The Prompt: Invest in AI platforms, not just models + a recap of Google I/O
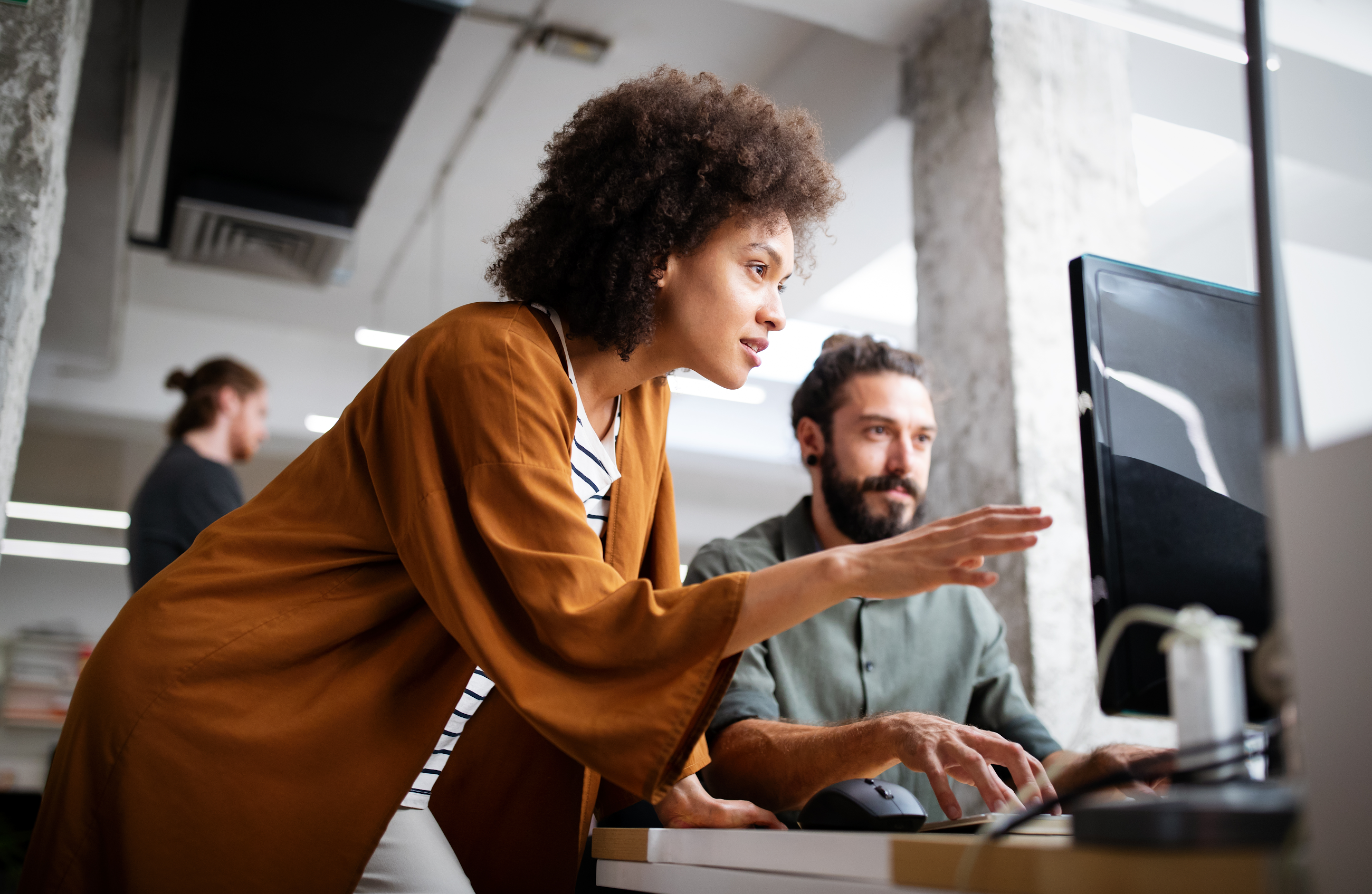
Philip Moyer
Global VP, AI & Business Solutions at Google Cloud
Stay up to speed on transformative trends in generative AI
Business leaders are buzzing about generative AI. To help you keep up with this fast-moving, transformative topic, each week in “The Prompt,” we’ll bring you observations from our work with customers and partners, as well as the newest AI happenings at Google. In this edition, Philip Moyer, Global VP, AI & Business Solutions at Google Cloud, reviews Google I/O 2023 and discusses how organizations can combine their data with generative AI.
We just finished Google I/O 2023, and I’m still feeling the rush! Some of the standout moments involved foundation models, led by the debut of PaLM 2 — our most advanced model in production and the AI engine powering dozens of Google products — as well as expanded access in Vertex AI to not only PaLM 2’s text and chat capabilities, but also new foundation models for image generation, code generation, and speech.
All the excitement around models leads to my topic this week: combining enterprise data with generative models. Every executive I’ve talked with agrees that generative AI models are impressive, but they’re also cautious because “impressive” isn’t the only criteria for enterprise-ready use.
For example, leaders are deeply concerned whether the outputs of generative AI foundation models will be reliable and accurate enough for their use cases. “Often useful, sometimes wrong” just won’t cut it in a lot of scenarios.
Moreover, leaders are also unsure whether methods to ensure reliability can be easily implemented or scaled, since even in large organizations, there are only so many data scientists and developers available for deep customization. Executives want to know how to get going fast with generative AI, and they aren’t interested in growing pains that can waste investments or damage their brands.
Thanks to these requirements and challenges, leaders increasingly realize that organizations need to invest in not only generative AI models, but also platforms that make models easy to use.
In this column, I’ll explore what these platform capabilities should entail and why strong models alone aren’t sufficient for enterprise adoption. As an example, we’ll focus on enterprise search, which is far and away the most widely applicable use case we discuss with executives across different industries.
Narrow the aperture to improve accuracy
As I wrote in an earlier edition of “The Prompt,” almost every business wants to combine their data with generative AI, letting employees ask questions and get actionable, reliable answers drawn from all the resources relevant to their job.
The possible use cases are endless: researchers investigating a collection of studies, store clerks helping customers, different teams collaborating and keeping everyone updated—basically any scenario in which people are interacting with information. But doing this isn’t per se simple. Let's go through what it means to combine proprietary data with generative AI.
For specialized use cases, organizations can update a foundation model’s training by fine-tuning on additional data. This can help ensure the model is suited for a domain with specific language and requirements, like Google has done with Med-PaLM 2 and Sec-PaLM.
That approach is not going to work for enterprise search, however. The information is updated far too often, and retraining is far too complicated and costly to keep up.
For this kind of frequently-changing data, organizations can limit the information the model considers. Rather than asking the model to devise answers from training data, they can direct it to specific sources that are constantly refreshed, like an internal repository or a public database. By narrowing the aperture, and focusing the model on specific information instead of every bit of data to which it’s ever been exposed, organizations can enjoy more accurate responses based on the most up-to-date data.
Get fresh answers with the right retrieval methods
Unfortunately, many methods for “focusing the model” are problematic. For example, some people simply paste the text of a document into a generative chat, then ask questions. This may be fine as a one-off but is not scalable. The model will lose context with longer documents or if a single large document is chunked across multiple prompts.
Organizations might connect a generative model to search APIs to help direct it toward the right data, but this approach can also be limited, depending on the search technologies involved. Keyword-based search, for example, will only find the most explicit connections across sources, limiting the model’s ability to find deeper insights or holistically synthesize sources.
A better approach is to connect the model to more powerful search and retrieval methods, like semantic search, which looks at multi-dimensional relationships within the data instead of just keywords. But yet again, this can be difficult. An IT team might use an embeddings API to map semantic relationships, but creating a custom pipeline across many constantly-updating sources not only adds toil and complexity, but also risks limiting future strategies, such as switching to newer foundation models. Especially for organizations looking to quickly begin leveraging generative AI, more user-friendly options are preferable.
AI is complex—but adopting it doesn’t need to be
A good AI platform should include not only access to powerful models, ways to narrow the data models consider, and advanced search techniques for retrieving that data, but also simple implementation that abstracts away complexity and lets companies get moving. That’s the message we’ve heard loud and clear talking to executives, and it’s what we’ve been building at Google to make adoption of generative AI easy, especially for widely-applicable use cases like enterprise search.
Products like Google Cloud’s Gen App Builder can create customized generative chat interfaces in minutes, for example, not just for enterprise search scenarios but also retail chatbots and a variety of other use cases, all with enterprise-grade governance, orchestration, and security built in. Customers can simply enter a public url or select from a list of available resources, then the product handles the rest, as the below session from I/O illustrates.
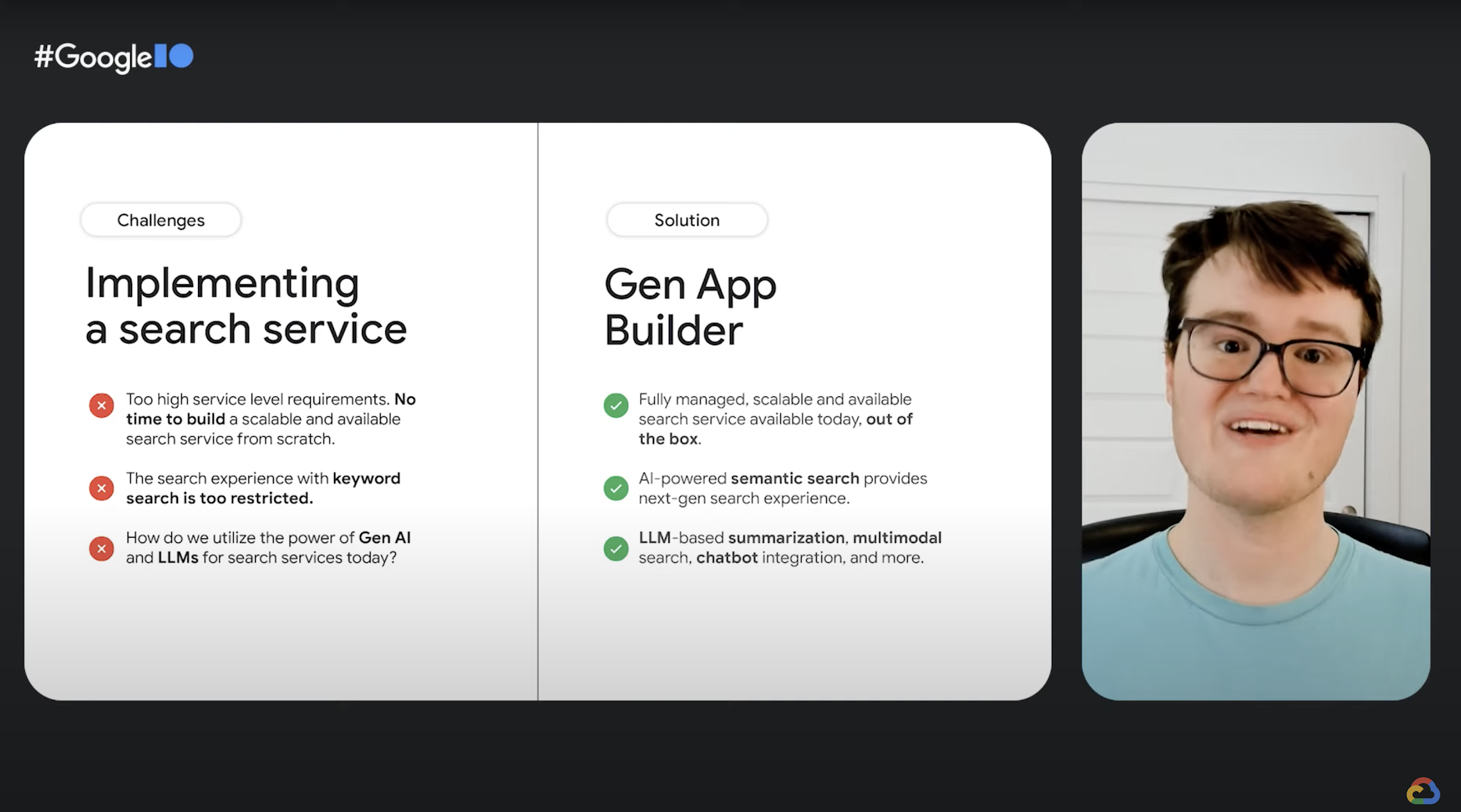
For more bespoke or sophisticated uses, we of course offer fine-tuning for models, Embeddings APIs, and more—and that’s the point: organizations need a spectrum of platform capabilities, not just great models.
This week in AI at Google
This was a huge week in Google AI, with a ton of incredible news throughout Google I/O.
Highlights included AI updates and announcements for Bard, Cloud, and Workspace; details about PaLM 2 and our in-development Gemini model; our vision to supercharge Search with generative AI; AI-powered features coming to Android, Maps, and Photos; and our commitment to investing in AI responsibly. You can check out the full keynote here— but these are some of the can’t miss details:
- Customer momentum: Google Cloud CEO Thomas Kurian shared innovative work from our generative AI and AI infrastructure customers, including Adore Me, Canva, Character.AI, Deutsche Bank, Instacart, Orange, Replit, Uber, and Wendy’s. Check out the below videos to hear customers talk about generative AI and Google Cloud in their own words.
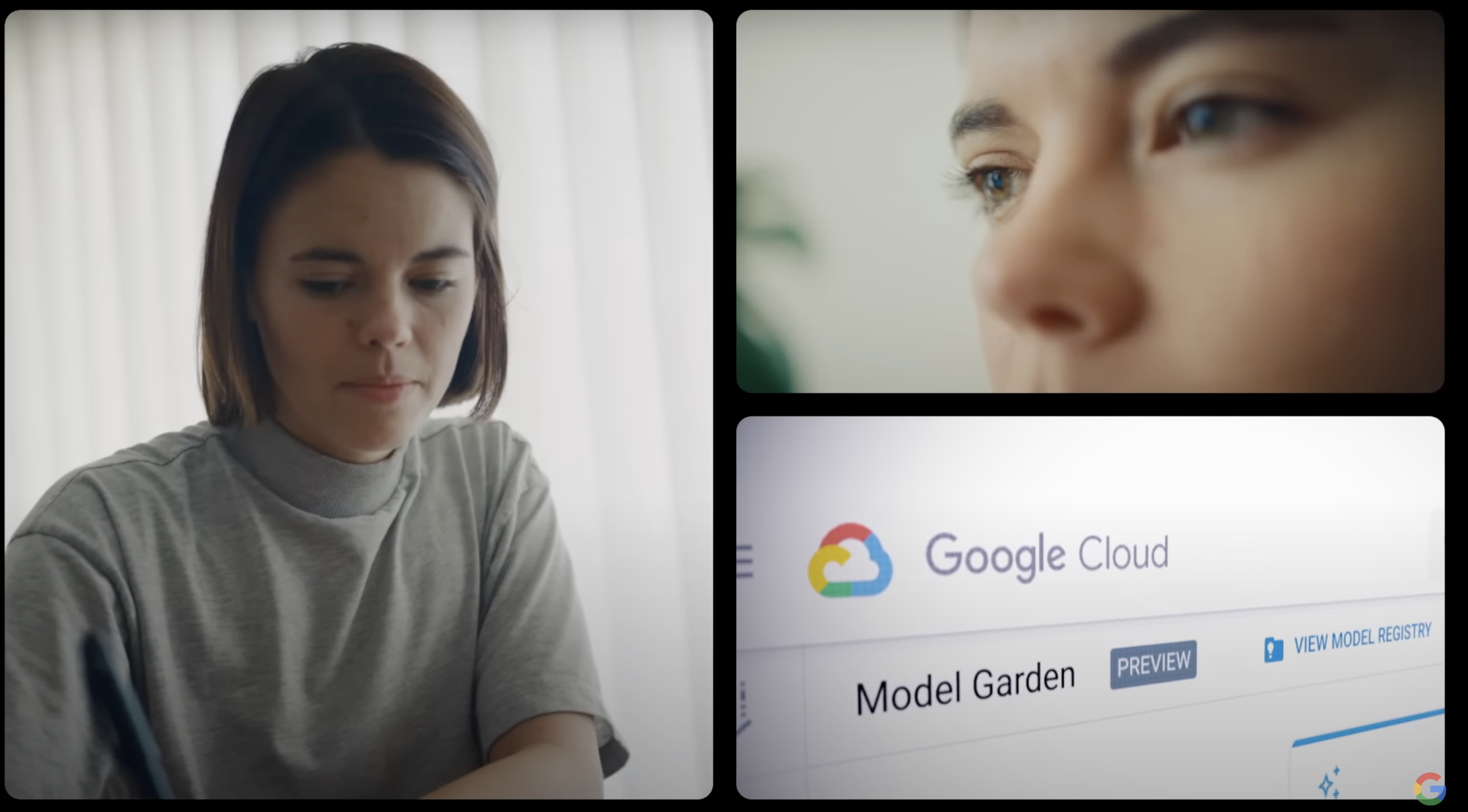
Charting the intersection of generative AI and Search: We shared how generative AI enables new possibilities for Google Search, including new shipping experiences and ways to understand topics faster and get diverse insights. Check out this blog for the details, and visit our new Search Labs, a new way to sign up to test new products and ideas we’re exploring.
Meet Duet: With Duet AI for Google Cloud and Duet AI for Google Workspace, we introduced always-on, generative AI-powered collaborators to help customers get work done faster.
Model Garden blossoms: As mentioned above, we unveiled PaLM 2 and introduced a number of updates to make Vertex AI, our AI development platform, one of the easiest ways for developers and data sciences to leverage foundation models. I/O announcements included the debut of new foundation models for code generation, image generation, and speech; the announcement of our Embeddings APIs; additional model customization tools like Reinforcement Learning with Human Feedback; and preview access to Generative AI Studio, Model Garden, and Palm 2 for Text and Chat, giving everyone with a Google Cloud account a chance to use them.
More powerful infrastructure for more powerful AI: We announced our next-generation A3 GPU supercomputer, purpose-built to train and serve the most demanding AI models by combining NVIDIA H100 Tensor Core GPUs with Google’s leading networking advancements.
Google AI comes to popular enterprise applications: We announced an expanded set of enterprise companies that are integrating Google Cloud generative AI capabilities into their applications, including Box, Canvas, Dialpad, Jasper, Salesforce, and UKG.
Bard gets smarter and more accessible with PaLM 2: Powered in part by PaLM 2, Bard continues to get smarter, including multimodal capabilities and extensions for outside partners—and at I/O, we removed the waiting list for Bard access for over 180 countries and territories, with more coming soon.
Responsibility from the start: A recurring theme across many of these announcements was our commitment to responsible AI, and at I/O, we demonstrated some of the work we’re doing to make AI safe and useful for everyone, such as helping users identify AI-generated content.
I/O was just the latest step in a continuing journey, and we’ll have more news soon—but in the meantime, we look forward to discussing our latest announcements with enterprise leaders and seeing the amazing generative AI use cases their organizations build.