Three proven strategies for optimizing AI costs

Marcus Oliver
Managing Director, Google Cloud Consulting
Eric Lam
Head of Cloud FinOps, delta, Google Cloud Consulting
Identifying specific use cases, understanding total cost of ownership (TCO) of AI models and embracing cloud FinOps together lead to greater financial value from generative AI initiatives.
Imagine this: For years, your marketing team has been struggling to personalize marketing content at scale. With gen AI, the ability to customize every campaign, offer, and interaction for each of your customers is suddenly within reach. The question is: Do you have the funds to make it happen?
Though gen AI potential may be unlimited, budgets are not — and harnessing this powerful new technology can be a minefield of unexpected expenditure. Not only does AI require computational resources, the overall costs for implementing and maintaining AI can vary widely depending on various factors, such as scale, model complexity, data requirements, infrastructure maintenance, and existing talent.
As a result, many organizations are finding it difficult to put a price on gen AI and quantify its business value – but, cost optimization is both a financial and strategic imperative. Accurately predicting and managing costs, allocating resources more effectively, and measuring the value of gen AI initiatives is critical.
At Google Cloud, we recognize that understanding and optimizing AI costs is needed to achieving future success. Time and time again, we hear in our conversations with executives and top leadership that they are looking for ways to effectively budget for gen AI implementation while delivering real value from their investments towards the long-term goals of their organization.
With that in mind, here are three practical strategies for optimizing AI costs that we use in our own work and when helping customers embark on their gen AI journeys.
Identify your AI use case(s)
Whether you’re integrating gen AI, or another type of AI technology, a golden rule is making sure you align AI initiatives with your business goals. Though powerful, AI is still just one technology available in your toolbox. Using it in isolation without intention is like driving a car without a map – you’re bound to end up lost or, even worse, at a dead end. To maximize the impact of your investments, it’s critical to identify your AI use cases.
A strong use case should capture input about the ability to solve user pain points while driving alignment with overall strategic vision. Our customers tell us their primary use cases are increasingly focused around developing intelligent AI agents to improve productivity, automate processes, or modernize customer experiences, and we’ve seen hundreds of these solutions come to life in real-world applications over the last year.
Our Google Cloud Consulting team works with customers to identify and prioritize AI use cases that drive the most business impact. We like to start by identifying the specific, measurable business objectives we want to address with AI and work backwards to find a solution that contributes directly to achieving those goals. This process should include determining plans for measuring success, evaluating whether or not AI can actually provide the output needed to solve the identified problem, and ensuring solutions connect to overarching strategic objectives and priorities. As Lee Moore, VP of Google Cloud Consulting, puts it, "We want to ensure that AI is not just a technological implementation, but a strategic enabler for our customers' businesses."
Framing use cases around a particular business problem not only helps prevent wasting resources on projects that don’t deliver significant value but also makes it easier to clearly define the individual elements and next steps needed to implement AI successfully. This approach helps determine a project’s technical feasibility and business viability along with a clear set of goals and criteria to measure success, enabling more effective resource allocation.
Get familiar with the TCO of AI
Another essential aspect of effective AI cost optimization is establishing a holistic view of your expenses, particularly the incremental costs that can accumulate as AI projects scale. We find it helpful to model the Total Cost of Ownership (TCO) of each AI use case to help inform decision-making and discover opportunities for optimization, such as exploring more efficient models or licensing options, rightsizing hardware, and improving data quality.
Our approach is to break down business use cases into product-specific use cases, mapping each one to specific gen AI models. We then decompose each model into different components of cost to help us estimate the future investment needed to build and scale gen AI projects. This exercise also helps to illustrate the different levers organizations can employ to reduce or optimize the TCO of their AI investments, including:
- Model serving costs: Inference costs for serving models
- Training and tuning costs: Costs for additional model training and tuning
- Cloud hosting costs: Costs for running models on cloud infrastructure
- Training data storage and adapter layers costs: Storage costs for additional training data and resulting adapter layers
- Application layer and setup costs: Costs for additional cloud services to run models
- Operational support costs: Costs for ongoing support of AI models
For instance, a pre-trained AI model will carry different costs than opting for a foundation model, which requires building, training, and deploying a custom gen AI model from the ground up. The TCO of choosing a pre-trained model might include costs for model serving, training and tuning, and cloud infrastructure. By comparison, building and training your own custom gen AI model will likely incur expenses across all cost categories, including operational support, application layers, training and tuning, and model serving and inference.
At Google Cloud, our objective is to give our customers access to the best models suited for enterprise use cases while also providing the ability to easily optimize the costs of their AI projects according to a wide range of needs and requirements.
Our Model Garden on Vertex AI includes a curated collection of more than 160 first-party, third-party, and open models, allowing organizations to experiment with and choose the best model for their use case, budget, and performance needs — and switch between them as needed. We help customers unify all their data and make it easy to connect it with transformative AI technologies, offering robust data analytics and storage services that can provide significant savings when storing and querying data.
Gemini 1.5 Flash, the newest addition to the Gemini model family, is optimized for high-volume, high-frequency tasks at scale and is more cost-efficient to serve. We’re also constantly innovating to find fresh ways to realize even more savings, introducing new capabilities, such as dynamic workload scheduler, context caching and provisional throughput, to reduce the costs of requests and help make gen AI costs more predictable.
By effectively utilizing these different TCO levers, organizations can adapt and make adjustments to their AI strategies to optimize costs, increase efficiency, and gain more overall value from gen AI.
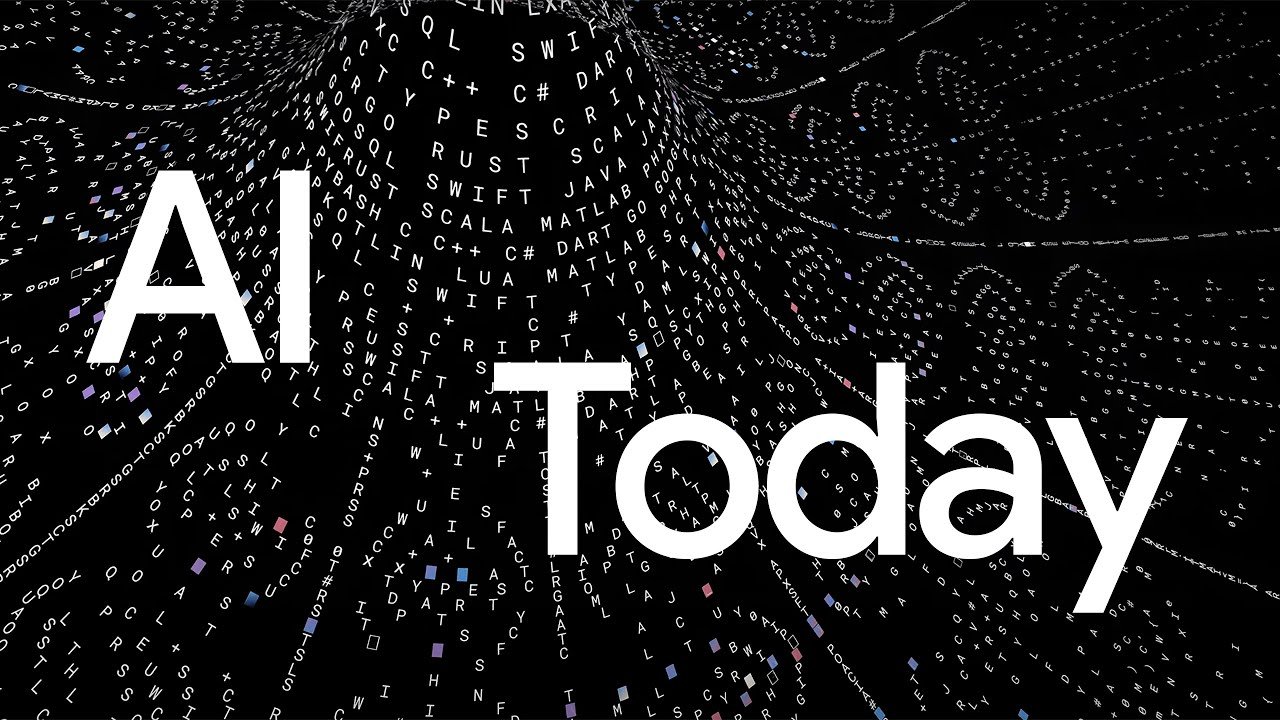
Embrace cloud FinOps
One of the biggest hurdles when adopting gen AI is figuring out effective ways to control cloud costs. Gen AI models require an extensive amount of computing resources and data storage to process and store training data and generate outputs. The demand for cloud services also tends to surge as projects scale, leading to increased cloud usage and driving up costs even more.
To keep costs in check, we believe it’s crucial for teams to adopt Cloud FinOps — an operational discipline and cultural shift that brings together technology, finance, and business teams to drive sustainable financial value and maximize their cloud investments. In addition, gen AI technology can also serve to turbocharge FinOps workflows, amplifying the impact of these practices and helping organizations to hit cloud cost optimization targets even faster.
For instance, the introduction of gen AI capabilities in business intelligence (BI) tools like Looker is transforming the way teams can interact with and explore BI data, enabling everyone in an organization to uncover patterns and trends using natural language. CME Group and Palo Alto Networks, for instance, implemented cost anomaly detection using Google Cloud to help detect unexpected cloud spend, so they can find and address issues early to avoid billing surprises later on.
At Google Cloud, we have developed the Cloud FinOps for Generative AI framework to help customers assess gen AI adoption readiness across people, processes, and technology. The framework anchors around five key pillars:
- Gen AI enablement: Using enablement campaigns and on-demand training to ensure successful gen AI integration from a fiscal perspective across all roles, from technical and financial roles all the way to the C-suite.
- Cost allocation: Understanding the value of gen AI along with the lifetime cost of developing and deploying gen AI models, including the allocation of different cost components and measuring their impact on the bottomline.
- Model optimization: Continuously monitoring and optimizing model data and features, training and deployment processes, and model development automation (MLOps) to improve their performance.
- Pricing model: Understanding the various pricing constructs of different types of gen AI models and providers to drive better decision-making about what models to use and how to implement them.
- Value reporting: Reporting the cost and value metrics of gen AI for technology to demonstrate the value of gen AI to business, and finance teams, so they can make more informed decisions about how to scale it across the organization.
Cloud FinOps principles and practices can help organizations to get the most value of their gen AI investments, empowering them to accelerate business value realization and innovation, increase financial accountability, optimize cloud usage and costs, and prevent the sprawl of cloud spend.
With the invaluable assistance of the Google Cloud Consulting delta FinOps team, we were able to establish a pivotal FinOps function within our organization, enabling us to unlock the value of the cloud from the outset.
Leslie Nolan, Executive Director of Finance Digital Transformation, CME Group
Growing your bottom line
The AI landscape may be vast, but budgets remain finite. It’s imperative for leaders to master navigating the nuances of AI costs and make informed decisions that will allow them to sustain their initiatives and tap into the full potential of gen AI. Understanding use cases, getting comfortable with the costs of AI products and features, and fostering a culture around cloud FinOps within your organization are all strategies that can make a significant difference in costs when deploying AI and ultimately allow you to achieve a greater return on your investments.
Google Cloud Consulting is helping customers around the world adopt AI technology and achieve business impact. Learn more about innovation and transformation services for your organization.