The Prompt: Prototype to production
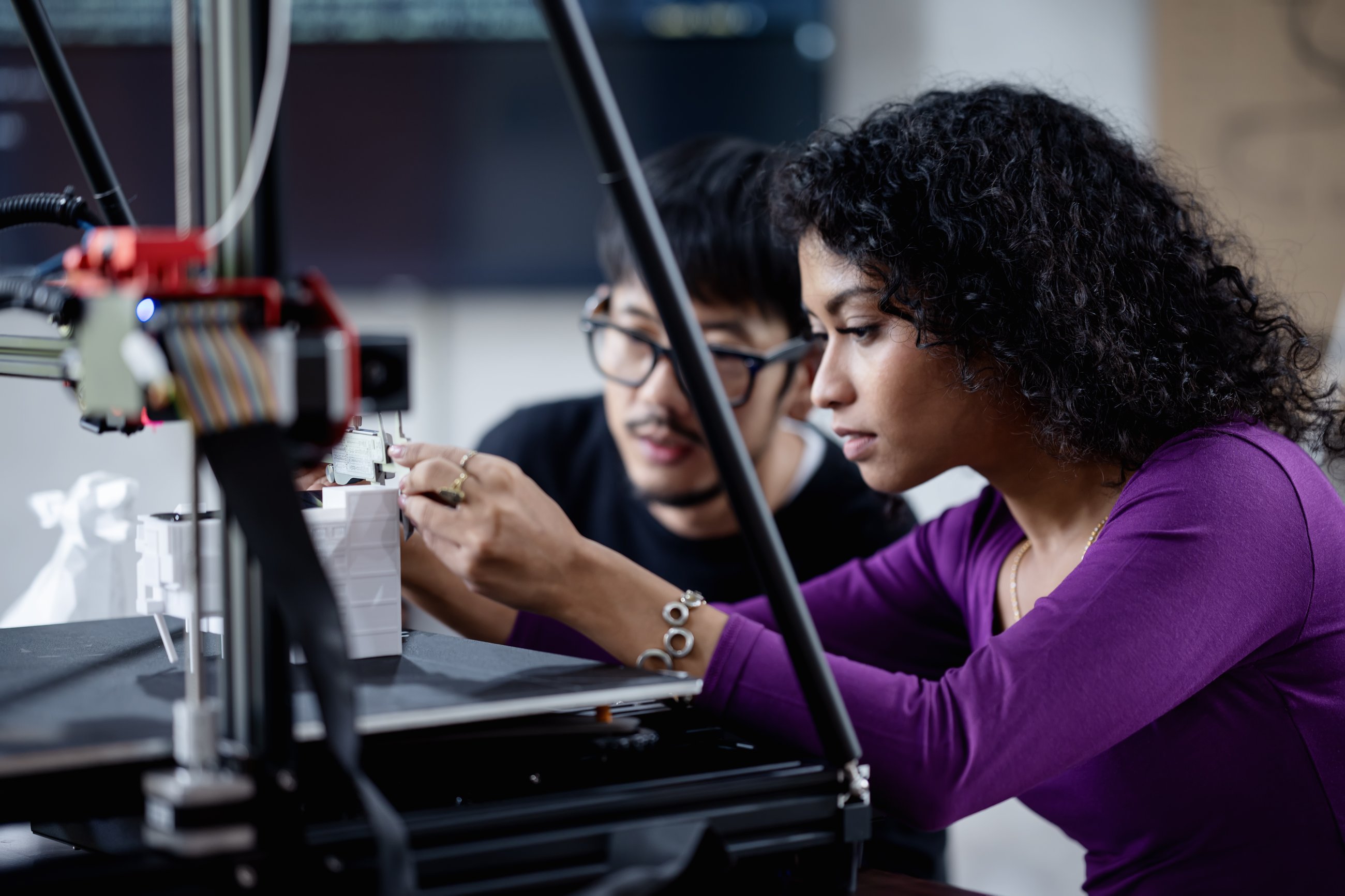
Warren Barkley
Sr. Director of Product Management
Generative AI is transforming industries, but scaling it requires strategic planning. Key considerations include platform selection, performance measurement, and responsible AI practices.
Business leaders are buzzing about generative AI. To help you keep up with this fast-moving, transformative topic, our regular column “The Prompt” brings you observations from the field, where Google Cloud leaders are working closely with customers and partners to define the future of AI. In this edition, Warren Barkley, AI product leader, shares some best practices and essential guidance to help organizations successfully move AI pilots to production.
A few years ago, generative AI was still just a whisper in the tech world. Today, it seems to be everywhere you turn, reshaping entire industries and evolving faster than we can imagine.
More than 60% of enterprises are now actively using gen AI in production, helping to boost productivity and business growth, bolster security, and improve user experiences. In the last year alone, we witnessed a staggering 36x increase in Gemini API usage and a nearly 5x increase of Imagen API usage on Vertex AI — clear evidence that our customers are making the move towards bringing gen AI to their real-world applications.
But many organizations are facing a hard truth: Extracting value from gen AI’s powerful capabilities isn’t as simple as typing in a query and getting a response. It requires identifying the business problems you’d like to solve with gen AI, prioritizing your top use cases, and putting together a comprehensive AI strategy. More specifically, many leaders are struggling to manage the complexities of integrating and deploying gen AI tools in ways that drive innovation and creativity while also balancing the privacy, security, control, and compliance needs demanded by enterprise environments.
To help navigate this challenge, we recently created a new ebook, “Gen AI: From prototype to production.” Drawing on two decades of experience operationalizing AI at scale and insights from our work with customers, this guide outlines steps to follow and best practices that we have seen help organizations move past AI experimentation successfully. With this in mind, I wanted to share three key insights from the ebook about scaling gen AI successfully that can help as you start putting your own use cases into production.
1. The right platform (and model) can make all the difference.
As we’ve previously discussed, you should invest in an AI platform, not just models. Choosing a gen AI model is rarely a one-and-done exercise; you’ll most likely need to update your model, upgrade the model version, or even change to a different model entirely as your business evolves. Some use cases might require employing multiple models to optimize performance and cost.
AI platforms provide access to the critical tools and capabilities needed to safely develop, deploy, and manage AI systems at scale, serving as a strong foundation for any AI initiative — not just gen AI. Therefore, organizations should not only evaluate different models but also carefully consider the platform capabilities they will need to achieve their goals throughout the selection process.
For example, you might look at whether a provider offers infrastructure that matches your given requirements, whether models can meet a wide range of needs and budgets, the different modalities and sizes available, and the resources available to customize, fine-tune, or switch between models.
In addition, understanding different types of gen AI models is critical for driving value and innovation with gen AI. When selecting models for testing and evaluation, you’ll need to weigh the strengths and trade-offs of various models against the specific requirements of your organization, including your unique use case, data and model governance, performance factors, and other capabilities like context windows, training datasets, multimodality, the number of model parameters, and more.
Many of our customers have found it helpful to start with a large proprietary model to get familiar with its capabilities and understand its inputs and outputs. Using foundation models as a starting point can allow you to build safe and high-quality applications fast without having to navigate implementing smaller or open models, which often require additional guardrails. From there, you can develop a better understanding of what your business needs from gen AI models, such as lower costs, faster response times, or more specialized domain knowledge.
2. You can’t improve what you don’t measure.
Optimizing gen AI models to ensure they are reliable and accurate enough to support enterprise use cases is one of the biggest hurdles organizations have to overcome when operationalizing AI systems. Gen AI models can produce different responses even when given the same input prompt — so it’s critical to develop a gen AI evaluation framework right from the start, including metrics and evaluation capabilities, to observe and monitor your gen AI models.
As you go beyond playing with and testing ideas, you should set key performance indicators (KPIs) for gen AI that can help you measure the quality, safety, and performance of your models. These metrics are key for identifying what areas in your AI system need improvement and tracking your progress to gain a clear picture of how your models are doing.
In particular, you will need to use different metrics to evaluate a wide range of possible inputs and scenarios that a model might encounter once it goes live. For example, summarization, Q&A, and content generation use cases will all require different KPIs — and these criteria will also likely vary across individual companies.
In general, metrics can help you determine what steps to take so your gen AI tools and systems can deliver towards your overall strategic objectives. Improving latency and costs, for instance, often requires changing your model and revisiting model selection. If you’re looking to refine model response, you’ll need to adapt model behavior through customization or augmentation, such as prompt optimization, fine-tuning, or retrieval augmented generation (RAG).
3. Responsible AI is at the heart of every gen AI journey.
Governance, safety, fairness, and equitable opportunities are not a step along the path from AI prototype to real-world application – these are core best practices that should be constantly upheld by model providers and organizations alike. In addition to establishing effective processes for governance and understanding the unique security risks associated with AI systems, you should endeavor to empower your organization to build and use gen AI, safely and responsibly.
To do this, you will need to address ethical considerations, legal compliance, and ensure the overall safety of your gen AI systems throughout the process of releasing, validating, and deploying your gen AI applications.
Specifically, gen AI introduces some unique vulnerabilities which can impact the overall safety of your systems, including:
- Hallucinations: When gen AI models lack factual real-world knowledge or accurate understanding of topics, they can hallucinate — generate outputs that sound plausible but are incorrect, irrelevant, inappropriate, or completely made up.
- Prompt injection and jailbreaking: Gen AI systems are vulnerable to new techniques that use malicious prompts or overrides to manipulate models into generating unintended outputs, altering their intended behavior or underlying logic, or exposing sensitive data.
- Training data poisoning: The training data that gen AI and other types of AI use to generate and make decisions can be contaminated with the aim of compromising the integrity, accuracy, and reliability of a model’s behavior and its outputs.
Your teams will need access to responsible AI tooling and AI-protection capabilities to identify, assess, and mitigate risks within your use cases and applications, such as tools for data preparation, content moderation, model safety, citation filtering, explainability and bias, and more. For instance, Model Armor enables users to configure policies and set up filters to protect gen AI model prompts and responses against a wide range of security and potential content safety violations.
Conducting regular product and use case reviews is another measure that can help you mitigate negative impacts before they are released. Furthermore, encouraging your teams to provide the latest educational resources, research, and best practices can help ensure everyone — from developers to data scientists and analysts to business users — understand the latest AI technologies, how to use them, and their associated risks.
Overall, building and deploying gen AI applications and systems is an iterative process that not only demands ongoing model measurement, evaluation, and refinement but should also incorporate responsible AI practices throughout the product development lifecycle.
Take your projects from prototype to production
Gen AI holds enormous potential for businesses worldwide, but to realize it, organizations must be prepared for what it actually takes to put models in production. It can feel overwhelming when you’re starting out, but it doesn’t have to be. Hopefully, the insights I’ve shared above have given you more clarity about how to tackle the challenges of building with gen AI.
I also recommend reading the entire guide to learn how to:
- Clarify your AI objectives.
- Choose the right gen AI model for your use case.
- Evaluate and improve model behavior over time.
- Release, validate, and deploy gen AI models.
- Monitor and maintain gen AI in production, effectively.
You can access the full ebook for more details on transitioning from gen AI experimentation to production, and find even more insights about how organizations are leveraging gen AI in our 2025 AI Trends Report.