Archive Data: Old or historic?
Aman Gupta
Enterprise Solutions Specialist, Google Cloud
Eddy Ciliendo
Vice President, Model9
Try Google Cloud
Start building on Google Cloud with $300 in free credits and 20+ always free products.
Free trialTo be “historic,” an event or place must be associated with a degree of importance that makes it worthy of notice, study, or preservation.
To be “old” merely means something has been around a long time or occurred long ago.
Regulations and compliance enforce companies notably Insurance, finance, healthcare to preserve their customer, applications and transaction data for a minimum period. The period can be as low as 7 years and as high as forever. As a result, organizations end up having a huge amount of archive data which is typically stored in physical or virtual tapes. The archive data stays inactive and becomes ‘old’ in no time unless the government agencies ask for past data or reports.
With increased adoption of cloud technologies, there are now innovative ways and means to transform the archive data from ‘old’ to ‘historic’. Google cloud and Model9 have partnered to transform legacy archive data into a valuable asset and means of differentiation.
Below are the few reasons why archive data transformation should be given a serious thought.
Simplification: On-premises tape and virtual tape infrastructure can be complex to operate and maintain. Mainframe tape subsystems need specialized skills in a field where skills are a scarce resource as it is. Not only do on-premises tape systems require specialized skills but they also require a proprietary FICON infrastructure that can not be shared with other platforms and that needs to be maintained and replaced every 5 years. In addition to the aforementioned shortcomings, physical tapes are also prone to failure requiring on-site personnel to replace physical media, restart failed backup jobs etc. A cloud based solution to backup and archive data removes all that complexity with the simplicity of an industry standard cloud access, freeing up critical skills and eliminating complex infrastructure operations and planning.
Cost benefits: Cloud based Coldline and Nearline storage has reached incredibly competitive price points compared to virtual or physical tape libraries and their specialized FICON SAN infrastructure.
MIPS Reduction: Model9 moves the data processing from General Purpose (GP) MIPS on the mainframe to zIIP processors thereby reducing GP MIPS consumption and hence lowering overall mainframe consumption and cost (monthly license charge or tailor fit charges)
Leaner Mainframe: Moving the archive data which typically runs in 100’s of terabytes to 10’s petabytes, to cloud storage makes overall mainframe estate much leaner and hence becomes manageable to move to cloud.
Data corruption/Cyber Resiliency: Backup copy on cloud can be used to restore mainframe applications in the event of any unforeseeable cyber attack or data corruption due to any software upgrade, data contention or any other reason. Google cloud can provide pristine backup anywhere in the globe.
While the aforementioned use cases have a lot of intrinsic value, they are geared more towards keeping archive data without changing their value for an enterprise from ‘old’ to ‘historic’. The next set of use cases focus on extracting value from an organization’s archive data. Mainframes have long been the backbone of many organizations and oftentimes contain decades worth of data such as customer interactions, credit card transactions, airline bookings and policy data just to name a few. Unlocking this treasure trove of data for some of the below use cases can yield tremendous value out of an asset that was previously thought of as a liability or technical debt.
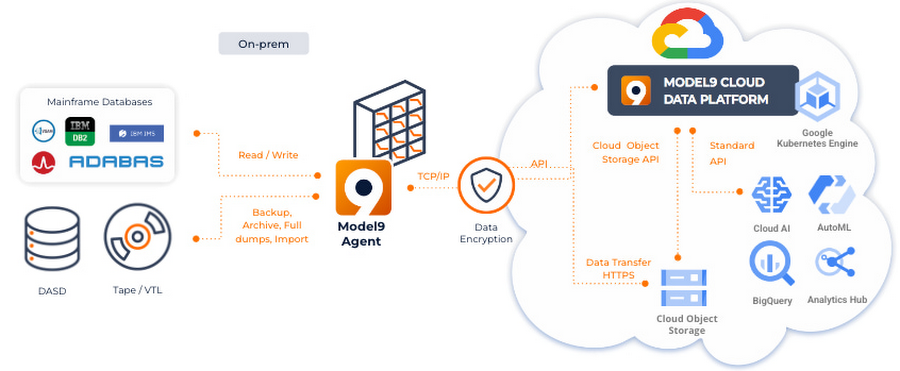
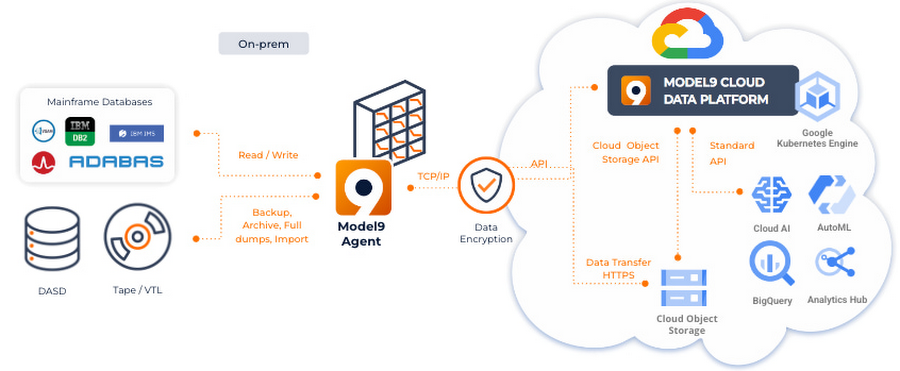
Massive data sets to train algorithms: Google cloud is focused on building numerous industry specific use cases leveraging the power of Vertex AI to transform the business. Some examples are fraud detection, automated claim settlement, inventory management. Mainframe archive data can contain decades and 100’s of Terabytes of historical data can be used to better train and refine machine models.
New product/business model: Rising trends in Gen Z like Micro lending, social economy, everything-as-a-service are sprouting new business models and products. Archive data transposed with current socio-economic patterns can help validate the success and viability of new models before these are rolled out to a large market.
Consumer behavior: Predictive analysis of historical data can provide valuable insights about the consumer behavior at individual, region and geographic levels which can help organizations take critical decisions about cross-selling and upselling along with decommissioning of slow moving catalog items. These optimizations will lead to a leaner catalog with lower shelf life and higher margin.
Reporting: When authorities ask for data or reports from the archives, a typical turnaround is 4-5 weeks to identify the right dataset, restore the tape, run the jobs, create the report and then share. With archive data on Google cloud, commonly requested reports can be configured with Looker so the authorities request can be responded to in a timely manner which will foster a better public-private partnership.
Above use cases just scratch the surface of what can be done once Google’s BigQuery and Analytics tools start working their magic on the decades of historical data stored in mainframe archives.
Historical data processing by Google’s BigQuery and smart analytics solutions enable limitless data-driven innovation to drive customer delight and business amplification.
On the data transformation roadmap, organizations can commence from moving archive data to Google cloud, then HSM storage and finally active DASD storage. Once a solid, consolidated and comprehensive data management and governance platform is established on Google cloud, focus may be shifted to either moving workload or building new applications using cloud native technologies on the available data on Google cloud.