Your data is the fuel for AI: 5 steps to build strong data foundations
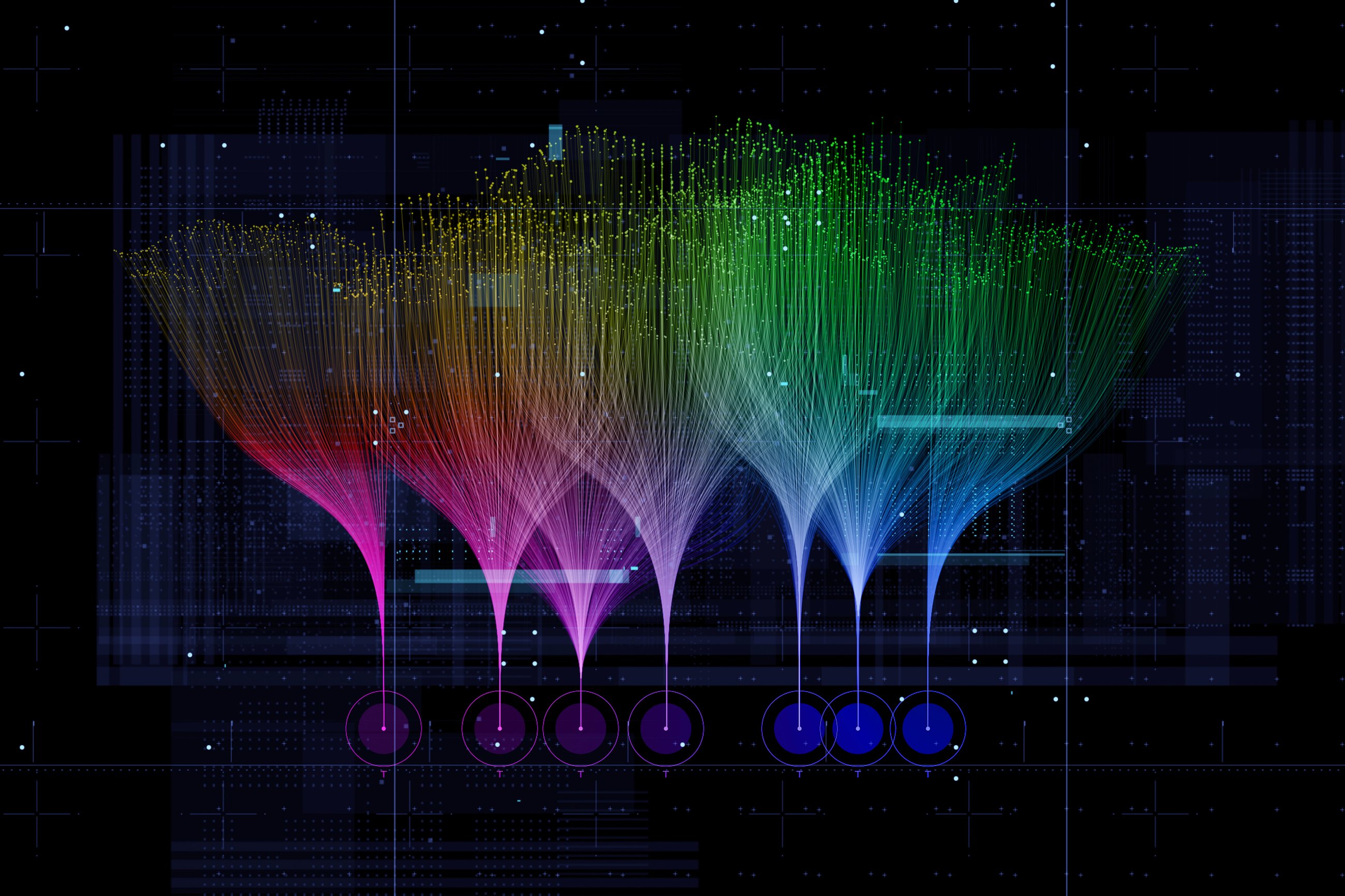
Ami Dave
Senior Principal, Data Analytics, Value Creation, Google Cloud
Joyeeta Banerjee
Global Head Data Analytics and Security, Value Creation, Google Cloud
Data challenges impede generative AI scaling, necessitating a strong, unified data strategy for AI success.
Even as generative AI adoption booms, many organizations are encountering a significant roadblock standing in the way of success — their data. While businesses may be ready to embrace AI technologies, many lack the proper foundation to turn their visions into reality.
According to McKinsey, 70% of organizations adopting generative AI experience difficulties with data governance, integrating data into AI models, and insufficient amounts of training data. As a result, they are struggling to scale pilots successfully to production, held back by data strategies that limit accessibility, create barriers to discoverability, and compromise data quality.
To help navigate these issues, we recently published “An executive’s guide to delivering value from data and AI,” based on our work with tens of thousands of organizations on their data and AI platform strategies. If you’re looking for guidance as you build your own data strategy, we’ve outlined five fundamental steps for you here to build a solid data foundation for AI success.
1. Build a strong AI-first data strategy.
Meeting the demands of the AI era starts with a strong data strategy that incorporates the key tenets of your organizational culture, fosters collaboration between business and data practitioners, and contributes towards building an end-to-end data ecosystem. In many cases, rebuilding data strategies not only requires aligning people, processes, policies, and technologies but also a shift in your company mindset about the intrinsic value of data.
Data is a strategic asset and should be treated accordingly. One of the hallmarks of a strong data strategy means considering whether it supports your future AI strategy at scale and broader strategic objectives — whether that’s driving revenue growth, optimizing costs and processes, enhancing customer experiences, or improving productivity. Critically, AI-first data strategies leverage data to advance strategic priorities, establish clear governance policies, and provide the skills, tools, and processes that empower everyone within your organization to access, discover, and use data effectively.
2. Establish a single source of truth for your AI initiatives.
For gen AI to deliver on its promises, you first need to be able to connect your data to AI. More specifically, you need all your data available as input — not locked away in silos or inaccessible. In particular, unstructured, multimodal data, such as videos, images, and text, is often scattered across different platforms and stored in diverse formats, making it difficult to consolidate and implement comprehensive security and governance policies.
At Google Cloud, we have found that copying or migrating data to one place is often not practical, nor is it cost-effective. Instead, we recommend pursuing an open approach to data, underpinned by a unified platform that brings together all types and formats of data, including open formats, into a single source of truth. This open approach makes it easier to manage the entire data lifecycle while providing a single, reliable source that everyone can use to extract insights and make decisions.
A great example of this is Bayer created a modern data solution to efficiently collect and analyze billions of environmental data points to support its innovative agricultural division. The ability to store and connect all of its data has helped streamline operations, centralize its technology, and improve collaboration across the company, enabling hundreds of teams and applications to easily access vital datasets for research and development.
3. Accelerate your data to AI journey…with AI.
Unified data enables you to harness AI, but AI is also key for unlocking the full potential of your data. With the rise of agentic workflows, we are increasingly seeing organizations integrate gen AI and AI agent experiences into their user journeys to improve productivity and outcomes.
For instance, AI agents can assist with a variety of tasks, including writing queries, data migration and management, data transformation creation, and even data visualization. Imagine being able to query data simply or getting recommendations on how to optimize costs or ensure compliance simply by asking questions and getting answers in natural language, right in the tools you use everyday.
We see many of our customers already benefiting from gen AI capabilities embedded directly into their data platforms, improving knowledge discovery and data analysis by enabling employees to “chat” with their enterprise data. Introducing conversational analytics, for instance, which combine natural language processing with gen AI, can help enable users to ask questions about their data in plain language and get back clear, concise answers. Instead of relying on centralized IT and data analysts for answers, anyone — regardless of technical expertise — can access data, find information, and gain accurate insights quickly.
4. Manage, govern, and secure data across the AI lifecycle.
The more data you collect, the more security and governance matter. Data can be a liability, especially given the cost of data breaches can potentially reach into the millions and how quickly both threats and regulations are evolving. To keep data secure and compliant, organizations will need the ability to manage and govern data but also secure it — from end to end.
A good place to start is building a durable data governance framework that prioritizes data quality while also implementing umbrella policies that you can manage and enforce across all your environments. Some steps might include implementing data observability tools to monitor data lineage, detect anomalies, and address data quality issues. For instance, cloud content management leader Box adopted Dataplex, Google Cloud’s data governance solution, as its central data catalog, providing streamlined data discovery, end-to-end observability for its data ingestion pipelines, and comprehensive capabilities for data classification.
5. Improve efficiency to reduce data costs and scale
The amount of data isn’t the only thing growing at an unprecedented rate, the costs to store and process it are too. In particular, AI projects require significant computing power and data storage — and unchecked, escalating costs can quickly strain budgets and limit innovation. To extract the most value from gen AI investments, organizations will need to balance capitalizing on their data while keeping tight control over costs and resource demands.
We have several best practices we like to focus on when working with customers to help them reduce data costs and scale, including automating workloads with managed services, optimizing storage and compute, refining data ingestion and extraction processes, and adopting cloud FinOps. Managed cloud services and serverless platforms, for example, can minimize overhead and costs by eliminating routine database management and maintenance tasks while providing flexibility to scale infrastructure up and down quickly to meet changing demands. Ford Motor Company experienced a significant drop in the time its teams spent on operational database tasks and a 30% performance boost after migrating a large portion of its database estate to Google Cloud managed services.
Ultimately, AI is only as good as the data that fuels it — and organizations will need to focus on building a robust, scalable, and unified data foundation that allows their gen AI initiatives to thrive and empowers data teams to accelerate AI innovation.
To dive deeper into each of these steps and discover detailed insights about how Google Cloud can help get your data AI-ready, read the full guide. If you want to unlock the next steps and expert recommendations to fuel your organization’s AI-powered data transformation, check your AI-readiness with this data and AI strategy assessment.