So much more than gen AI: Meet all the other AI making AI agents possible

Hamidou Dia
Vice President, Applied AI Engineering
There are lots of promising AI technologies suited to different activities. Their real strength comes when combined to handle multiple or more complex tasks.
The same way you’ve been hearing all about generative AI for the past year, you may have noticed that the next next big thing has already entered the chat: AI agents.
As we’ve quickly discovered, generative AI is one of the most powerful technologies developed in generations. But there is also an entire constellation of established AI technologies — many of which are purpose-built to perform certain business tasks – and when combined with generative AI, can accomplish even more.
Much of the magic of gen AI that’s been capturing our attention is rarely achieved on its own. Some of the most exciting innovations we’ve come to associate with generative tools actually come from tapping into other AI systems that help enable advanced reasoning, intelligent decision-making, multi-step planning, and taking complex actions. This revelation is laying the foundations for building sophisticated, connected AI agents that can serve customers, empower employees, amplify creativity, and accelerate coding or data analytics.
Put simply: You don’t always need gen AI, but when you do, it can become even more powerful when used in concert with other types of AI.
As we enter the era of the AI agent, it’s worth remembering that gen AI is only one technology in a much larger AI toolbox that’s already currently available — and you’ll likely need to leverage every AI at your disposal to make the most of this transformative technological moment. That’s what AI leaders are already doing.
There’s more to AI than one technology
Foundation models and large language models, or LLMs, can create virtually any type of content, such as text, images, video, audio, or even computer code. The machine learning models that power generative AI capabilities can detect patterns and structures in their training data and create completely new content with similar characteristics.
The potential and general accessibility of this kind of AI is broadly what has excited so many business leaders. While other kinds of AI have been around for awhile, they often required specialized knowledge or expertise. The exciting confluence now is how gen AI is not only made more useful with input from other types of AI — gen AI interfaces can also make it easier to understand, operate, and manipulate more technical forms of AI, such as predictive modeling or vision and audio recognition.
Let’s say you ask an AI agent, “Which of these stocks outperformed their benchmarks in the last six months?” A gen AI model alone doesn't have the functional capabilities to look up and ask questions about different forecasts related to your business. In this case, gen AI is responsible for interpreting the prompt, triggering a function call to get the forecast, and then interpreting that forecast to provide an answer. But actually generating the forecast falls to a different type of AI system entirely, not the gen AI model running the rest of that experience.


Additionally, if you want your model to be smarter about interpreting your prompt asking about stock performance, grounding the model in relevant information — whether that be search data or your enterprise data — can greatly enhance the outputs. Also, by understanding the semantic meaning of a user's prompt, generative AI models can better grasp the desired outcome. These two steps — grounding and semantic search — help users generate results from generative AI more relevant to their business and their use case.
According to McKinsey research, generative AI could increase the impact of all AI technologies by as much as 40% — an estimate that roughly doubled when considering generative capabilities embedded into other tools used for tasks beyond established use cases.
Overall, such predictions underscore that as AI continues to mature, organizations will increasingly rely on a mix of several different technologies to create the AI agents that bring gen AI potential to life. And as organizations are eagerly exploring the future potential of AI, it’s imperative to start exploring all the AI technologies out there and start planning a clear path to AI agents.
With that in mind, let’s take a look at some of the most popular AI technologies that are becoming more accessible, extendable, and powerful with help from gen AI:
Predictive AI
Predictive AI technologies analyze historical data and identify patterns to forecast future outcomes. These insights can help support your decision-making, guiding your responses and actions. Predictive models also power recommendation engines that can analyze browsing behavior, purchase history, and personal preference to serve up anything from the ideal sheet set for a customer’s favorite comforter to a perfectly curated playlist.
Already, predictive modeling is helping organizations to optimize fleet delivery routes, forecast weather patterns to reduce flight delays, and catch potential quality failures on the factory floor — and gen AI is making these forecasts even more innovative, robust, and accurate. With gen AI, for example, you can generate realistic future scenarios or fill gaps in training datasets with synthetic data. Likewise, predictive AI can help steer more targeted content creation and optimization with predictions about user preferences or behavior, enabling more personalized experiences.
Vision AI
Vision AI can help you understand, analyze, and extract information from images and videos. You can train computer vision models for a wide range of business applications, from the straightforward to the complex.
For instance, you can use vision AI to detect and classify specific objects (like potholes on a city street), places, or even actions within videos or images. You can also create models that can scan images, detect objects, and generate valuable image metadata. Some brands have even used computer vision techniques to help them achieve 3D style transfers, such as applying a 3D makeup look that moves with a customer’s face.
Combined with multimodal gen AI models like Gemini, organizations can now deliver creative AI agents capable of performing pro-level edits to photos and videos or finding the most exciting moments from 25 years of video footage.
Conversational AI
Conversational AI refers to a category of technologies that can simulate human conversation and interaction, including machine learning, natural language processing (NLP), automatic speech recognition (ASR), and text-to-speech (TTS). Together, these technologies can enable computer systems to interpret human language as structured data and then trigger appropriate responses to customers like a human agent would.
Additionally, conversational AI models can also assist and empower human agents by helping to detect customer sentiment or intent and providing continuous support to help them resolve issues more quickly and increase customer satisfaction. One of the most common examples is building virtual call center agents that are able to provide natural, intuitive customer experiences while handling complex conversations and requests.
While gen AI and conversational AI are often used synonymously, the reality is that creating agents that can interpret, respond, and interact with people in human-like ways relies on multiple technologies However, the rise of generative AI has naturally served to improve conversational AI capabilities, allowing organizations to create ever more realistic and intelligent AI agents that can translate conversations between two languages in real time, deliver news and audio recordings, or even help you pick out your next luxury car.
Transcription and Translation AI
Similarly, speech and audio AI technologies play a big role in delivering conversational AI experiences, but they can be used for a wide variety of other business applications beyond chatbots and virtual agents and assistants. For instance, you can leverage specialized speech and audio AI models to transcribe audio like conference calls into text notes or create subtitles for videos. There are even models that can automatically detect languages and translate text or speech in real time.
These technologies are not future innovations taking shape, they are more established and in many cases, better understood. More importantly, they are still relatively underutilized, meaning there are many opportunities still ripe for the taking, especially when integrated with gen AI tools.
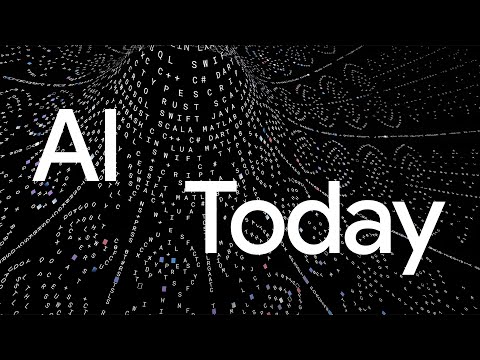
AI agents on the horizon
One of the most striking shifts with the emergence of generative AI is how it has unleashed everyone’s imagination and changed how we think about interacting with data, systems, and each other. Organizations may have considered AI investments in the past and passed, but now, they see possibilities where they didn’t before.
This fresh outlook has businesses looking at how they work and operate, and asking new questions:
- Can a camera look at a warehouse stack or a retail store and determine whether stock has been shelved correctly and safely?
- Is it possible to find out how many times a customer purchased a specific type or brand of orange juice? And what complementary products are most likely to result in additional purchases?
- How much faster does an AI system make researching and developing new materials and protein applications?
- Can you detect and diagnose diseases faster and more accurately by analyzing imaging scans?
- Can you automatically surface every video clip in a database that contains fireworks, or home runs?
- Can an automated system interact with customers the same way a human employee would?
What once were seen as specialized use cases are increasingly becoming more generic as organizations gain the capabilities and tools to manage their data and leverage AI and machine learning. The bar on digital-meets-real-world experiences has been raised, reigniting interest in other types of AI technologies — and increasingly, their context when building AI agents — that might have been pushed to the bottom of the priority list before.
Generative AI is groundbreaking because it enables you to generate, synthesize, and summarize unstructured data. But its true potential is helping to remove many of the most significant roadblocks that have limited AI to the realm of experts and engineers. Much like a team that works together to deliver a project, gen AI acts as a leader helping to connect the right people and technologies together to provide different types of knowledge, insights, capabilities to reach the final goal.
While generative AI can help extend the value of AI within the enterprise, it would be shortsighted to view it as the only AI technology with the power to do so. In reality, the future of AI will likely involve using multiple models and AI techniques, some existing and some new, as we continue to discover novel use cases and capabilities.
Opening image created with Imagen 3 in Vertex AI, using the prompt: "a world of possibilities drawn in a flat style for a magazine, please make it look technological but not futuristic with lots of business activity and excitement circling the globe."