IT prediction: Barriers between transactional and analytical workloads fall away
Andi Gutmans
GM & VP of Engineering, Databases
Try Google Cloud
Start building on Google Cloud with $300 in free credits and 20+ always free products.
Free trialEditor’s note: This post is part of an ongoing series on IT predictions from Google Cloud experts. Check out the full list of our predictions on how IT will change in the coming years.
Prediction: The barriers between transactional and analytical workloads will mostly disappear
Traditionally, transactional and analytical workloads have had separate data architectures. Transactional databases are optimized for fast reads and writes, while analytical databases are optimized for aggregating large data sets. As a result, legacy transactional and analytical data systems are largely decoupled from one another, leaving many teams struggling to find a way to piece together solutions that can support today’s intelligent, data-driven applications.
With a unified data platform, it’s possible to support both transactional and analytical workloads on the same data set — without negatively impacting performance. These modern data platforms are built on highly-scalable, disaggregated compute and storage systems and high-performance global networking, which means they can provide tightly integrated, scalable, and performant data services.
Let’s imagine, for example, that a bank wants to provide a personalized, real-time investment dashboard to its customers. To do this, it needs to integrate its app’s core banking features with market data. If the app’s backend is already optimized for transactional workloads, the challenge becomes maintaining app performance when you add analytical capabilities. The bank will also need to migrate all of its existing data to a data architecture that can analyze enterprise transactional data in real-time.
So, what new technologies can help remove the barriers between transactional and analytical workloads and make it easier to migrate existing data? Google Cloud’s new fully-managed PostgreSQL-compatible database, AlloyDB for PostgreSQL can analyze transactional data in real time, while being four times faster for transactional workloads and up to a 100 times faster for analytical queries compared to standard PostgreSQL, according to our performance tests. This kind of performance makes it the ideal database for these kinds of hybrid workloads.
You can migrate from PostgreSQL to AlloyDB with one click using our Database Migration Service, which lets you pre-define the data sources and destinations and then migrate using continuous replication, for minimal downtime.
In addition, we recently announced new capabilities like real-time data replication from transactional databases sources such as AlloyDB, PostgreSQL, MySQL, and Oracle directly to BigQuery with our change data capture and replication service Datastream for BigQuery. We also offer query federation with Cloud Spanner, Cloud SQL, and Cloud Bigtable, right from the BigQuery console, so you can analyze data in transactional databases. To learn more about the future of transactional and analytical workloads, check out my talk from Google Cloud Next ‘22.
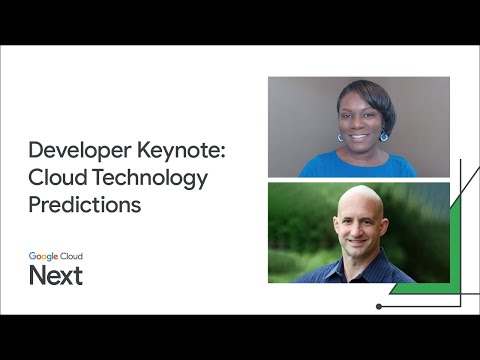