Using machine learning to help ZSL & Network Rail monitor and improve biodiversity near British railways
Anthony Dancer
Monitoring & Technology Lead, Conservation & Policy, ZSL
Omer Mahmood
Executive Sponsor, ZSL Partnership, Google Cloud


Network Rail recently commissioned international conservation charity, ZSL (Zoological Society of London) to develop cutting-edge methods for monitoring lineside biodiversity throughout the British rail network. Building on our longstanding partnership, we helped ZSL build a data and machine learning pipeline to analyse data captured during trial surveys in the Greater London Area.
British biodiversity
In Great Britain biodiversity plays a critical role in the health of our society. This is particularly true in urban environments, where the vast majority of people now live. Londoners, for example, share their city with over 14,000 species of plants and animals, which benefit urban residents in multiple ways: from lowering pollution and helping us to adapt to climate-driven heat waves, to reducing stress and improving mental health, to providing opportunities for physical activity and even food provision through pollinators.
Yet British wildlife is declining at unprecedented rates. The UK has lost nearly half of its biodiversity to urban spread and agriculture since the industrial revolution, ranking in the bottom 10% of nations globally and worst among G7 countries. These declines continue today: the 2019 UK State of Nature report found a further 13% decrease in the average abundance of species since scientific monitoring began in the 1970s.
How railways could help
Great Britain’s vast railway network has the potential to play a critical role in conservation of British wildlife, as well as providing a greener way to travel.
Rail networks frequently involve extensive tracts of green space, including verges, unused tracks, and other lineside landholdings. As a result, railways can have multiple, important effects on biodiversity, from acting as refugia for wildlife in urban or intensive agricultural landscapes, to connecting fragmented habitats and enabling species movement via corridors, or even acting as barriers to species dispersal where tracks divide habitats.
In Great Britain, it’s Network Rail’s job to maintain railway land to provide a safe and reliable service for passengers and freight customers, and balance that with supporting the rich range of wildlife that those linesides support. The company is also one of the country’s largest public landowners, with a total estate of approximately 52,000 hectares across its 20,000 kilometres of railway corridor. These landholdings traverse all major British terrestrial habitat types, and are home to a rich array of rare, protected, and valued species. With careful monitoring and management, the rail network could help protect and restore our wildlife, for the benefit of nature and people.
What does Network Rail hope to achieve
Network Rail have committed to an ambitious vision, via their 2020 Biodiversity Action Plan, for improving lineside biodiversity, including achieving no net loss in biodiversity by 2024, biodiversity net gain by 2040, and maximising the value and connectivity of their landholdings as wildlife corridors.
To help support monitoring progress towards these commitments, Network Rail sought the assistance of ZSL, with its deep experience in wildlife monitoring. The purpose of this preliminary study was to trial a range of remote and automated technologies for monitoring wildlife and anthropogenic activity, including networked and standalone cameras, and innovative acoustic sensors, and to evaluate the viability of the proposed methods for tracking Network Rail’s progress towards their biodiversity mission.
Using Vertex AI and Looker Studio to identify and map species to the Network Rail estate
ZSL captured 35,000 data files, or 3000 hours of audio, captured by 33 acoustic monitors placed across Network Rail’s estate in South London during 2022. Working with Google Cloud, the ZSL team was able to identify "lineside habitats" using this data.
The initial data analysis targeted bird and bat species. To do this, they leveraged three different pre-trained machine learning models called BirdNet, BatDetect, and CityNet to detect birds, bats, and anthropogenic sounds respectively.
Using a combination of these models, they were able to identify the type of species, and map the occurrences to a geographical location.
For each audio file in the dataset they needed to answer the following questions:
Does it contain a bat or a bird sound?
What is the predicted species?
What is the confidence level?
To speed up the analysis and find answers to these questions, the team leveraged the pre-trained models in combination with Vertex AI. The managed notebook experience in Vertex AI allows data scientists to rapidly prototype and test machine learning pipelines, without needing to worry about the underlying infrastructure.
The first step was to mount the data ZSL had collected using Cloud Storage FUSE (File System in User Space), which allowed the team to access the audio data files from within the notebook. Then the team created steps in the notebook for getting predictions from the data using the pre-trained models, and generated results which were pushed back to Cloud Storage for further analysis.
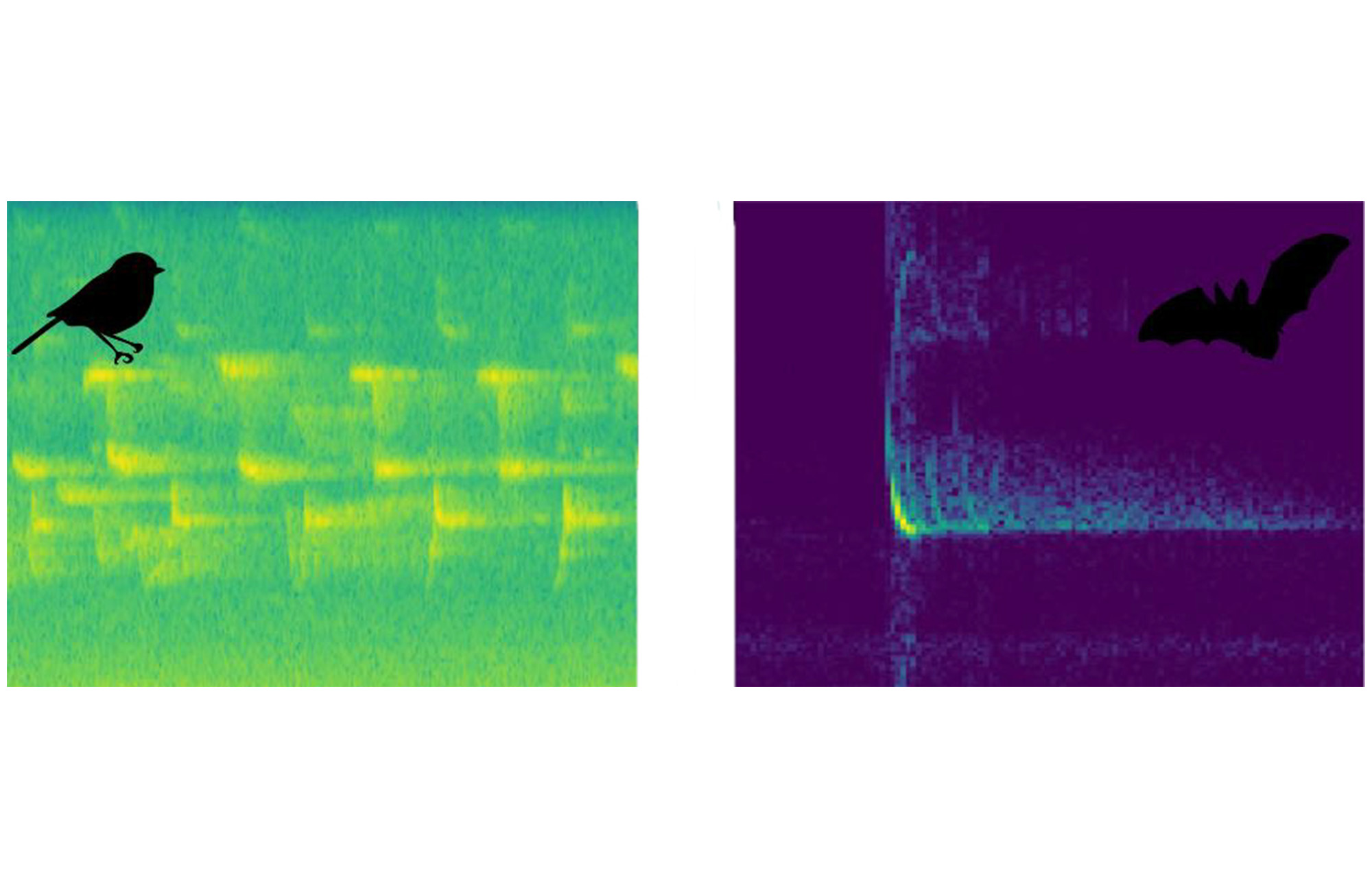
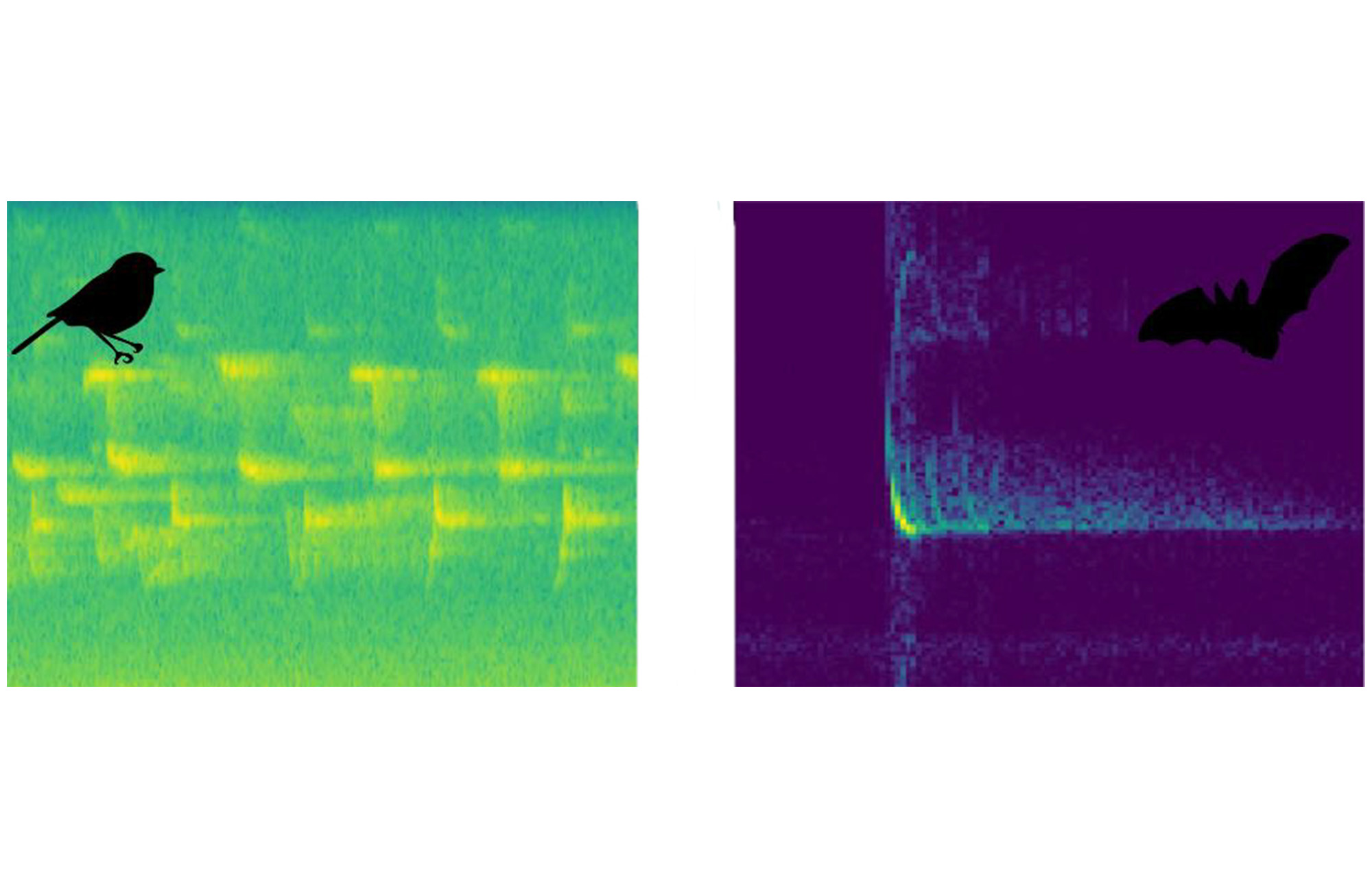
Once the predictions for each model were run on all Network Rail audio recordings, the data was further transformed in BigQuery to calculate the frequency of each species for each geographic location, and other trends. This process involved taking the predictions from each model and combining them to create a single prediction for each species. The predictions were then transformed into a frequency count, which is used to calculate the relative abundance of each species at a given location.
The final transformed data in BigQuery was visualised in Looker Studio to map the biodiversity denoted for each species by volume on a map. It also allows the user to specify the time window that classifies as a unique sighting of that species.
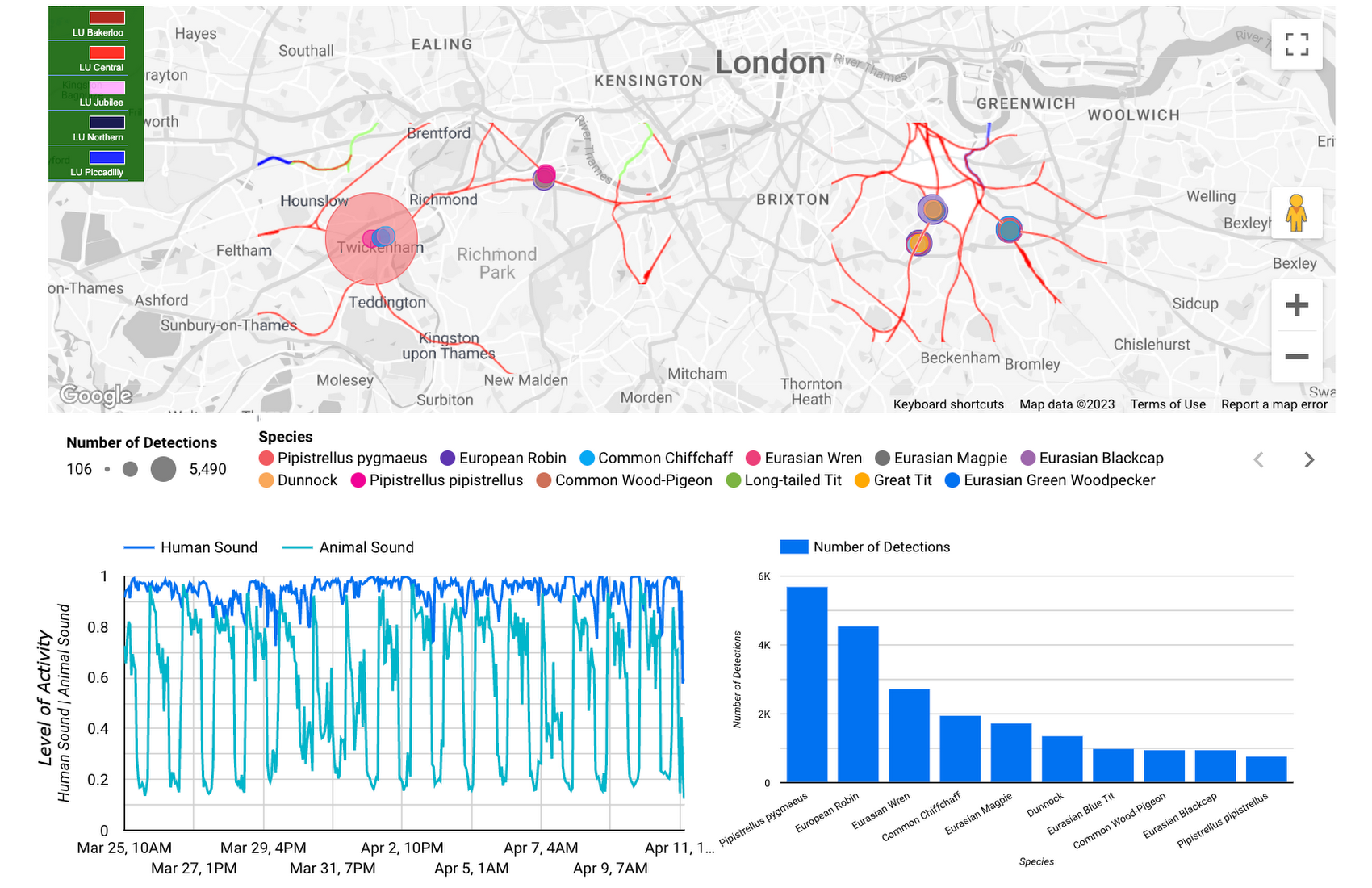
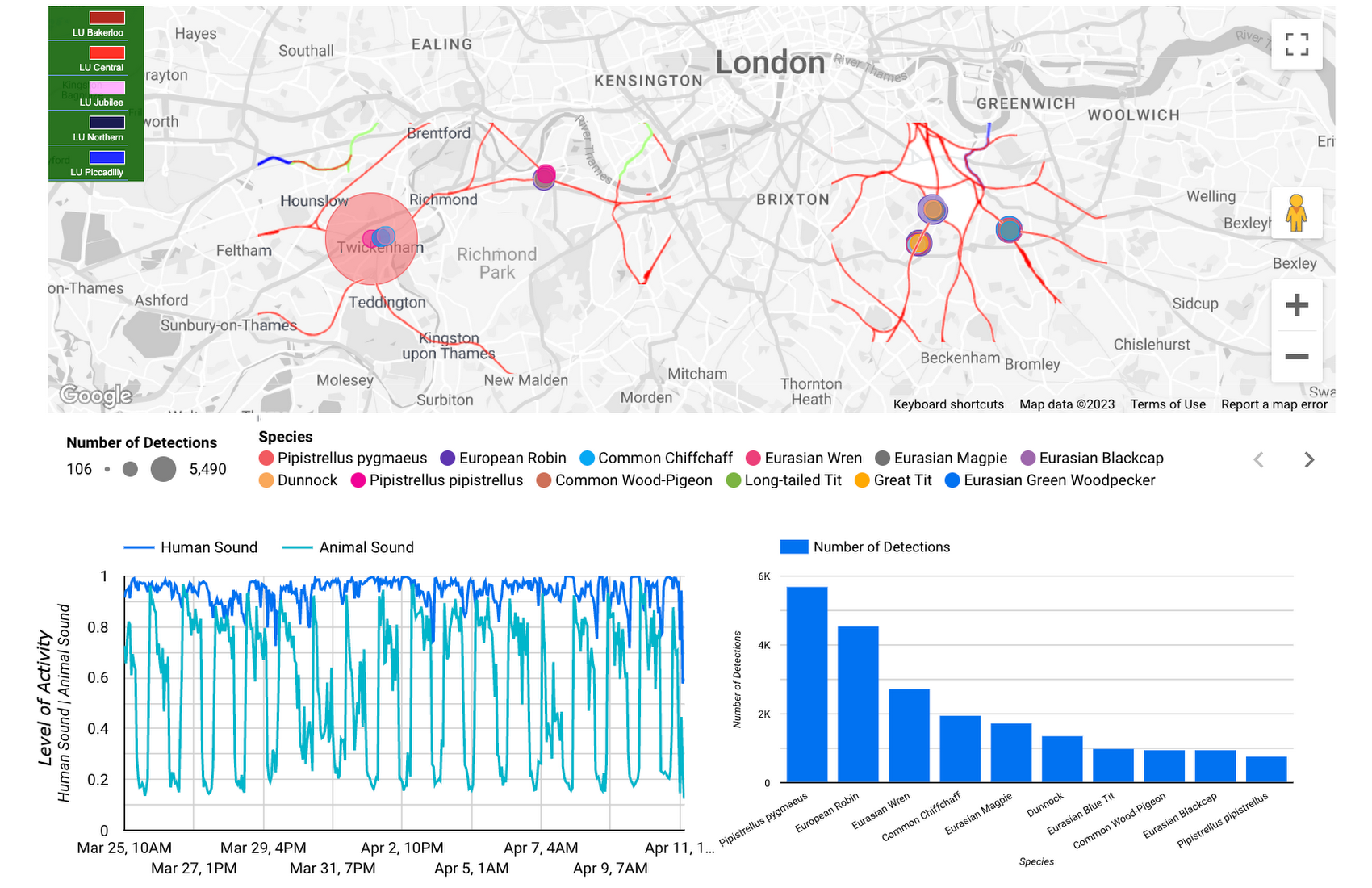
Next steps
ZSL intends to use the methods and tools developed during this project to help Network Rail monitor, understand and improve its impact on lineside biodiversity, with a focus on technologies which allow safe, rapid, remote monitoring. The application of these innovative techniques for monitoring lineside biodiversity is a highly emergent field.
The study also uncovered indicator species, which are species that are used to assess the health of an environment. For example a recent study of bird species identified the Eurasian Blackcap, Blackbird, and Great Tit as the top three species to indicate high environmental quality in urban areas. This is due to their requirement for an abundance of invertebrates and connected habitats that support the growth of berries, fruit and nuts. All three of these species were detected in the acoustic dataset for Barnes, Twickenham, and Lewisham, providing an important benchmark for biodiversity.
The study suggests that acoustic sensors are an effective method for surveying UK conservation concern species. The data collected from these surveys could be used to identify priority areas for conservation and to monitor the effectiveness of conservation measures.
This year we'll start to build out these surveys to new locations and for more species (e.g. invertebrates, and small mammals). We’ll also begin assessing the difference that lineside habitat makes to British biodiversity, and evaluate how Network Rail’s conservation and habitat management could be improved, while developing and testing new remote monitoring tools and data pipelines, for implementing monitoring at scale across the rail network in the years to come.
Special thanks to the following contributors to this post: Michelle Liu, Lydia Katsis and Neil Rutherfoord.