How AI-curated suggestions serve a better client experience at Richemont
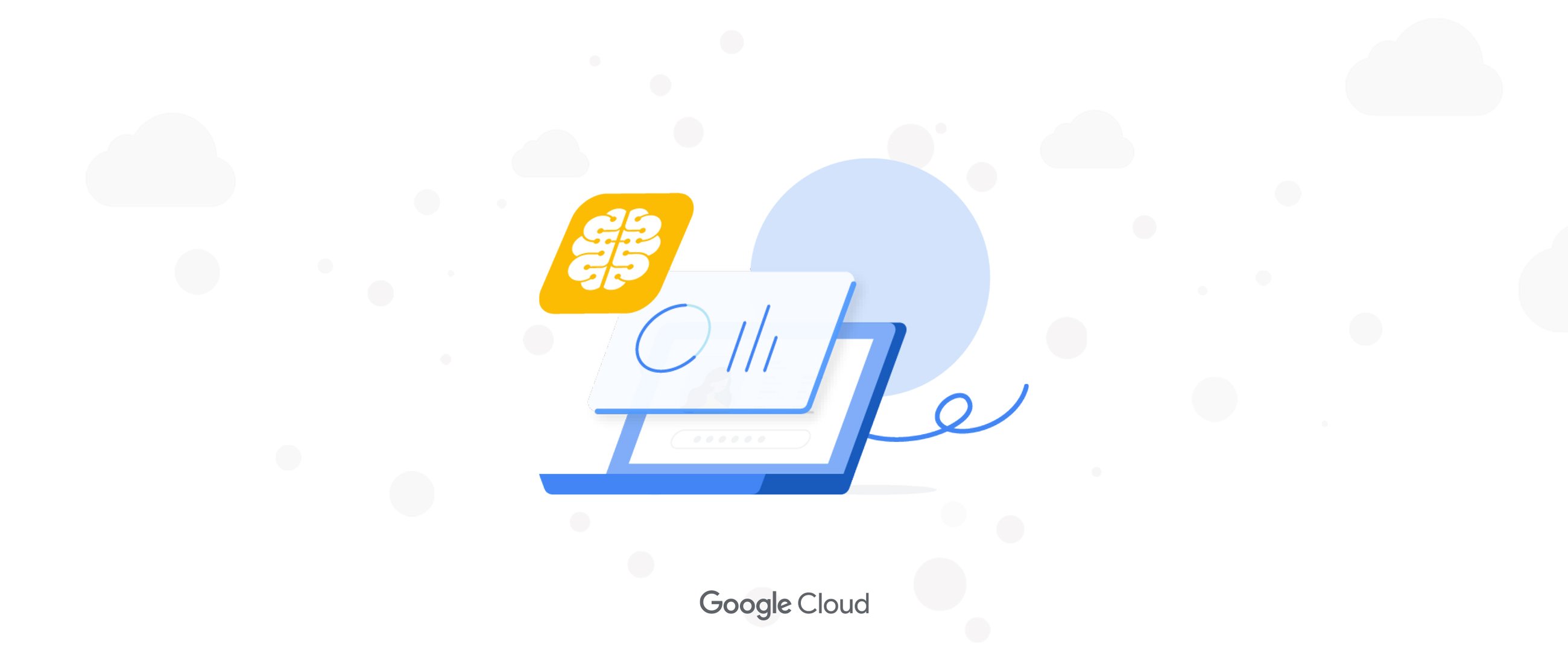
Manuel Altermatt
Enterprise Field Sales Manager, Google Cloud
Phillippe Meyer
Group Client Marketing Director, entity Richemont International SA, Richemont
Whether they meet customers online, offline, or in some combination, retailers share a big problem: How can they offer the right choices, when and how the customer wants, without overwhelming (and often losing) the buyer?
More than anything, this is an information problem. As such, it's a good candidate for using artificial intelligence (AI) for greater success. Here's how Richemont tackled the problem.
Richemont owns a portfolio of leading luxury goods brands, recognized for their distinctive heritage, craftsmanship and creativity. It has strengths and specialties in jewelry (Cartier, Van Cleef & Arpels), luxury watches (IWC, Jaeger-LeCoultre, Panerai, Vacheron Constantin), and fashion & accessories (Chloé, Montblanc, dunhill).
People shop for such goods in a number of ways, from online searching to individual meetings in boutiques, and Richemont must be prepared for every context. Understanding which shoppers are likely to buy or repurchase, when to engage directly, and what creation to suggest enables sales associates to spend quality time with clients, engaging at the right time with meaningful advice. Richemont solves these retail challenges with an integrated Client Platform leveraging Google Cloud and its AI/ML capabilities.
Enticing peoples’ desires with Machine Learning
Richemont began by posing two questions:
Which prospects or clients need extra attention? Specifically, who is likely to convert or to repurchase?
What would be meaningful items to suggest to each client and prospect?
Both questions were addressed with machine learning algorithms. Their challenges included deploying and monitoring algorithms at scale for several brands across the globe, while addressing the specific business needs for each brand. For instance, it may be more relevant to recommend in-season items for fashion brands, while for watchmakers it is more about cross-fertilization across each brand's iconic creations.
This graph summarizes the prediction process implemented by Richemont:
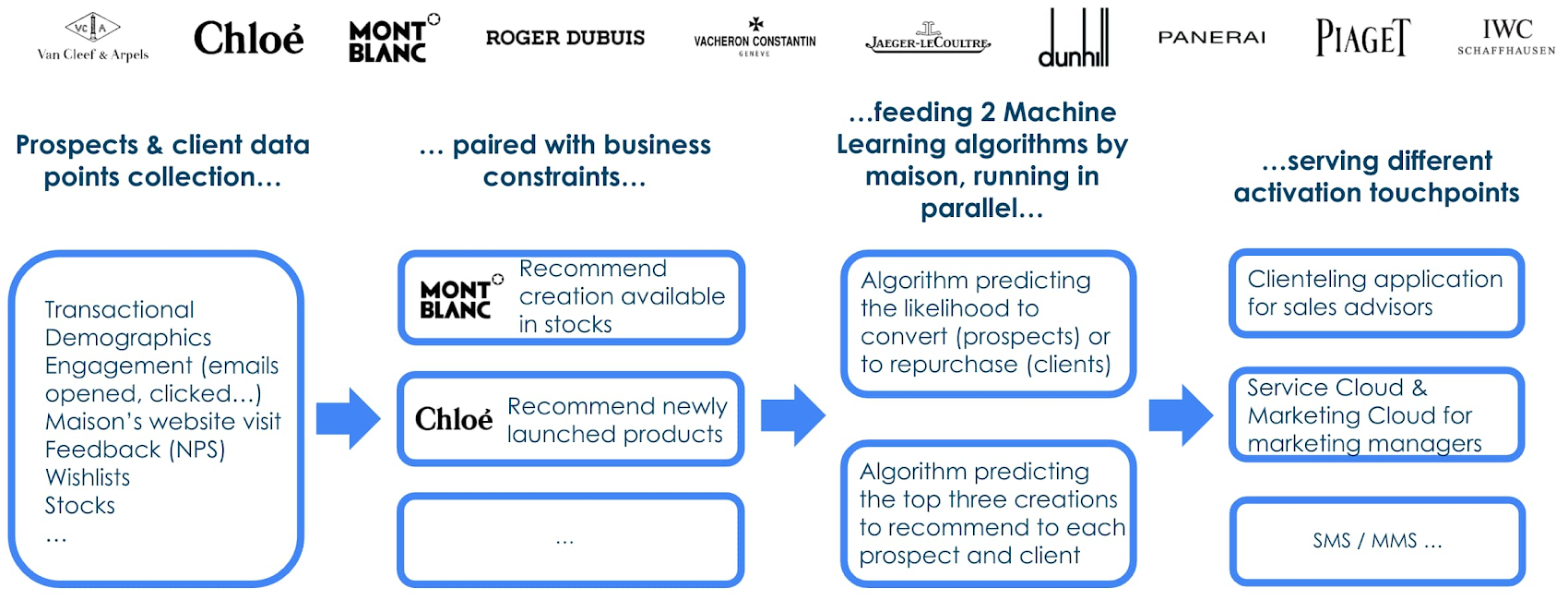
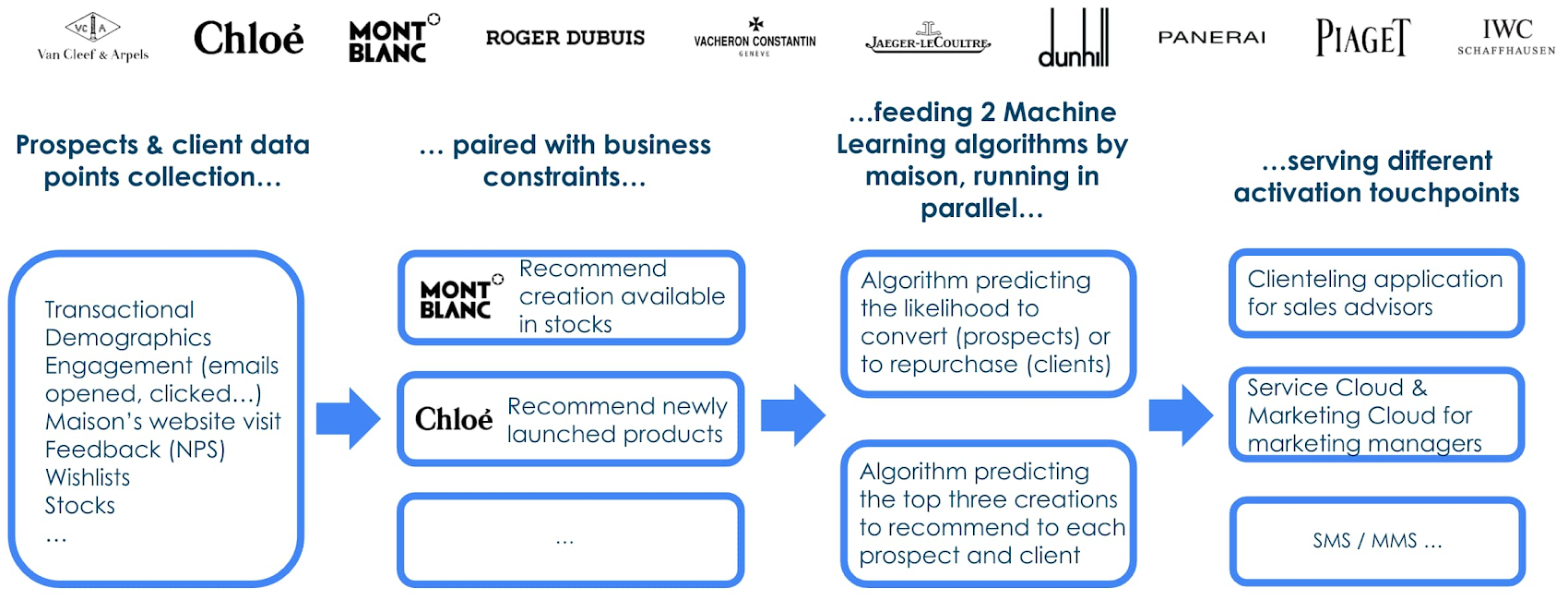
Engagement data (email opened, clicked, SMS/MMS, website visits…) was found crucial to predict conversion of prospects for whom per definition no transaction history is available. For website interactions Richemont leverages the Google x Salesforce Connector.
To deploy the Machine Learning algorithms and to monitor them, Richemont leveraged Vertex AI, along with BigQuery, Cloud Functions and Google Storage, all orchestrated with Google Cloud Composer.
The role of product recommendation algorithms
Richemont used the deep learning library TensorFlow Recommenders to perform the product recommendation tasks. This library enables companies to build state of the art deep learning algorithms to achieve relevant and robust predictions.
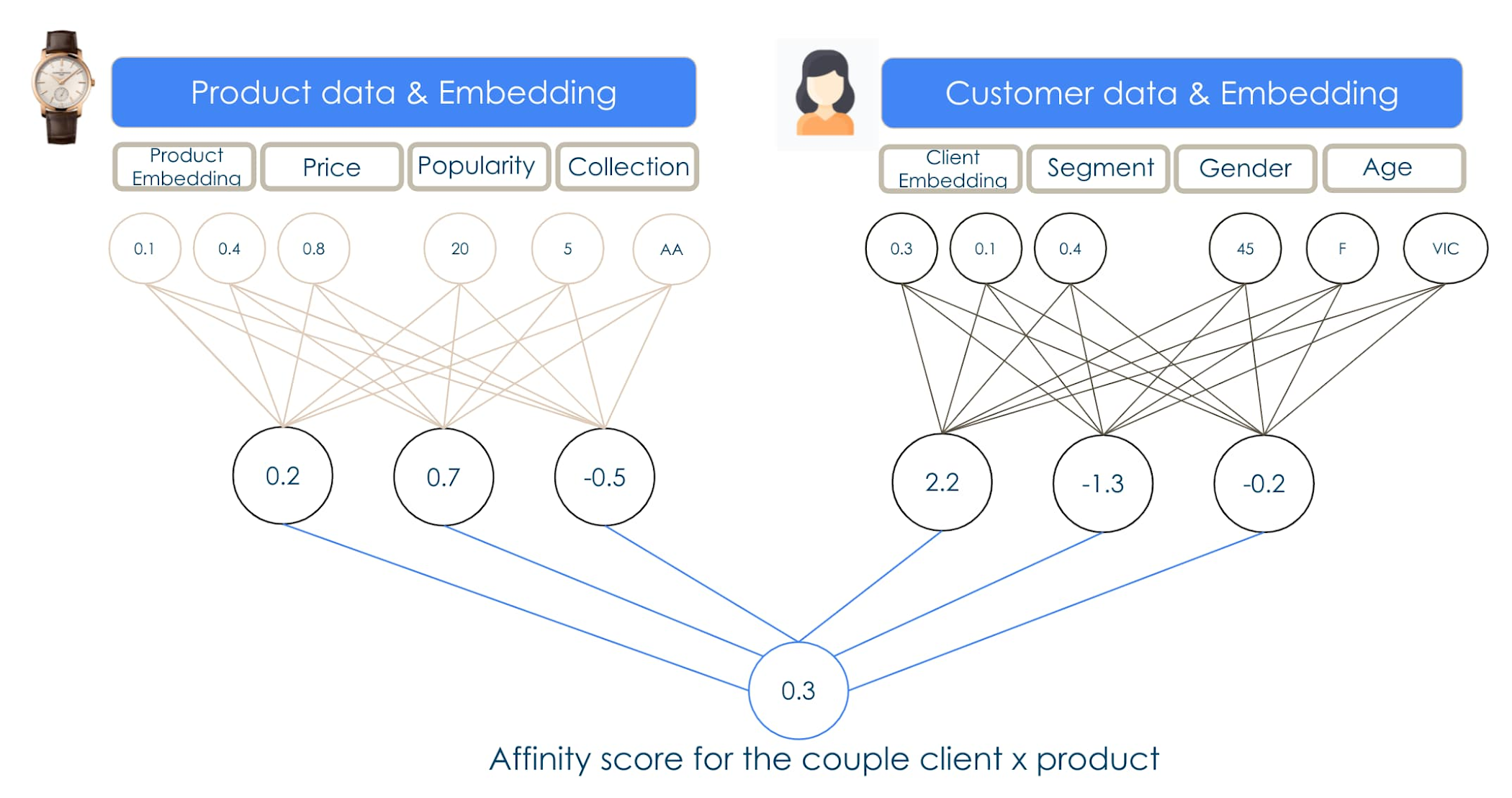
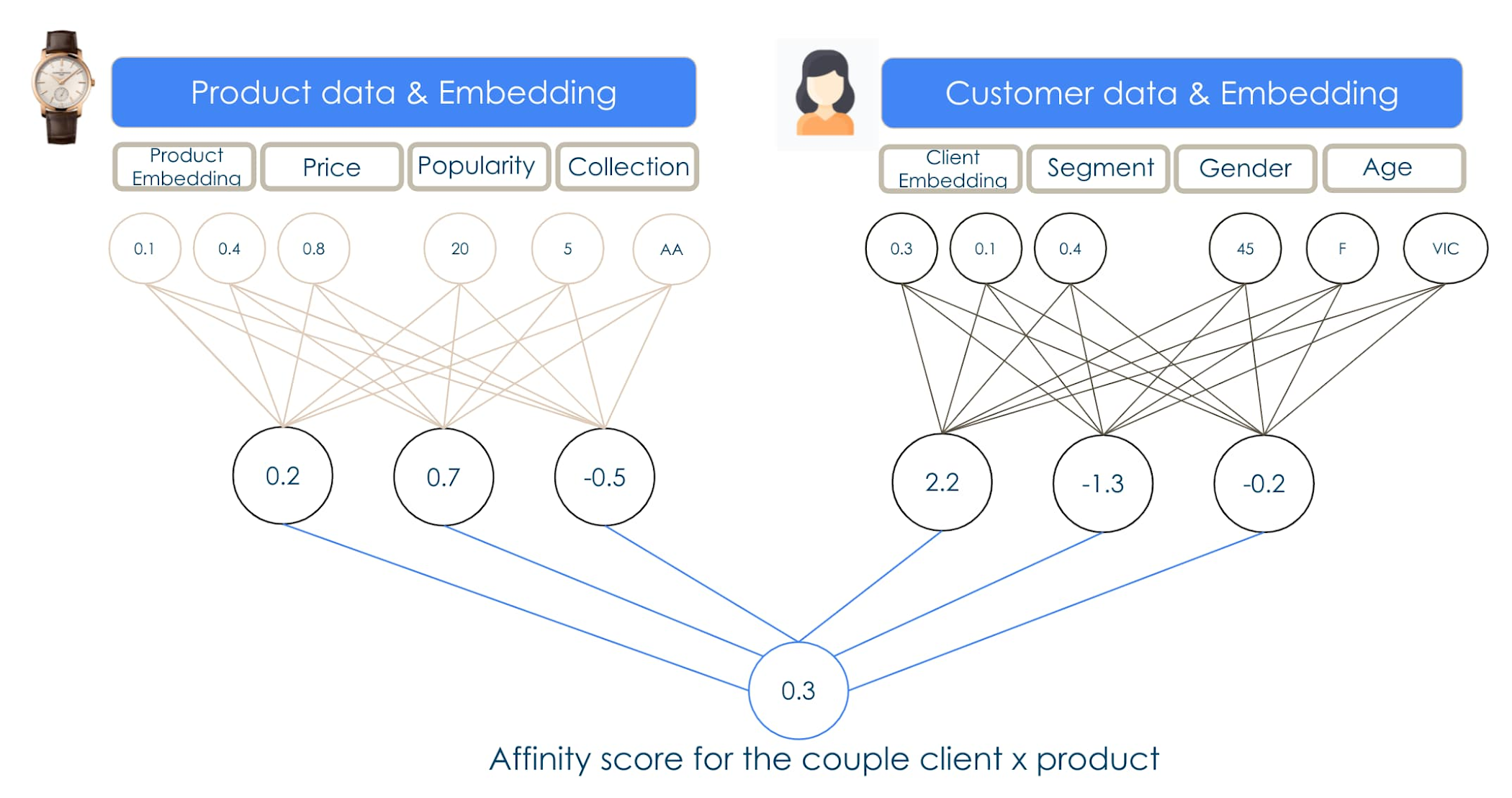
Unlocking client value with integrated technology
Richemont's innovations show how technology that considers many parts of the customer experience creates more value. In this case, the company used in store applications to invite people with a strong propensity to buy for boutique visits, while others at a different point in the purchasing journey were offered different options more suited to their tastes and inclinations.This solution, now deployed across 11 brands in over 25 countries, shows just one way that AI can improve customer experience, for better customer loyalty.
Key to the process, here and elsewhere, is the way a retailer and its partners put customer understanding at the center of the process. As AI becomes more important not only in retail, but in every industry, this human understanding will become even more important as a fundamental organizing principle. Much is changing, but once again, the winners will be the companies that focus best on their customers.