The Prompt: Debunking five generative AI misconceptions
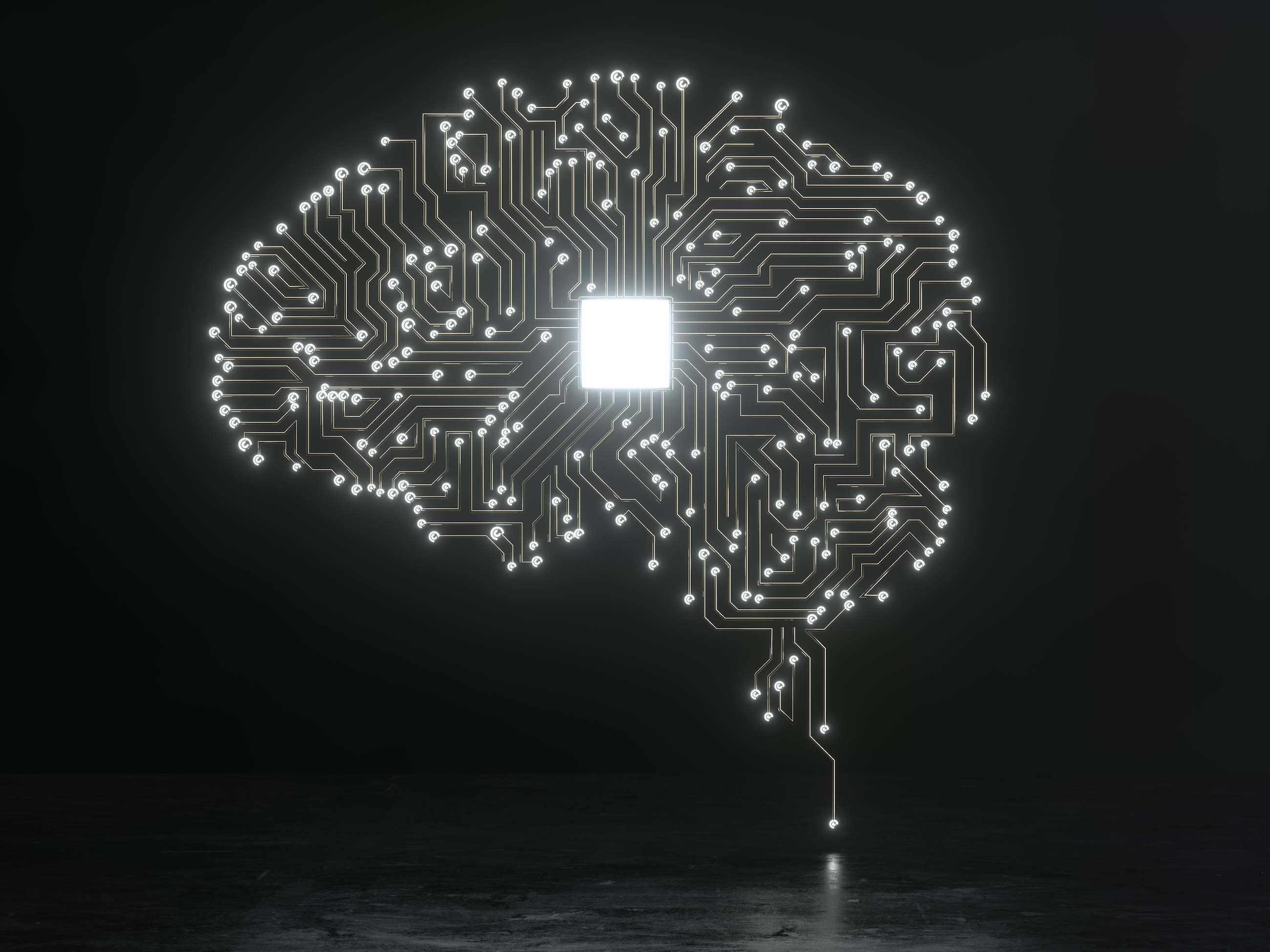
Philip Moyer
Global VP, AI & Business Solutions at Google Cloud
Stay up to speed on transformative trends in generative AI
Business leaders are buzzing about generative AI. To help you keep up with this fast-moving, transformative topic, each week in “The Prompt,” we’ll bring you observations from our work with customers and partners, as well as the newest AI happenings at Google. In this edition, Philip Moyer, Global VP, AI & Business Solutions at Google Cloud, discusses five misconceptions customers have about generative AI.
As you can imagine, I’ve been in a lot of meetings this year with Fortune 500 leaders discussing how they want to bring generative AI into their business. It’s hard to think of a time when there has been so much interest by enterprises in a new technology so quickly. Generative AI is significantly more approachable than previous generations of AI, and I'm excited by all the possibilities.
But matching this excitement, of course, is a lot of hype, so I’ve had to debunk as many ideas as I’ve had to put forward. In this first edition of “The Prompt,” I want to share some of these misconceptions and help demystify this fast-evolving technology.
Misconception 1: One model to rule them all
It is a myth that a single defining large language model (LLM) or other type of generative AI model will define all use cases.
Many corners of the technology market have come to be defined by a handful of companies. The nature of generative AI, especially for enterprises, suggests we will be looking at thousands of models or more.
The reasons vary, but it is already clear that some models are good at summarization, others are good at bulleted lists, others are good at reasoning, and so forth. Industries, lines of business, and companies have very different editorial tones for expression of knowledge. All these should be considered when choosing your models.
Misconception 2: Bigger is better
Generative AI models consume large amounts of computing resources. The large funding rounds required for companies creating foundation models is just one testament to these costs.
Potentially high compute costs are one reason why using the right model for the job is so important. The larger the model, the more it costs to query.
Your enterprise model doesn’t need to know the words to every Taylor Swift song to generate a summary report on next quarter’s sales goals by region. Context is king and you need to be selective in just how much IQ a model requires for your use case.
Misconception 3: Just me and my bot
Just as past “bring your own device” and “bring your own app” movements raised “shadow IT” concerns, some financial institutions I work with have shut down access to publicly available generative AI for fear that models could leak proprietary information.
Some public generative AI services may leverage user data for future training sets, potentially exposing proprietary data. Let’s say a bank is exploring a merger for a large industrial client, and someone in the M&A department queries a public model, asking, “What are some good takeover targets for XYZ Industries?” If that information contributes to the model’s training data, the service could become trained to answer this question for anyone. By default, Google Cloud AI services do not use private data in this way.
Most customers I speak to are worried about the security of the questions they ask models, the content they train on, and their models’ outputs. And they probably should be.
Misconception 4: No questions asked
The accuracy and reliability of generative AI has been one of the biggest topics around the new technology. An algorithm is designed to give an answer no matter what, and in some cases, generative AI models can produce answers that aren’t true.
Every company I know has deeply invested in creating verifiable facts and data. It’s essential for enterprise customers to use models and a technology architecture that is grounded in the factuality of their data.
Most generative AI models punt on this enterprise data requirement. It is essential, especially in regulated industries, to not punt.
Misconception 5: Ask me any question?
Enterprise customers have many information sources: pricing, HR, legal, finance, etc. But I dont know of any company that allows open access to all of this information.
Some business leaders are increasingly interested in building all their information into a large language model, so it can conceivably answer all questions, whether that’s at the organizational level or the global level.
After a company thinks through how they can keep their information private and factual, they quickly realize the next step: How do I manage who can ask questions of my models, and at what level?
Some business leaders are interested in building their information into an LLM, so it can conceivably answer all questions, whether at the organizational level or the global level.
Philip Moyer, Global VP, AI & Business Solutions at Google Cloud
This week in AI at Google Cloud
The preceding observations from the field are just one angle on this exciting topic. Courtesy of Forrest Brazeal, here is the latest Google Cloud generative AI news.
Med-PaLM 2. Ahead of this month’s HIMSS conference in Chicago, Google Cloud discussed limited access to Med-PaLM 2, the first LLM to perform at an “expert” doctor level on the MedQA dataset of US Medical Licensing Examination (USMLE)-style questions. We believe generative AI can be a powerful force within healthcare, and we’re looking forward to working with doctors and researchers to define use cases.
Garden of AI. Last month’s Data Cloud & AI Summit yielded a bountiful harvest of announcements and news, but few were more exciting than the deep dive into Model Garden and Generative AI Studio for Vertex AI. Think of these as your one-stop shop for browsing, tweaking, and deploying foundation models, from Google or the larger AI ecosystem, all within your established Vertex AI workflow.
Model citizens. But if BigQuery is your insight-wrangling tool of choice, you’ll be glad to know it received an AI-flavored upgrade as well in the form of a brand-new inference engine. Use our pre-trained models or import your own from your secret modeling lair, right to where your data lives.
Chips abound. Okay, one last blast from the Data Cloud & AI Summit: we announced an expanded partnership with NVIDIA that includes being the first cloud to offer their AI-optimized L4 Tensor Core GPUs. You can read all about it here.
Contact lens. One of the most immediately useful enterprise applications for AI is customer support, which is why Google Cloud has been hard at work for a while now on Contact Center AI. This post explains how the new Gen App Builder product can help you build even more compelling Contact Center AI experiences.
Revving up devs. My personal favorite recent announcement is Google Cloud’s new AI-focused partnership with developer tooling company Replit. Replit’s AI coding assistant tools will run on Google Cloud services and foundation models to help level up the productivity of more than 20 million developers. That’s pretty darn cool.
Chatterbox. Each week, the Google Cloud team creates lots of additional content to help fill in the context around the fast-moving AI space. If you have limited time this week, I recommend checking out this Twitter space discussing our recent generative AI announcements, Stephanie Wong’s short video on “gen apps,” and Phil Venables explaining our approach to AI in cybersecurity.