The Prompt: Making AI easy, manageable, and personal
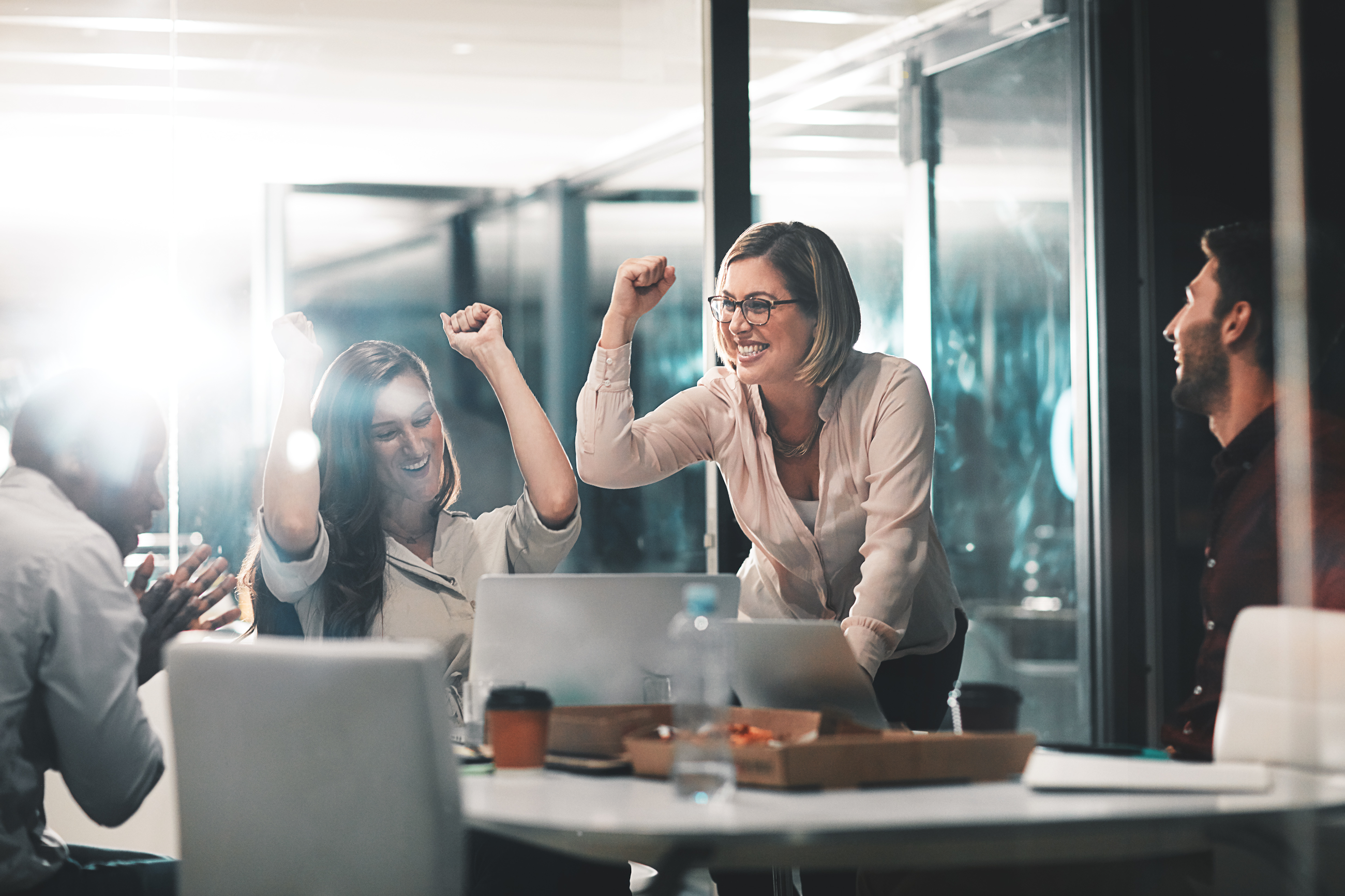
Philip Moyer
Global VP, AI & Business Solutions at Google Cloud
Stay up to speed on transformative trends in generative AI
Business leaders are buzzing about generative AI. To help you keep up with this fast-moving, transformative topic, each week in “The Prompt,” we’ll bring you observations from our work with customers and partners, as well as the newest AI happenings at Google. In this edition, Philip Moyer, Global VP, AI & Business Solutions at Google Cloud, introduces three themes for organizations adopting generative AI: fast, manageable, and personal.
Hot on the heels of our AI announcements at Google I/O 2023, we’ve added a new collaborator for this week’s “The Prompt”: generative AI in Google Workspace.
Many articles in this series begin as wide-ranging, free-flowing conversations between myself and the editorial team. From the start, we’ve used Google Meet’s AI-powered transcription capabilities to create records of these discussions, and now with Workspace’s new generative AI features, we can transform a meandering transcript into coherent, focused notes in just minutes. If the conversation goes off track or splinters into tangents, Workspace’s summarization abilities help to rein us in, identifying the recurring themes from the conversation. AI didn’t write any of the final copy in this blog, but it helped us save hours during the drafting and editing process—and now that we’ve adopted this workflow, we don’t plan to look back!
That leads to my topic for this week: the adoption of generative AI.
Executives increasingly realize that to adopt generative AI, their organizations need more than amazing foundation models. They also need adoption to be easy, with robust management and safety features, and clear, fast ways to create personalized experiences. In this column, we’ll investigate each of these traits, relating them to the strategy Google Cloud has been developing and that was on display at I/O.
Adopting generative AI should be easy
I’ve written before that we don’t think there’s “one model to rule them all.” Foundation models are able to handle an array of downstream tasks without additional training, which means they can be useful out-of-box in a lot of scenarios. But for many use cases, organizations need models that have been fine-tuned for specialized domains, tools for further customization, and a variety of models to fit different cost and latency requirements. Moreover, they need all this to be easy.
Our I/O unveilings reflected these needs. PaLM 2, our newest and most capable model in production, not only encompasses a family of models of different sizes, but also powers a variety of offerings tailored to specific tasks with specialized demands. These include our Codey model for code generation, Med-PaLM 2 for healthcare and life sciences, and Sec-PaLM for security. And with Vertex AI’s Model Garden supplying access to not only many of Google’s foundation models, but also options from third parties, we see organizations using a variety of models in production as they build out their generative AI strategies.
Access is only part of the solution—again, implementation has to be easy. too.
We’ve worked toward this goal with products that mirror the spectrum of customer needs, whether that’s organizations with little AI experience or those with sophisticated data science teams.
For example, Gen App Builder lets developers leverage foundation models to build generative chat and search apps in as little as a few minutes, and getting started doesn’t require any data science experience or even significant coding. Meanwhile, for more in-depth model customization and data science work, Vertex AI not only offers developer-friendly APIs, but also an interface that abstracts many of the complexities of model tuning, prompt engineering, and other tasks that have traditionally required organizations to have significant data science expertise.
Generative AI needs to be manageable and include safeguards
Ease-of-use factors speak to my theme from last week: that organizations need to invest in not only foundation models, but also platforms. The same is true for adopting generative AI safely.
One aspect of safety is having all the built-in governance, auditing, compliance, security, and privacy capabilities required for enterprise use — all of which are necessities we prioritize with every Google Cloud product, backed by our AI Principles. But another aspect is how some of these capabilities work.
Customizing foundation models can help ensure outputs will be safe and effective, for example. But not all forms of customization are equally effective for all use cases, and not all methods are equally scalable or simple to integrate into MLOps workflows.
That’s one reason we’ve built both MLOps tools and a variety of tuning mechanisms into Vertex AI, including being the first hyperscale cloud provider to offer reinforcement learning from human feedback (RLHF), which lets organizations easily integrate human input to fine-tune models. Likewise, Gen App Builder makes it simple to limit generative AI’s outputs to specific data sources, helping to keep the model focused and accurate at scale.
As important as ease-of-use is, safety-minded implementation — backed by governable, trainable, updatable models — is just as crucial.
The best generative AI use cases are often personal
In my recent conversations with enterprise leaders, I’ve heard more and more of them embrace the argument that effective generative AI implementations are often personal.
At I/O, we announced a variety of products around this ideal, like always-on AI collaborators in Duet for Google Cloud and Duet for Google Workspace, which provide contextual AI collaboration for whatever work the user is trying to get done. Magic Editor emphasized meaningful digital interactions too, using AI to edit photos so they better capture important memories. And with products like Vertex AI and Gen App Builder, we’re also making it easy for organizations to create these personal applications for their own users.
Check out the below video to see how Duet for Google Cloud enables more personal experiences with AI for Google Cloud users, and for a deeper dive into Duet for Google Workspace, see these demos from the I/O keynote.
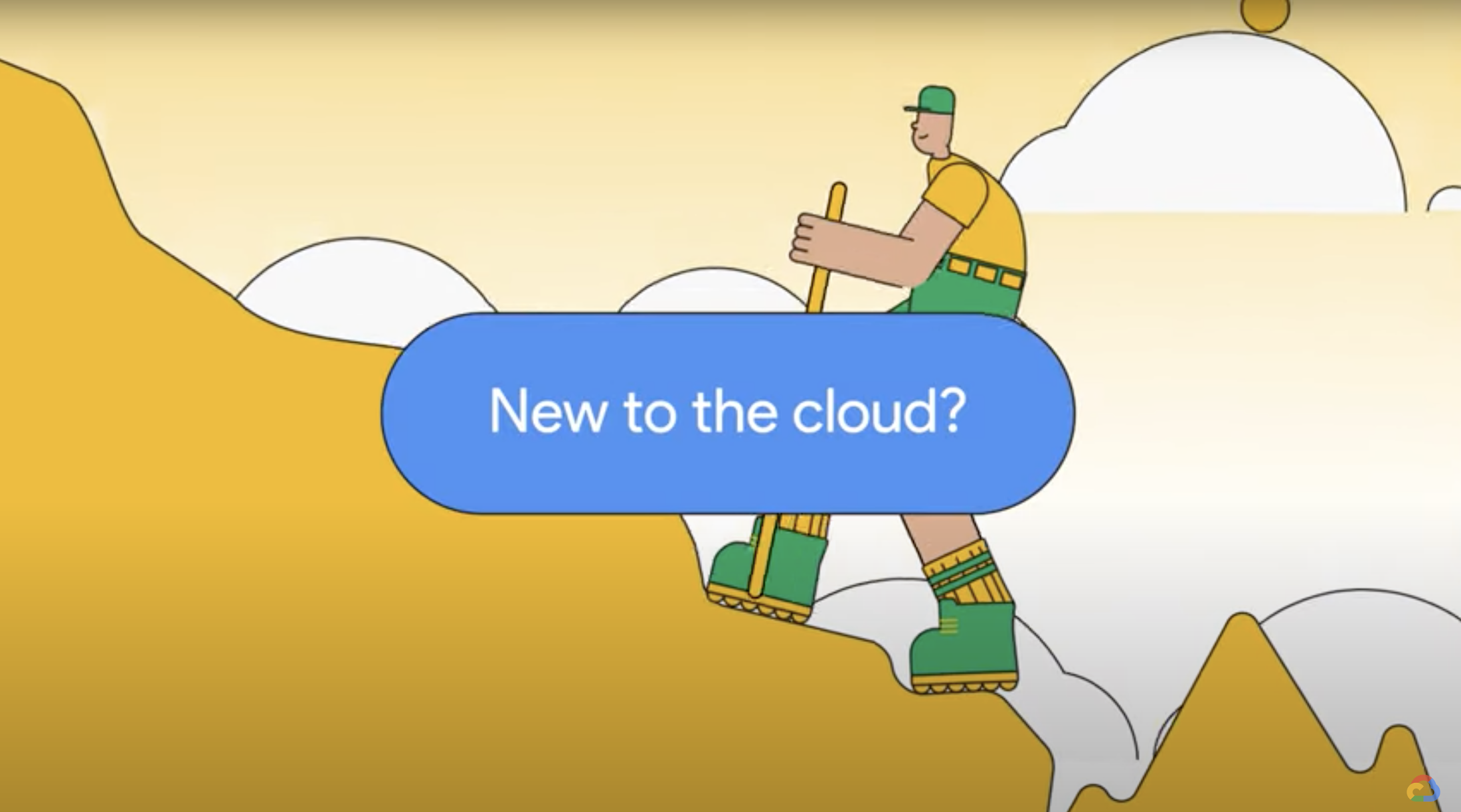
Generative AI is just getting started
Our collaborations with customers have reinforced that organizations are eager to benefit from this technology’s awesome potential, but executives aren’t always sure where to start, from either a use case or technology perspective. We see that customer needs form a wide spectrum, from simple, quick onramps to generative AI all the way to sophisticated training, fine-tuning, customization, and MLOps. This technology needs to meet businesses where they are, and give them choice and flexibility to mature. We’ve learned a lot as our generative AI products have reached customers’ hands, and though I/O was a big day, we’re excited to share much more soon.
This week in AI at Google
Google Cloud is rolling out new training for generative AI—check out this blog for the details and to get started.
Google Cloud announced the Target and Lead Identification Suite, an AI tool for life science researchers to better identify the function of amino acids and predict the structure of proteins, and the Multiomics Suite, for discovering and interpreting genomic data, and designing personalized genomic treatments. See this blog for the details and don’t miss this coverage of the announcements from CNBC.
Google Research continues to rapidly announce new insights, techniques, and approaches. This week’s highlights include a look at the People + AI Research group within Google’s Responsible AI and Human-Centered Technology team; new research to help build more equitable computer vision models that better handle diverse skin tones; and advances in reinforcement learning to help AI assistants plan multi-turn conversations toward a goal and adapt as the conversation flows.