Spotlighting ‘shadow AI’: How to protect against risky AI practices
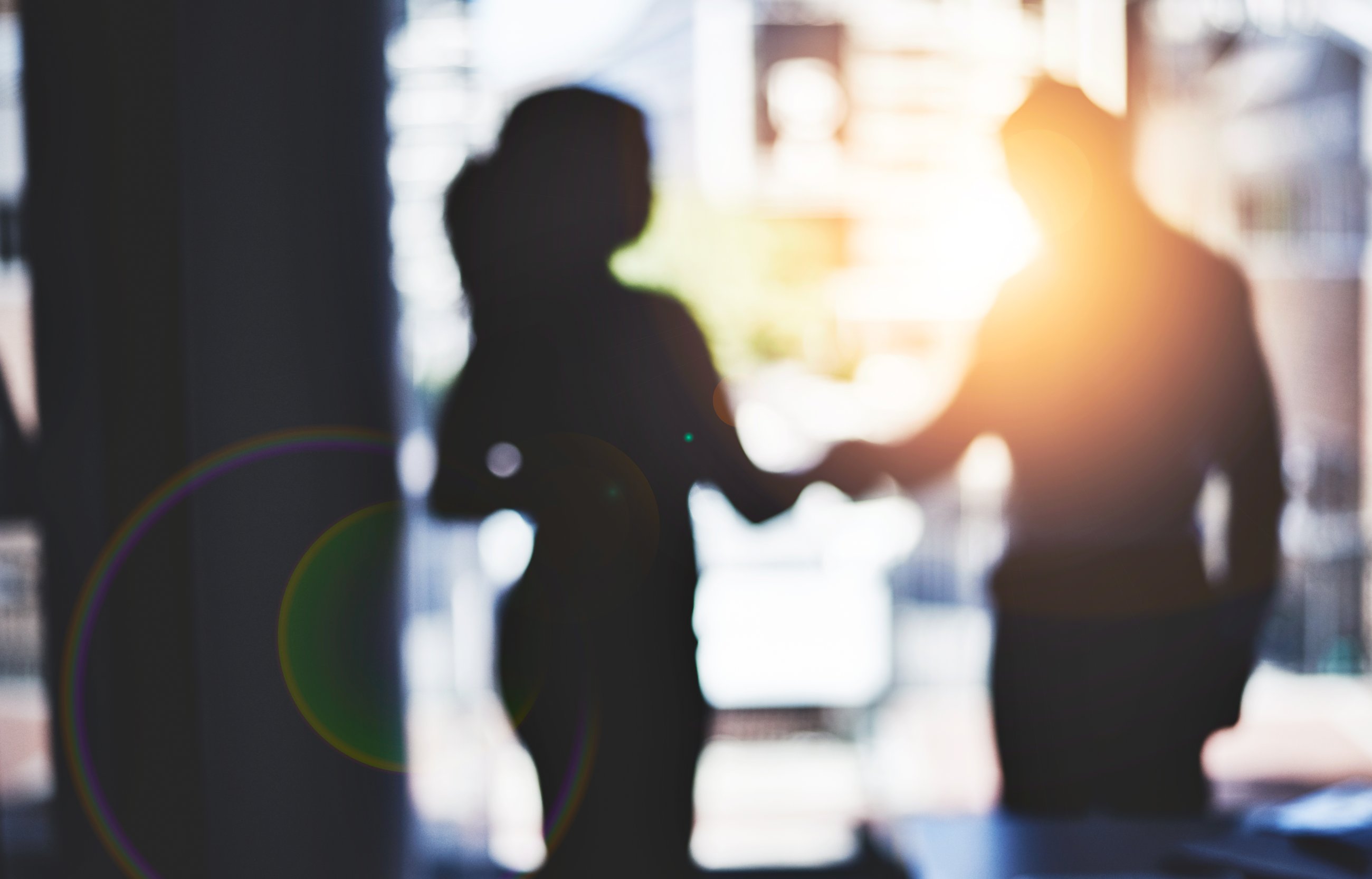
Anton Chuvakin
Security Advisor, Office of the CISO, Google Cloud
Marina Kaganovich
Executive Trust Lead, Office of the CISO, Google Cloud
Why enterprise-grade AI is superior for the workplace
Hear monthly from our Cloud CISO in your inbox
Get the latest on security from Cloud CISO Phil Venables.
SubscribeOver the past two decades, we’ve seen the challenges of “bring your own device” and the broader “bring your own technology” movement, also known as shadow IT. Today, organizations are grappling with an emerging artificial intelligence trend where employees use consumer-grade AI in business situations that we call “shadow AI.”
The incredibly rapid adoption of generative AI poses a significant challenge when employees want to use gen AI tools that haven’t been explicitly approved for corporate use. The use of shadow AI is likely to increase the risks that an organization faces, raising serious questions about data security, compliance, and privacy.
“We know that to protect against many kinds of attacks, traditional security controls, such as ensuring the systems and models are properly locked down, can significantly mitigate risk. This is true in particular for protecting the integrity of AI models throughout their lifecycle, which can help prevent data poisoning and backdoor attacks,” said Royal Hansen, vice president of Privacy, Safety, and Security Engineering at Google, in a recent blog. “Traditional security philosophies, such as validating and sanitizing both input and output to the models, still apply in the AI space.”
Organizations are rightly looking to AI to help tackle challenges and advance their business goals, but not all AI is created equal. In our discussions with enterprise customers, we’ve observed several new potential shadow AI risks emerge, including:
- Sensitive data leakage, data being shared by the model with other users, inadvertent sharing of secrets;
- Unauthorized access to the customer’s corporate information by the operator of the gen AI offering, such as human review of customer prompt data;
- Non-compliance with applicable cybersecurity or data privacy requirements; and
- “Hallucinations,” which are inaccuracies in the chatbot output.
In an attempt to mitigate these risks, corporate leaders often react by banning gen AI in the workplace. Such measures are security theater because they offer minimal risk reduction benefits, and may even increase risks by pushing the usage deeper underground and thus further away from deployed security controls.
While it’s true that using enterprise-grade AI for business comes with its own risks, it’s important to note crucial differences between enterprise-grade AI and shadow AI, the unapproved business use by employees of consumer-grade AI. In general, additional safeguards included in enterprise-grade AI can address risks with technical and procedural means to help secure your models and training data.
For example, enterprise-grade AI tools should include the ability to protect against accidentally sharing sensitive and private data in the chatbot’s prompt, and the subsequent use of that data to train foundation models. An employee who wants to be more productive by asking a gen AI-powered chatbot for an executive summary of their meeting notes to share with their manager seems perfectly aligned with the chatbot's core functionality.
Keeping private data private is the bedrock of building trust.
Doug English, CTO, Culture Amp
However, pasting their notes into a consumer gen AI tool and then asking that tool to summarize them, can risk divulging personally identifiable information, such as the names of meeting attendees, or expose non-public customer and corporate information.
The same task can be accomplished in a manner that ensures your organization’s data remains private and secure by using an enterprise-grade productivity tool such as Duet AI in Google Workspace that operates within the security confines of your organization.
In general, the risks you face will increase when using consumer-oriented technology to solve business problems. (Our Security AI Framework sheds more light on how best to secure AI systems.)
Risk management can vary depending on how the organization has chosen to use AI and how sensitive the data is: Is the business developing its own AI applications, using AI applications developed by a third party (including those developed by Google Cloud), or a mix of both? How enterprise-ready those services are is also a factor we discuss here.
“Keeping private data private is the bedrock of building trust,” said Doug English, CTO, Culture Amp.
To further that goal, we believe that enterprise-grade generative AI should offer security controls. For example, Google Cloud’s Vertex AI offers encryption by default, encryption key management options, VPC service controls, and data residency controls. When combined with a service such as Google Cloud’s Sensitive Data Protection, you can more easily discover, classify, and protect your data, as well as mitigate risk and guard against unintended data disclosure.
“While AI does represent a new security world, it’s not the end of the old security world, either. Securing AI does not magically upend security best practices, and much of the wisdom that security teams have learned is still correct and applicable,” we wrote in a recent blog post. At Google, we believe that it’s important to build AI tools boldly and responsibly, and part of that is understanding that many of the security principles and practices that apply to traditional systems also apply to AI systems.
In addition, we believe that data governance controls can help ensure that customer data is not used to train foundation models. At Google Cloud, we support this approach with contractual commitments regarding customer data processing and customer IP ownership for generated output, along with IP indemnification.
Enterprise gen AI tools can deliver on user expectations with more transparent model training, utilizing frozen models, and supporting legal and privacy commitments. Developing AI boldly and responsibly can bring productivity and operational efficiency benefits, all in a secure environment with appropriate controls that support data privacy and governance. We see general-purpose generative AI assistants and apps as collaborators in most use cases. These are tools to help us save time and find inspiration, to enhance our human abilities — but not replace them with “worry-free automation.”
For further reading on how to securely use gen AI in an enterprise-ready manner, refer to our recent blog on enterprise readiness in the age of generative AI and a paper articulating our Trust in AI posture for a view into how our gen AI capabilities are designed and built with security, privacy, governance, compliance and Responsible AI in mind.