How to build an effective AI strategy

Raymond Peng
Senior Principal, AI Value Creation, Google Cloud
Amy Liu
Head of AI Solutions, Value Creation
Leading organizations are establishing a clear vision, strategically prioritizing use cases, and rigorously measuring results to maximize AI's impact.
A year ago, generative AI was still more dream than reality, with most organizations cautiously dipping their toes into its potential. Today, we face a very different landscape: More than 60% of organizations — a more than 4X increase in the last 12 months — now have gen AI use cases in production, according to a recent study from the National Research Group, commissioned by Google Cloud. Not only that, two out of three organizations have plans to allocate more than 50% of future AI budgets to gen AI initiatives.
It’s clear the race to capture gen AI potential is officially on. But while some companies are already making incredible breakthroughs bringing gen AI to real-world applications, others have yet to take the leap to invest. The new global research, based on interviews with 2,500 C-suite leaders from large global enterprises, delves into how organizations are harnessing gen AI to deliver tangible return on investment (ROI) and, more critically, the specific factors helping them achieve this success.
In particular, the study emphasized that a comprehensive AI strategy is vital for driving business value faster — or, at the very least, ahead of the competition. While only 35% of companies currently say they have an AI strategy in place, those that do, see a dramatic impact faster, with the large majority (78%) already seeing ROI from gen AI.
So, what does an effective AI strategy actually look like?
Based on our decades of experience pioneering AI innovation, both for ourselves and our customers, we have found that winning AI strategies anchor around a clear AI vision, focused implementation of the right use cases, and consistent tracking of results. For executives currently in the midst of developing their own AI strategies, incorporating these three core fundamentals can help map out a clear path to long-term, sustainable success.
1. Create a clear and compelling vision for AI
The foundation of a robust AI strategy lies in the ability to create a holistic view of AI within the context of your organization. AI initiatives shouldn’t be treated as standalone projects, but tightly integrated as part of your overall corporate strategy. Achieving this perspective requires a different operational model, in which IT and business work more closely together.
At Google Cloud, we often take a top-down and bottom-up approach, which allows us to combine high-level strategy with tactical technology use cases. This dual-pronged approach makes it easier to align gen AI implementations with broader strategic objectives while gaining insights about real challenges from the relevant teams and stakeholders that will benefit most from them.
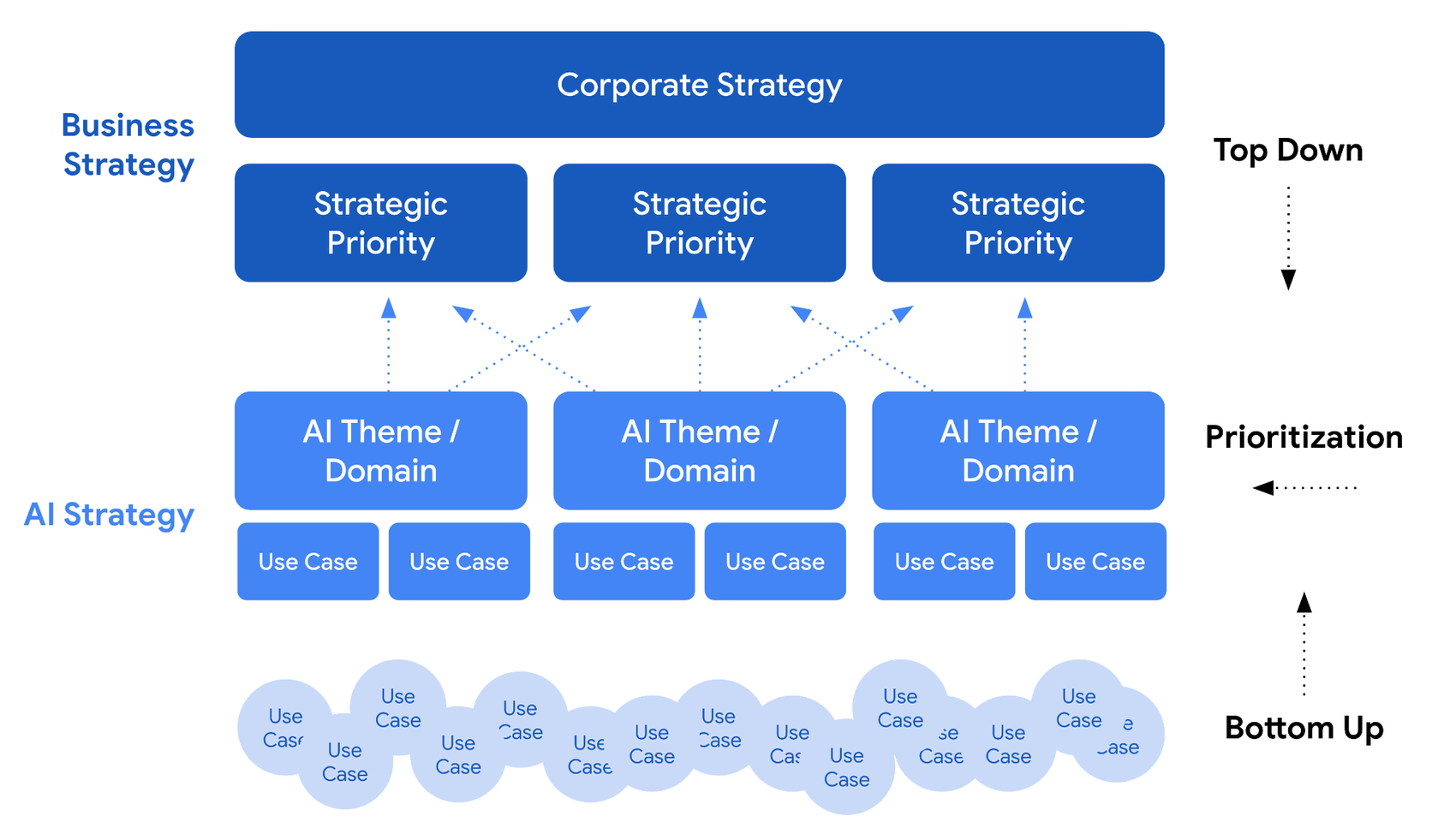
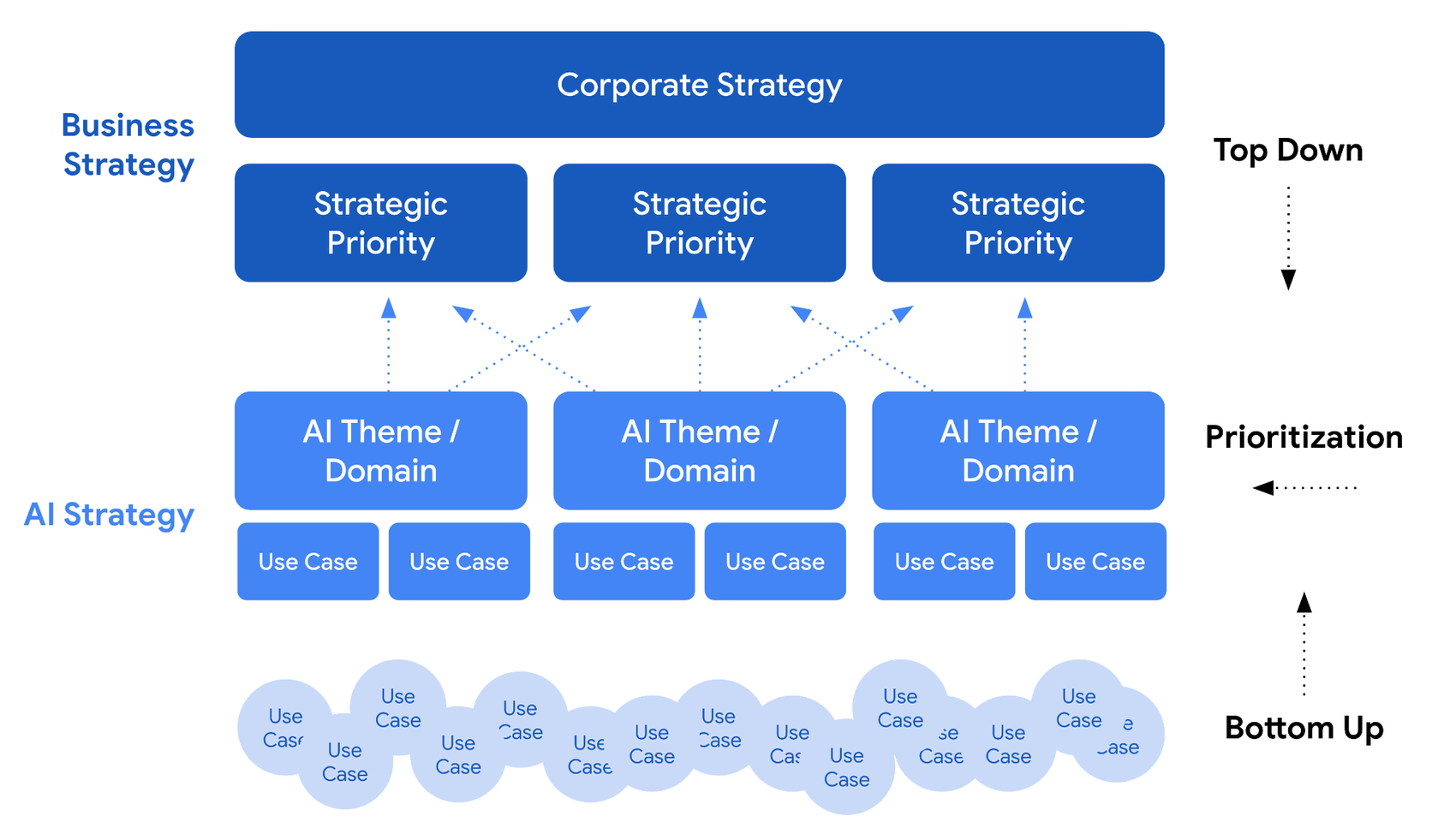
Connecting strategic priorities from the overall business strategy to specific AI domains
From a top-down perspective, we start by connecting strategic priorities from the overall business strategy to specific AI domains — key areas where we can focus investment. These domains can be a department, a core product, or even an end-to-end process, such as a customer journey or a contact center workflow.
At the same time, we recommend that leadership gather feedback from teams on the ground to crowdsource ideas and get a better understanding of the concrete issues and roadblocks they encounter when working. Organizations have sent submission forms across the company, hosted hackathons with Google Cloud, or attended our Cloud Briefing sessions on AI to collect the latest use cases and ideas from across the industry and see how Google is leveraging AI internally. Big picture strategy tends to overlook the finer details of daily operations, which is crucial for identifying the right AI opportunities and managing change.
The reason we emphasize domains is that a single AI implementation isn’t likely to move the financial needle on its own, especially if it’s adopted in isolation. The most significant impact typically comes from multiple use cases that can work together to reimagine the entire chain of value, such as revamping how customers place fast food orders to the way baseball fans watch games — or even how scientists explore novel compounds and combinations.
2. Prioritize the right use cases
One of the biggest challenges we frequently hear in our conversations about building AI strategies is figuring out the use cases that hold the most potential and prioritizing them to drive the biggest impact.
While most organizations have made strong financial commitments towards gen AI, they often struggle to determine where to invest first. Gen AI’s versatility is both a blessing and a curse; there’s a vast range of applications, but it can often be hard to determine which ones provide the most significant value. Not only that, organizations must also assess a variety of other factors, such as technical complexity, data readiness, stakeholder buy-in, and alignment with broader strategic objectives.
When prioritizing gen AI use cases, we find it helpful to use a simple matrix to plot out each potential use case based on the expected value generation vs. the estimated actionability and feasibility of that use case.
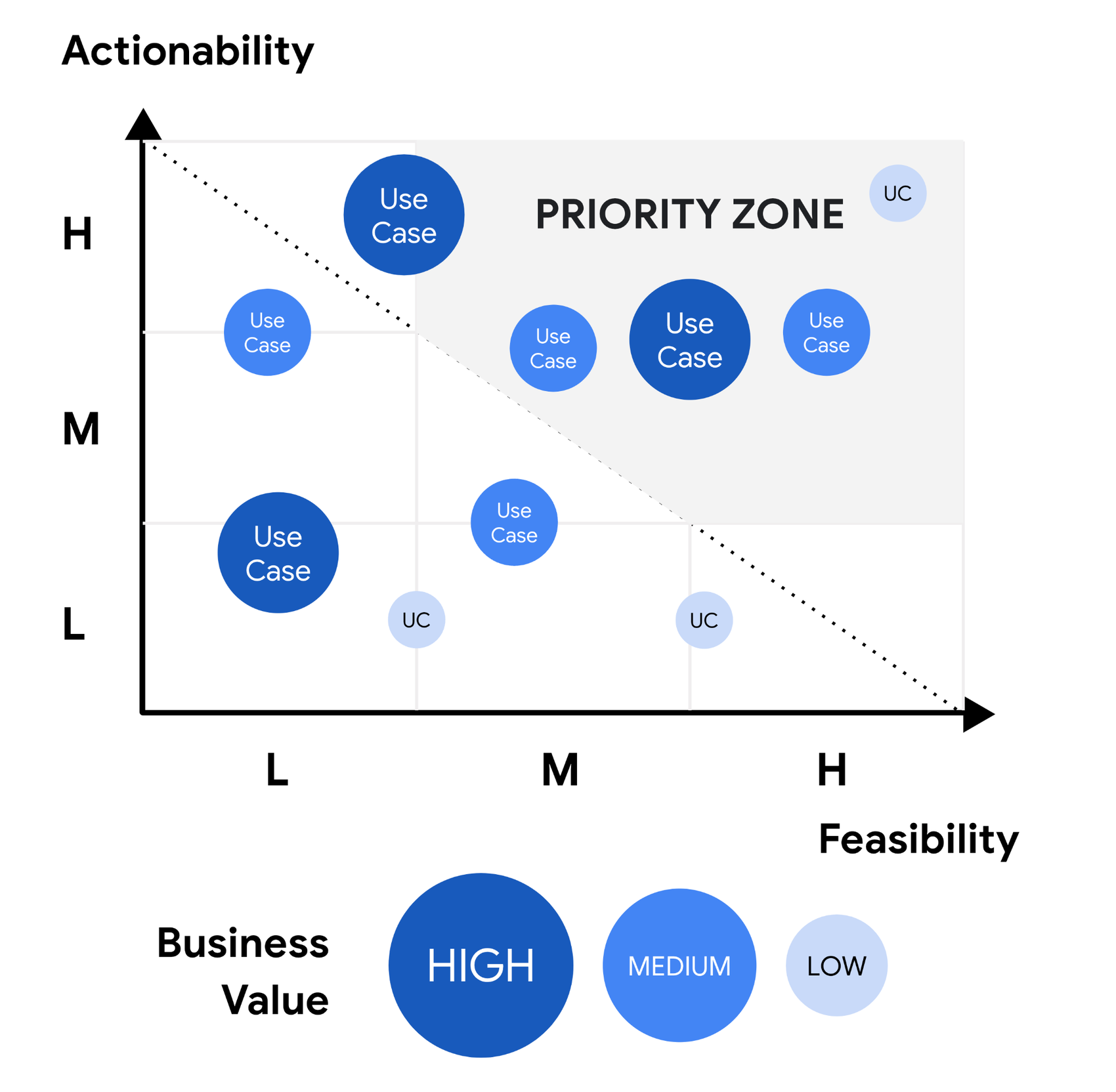
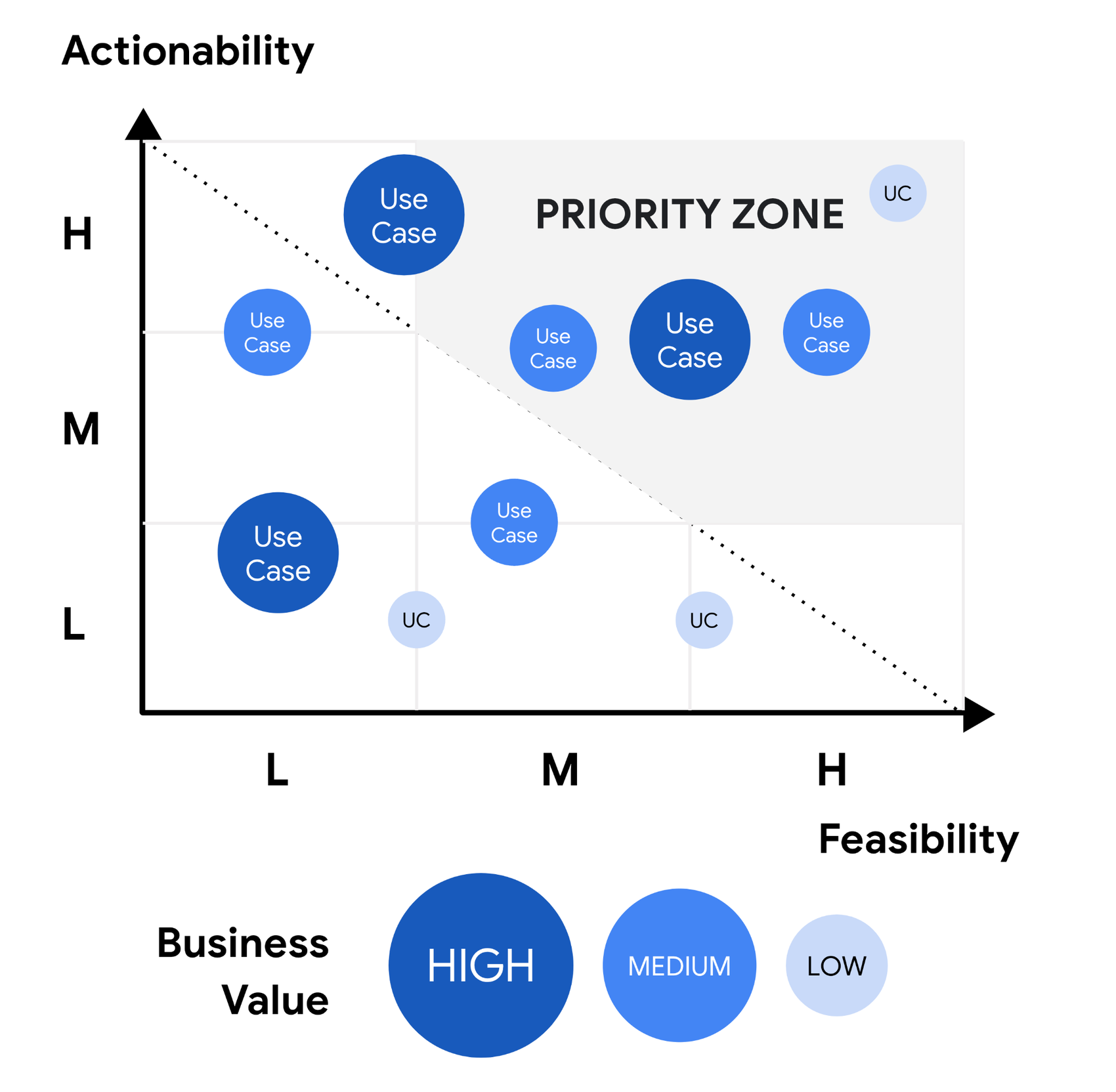
A simple matrix to plot out each potential use case based on the expected value generation vs. the estimated actionability and feasibility of that use case.
Here are some examples of questions you can ask to map out your own use cases:
Business Value
- Impact: Will this move the needle for our customer and employee needs?
- Alignment: How does this support our top business objectives?
- Reuse / extensibility: Can we reuse and build on it for future use cases?
Actionability
- Usability: How accurate does AI have to be to make it truly useful?
- Adoption: How easily can we adapt this into our existing processes?
- Speed: How quickly can we drive value for our organization?
Feasibility
- Technical fit: Is AI actually the right fit for this use case?
- Data readiness: How accessible and trustworthy is the data for this use case?
- Risk tolerance: Are there any implications of inaccuracy or misuse?
Overall, using a matrix like the one above simplifies the process of understanding all the possibilities for AI and clearly visualizes the different tradeoffs between all your different use cases.
For instance, this prioritization process helped us define a clear and actionable AI roadmap for one global financial services customer. With both retail and commercial customers, AI represented a huge opportunity with a long list of potential use cases, from improving front-office customer experiences to streamlining back office operations, such as data and document processing.
Using our matrix, we helped the company hone in on the area where AI could have the most impact — its customer contact center, which fields a massive volume of calls every day. Implementing virtual AI agents to help answer commonly asked questions and route customers offered high business value by reducing contact center operating costs across thousands of agents. At the same time, using Google Cloud AI to build these virtual agents offered a low-friction and highly feasible replacement, accelerating adoption and time to realize value.
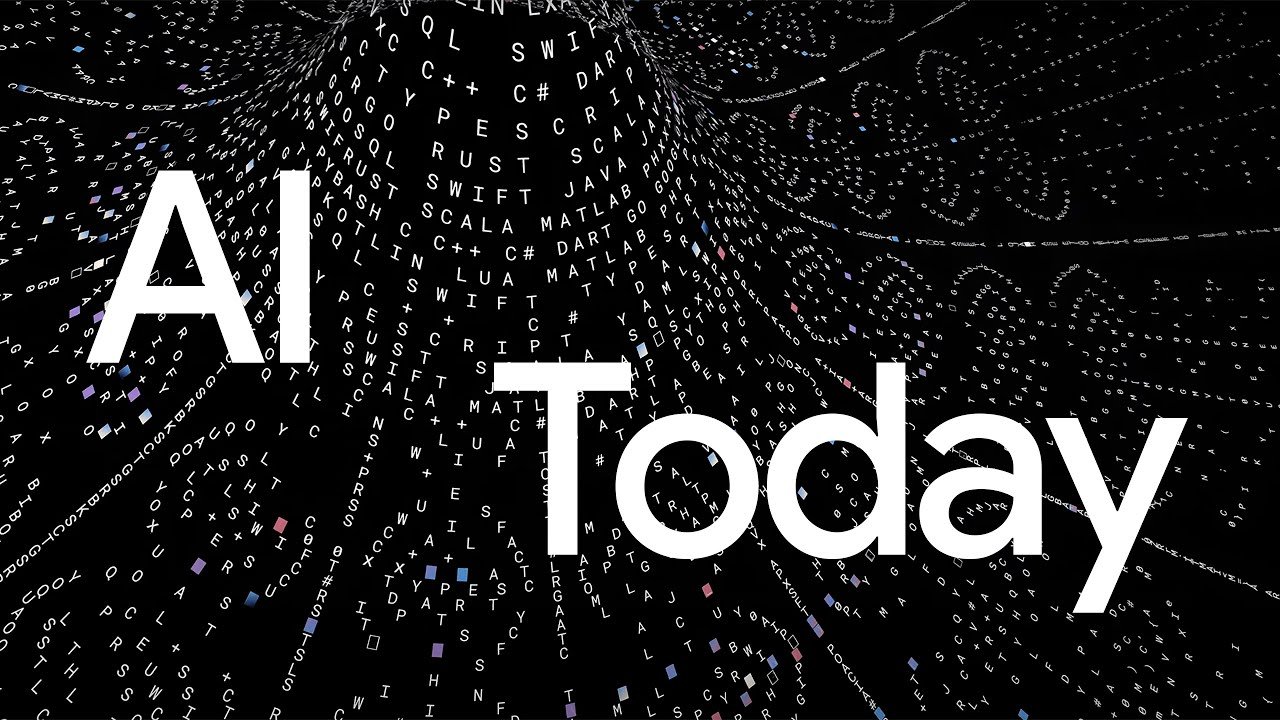
As a second priority, the company identified use cases that empower clients to access their financial data without database query skills, receive personalized financial advice with safeguards, and streamline document processing. While high on value, these use cases were more complex, requiring more data integration, operational workflow changes, risk management processes, and stakeholder alignment, delaying their ability to realize value quickly.
3. Measure your gen AI progress
Whether piloting a use case or launching it in production, organizations also need to be sure that AI strategies are actually delivering on their promised outcomes. Just like any other initiatives, evaluating the performance of gen AI is essential to its success.
To fully understand the business value of gen AI, it’s necessary to measure the entire lifecycle of each use case — from pilot to launch and beyond — and continuously optimize and make improvements. Here, key performance indicators (KPIs) are critical for helping you to objectively assess performance, align strategy with business goals, demonstrate tangible returns, and make data-driven adjustments. Rigorously measuring impact can also help drive accountability within leadership across the organization and provide valuable insights for prioritizing future use cases.
At Google Cloud, we recommend measuring AI across five key areas:
- Model quality metrics: These metrics help evaluate and monitor the accuracy, factuality, reliability, and security of your AI models. They help set realistic expectations in relation to the end goal while supporting responsible AI principles and practices.
- System metrics: It’s crucial to ensure your AI systems run efficiently, reliably, and at scale to support the needs of your organization. These metrics help to monitor the health and performance of your AI platforms and infrastructure. They can reveal bottlenecks and uncover areas for compute cost and performance optimization.
- Adoption metrics: AI adoption is not an overnight process, so it’s critical to have a strong understanding of how users interact with new gen AI tools. These metrics provide insights into adoption, usage frequency, and other types of qualitative user feedback.
- Operational metrics: To determine the ROI of your AI investments, you’ll need to understand how they impact your business processes. These metrics help you measure whether AI features and capabilities are delivering downstream returns such as click-throughs and call containments.
- Business Impact: Finally, these financial metrics provide visibility into whether you’re meeting your strategic goals, such as increasing revenue, reducing costs, mitigating risk, or accelerating innovation in key areas in relation to your investments.
By segmenting your KPIs across these five areas, you can gain a comprehensive understanding of gen AI value while gaining deep insights into both the technical and business impact of your investments.
Lastly, it’s important to establish a baseline of current metrics today, so that you can compare the results of AI once it has been released. This might require a period of pre-launch measurement or strategy to A/B test your production AI use cases against results for the same process without AI.
In our work with many customers, consistent practices and efforts devoted to measurement is one of the top concerns for leaders. A best practice is to develop this measurement plan during the design phase of the use case and not treat it as an afterthought that is bolted on during implementation.
Unlock the full potential of your gen AI investments
AI strategies need to ensure these new capabilities map to strategic objectives and business requirements. In addition, AI strategies are about more than deploying and scaling AI technologies and infrastructure; they also need to address people, process and organizational factors. Without this context, gen AI initiatives, however impressive, have little chance of meeting expectations and reaching their full potential.
AI is a transformative opportunity, uniquely positioned to create unprecedented areas of organizational value, but it will require crafting the right strategy to make the most of it. For leaders and executives looking to seize the moment, understanding the core fundamental outline above will enable you to craft a thoughtful and comprehensive strategy that will lay the foundation for your AI initiatives to thrive — both today and into the future.