Empowering consumer finance apps with highly personalized investment recommendations using AI
Zac Maufe
Global Head of Regulated Industries, Google Cloud
Try Google Cloud
Start building on Google Cloud with $300 in free credits and 20+ always free products.
Free trial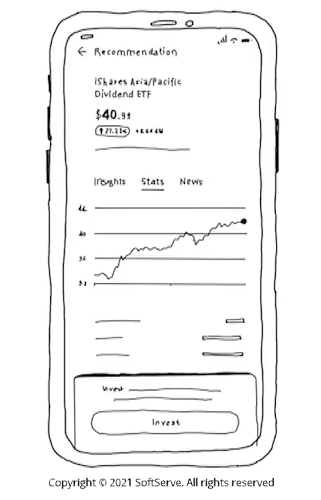
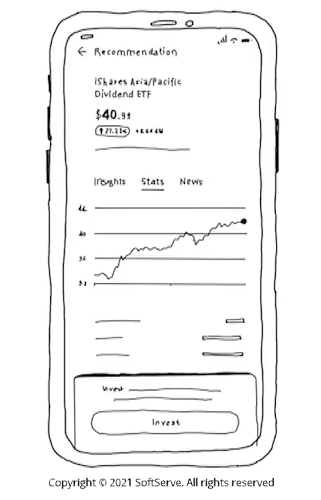
Data science is one of today’s key priorities for finance industry leaders. Data Scientists harness knowledge to draw meaning from data, to turn data into information, and to translate information into practical insights that will bring a better understanding of how to gain customer loyalty, minimize churn, and grow revenue. In this blog post we will look at a comprehensive investment banking solution that builds a bridge between retail investors and the complexity of the capital markets.
Let’s explore how Google Cloud Data and Analytics services can be used to turn real-time insight into an automated process, creating frictionless digital experiences to help retail investors with little capital markets expertise. The solution developed by SoftServe provides users with personalized investment recommendations to help make better decisions. Called the Investment Products Recommendation Engine (IPRE), SoftServe designed this solution to recommend the most suitable investment product by balancing an individual’s risk preferences and expected return on investment.
SoftServe’s IPRE collects and processes market data (e.g., quotes, daily or weekly open, high, low, close prices) on available investment products such as stocks, bonds, powered by BigQuery and Cloud Functions. The IPRE prepares the raw data via Dataflow and constructs an optimal mean-variance portfolio for a given level of risk. So, the investment portfolio is optimized to provide the highest expected return on investment for a given risk level.
An investor’s risk appetite depends on various factors and may exhibit non-stationary evolution over time. To produce recommendations in accordance with the optimal risk level for an individual investor, SoftServe used an AutoML Tables model, based on a variety of customer characteristics: level of income, level of savings, level of education, employment, geography, etc. This approach provides more flexibility when compared to classical investment theory metrics such as Constant Relative Risk Aversion (“CRRA”), Constant Absolute Risk Aversion (“CARA”), etc., consequently enabling the IPRE to unlock new customer segments.
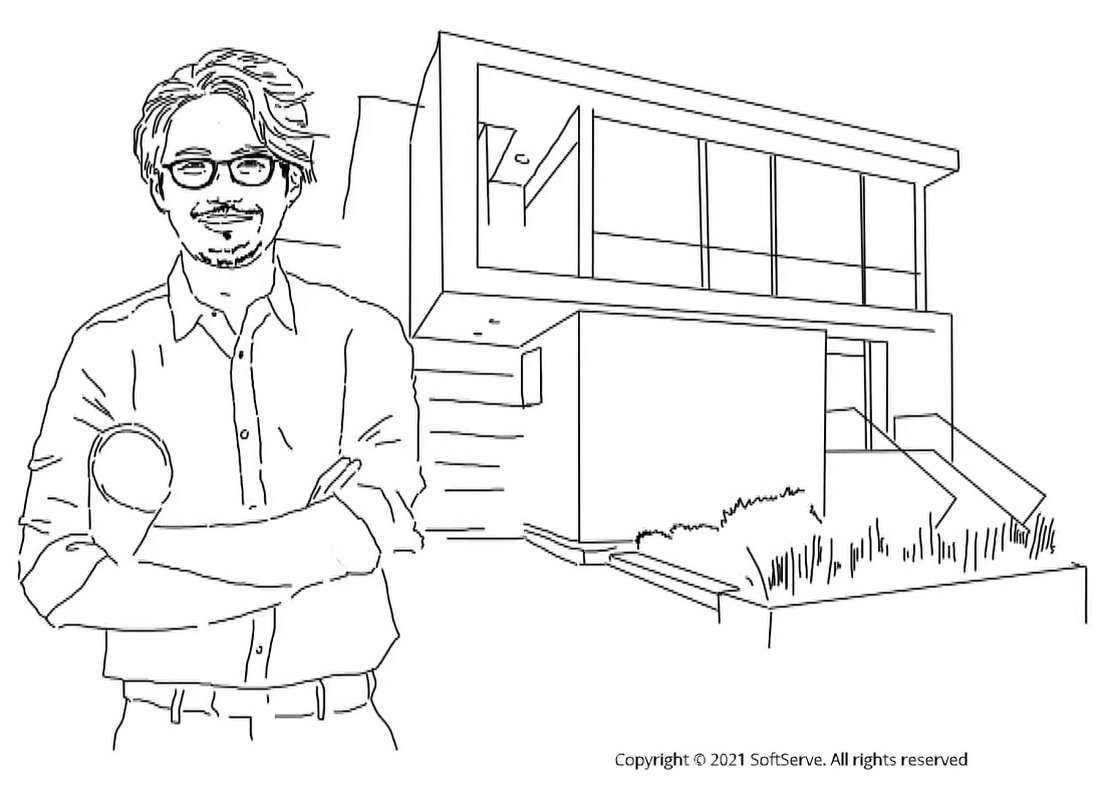
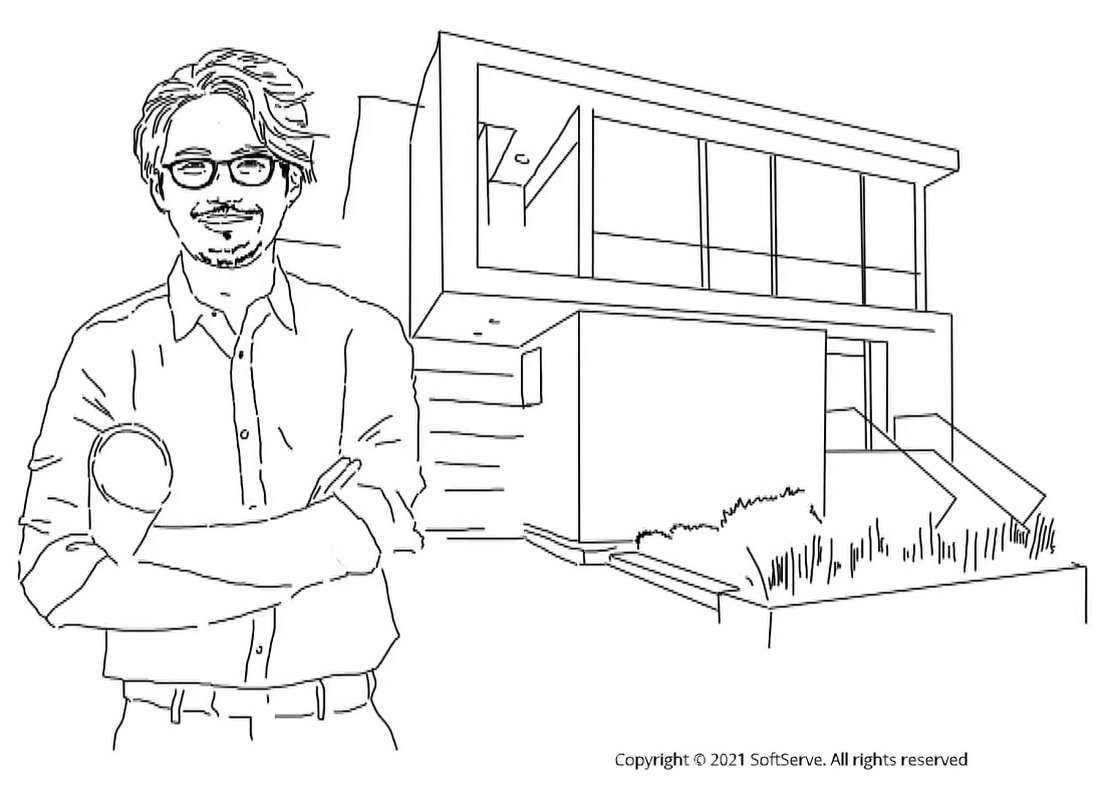
Finally, after providing recommendations for a portfolio of optimal assets based on risk levels, the IPRE estimates the qualitative and quantitative characteristics of the portfolio. It computes sophisticated industry-grade investment metrics describing the marginal risks, Conditional Value at Risk (CVaR), diversification effects, Sharpe Ratio, sensitivity of the portfolio to market fluctuations, etc.
Let's take a look at a hypothetical user journey to better understand the purpose of the solution and the value it brings to market.
Meet Felix, a 33-year old architect whose dream is to buy his own flat in the next five years. He realizes he must accumulate more savings. A few months ago, Felix opened an account in the For-the-Future bank because of the smart investment feature on their mobile app, where he receives investment recommendations and can make decisions on the go. Felix has set a financial goal and built up a portfolio of investment funds aligned with his risk tolerance and his investment goals.
One day, on his way to work, Felix receives a personalized investment recommendation from the For-the-Future bank’s mobile app. The app is constantly working to help Felix reach his goal and does all the time-consuming work on collecting and processing market data. The machine learning model generates recommendations, such as expected rate of return, popularity of the asset among people with portfolios like Felix’s, and information about the risk level that matches Felix’s portfolio. Felix can use that information to make a decision.
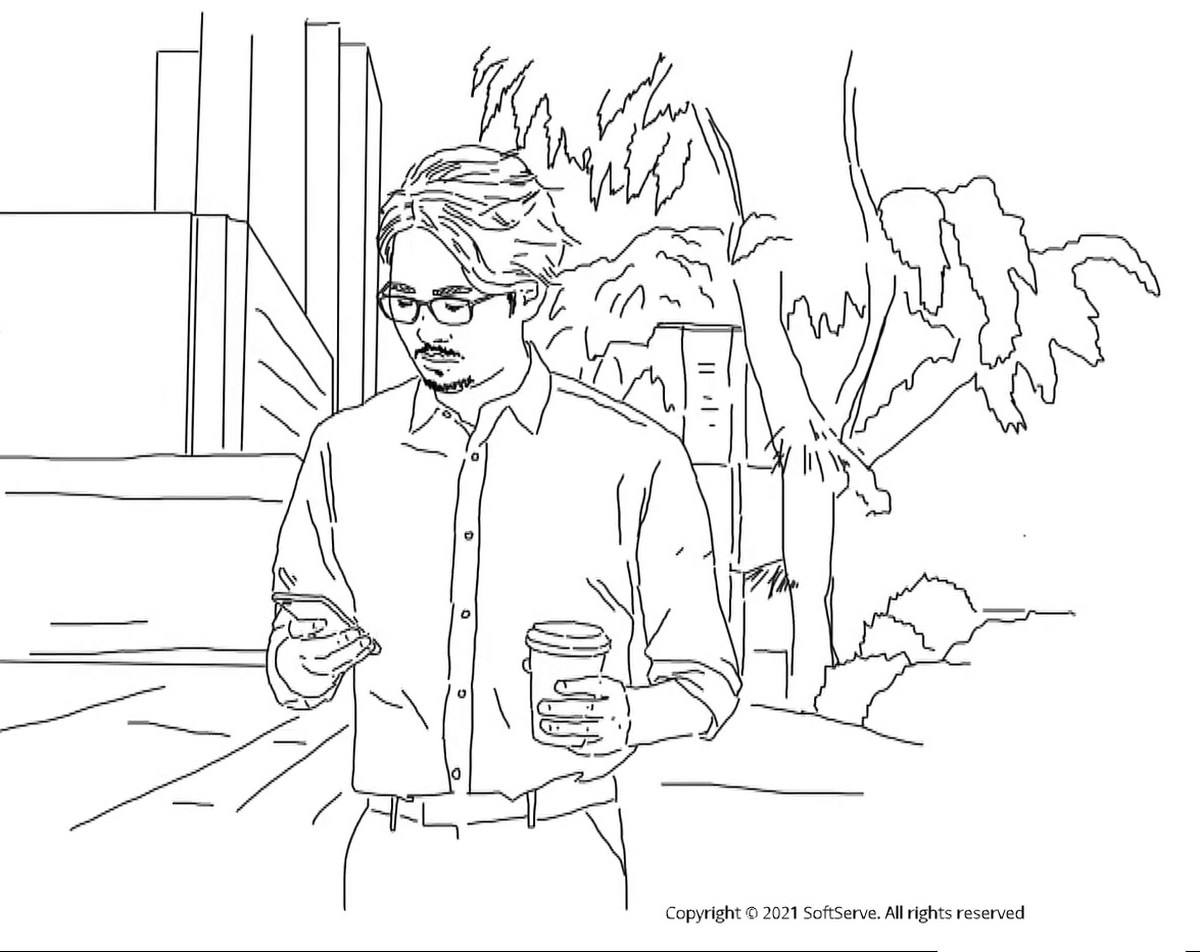
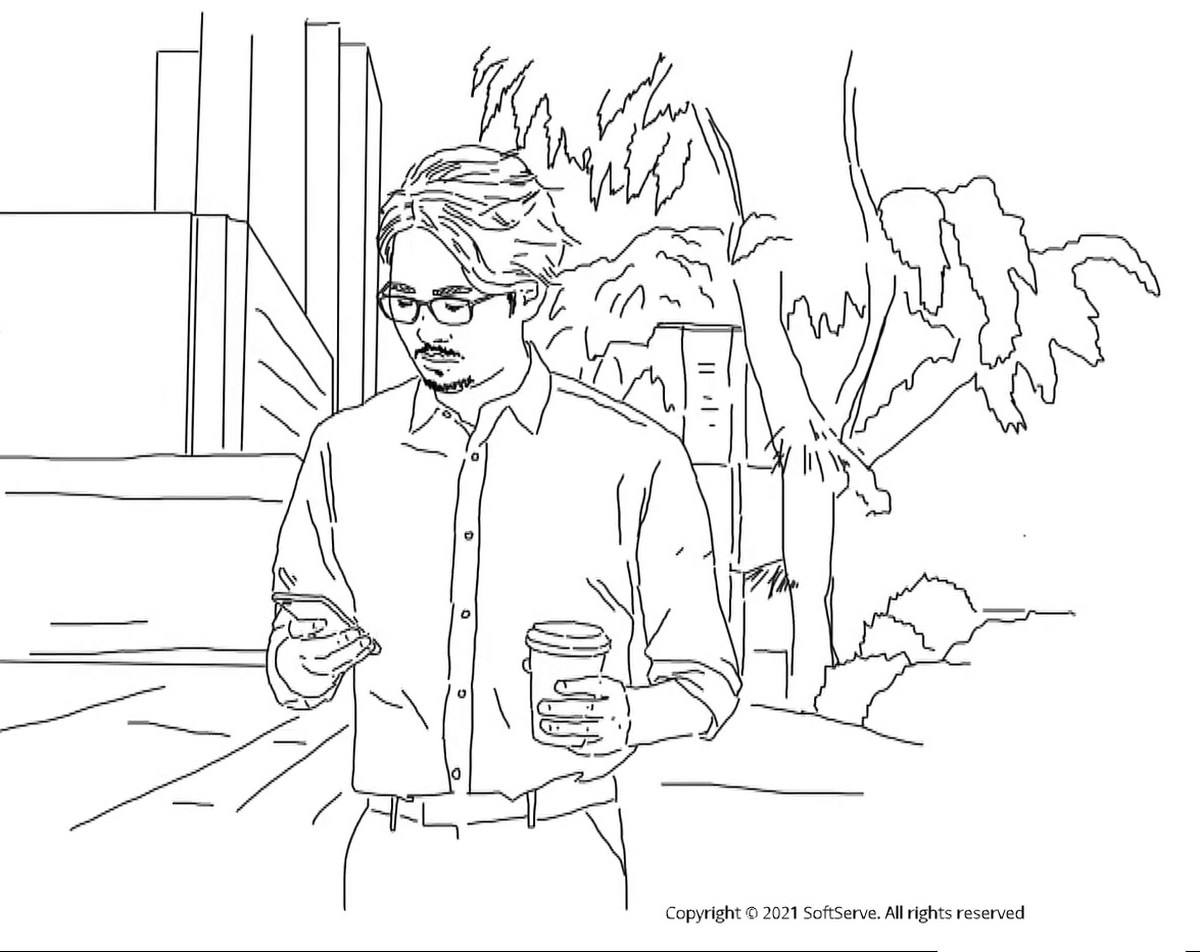
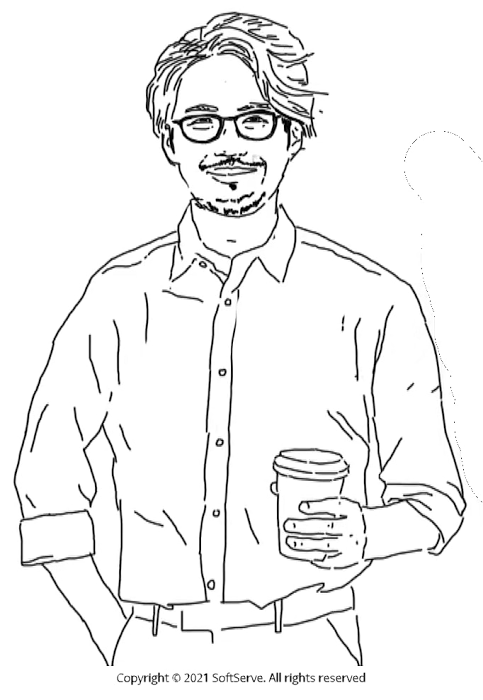
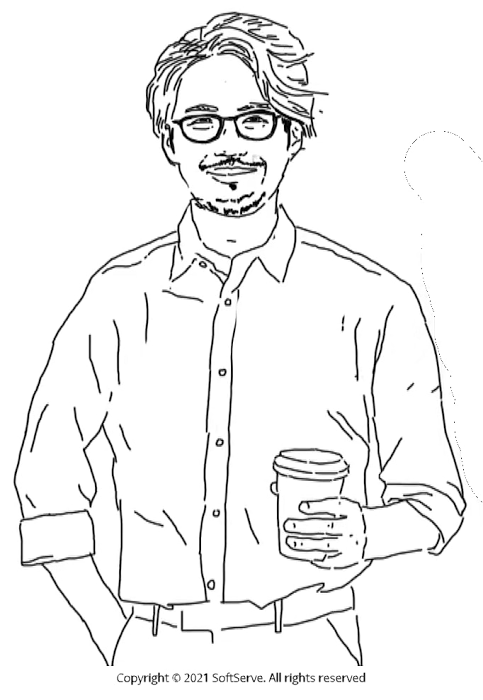
The process of using the app was quick and simple. Felix’s portfolio gets automatic updates with the total value of the portfolio and tracks its performance against his financial goals. Felix continues his way to work smiling to himself, knowing that he is a little bit closer to owning his dream home.
The technical implementation of the solution in Google Cloud incorporates Dataflow batch processing pipelines as well as trained investment recommendation Big Query Machine Learning (“BQML”) models and data analytic services such as BigQuery, Cloud Storage, and Pub/Sub. The solution is described in the blog post How to implement an Investment Product Recommendation solution in GCP.
In partnership with Google Cloud, SoftServe helps our clients solve complex problems with innovative solutions to achieve a faster time to market, increase ROI, and provide great user experiences.
To gain a broader understanding of the solution and see how its architecture works in real life, watch SoftServe's user journey presented at Google Southeast Asia Financial Services Cloud OnAir: Creating aha moments in Financial Services.