Built with Google AI: Achieve better observability for ML models with Fiddler AI
Krishnaram Kenthapadi
Fiddler AI
Dr. Ali Arsanjani
Director, Applied AI Engineering, Google
Artificial Intelligence (AI) is increasingly playing an integral role in determining our day-to-day experiences. The applications of AI are rapidly expanding beyond search and recommendation systems to encompass high-stakes domains such as hiring, lending, criminal justice, healthcare, and education. The potential impact of AI on individuals, businesses, and society is vast, and it is essential that AI models are designed to be accurate, reliable, and ethical. Consequently, it becomes critical to ensure that AI models are making accurate predictions, are robust to shifts in the data, are not relying on spurious features, and are not unduly discriminating against certain subgroups of users. As such, there is a critical need for ongoing research and development efforts to create reliable, robust, and ethical AI solutions that can be trusted to enhance our daily lives while minimizing potential risks.
Challenge: The need for AI observability
For data scientists and machine learning (ML) engineers, ensuring the accuracy and performance of ML models is a top priority. However, it's also essential to check for biases, spurious features, and model robustness as part of the model validation process to ensure responsible deployment of ML. Ensuring that the models continue to perform as intended after deployment is very important. A key challenge is that ML practitioners do not have easy-to-use tools for performing such checks. While there are several open source toolkits designed to address each of these issues in isolation, ML practitioners don’t have a way to easily integrate such tools into their workflows and get a coherent experience for managing their models. In other words, customers need deep tools for model validation, model monitoring, and model governance to ensure business metrics powered by AI are not impacted over time and ML is adopted responsibly, especially in regulated domains.
Solution approach: Fiddler’s AI Observability platform
Fiddler has teamed up with Google Cloud to provide customers with a unified easy-to-use single pane of glass to ensure that their models are behaving as expected and to identify the root causes behind performance degradation. Fiddler’s AI Observability platform helps MLOps teams validate models before deployment, detect issues with deployed models in a timely fashion, and thereby ensure that models continue to retain performance, comply with regulations, and satisfy responsible AI principles. By providing continuous operational visibility into ML, understanding why predictions are made, and enabling teams with actionable insights to improve their models, Fiddler can help improve ML practitioner productivity and reduce the time needed to detect and resolve issues. Top-tier banks, fintech companies, and companies from other regulated industries use Fiddler to not only get operational ML visibility but also achieve regulatory compliance with risk management of AI/ML.
Fiddler AI + Google Cloud
Fiddler serves as a single pane of glass for AI/ML practitioners to monitor, explain, and analyze AI models so that customers deploying AI on Google Vertex AI and other Google Cloud ML offerings could leverage Fiddler to build trustworthy and responsible AI applications.
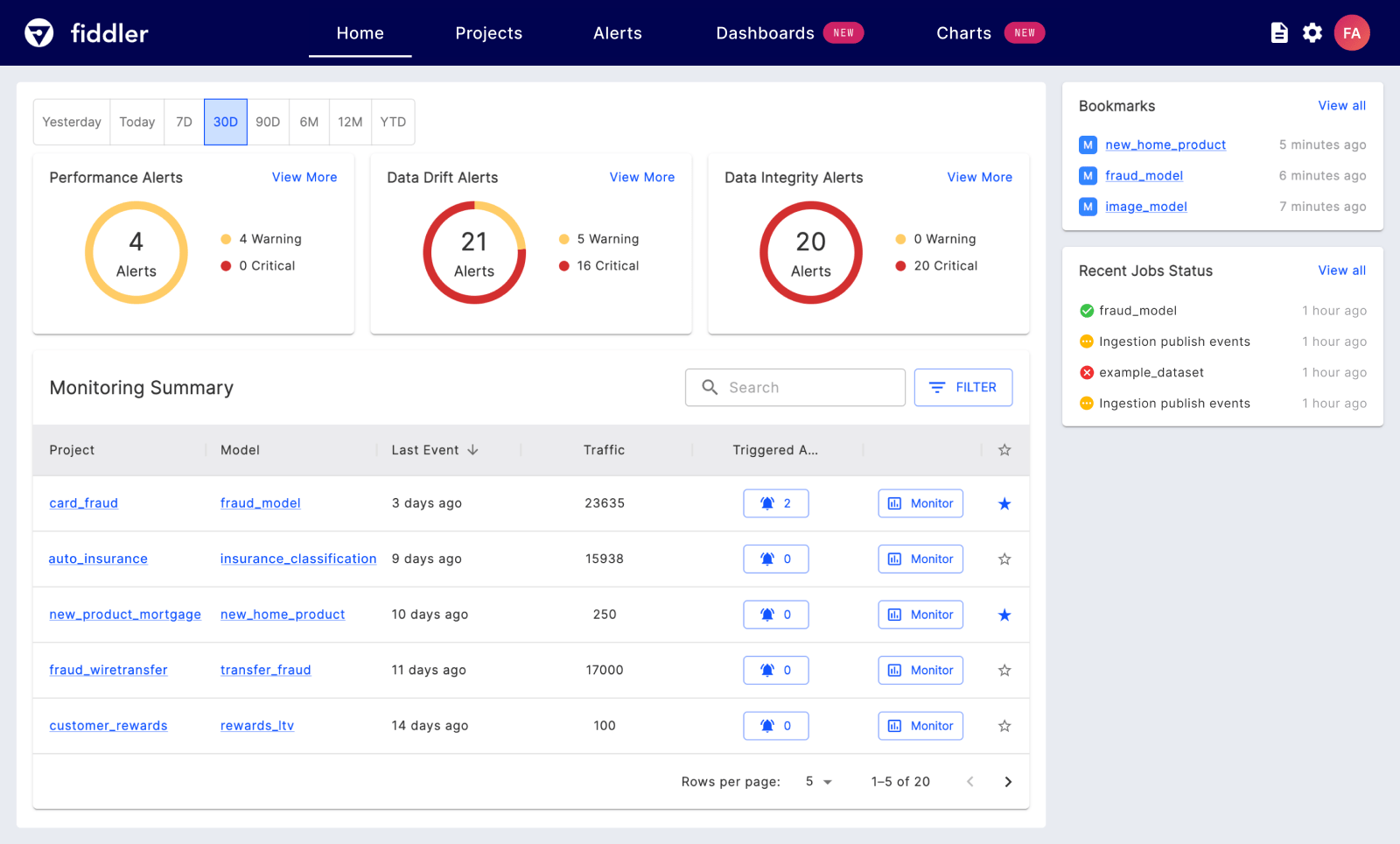
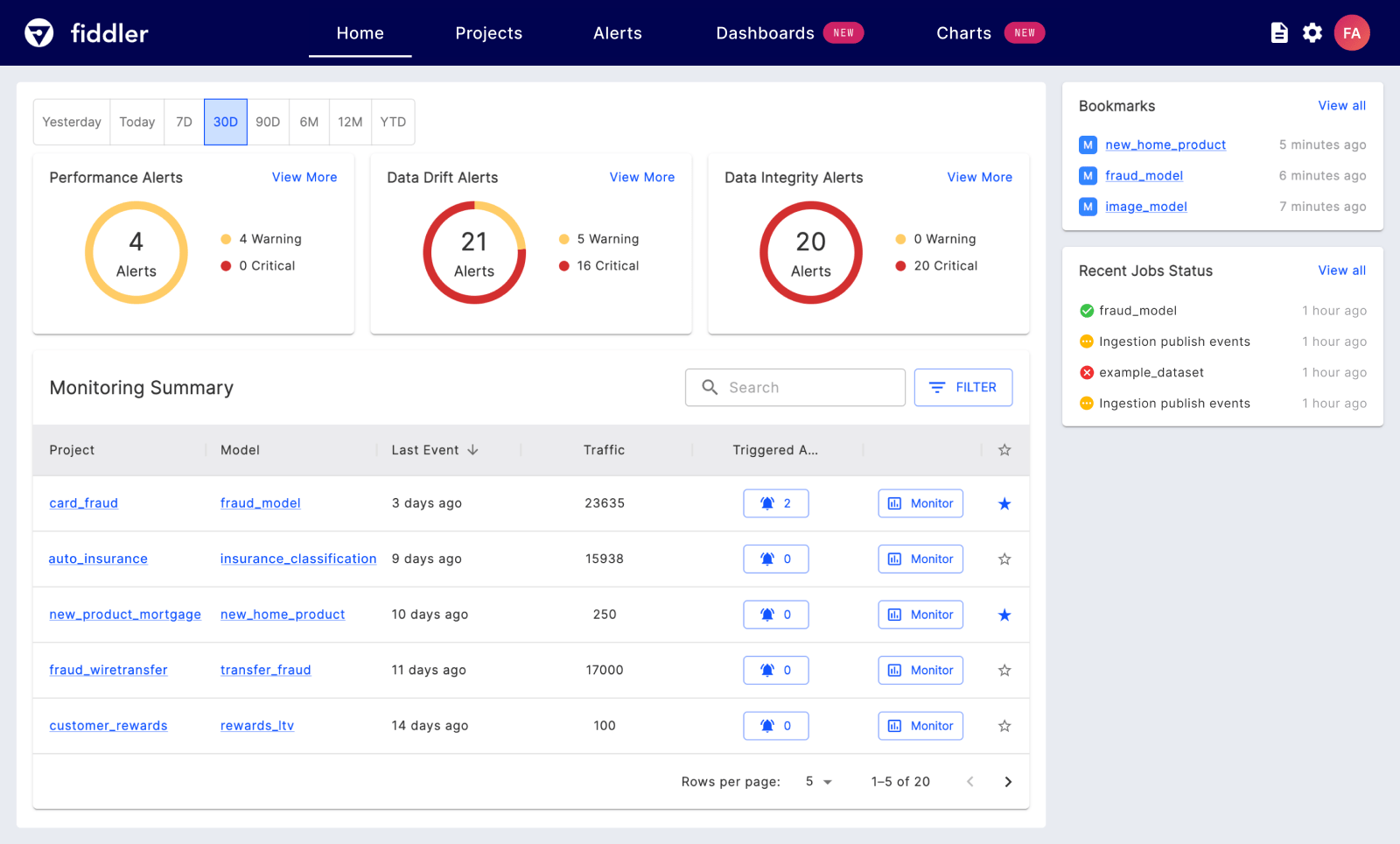
Observe AI/ML models using Fiddler and Google Vertex AI
Fiddler’s monitoring integration is an easy two-step process using a Python library.
- Ingest training data sample from Vertex AI Feature Store, BigQuery, or Cloud Storage. Fiddler uses this data as the baseline when computing explanations and performing monitoring.
- Publish inferences made by the model to Fiddler. First, the inference data needs to be captured in Vertex AI Feature Store, BigQuery, or Cloud Storage, and then the stored inference data needs to be published to Fiddler.
In addition, to fully leverage Fiddler’s explainability functionality, the customer also needs to upload the trained ML model from Vertex AI Model Registry. Fiddler uses the uploaded model for faithfully explaining the model behavior and for computing feature attributions in real-time.
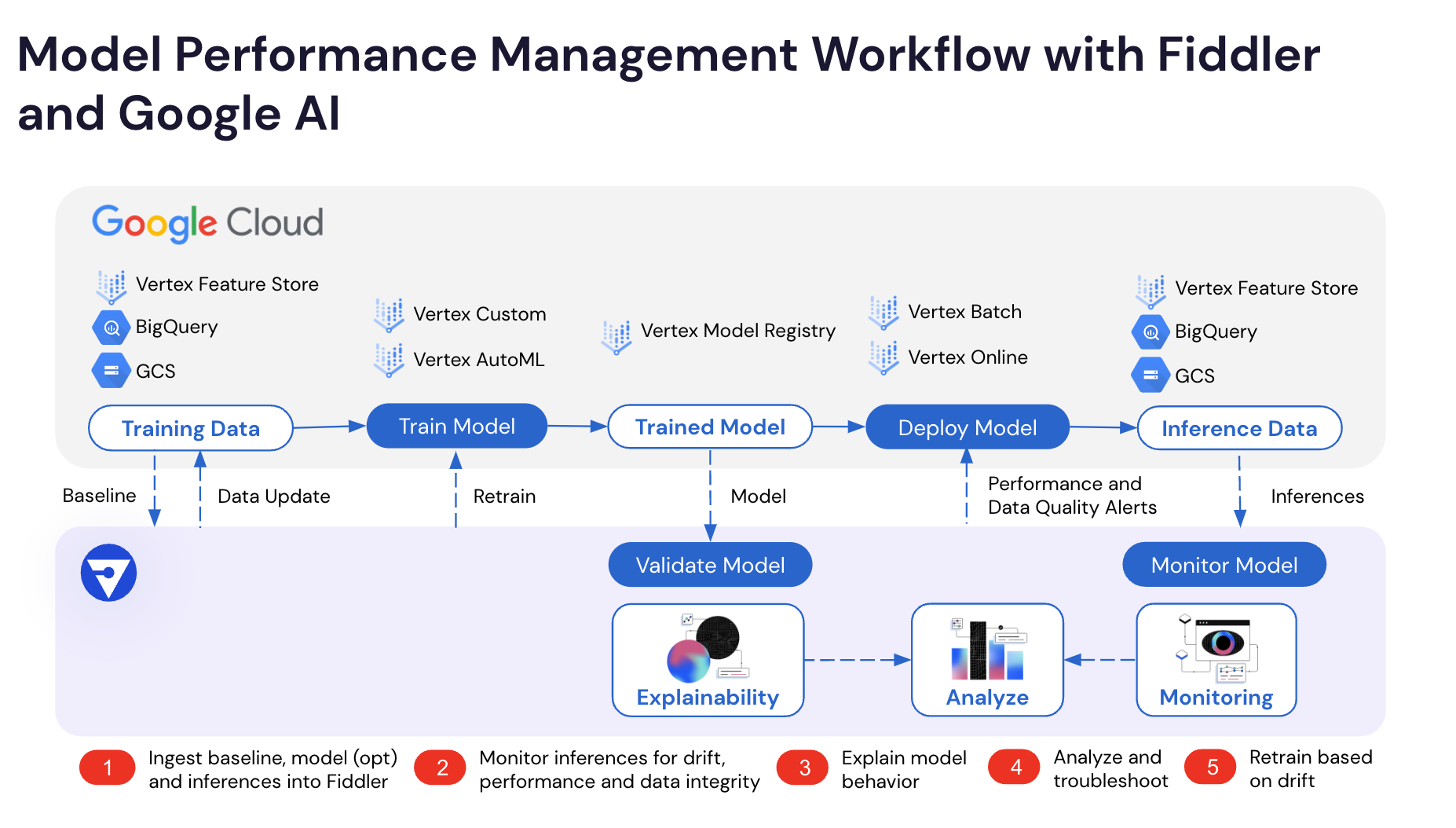
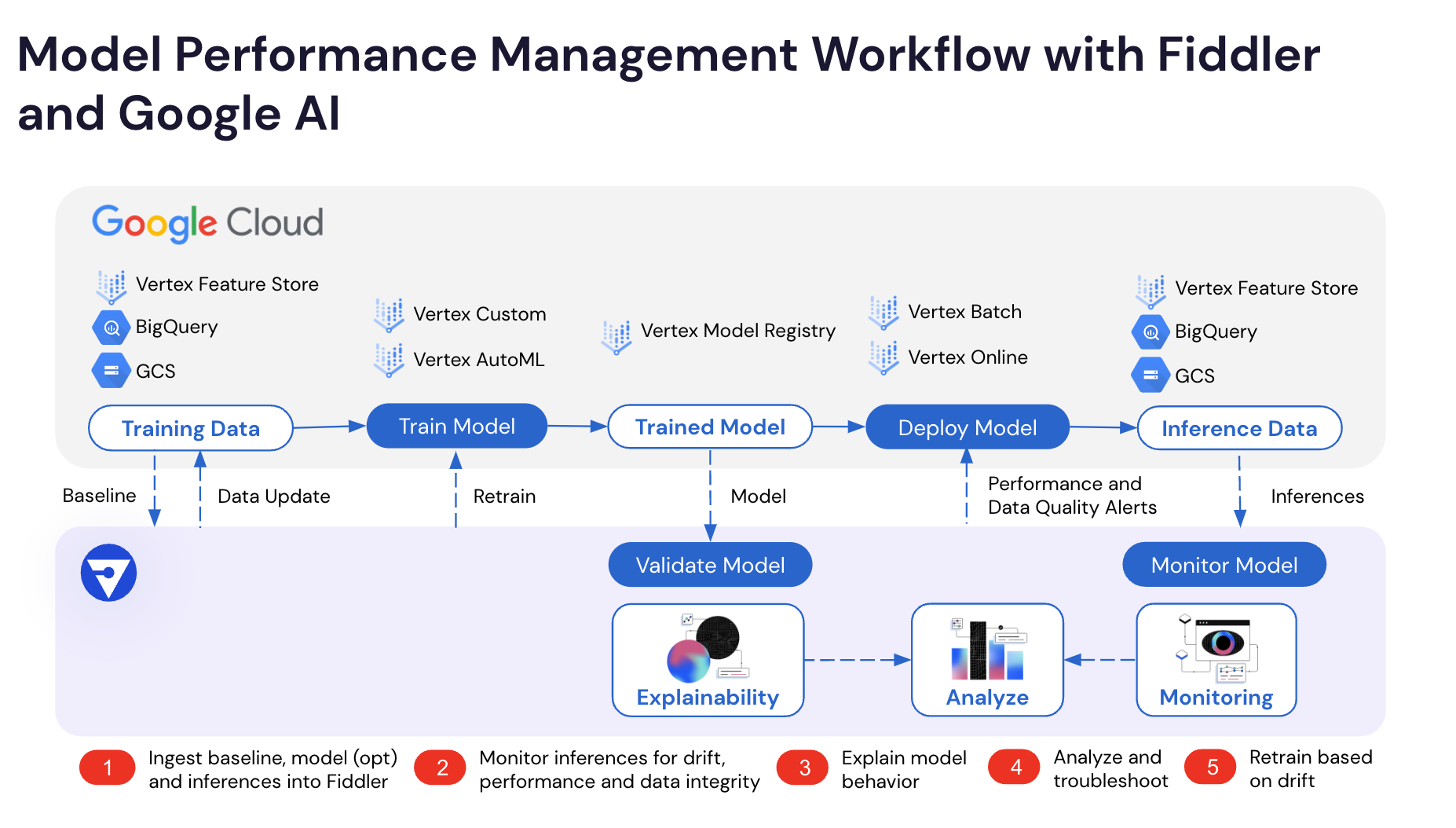
AI Observability Workflow with Fiddler AI and Google Cloud
Real-world impact
Top U.S. banks, fintech, e-commerce, hiring, and other Fortune 500 companies have used Fiddler to explain the predictions of their models, monitor deployed models, perform root cause analysis when issues are detected, and thereby manage the risk associated with their models.
Model risk management: The opaque nature of AI leads to risk exposure and costly inefficiencies. A top-five bank partners with Fiddler to implement explainable monitoring, mitigate risk, and solve operational inefficiencies. In particular, the bank needed to monitor ML models deployed to production across several lines of business due to regulatory auditing and compliance reasons. Furthermore, the bank had multiple redundant ML serving systems across lines of business, resulting in undue maintenance overhead and lack of centralized best practices and oversight mechanisms. By partnering with Fiddler, data scientists were able to upload trained models to Fiddler to allow model validators to review and test models for risk and bias. Once models are validated and then deployed in production, Fiddler presents insights on model performance and behavior over time. The oversight and consistency provided by this centralized platform helped eliminate both the risk of untracked production models and the cost and overhead required to build and maintain multiple serving systems across lines of business. Combined, the bank projects these savings to be several millions of dollars per year.
Fraud detection: Fraud detection is critical to maintain user trust and operational efficiency in e-commerce platforms. Traditional fraud detection systems rely on rule-based logic backed by operations personnel manually reviewing potential fraudulent cases, often resulting in many unmanageable rules and exceptions. Fiddler helped a large e-commerce company to transition from such a traditional system to an ML-based fraud detection system. The fraud detection team’s data scientists partnered with Fiddler to build an in-house buyer fraud classification model with improved accuracy and fewer false positives compared to the earlier rule-based system. Fiddler’s explanations and insights help the agents understand why certain transactions were classified as fraudulent. Using Fiddler, the team increased the speed of their fraudulent transaction review process considerably and enabled agents to provide better feedback to internal stakeholders and customers.
Model drift detection and resolution: Timely detection and resolution of drifts in the behavior of ML models is a crucial problem faced by any company that has deployed ML models in production. The lack of reliable tools to detect and resolve such issues can significantly impact business metrics such as revenue and profit as well as result in reputational risk and poor quality of service. Fiddler partnered with a company that offers a specialized large scale business analytics and data science platform for e-commerce companies. The company expanded the number of ML models in production without having sufficient tooling for monitoring the deployed models. As a result, timely insights into model performance were sometimes missed, causing a reactive rather than proactive allocation of resources to production issues. The Fiddler MPM platform helped this company to improve their model lifecycle management and data understanding capabilities by adopting automation and standardization tools. In particular, Fiddler helped the company obtain visibility into:
- model predictions, changes, and other events
- a record of how models have been performing over time; a single source of truth for model predictions
- a centralized tool to analyze and debug model performance issues
- and standardized model interpretations.
Fiddler also helped the team to decide when and how often to retrain their models, and more broadly, to refine their model maintenance and governance procedures.
Better together: Fiddler AI + Google Cloud
Fiddler AI running on Google Cloud enables Fortune 500 companies and other companies adopting AI/ML models to validate their models before deployment, detect and resolve issues encountered by deployed models, perform root cause analysis powered by explainable AI, and thereby ensure that models continue to retain performance, comply with regulations, and satisfy responsible AI principles.
LLMs and other generative AI-based applications have recently opened up exciting new possibilities and are set to turbocharge enterprise use cases across industries. However, there is an acute need for appropriate AI safety and monitoring mechanisms for Generative AI models and applications. Fiddler can seamlessly extend visibility into these advanced AI deployments on Google Cloud with robustness auditing, embedding, input, and output monitoring and other responsible AI functionality in one single pane of glass.
Learn more about Google Cloud’s open and innovative generative AI partner ecosystem and about Fiddler on Google Cloud.