Here are 5 gen AI security terms busy business leaders should know
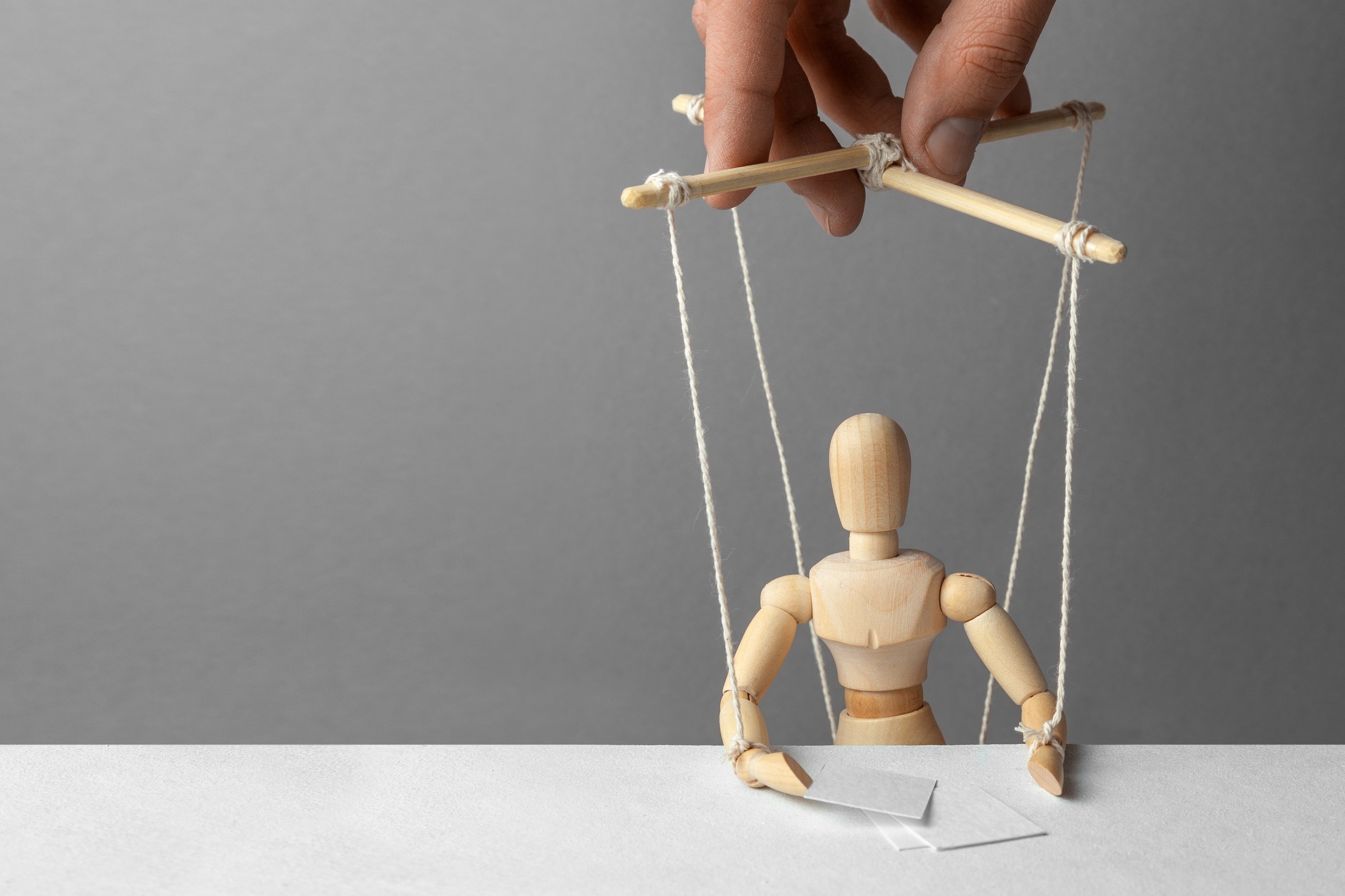
Anton Chuvakin
Security Advisor, Office of the CISO, Google Cloud
Seth Rosenblatt
Security Editor, Google Cloud
Know your data poisoning from data leakage? Check out our gen AI security primer
Hear monthly from our Cloud CISO in your inbox
Get the latest on security from Cloud CISO Phil Venables.
SubscribeDo you worry that your bespoke AI foundation model is plagued by hallucinations? Is your security team defending against model theft? As generative AI has begun to proliferate across businesses and industries, the new technology has brought its own hip lingo to security teams.
Generative AI is new and complex, powerful and developing fast, a rare combination which means that it’s also strategically urgent for business and security leaders to stay on top of developments.
It’s important for organizations to focus on this now. AI is here to stay, and we have to start addressing it.
David Bailey, vice-president of Consulting Services, Clearwater
While AI has almost suddenly commanded the pole position in IT conversations, its use still raises significant questions, according to an ISMG report conducted during the third quarter of 2023 and commissioned by Google, Microsoft, Clearwater, Exabeam, and OneTrust. Respondents said that their top concerns involved sensitive data leaks, according to 80% of business leaders and 82% of cybersecurity professionals. The risk of inaccurate data, especially hallucinations, was cited by 71% of business leaders and 67% of cybersecurity professionals.
Importantly, business and cybersecurity leaders indicated they were cautious about AI adoption. The 73% of business leaders and 78% of cybersecurity professionals who say they are planning to take either a walled garden or build-your-own approach to AI are joined by 38% of business leaders and 48% of cybersecurity leaders, who say that they expect their organizations to continue banning all use of generative AI in the workplace.
“Organizations that are not in front of this train will get run over by the train, and it’s important for organizations to focus on this now. AI is here to stay, and we have to start addressing it,” said David Bailey, vice-president of Consulting Services, Clearwater.
To help demystify some of the AI lingo, below, we highlight five important terms at the intersection of AI, security, and risk that can help you better understand ongoing AI developments — and what you can do about them.
Real risk of prompt abuse
- Prompt manipulation is a foundation model input risk that can be exploited when the attacker uses prompt design, prompt engineering, or prompt injection to force an unintended response by the foundation model. Prompt manipulation can occur intentionally, such as when a threat actor uses it to reveal sensitive data, or it can be accidental, such as when a benign prompt input generates an output of sensitive data.
How it affects your business: Prompt manipulation can harm organizations in several ways. It can damage the reputation of an organization by producing text that is offensive, discriminatory, or promotes misinformation, potentially affecting stock prices and financial markets; it can aid attackers in extracting sensitive data and running malicious code within a system that uses the foundation model; and it can disrupt customer service with biased chatbots and create workflow errors, especially in summarization tasks.
Reduce the risk: There are several techniques that organizations can use to reduce the risk of prompt manipulation. Sanitize and analyze prompts before allowing them to be processed, and review and monitor generated outputs. It’s crucial to have procedures in place that can flag and remediate potentially harmful content. Meanwhile, foundation models should be kept current with security patches and known threat information, and employees who interact with the models should be educated about prompt manipulation and potential risks.
Plug leaks to better secure sensitive data
- Data leakage occurs when the model reveals sensitive information in outputs that was never intended to be part of an output response. It can result in output inaccuracies as well as unauthorized access to sensitive data. Last year, researchers found that they could force a popular generative AI-assisted chatbot to repeat the word “poem” and subsequently force it to cough up sensitive data unintentionally.
How it affects your business: Data leakage can lead to privacy violations; the loss of trade secrets and intellectual property; an increased risk of security breaches aided by the leaked data; and financial penalties from lawsuits and regulatory fines related to these incidents.
Reduce the risk: Preventing data leakage from foundation models is an ongoing process that will likely evolve along with potential threats. Strict data governance policies, access controls and monitoring, advanced security measures, and regular risk assessments are techniques that can be layered to build a robust AI risk management practice.
Protect sensitive, labor-intensive model design
- Model theft poses a serious risk because AI models often include sensitive intellectual property. Organizations that have developed their own models should place a high priority on protecting these assets. During a simple exfiltration attack, an attacker could copy the file representation of the model. Attackers with more resources could deploy more advanced attacks, such as querying a specific model to determine its capabilities and using that information to generate their own.
How it affects your business: Most clearly, model theft can lead to financial losses because creating and training effective foundation models requires specialized knowledge, computing resources, and a significant time investment. It also can incur reputational damages, increase the risk of security breaches, disrupt innovation, and lead to costly lawsuits if the model winds up in a competitor’s hands.
Reduce the risk: Similar to data leakage risk management, organizations can reduce the risk of model theft with output filtering, access controls, network security techniques, monitoring for anomalous use, watermarking, and legal safeguards.
Stronger data controls are the antidote
- Data poisoning exploits the risk of improperly secured training data. The attacker manipulates the model’s training data to maliciously influence the model’s output. Training data can be poisoned across in the development pipeline.
For example, an attacker can store poisoned data on the Internet and wait for it to be scraped by the model, if the model is trained on Internet data. An attacker who has access to the training or fine-tuning corpus could store poisoned data there, similar to a backdoor in the model that uses specific triggers to influence the model’s output.
Research suggests that attackers need to control only 0.01% of the model’s dataset to poison it, which indicates that Internet-derived datasets don’t require an attacker to be well-resourced to achieve their goal.
How it affects your business: Similar to data leakage, data poisoning can negatively impact an organization’s reputation, lead to financial losses, increase the risk of security breaches and legal liability, and even disrupt daily operations if the poisoned foundation model has been integrated into the organization’s internal processes.
Reduce the risk: Organizations can prevent data poisoning by carefully vetting data sources, implementing strict data controls, and continuously monitoring incoming data. Data filtering and cleaning processes can help remove biases and potentially harmful content, as can training the model with adversarial training techniques to make it more resilient to data poisoning attacks.
Keep your foundation model grounded
- Hallucinations occur when an artificial intelligence model generates responses that are factually incorrect, nonsensical, or completely fabricated. They can occur for many reasons, including not enough and biased training data; models that adhere too closely to their training data and therefore can’t extrapolate accurately; inaccurate contextual analysis; poorly designed reinforcement models within the foundational model; and threat actors who inject malicious inputs designed to induce inaccurate responses to otherwise benign queries.
How it affects your business: While the term may seem glib, the consequences of foundation model hallucinations are hardly a cozy trip. They can cause reputation damage, especially through the spread of false information; increase legal and regulatory liabilities including costly compliance violations; and create operational inefficiencies that drive up costs.
Reduce the risk: Like any technology, foundation models are not perfect and hallucinations may occur. A good place to start is with grounding, when a model is designed to retrieve information from a trusted source first and then create a prompt for the generative model. Organizations also should have processes in place to fact-check and verify model-generated content, especially high-risk outputs. Bias mitigation techniques should be supplemented with human oversight, especially for outputs that rely on mission-critical or potentially sensitive data.
Pursue AI boldly and responsibly
Responsible AI practices are critical for navigating the emerging risks that accompany the technology’s growing use. At Google Cloud, we take a multi-pronged approach, with AI products built through responsible practices that guide us from development to deployment. We responsibly curate datasets for large models to address downstream concerns including hate speech, racial bias, and toxicity.
During training, we stress-test models on scenarios that have the potential to produce harmful results and adjust the model to mitigate these risks. In deployment, we give customers the tools they need to use AI responsibly, including working with customers to create and scale responsible AI frameworks and assessments. For example, we provide safety filters for customers to address concerns about harmful content, including bias and toxicity.
To learn more about how to build foundation models and use gen AI to support your organization, read our blog on enterprise readiness in the age of generative AI.