The Prompt: Let’s talk about recent AI missteps
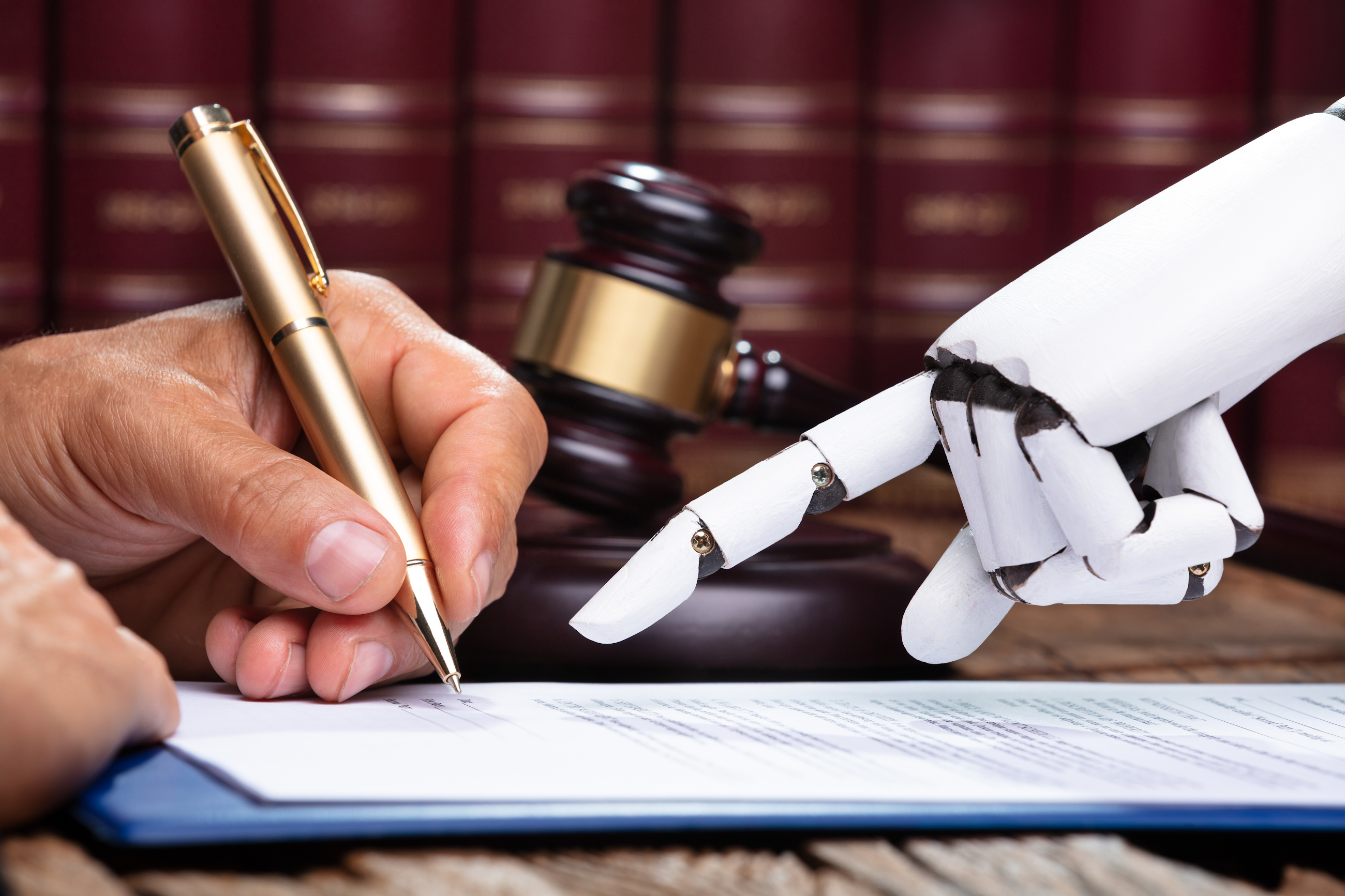
Philip Moyer
Global VP, AI & Business Solutions at Google Cloud
Business leaders are buzzing about generative AI. To help you keep up with this fast-moving, transformative topic, each week in “The Prompt,” we’ll bring you observations from our work with customers and partners, as well as the newest AI happenings at Google. In this edition, Philip Moyer, Global VP, AI & Business Solutions at Google Cloud, discusses reports of recent AI-related mishaps.
An unfortunate New York lawyer was recently in the news for submitting a court brief built on fictional case citations conjured up by a generative AI assistant. I don’t say this to criticize the AI solution or the lawyer, who faces potential sanctions but also made an all-too-common mistake. Rather, I want to discuss this because it’s an all-too-common mistake.
By now, most of us have heard about “hallucinations,” which are when a generative AI model outputs nonsense or invented information in response to a prompt. You’ve probably also heard about companies accidentally exposing proprietary information to AI assistants without first verifying that interactions won’t be used to further train models. This oversight could potentially expose private information to anyone in the world using the assistant, and as we discussed in earlier editions of “The Prompt,” these risks have compelled some companies to ban use of consumer AI apps across the workforce.
Hallucinations and inadequate data security are two of the biggest potential stumbling blocks in generative AI adoption. The New York attorney — who told a reprimanding judge he was unaware the AI assistant’s outputs could be false — hit at least the first stumbling block, and if his prompts included any client information, he might have hit the second as well. There are several important lessons here to help others avoid these missteps and adopt generative AI successfully.
Never forget the human in the loop
Make no mistake, generative AI apps are better equipped to handle hallucinations today than they were even a few months ago. We discussed at Google I/O that all generative models pose this challenge, and that it’s important to build more robust safety layers before we deploy more capable models. Models, and the ways that generative AI apps leverage them, will continue to get better, and many methods for reducing hallucinations are available to organizations—more on this in a minute. But general-purpose generative AI assistants and apps are collaborators in most use cases—-ways to save time or find inspiration, but not opportunities for full, worry-free automation.
A person using AI often outperforms AI alone or humans alone, so the default assumptions for new generative AI use cases should include a “human in the loop” to steer, interpret, refine, and even reject AI outputs. If inaccurate AI outputs end up in final work products, that’s both a shortcoming in the AI tool and improper AI adoption by one or many humans.
Specialized industries may require specialized solutions
Specialized domains — especially those in which accuracy is crucial — increase the importance of both human oversight and choosing the AI tools appropriate to the task. General-purpose generative AI apps can do a lot, but they’re not designed for the demands of specific industries.
That doesn’t mean these apps are useless for lawyers, doctors, or similar professionals. Directional accuracy can be better than starting from scratch as long as users verify generative AI outputs before putting them into action, and it’s not outlandish to imagine an AI assistant outputting clever ideas if fed domain data, such as court precedents, and prompted for insights.
Still, these benefits aside, consumer-oriented apps probably aren’t ever going to offer enterprise-grade SLAs, and they certainly don’t now. Moreover, the more demanding the field, the more necessary specialized apps and models may become.
General-purpose models’ outputs are largely influenced by all the data in the training set. If that corpus includes not only references to the law or medicine, but also pop culture, literature, sports, world history, and billions of other topics, prompts are more likely to trigger strange mashups of probability within the model, resulting in hallucinations. Techniques for training and tuning general-purpose foundation models continue to improve, and the likelihood of problematic hallucinations is diminishing—but nonetheless, there probably won’t ever be one perfect model for all use cases.
That’s one reason why Google Cloud has invested in models fine-tuned for sensitive, highly-specific domains, like Med-PaLM 2 for healthcare and life sciences. And while Bard gets better every day as a general-purpose collaborator, we’ve also integrated generative AI in products to make models easier to use in specific contexts. In Google Workspace, for example, if the user highlights text in a Doc, options for “shorten,” “formalize,” “elaborate,” and “rephrase” automatically surface to make prompting easier and more effective. As foundation models and AI-enabled infrastructure continue to become more accessible to builders and innovators, we’re likely to see scores of industry-focused generative AI apps powered by both custom models and job-oriented design.
Adapting foundation models for enterprise use
For many organizations, custom apps may be the best way to handle sensitive data or use cases that demand higher accuracy. Approaches include grounding the generative AI app in specific data to limit hallucinations and focus responses on relevant information, like our customers can do with Enterprise Search in Generative AI App Builder, or they can fine-tune foundation models or even create their own, like our customers do with Generative AI support in Vertex AI—all with data sovereignty, security, and governance built in. These efforts don’t eliminate the need for humans in the loop once a generative AI app is deployed—but they can make the human’s life much easier by offering more precise, personalized, and relevant experiences, including citations to speed up verification.