Google Cloud, Harvard Global Health Institute release improved COVID-19 Public Forecasts, share lessons learned
Tomas Pfister
Head of Google Cloud AI Research
Harvard Global Health Institute and Google Cloud have been working the past three months to improve the COVID-19 Public Forecasts, to give first responders and healthcare organizations the best possible information to prepare for what lies ahead.
These forecasts use AI to provide a projection of COVID-19 cases, deaths, and other metrics for U.S. counties and states. Since their original release, the COVID-19 Public Forecasts are now used by many organizations across the United States, and have been significantly improved in five major ways:
1. Longer forecasts & confidence intervals. When initially launched, the COVID-19 Public Forecasts included predictions for 14 days in the future. They now include predictions for a 28-day horizon. Because predictions in general become more uncertain as they predict events further into the future, we have added confidence intervals to help users model that uncertainty.
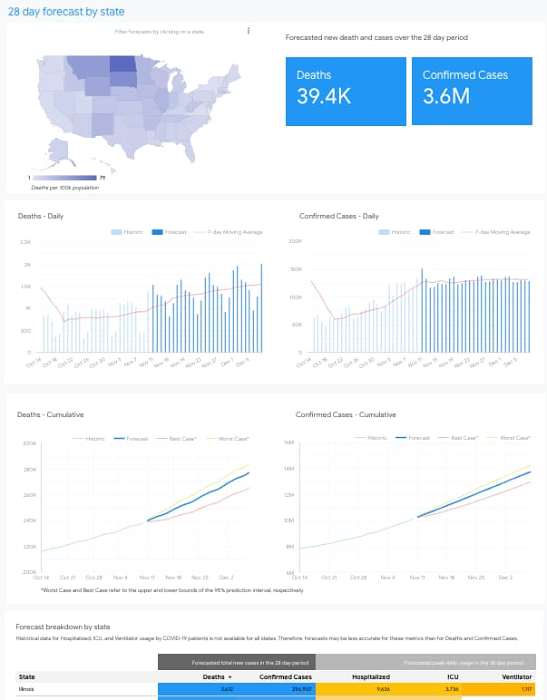
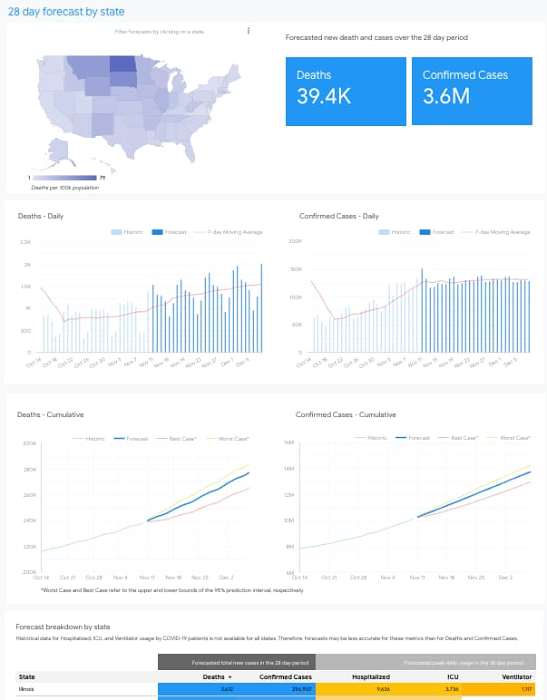
2. Improved model quality. A dedicated team has been continuously improving the model quality with cutting edge AI research. A white paper detailing some of these innovations was published in the machine learning conference, NeurIPS 2020. The model was one of the few to correctly forecast a surge in cases in October and November. The accuracy of the model has continuously improved over time and is retrained daily as more data becomes available. Since the forecasts were first published, we’ve seen the predictions improve by approximately 50%.
“The COVID-19 Public Forecasts is an important public health tool for guiding the policy response to the COVID-19 pandemic. By providing an ‘early warning system’ of COVID-19 cases, hospitalizations, ICU admissions, ventilator utilization, and deaths, the COVID-19 Public Forecasts create the opportunity for public health officials and policymakers to move from a reactive to a proactive approach to suppress the pandemic,” said Dr. Thomas Tsai, MD, MPH, surgeon and health policy researcher at Harvard T.H. Chan School of Public Health. His research team is using the COVID-19 Public Forecasts to develop state and national testing targets to guide a testing strategy around screening of asymptomatic individuals to suppress the silent transmission of SARS-CoV-2.
3. Ability to expand to other countries. We have added support for expanding the COVID-19 Public Forecasts to other countries, and today we are launching forecasts for Japan. As with the United States, these forecasts are free and based on public data such as the public COVID-19 Situation Report in Japan. The model predicts confirmed cases, deaths, recoveries, and hospitalizations, per day, and will look ahead 28 days into the future. Japan is made up of several prefectures and we offer these forecasts for each one. This information is available now on the Japan forecast dashboard.
“We validated this COVID-19 forecast model for Japan from the academic perspective. The forecasts will be useful to professionals that understand the capabilities and constraints of the model, and will play a critical role for Japan’s public health and enhance our ability to understand and respond to the rapidly evolving COVID-19 pandemic. Coupled with other existing works such as Keio University’s COVID-19 response surveys partnering with Ministry of Health, Labour and Welfare and prefectures, this model will allow for more proactive and efficient public health interventions on a prefecture-by-prefecture basis," said Prof. Miyata, Department of Health Policy and Management, School of Medicine, Keio University.
4. Customized forecasts. Since the launch in August, we have worked with many organizations to better understand how these forecasts can help. In the process we have learned that many organizations have specific needs that go beyond just consuming our public forecast, such as wanting to use their own datasets as inputs. To that end, we have turned the initial forecasting model into a system that is customizable to new problems and datasets. We are working with public sector and healthcare leaders to help them create custom forecasts for their states and hospitals.
5. What-if analysis for informing policy decisions. We have also seen significant interest in using the forecasting model to ask “what-if” questions to help make better-informed policy decisions. For example, you can see how the forecasts change in response to policy changes such as if non-pharmaceutical interventions (e.g. mask mandates) are introduced, reopening plans are changed, or vaccination policies are modified. To that end we have been developing a novel AI-driven what-if model to be used for COVID-19 and other infectious disease decision making. We hope that it will be helpful for organizations interested in doing vaccine rollout planning and other important decision making that may impact COVID-19 outcomes. If you or your organization are interested in exploring this tool, please contact us at COVID19-public-forecasts-feedback@google.com.
Lessons learned
Over the course of the development of the COVID-19 forecasts, our team was faced with the risk of launching too quickly. On the one hand, the potential impact of more accurate and robust forecasts on the COVID-19 response was large, so launching quickly was important. However, we also needed to make sure that the quality was high enough to help inform decision makers as well as ensuring that it did not further any existing disparities through model bias.
The following sections share some background about our journey leading up to the original launch of the COVID-19 Public Forecasts.
Googlers across Alphabet came together in March and dove into literature to understand epidemiological forecasting, discover the best public datasets on which to train the model, build the infrastructure to train massive machine learning models, and design novel AI for time series forecasting. Over a hundred Googlers worked over many months to make sure the forecasts were robust, accurate, and fair.
Combining cutting-edge machine learning with traditional epidemiological models. Most AI forecasting models learn from data, such as forecasting weather based on historical data. In contrast, most COVID-19 forecasting models do not learn from data but simulated spread according to human epidemiological assumptions. We designed a new kind of time series forecasting model that learns from both epidemiological human prior knowledge as well as data. Another significant challenge was to design systems that learn in a non-stationary environment: interventions such as mask and movement restrictions change frequently and sometimes in response to forecasts – progression of the disease influences public policy and individuals’ public behaviors and vice versa.
Building on public Google Cloud products. From the beginning we knew that the tool we were creating would likely be shared broadly with the public and many organizations. That drove our decision to build this software on our public Google Cloud products, including Kubeflow pipeline, GCP hyper-parameter tuning, Kubernetes, BigQuery, Google Cloud Storage, and Cloud SQL. Using our own products helped us prepare our forecasts more quickly.
Improving robustness of forecasts. To help make sure we were developing a useful forecast, we partnered with the Harvard Global Health Institute who guided us on how to maximize policy-making impact and ensure the forecasts would be useful to those who most needed it. Additionally, we partnered with a handful of early testers, including HCA Healthcare, to help us understand what should be forecasted, how it is formatted, and even test early versions of the forecasts. These efforts helped improve the forecasts before they were made available to the general public. We also brought in experts within Google with statistical and epidemiological expertise to ensure our work met the highest scientific standards. We designed a daily forecast launch process that first runs over 100 checks looking for any abnormalities, and we required a manual review for a qualitative analysis to check for issues. Every day our model training searches over hundreds of hyperparameter options, and the team works to ensure the best models reach our users.
Fair and equitable forecasts. It was important to us that the forecasts were reliable and robust. Given the disproportionate impact that COVID-19 has had on communities of color in the United States, we conducted a fairness analysis, looking at how both relative and absolute errors are different across various groups (particularly African American and Hispanic populations) and interpreting the results. We wrote up our findings in a public Fairness Analysis.
We’re excited by what we were able to achieve in the eight months since this effort began, but more importantly, we’re proud of the people who have come together to make this small difference in the fight against the global pandemic and grateful for all of those on the front-lines saving lives, innovating, and bringing the world one step closer to returning to normal.
If you have any questions about the COVID-19 Public Forecasts (g.co/covidforecast), customizations or what-if analysis, please contact us at COVID19-public-forecasts-feedback@google.com.