Google Cloud VMware Engine, PowerCLI and BigQuery Analytics
Konrad Schieban
Strategic Cloud Engineer, Google Cloud Professional Services
Matthew Smith
Strategic Cloud Engineer
Google Cloud Billing allows Billing Account Administrators to configure the export of Google Cloud billing data to a BigQuery dataset for analysis and intercompany billback scenarios. Developers may choose to extract Google Cloud VMware Engine (GCVE) configuration and utilization data and apply internal cost and pricing data to create custom reports to support GCVE resource billback scenarios. Using VMware PowerCLI, a collection of modules for Windows PowerShell, data is extracted and loaded into Big Query. Once the data is loaded into Big Query, analysts may choose to create billing reports and dashboards using Looker or Google Sheets.
Exporting data from Google Cloud billing data into a BigQuery dataset is relatively straight-forward. However, exporting data from GCVE into BigQuery requires PowerShell scripting. The following blog details steps to extract data from GCVE and load it into BigQuery for reporting and analysis.
Initial Setup VMware PowerCLI Installation
Installing and configuring VMware PowerCLI is a relatively quick process, with the primary dependency being network connectivity between the host used to develop and run the PowerShell script and the GCVE Private Cloud.
[Option A] Provision a Google Compute Engine instance, for example Windows Server 2019, for use as a development server.
[Option B] Alternatively, use a Windows laptop with Powershell 3.0 or higher installed
Launch the Powershell ISE as Administrator. Install and configure the VMware PowerCLI, any required dependencies and perform a connection test.
Reference: https://www.powershellgallery.com/packages/VMware.PowerCLI/12.3.0.17860403
VMware PowerCLI Development
Next, develop a script to extract and load data into BigQuery. Note that this requires a developer to have permissions to create a BigQuery dataset, create tables and insert data. An example process including code samples follows.
1. Import the PowerCLI Module and connect to the GVCE cluster.
2. [Optional] If desired, a vCenter simulator docker container, nimmis/vcsim : vCenter and ESi API based simulator, may be useful for development purposes. For information on setting up a vCenter simulator, see the following link: https://www.altaro.com/vmware/powercli-scripting-vcsim/
3. Create a dataset to hold data tables in BigQuery. This dataset may hold multiple tables.
4. Create a list of vCenters you would like to collect data from.
5. Create a file name variable.
6. For a simple VM inventory, extract data using the Get-VM cmdlet. You may also choose to extract data using other cmdlets, for example, Get-VMHost and Get-DataStore. Review vSphere developer documentation for more information on available cmdlets along with specific examples.
7. View/Validate the json data as required.
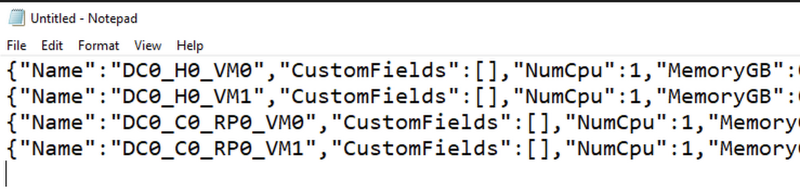
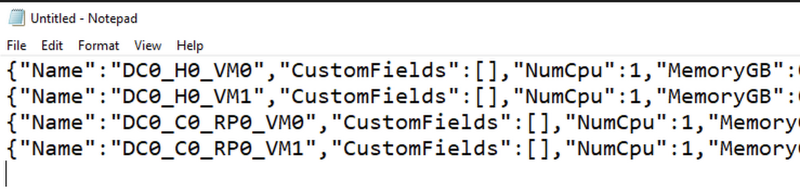
8. Create a table in BigQuery. Note that this only needs to be done once. In the example below the .json file was first loaded into a Cloud Storage bucket. The table was then created from the file in the bucket.
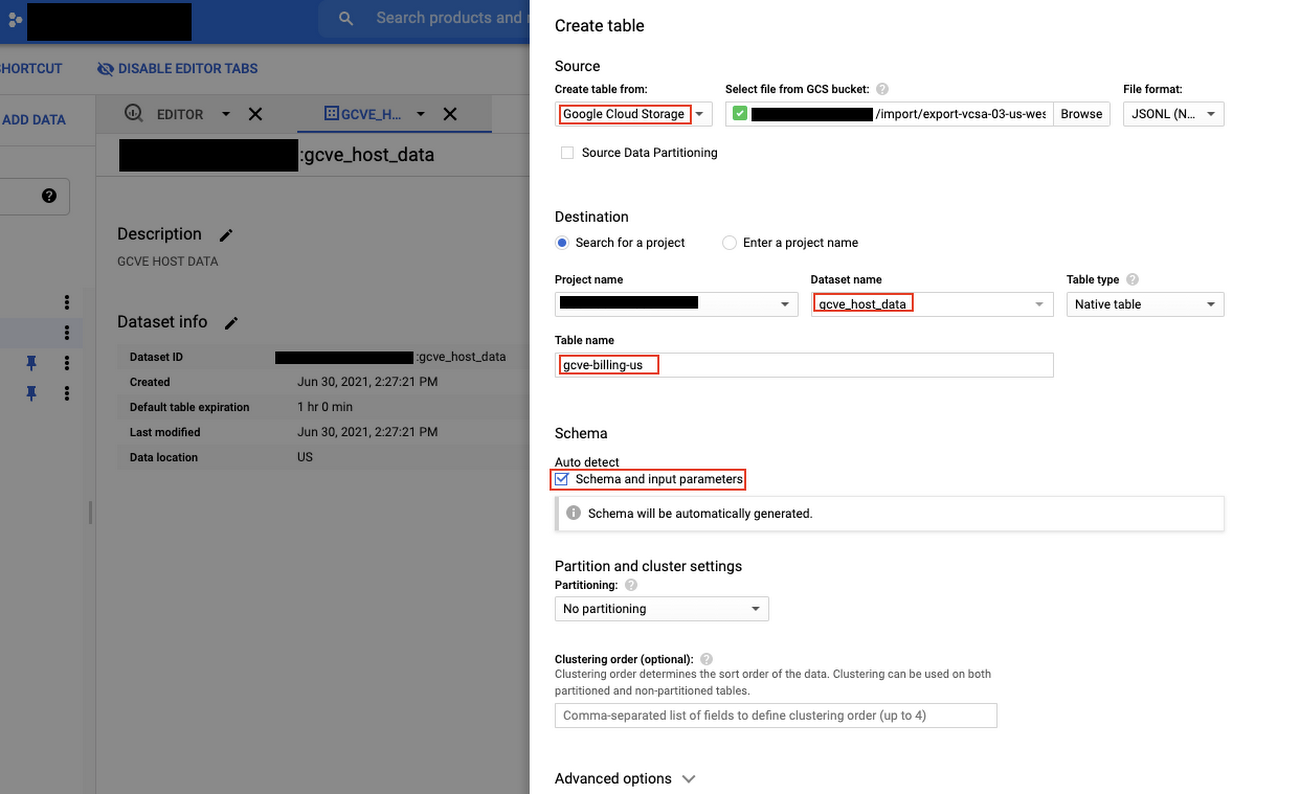
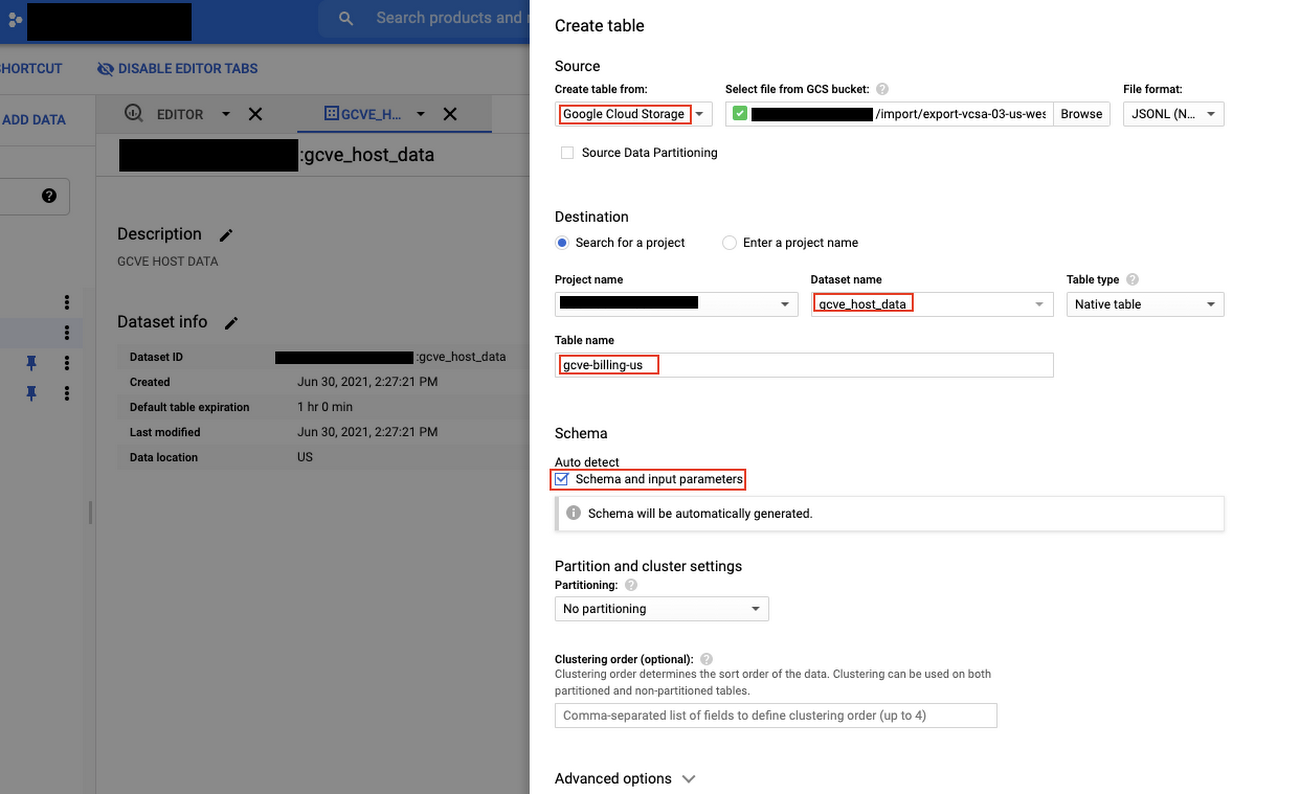
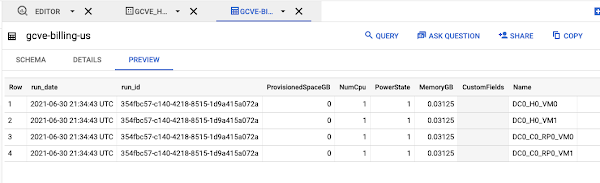
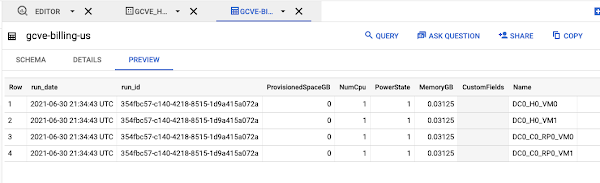
9. Load the file into Big Query.
10. Disconnect from a server.
11. Consider scheduling the script you developed using Windows Task Scheduler, cron or another scheduling tool so that it runs on the required schedule.
12. Using the BigQuery UI or Google Data Studio, create views and queries referencing the staging tables to extract and transform data for reporting and analysis purposes. It’s a good idea to create any supporting tables in BigQuery to support cost analysis such as a date dimension table, pricing schedule table and other relevant lookup tables to support allocations and departmental bill back scenarios. Connect to Big Query using Looker to create reports and dashboards.
Example: Connect to BigQuery from Google Sheets and Import data.
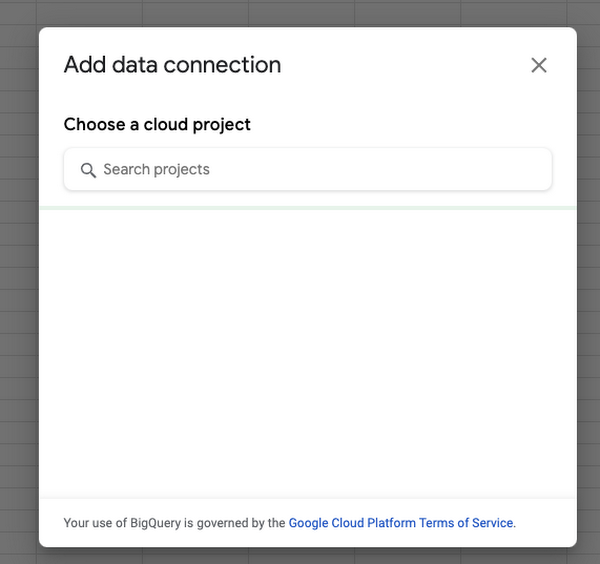
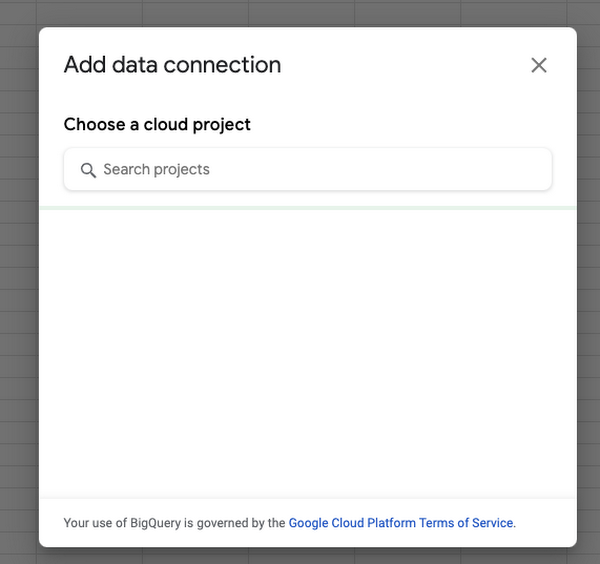
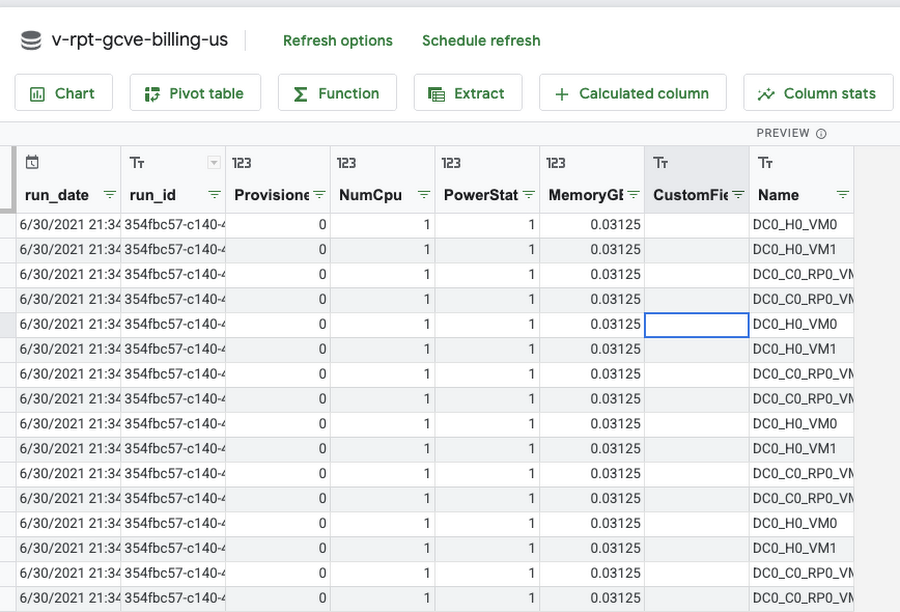
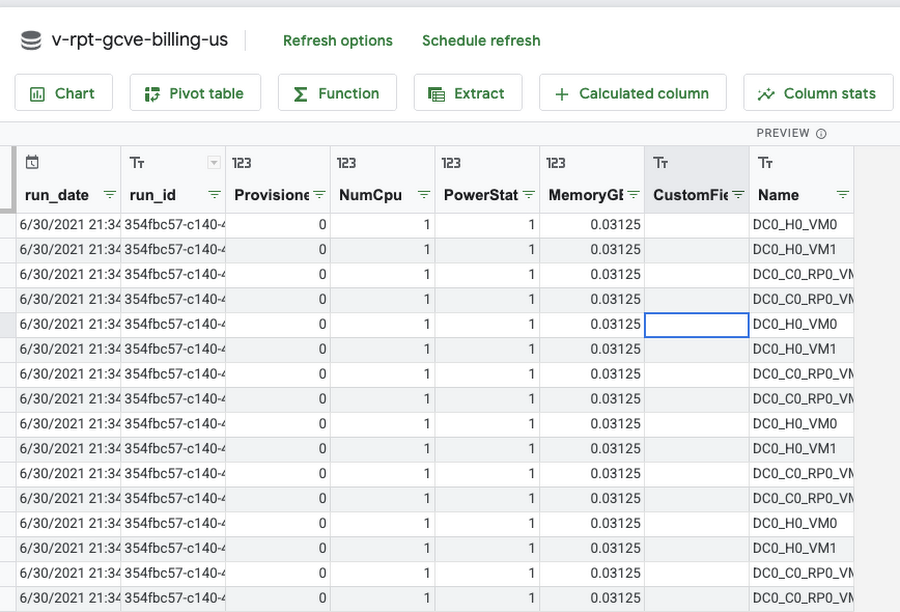
Using Custom Tags
Custom tags allow a GCVE administrator to associate a VM with a specific service or application and are useful for bill back and cost allocation. For example, vm’s that have a custom tag populated with a service name (ex. x-callcenter) can be grouped together to calculate direct costs required to deliver a service. Jump boxes or shared vm’s may be tagged accordingly and grouped to support shared service and indirect cost allocations. Custom tags combined with key metrics such as provisioned, utilized and available capacity enable GCVE administrators to optimize infrastructure and support budgeting and accounting requirements.
Serverless Billing Exports scheduled with Cloud Scheduler
In addition to running powershell code as a scheduled task, you may choose to host your script in a container and enable script execution using a web service. One possible solution could look something like this:
Create a Docker File running ubuntu 18:04. Install Python3, Powershell 7 and VmWare Power CLI
Example Docker File:
2. For your main.py script, use subprocess to run your powershell script. Push your container to Container Registry , deploy your container and schedule ELT using Cloud Scheduler.