The Prompt: What AI model is it anyway?
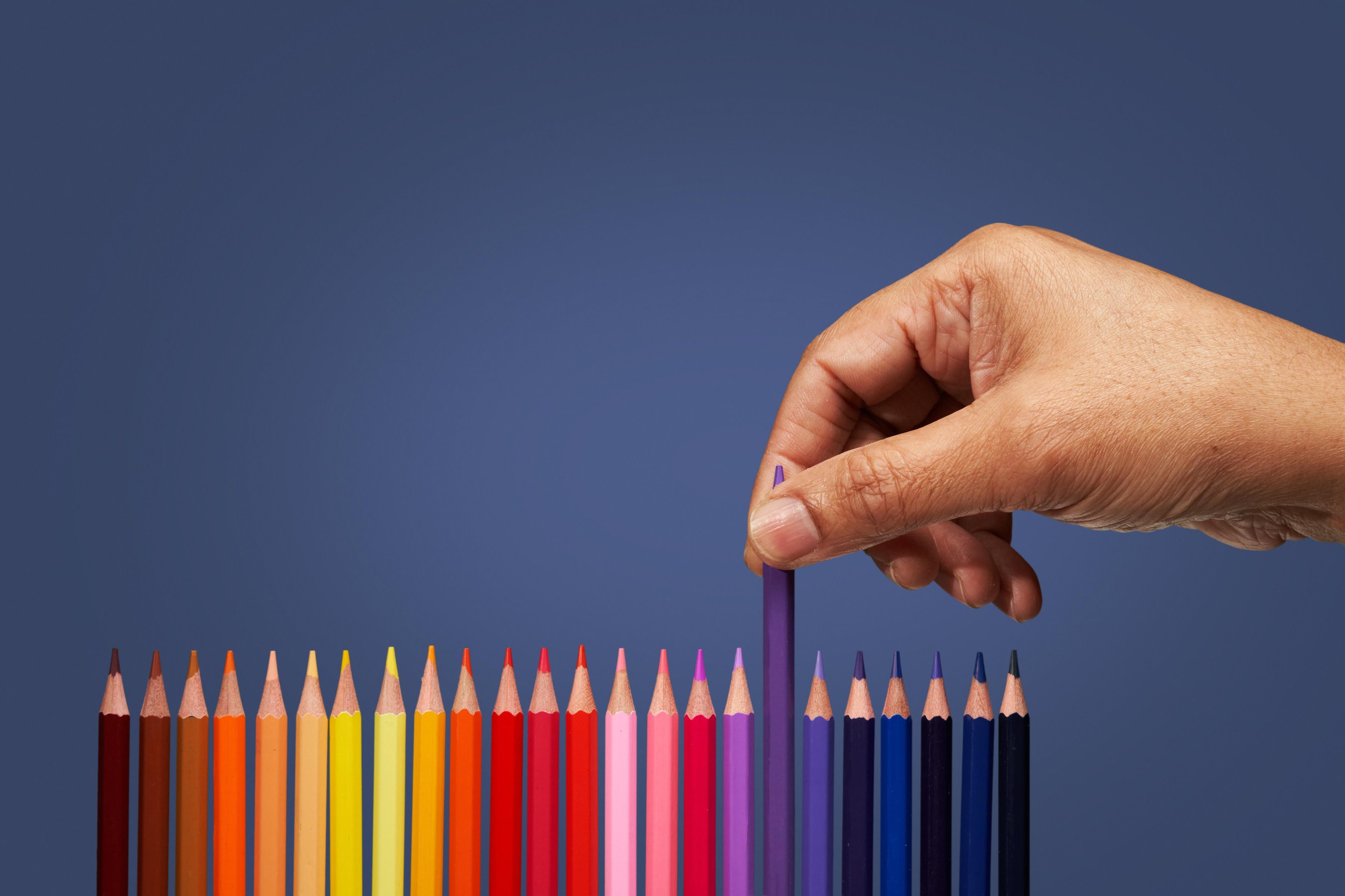
Warren Barkley
Sr. Director of Product Management
Understanding distinctions and trade-offs among various types of gen AI models is critical to successful gen AI adoption.
Business leaders are buzzing about generative AI. To help you keep up with this fast-moving, transformative topic, our regular column “The Prompt” brings you observations from the field, where Google Cloud leaders are working closely with customers and partners to define the future of AI. In this edition, Warren Barkley, Vertex AI product leader, explores some of the distinctions and trade-offs between various types of gen AI models.
Gen AI models, while incredibly flexible and versatile, are not a catch-all solution for every challenge — nor is a single model capable of solving all of your problems. Some gen AI models are better suited to certain tasks while others may be a better choice depending on your industry and other requirements you have around performance, privacy, complexity, and cost.
And with so many model types and sizes out there, how do you make sense of them all and understand how to use them?
In recent months, questions about model choice have come up again and again in my conversations about adopting and scaling gen AI. Many leaders want more clarification on what different gen AI models can actually do and guidance about how to use them to support their strategic objectives.
Understanding the nuances of different types of gen AI models and how they relate to the broader context of your applications is therefore a critical step towards using gen AI to drive business value and innovation. In this edition, we’ll explore some of the key points we often highlight that have helped our own customers navigate this topic successfully.
Considering different gen AI models
The gen AI model landscape is wide-ranging and diverse, from foundation models to domain- or task-specific large language models to smaller, single-purpose micro models. In general, when asking about gen AI models, many organizations may be referring to foundation models — large-scale AI models that have been pre-trained on massive datasets to perform a wide variety of tasks, including content generation, data augmentation, creative problem-solving, and many more.
For example, Google’s multimodal foundation model Gemini can generalize and understand, operate across, and combine different types of information, such as text, audio, image, videos, and code. Foundation models can be adapted and fine-tuned for a broad range of applications, providing a strong starting point for many types of business use cases.
With the rise of enterprise adoption of gen AI, there is an increasing need for models that can generate output, tailored for particular industries, fields, and types of tasks. Unlike general-purpose foundation models, domain-specific models are trained to interpret context, terminology, and even jargon in a particular area, such as healthcare or cybersecurity.
Similarly, task-specific models are built to perform a specific task or a set of closely related tasks, such as translation, code completion, and image or video generation. While these models can handle more specialized tasks with greater accuracy and relevancy, they offer lower adaptability and often carry higher development costs.
More recently, we’ve also seen a growing trend around lightweight models for low-latency use cases that require deploying models on devices with more limited computational power, such as smartphones, and other types of embedded systems. For instance, Google’s mobile-sized model Gemini Nano can operate on-device, allowing data to be securely analyzed and responded to faster and with limited connection. While highly efficient, these micro models come with tradeoffs in terms of overall accuracy and versatility compared to their larger counterparts. Especially for mobile devices, it comes back to the trade off of functionality vs power and compute.
Deciding between proprietary and open AI models
Another dimension organizations will need to take into account during the decision-making process is whether to use proprietary or open-source gen AI models. There’s a lot of excitement in the market about the possibilities offered by open models, particularly around Meta’s LLaMA models, Google’s Gemma, and Mistral AI’s diverse range of models for generalist tasks, specialized generation, and research.
Open-source gen AI models can offer greater customization and flexibility, enabling teams to fine tune or fully tune a model to very specific requirements and scenarios. For instance, one of our large pharmaceutical customers found that while there were a lot of proprietary models available for their domain, they didn’t work well for their specific use cases. The company wanted to train a model to understand the fundamental principles of biology and how different molecules and compounds can work together. As a solution, the team decided to train a large open-source model using all the data they had around formulas, drug discovery processes, and different chemical reactions, allowing them to deliver a model that could suggest novel combinations and interactions for further research and exploration.
However, this flexibility comes with obvious tradeoffs. Open models can carry more security and legal risks than proprietary models, most often because they require additional work to ensure they meet standard levels of privacy and compliance. Organizations will also need to thoroughly understand open model licensing requirements while also providing their own guardrails to prevent any potential copyright or rights of use infringements. By comparison, proprietary models often come with indemnification for training data and model outputs along with enterprise-grade security features, such as safety filters, citation filters, safety error codes, and more.
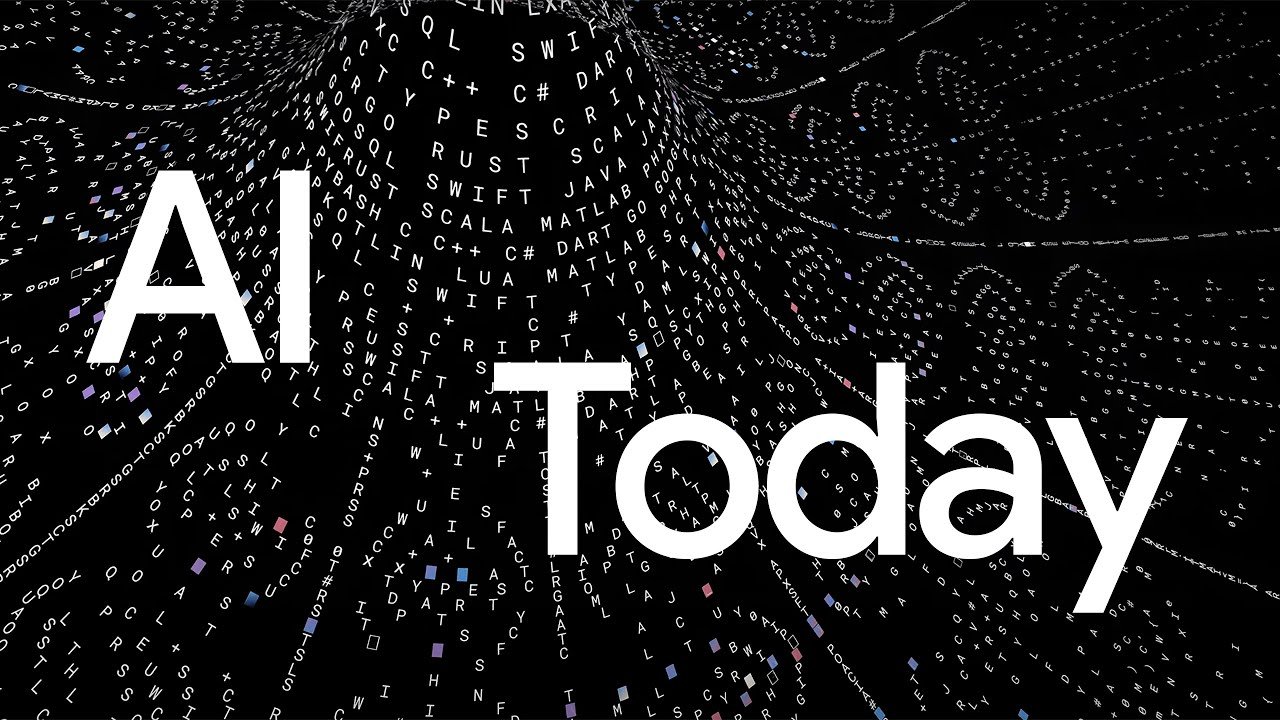
Additionally, adapting and using open models for specific business use cases typically calls for more specialized skill sets and technical expertise, which may not be feasible depending on an organization's current capabilities and available resources. Proprietary models often include more streamlined support and easier management and adaptability, minimizing the need for extensive in-house technical expertise.
Currently, we’re seeing companies considering both open and proprietary gen AI models, often choosing a mix of both, with the choice often depending on specific use cases, the level of control needed, and licensing requirements. This approach allows teams to leverage benefits from each, allowing them to tap into the flexibility and innovation of open models while also gaining the reliability and support of proprietary solutions when necessary.
Understanding your AI use cases
While generative models are a new category of AI models, the fundamentals don’t change. To use them effectively, you still need to understand what business problem you want to solve. I often see organizations simply going straight to gen AI without first considering if it’s the right solution for their specific challenges.
In our work with customers, we always recommend starting with the business problem. Thoroughly understanding the issue you want to solve first can go a long way towards helping you make more informed decisions. Ultimately, not all problems are AI problems and more critically, not all AI problems are gen AI problems. In some cases, you might find that you need a different type of AI altogether or a combination of different AI technologies to accomplish your end goals.
There are many areas where organizations can use models to reduce costs and create better experiences. In particular, we like to look at daily workflows that rely heavily on unstructured data or have data that is not well described or understood, such as paper-based processes.
These areas often benefit from using gen AI to synthesize and structure this information into more digestible formats without a lot of manual work. Gen AI models also excel at creative tasks, making them ideal for use in departments like marketing or sales. The key is looking for the top scenarios within your own organization and imagining ways where AI could be used to automate or augment processes.
To use gen AI models effectively, you need to understand what business problem you want to solve. I often see organizations simply going straight to gen AI without first considering if it’s the right solution for their specific challenges.
Warren Barkley, Sr Director, Product Management, Google Cloud
Choosing the right gen AI model
Gen AI models have their own unique strengths and limitations that need to be carefully weighed against the problem you wish to solve and the individual needs of your organization. I’ve distilled it down to five key decisions that should help you hone in on a few specific models for testing and evaluation.
- Governance: Right off the bat, are there any industry-specific constraints that might impact the type of model needed? Healthcare, finance, and government often have stringent requirements for data privacy, security, and explainability, which might necessitate using models that have the right type of certification, open models that allow for higher levels of transparency and customization, or even models that can be run on isolated networks and infrastructure.
- Use Case: Consider your specific use case. What tasks must the model perform? How complex are these tasks? Does the desired output need to be in a particular format or style? Answering these questions will help you narrow down your model options and potential sizing. Using AI evaluation tools and services at this stage can further refine your decision by assessing the feasibility of your use case with various models and pinpointing areas where performance might be enhanced (e.g., tuning, grounding, latency). For example, you can take the most basic scenario of your goal and compare the different responses of models to the same prompts.
- Performance: What factors are most important — latency, cost, or customizability? These are all crucial factors when selecting a model because they directly impact the effectiveness and feasibility of your AI solution. High latency leads to slow response times, which can frustrate users and make applications feel sluggish. Cost is also critical to delivering a return on investment, and customizability may be required to achieve the expected levels of performance and accuracy of your use case. A smaller, less complex model might have lower latency and cost, but may offer less customizability. A highly customizable model might be more expensive to train and deploy, and potentially have higher latency.
- Data: Do you have the data needed for customization? The availability of labeled data, domain specific data, data quality, and more are all important considerations for customizing models. Larger and more complex models generally require massive amounts of data to train effectively. If you have limited data, a smaller model might be a better choice to avoid overfitting and poor generalization.
- Skills and resources: To effectively customize and implement generative AI models, ensure you have the right team in place. Building a successful team requires assembling individuals with skills in machine learning, data science, software engineering, and in-depth understanding of the subject matter. On top of that, it's crucial to ensure you have the necessary computational resources (e.g., GPUs, memory, etc.) and the right tools and platforms for developing, deploying, and monitoring AI models.