourself
For experienced data scientists, machine learning engineers, and organizations solving highly custom problems, simply having access to an AI platform, such as Vertex AI, is often enough. It provides fully managed tools and access to the latest innovations including AutoML for specific uses. In this scenario, customers take control over a large portion of the end-to-end process and responsibilities as they build, train, and deploy their own AI models and services.
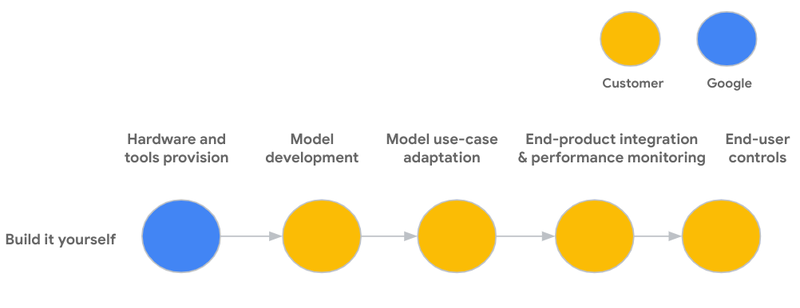
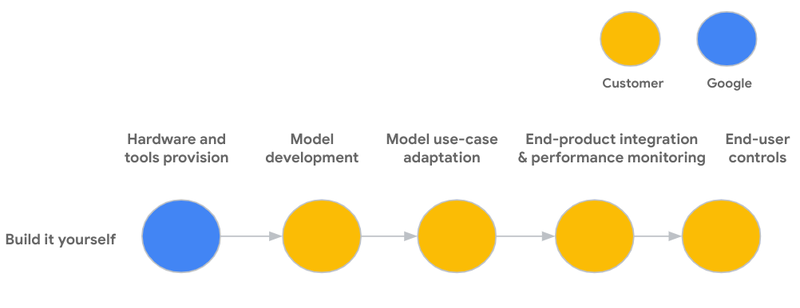
Consistent with our shared fate model, Google Cloud can help customers with tools and best practices, leveraging learnings from building our own AI solutions. For example, Google Cybersecurity Action Team can advise customers on AI security and risk management strategies.
Google Cloud customers can use our Responsible AI approach to mitigate risks from unintended or unforeseen outputs that models can produce. Vertex’s Explainable AI makes it easier to know how an AI model makes a decision. And, we are currently offering a Model Fairness tool in preview. Finally, features such as Model Evaluation, Model Monitoring, and Model Registry can all help to support data and model governance.
When it comes to security, our Secure AI Framework (SAIF) offers practical considerations organizations can implement to help mitigate risks specific to AI systems. To help protect customer data, Google Cloud offers a number of controls for mitigating the risk of data exfiltration from Vertex AI, including VPC Service Controls, customer managed encryption keys, and access transparency. Importantly, we offer security tools that use generative AI to help defend customer’s systems and help respond to and prevent cyber attacks.
2. Customize to your needs
Some organizations want to tune Google Cloud’s AI services and models with their own proprietary data to solve business problems across a wide set of domains including recommendations, search, conversation, and risk. This offers developers a scalable way to build custom business applications without starting from scratch.
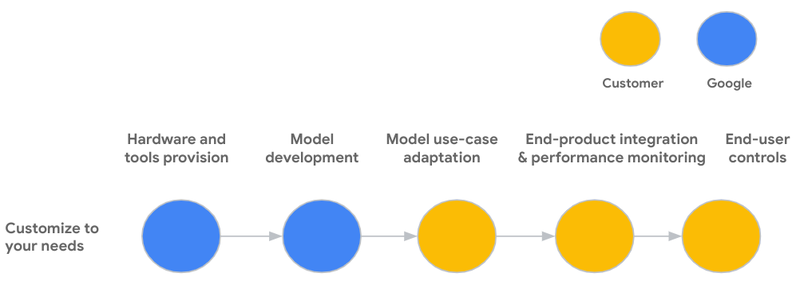
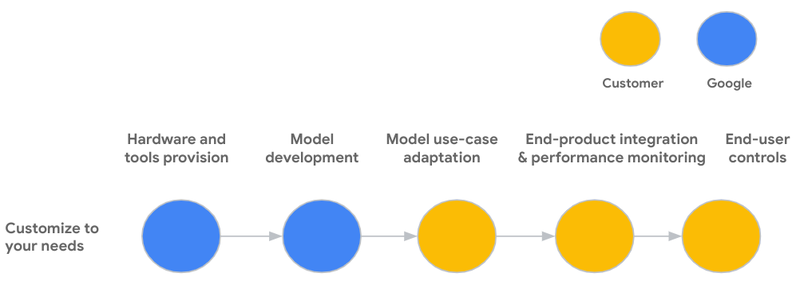
In this scenario, Google Cloud manages the AI service or foundation model development, including the associated data governance, security and compliance controls. When evaluating the end-to-end risks, customers should use Google Cloud product documentation for sample use cases, known limitations and recommended practices.
Similar to building it yourself, Google Cloud also provides tools to help customers manage the additional risks as they adapt, test, deploy, and integrate Google Cloud AI services and models into their final products. This includes data and model lineage capabilities, integrated security and identity management services, safety filters, multiple tuning options and support for third-party and openly available models.
We also recently announced an experimental version of a new tool called SynthID, in partnership with Google Deepmind. Following further testing with a limited number of customers, it’s our hope that this tool will help many institutions create AI-generated images responsibly and support image-origin identification with confidence.
Examples of additional features available for customers in the ‘Customize to your needs’ scenario include Reinforcement Learning from Human Feedback (RLHF). This enables direct feedback to customize and improve performance. Such capabilities are particularly useful in industries where accuracy is crucial, or customer satisfaction is critical, such as healthcare, finance, and e-commerce. RHLF lets humans more accurately review the model responses for bias, toxic content, and other considerations, teaching the model to avoid inappropriate outputs.
3. Integrate as-is
For developers that look to use the power of AI with minimal effort and ML expertise, using Google Cloud pre-trained models and services without any further adaptation is the way to go.
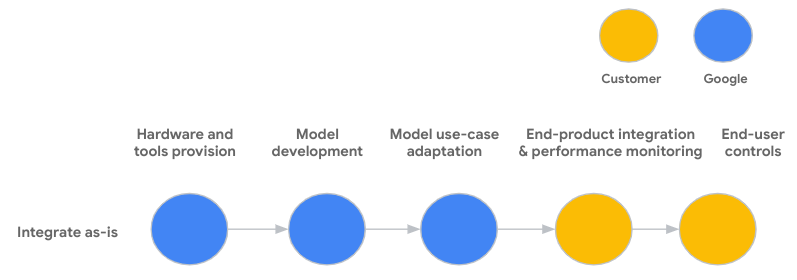
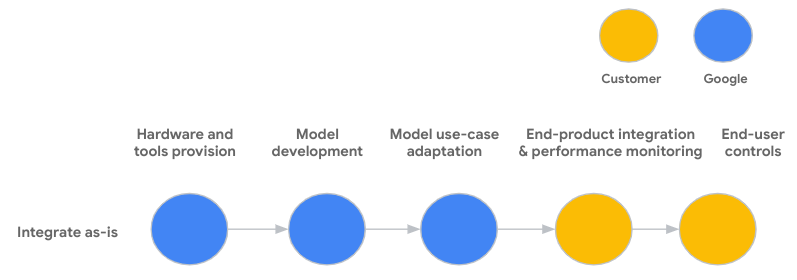
Here customers play a key role in choosing how the Google Cloud model or service is used and integrated into their business applications. For adequate risk management, it’s critical to align the intended business use with the use cases the model or service is designed for and the known limitations (outlined in the product documentation and our terms and conditions).
As before, Google Cloud can help with tools for data governance, integrated security and identity management, safety filters, etc. We also offer support for third-party and openly available models. The Secure AI Framework can help customers protect their systems and users from harm as they build the final business applications. Importantly, in conversations with customers, we recommend that anyone who uses AI must be responsible in their approach, implementing recommended best practices including conducting rigorous testing, as well as continuing to monitor and update the system after deployment.
4. Consume out-of-the-box
Finally, powerful applications with fully integrated AI capabilities offer organizations out-of-the box functionality to solve business challenges at scale by increasing productivity, boosting creativity, reducing toil, and bridging the talent gap.
For example, customers can use Duet AI in Chronicle Security Operations to search billions of security events and interact conversationally with the results, ask follow-up questions, and quickly generate detections, all without learning a new syntax or schema. With the help of AI in Workspace, customers can start writing or rewriting a doc with just a prompt, or generate original images from a few words.
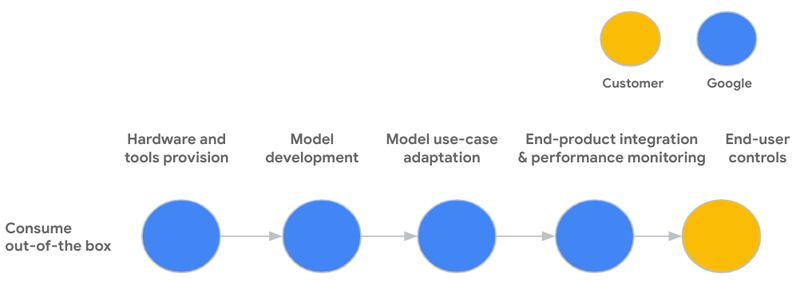
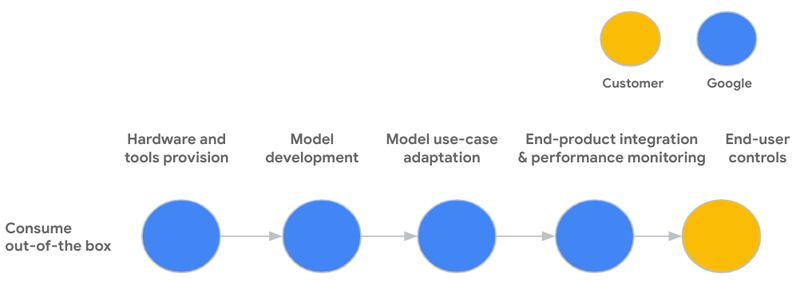
In this scenario, Google Cloud manages the entire development lifecycle. Importantly, customers remain in control and have the power to accept, decline, or edit the output that the AI-powered feature generates.
Building together, responsibly
At Google Cloud, we believe we have a responsibility to our customers to deliver best-in-class information and services in order to support your deployment of AI solutions. We aim to be at the forefront of advancing the frontier of AI to develop more capable and useful AI solutions, as well as methods that help utilize AI in a secure way.
Google Cloud customers are critical to ensuring delivery of responsible AI to every level of AI stakeholder. We also know that one size does not fit all. We are committed to working with our customers to develop cutting-edge AI solutions, tools and guidance that accommodate diverse risk appetites and governance needs, ensuring that this profoundly helpful technology works for everyone.