The 4 stages of machine learning: From BI to ML
The Google Cloud content marketing team
For today’s enterprises, integrating machine learning (ML) technologies offers a host of benefits: higher productivity, lower customer churn, actionable data, and more. Some companies may be able to do this quickly. Others may choose to take a staged approach. Here are some steps that organizations can take to move from a business intelligence strategy to a machine learning one.
Stage 1: Collect and prepare data
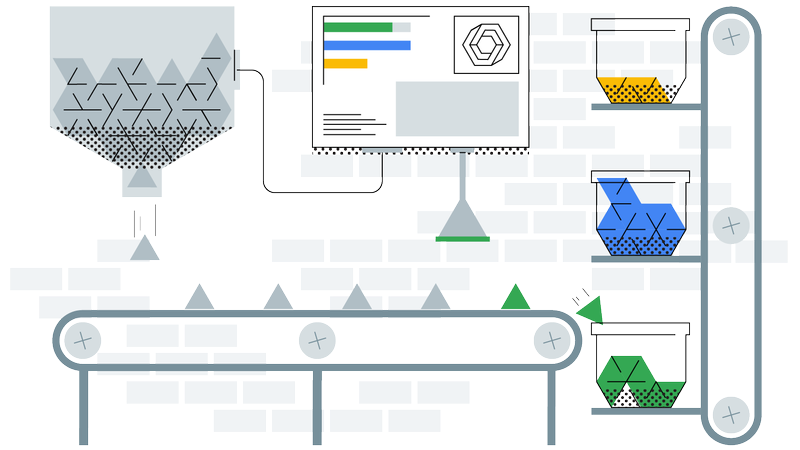
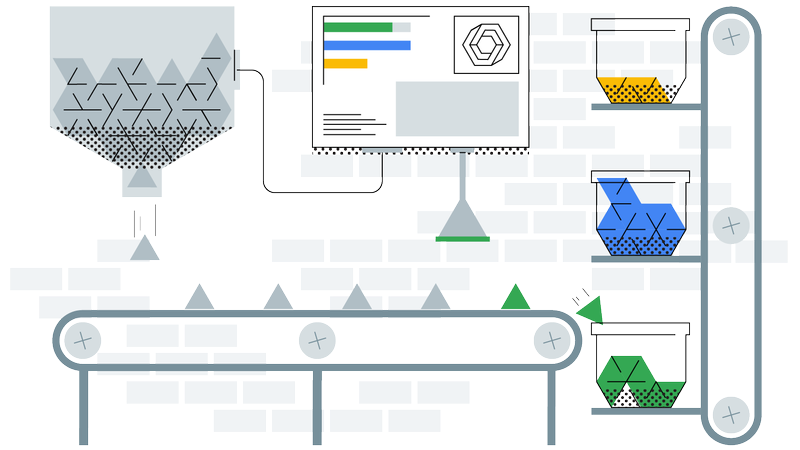
For most organizations, the first and easiest action happens to be the one that often delivers the biggest impact—that is, to organize where enterprise data lives and where it is coming from. Cloud storage makes it easy to collect all available data and its variables, which is important, since data that doesn’t seem useful now may be useful in the future. To be effective in ML applications, data should be time-stamped and labeled with outcomes when known—whether a loan was approved or denied, for example. Disparate data sources like online product reviews, email surveys, and support call transcripts can be consolidated, turning fragmented information into comprehensive knowledge bases.
Stage 2: Make sense of data
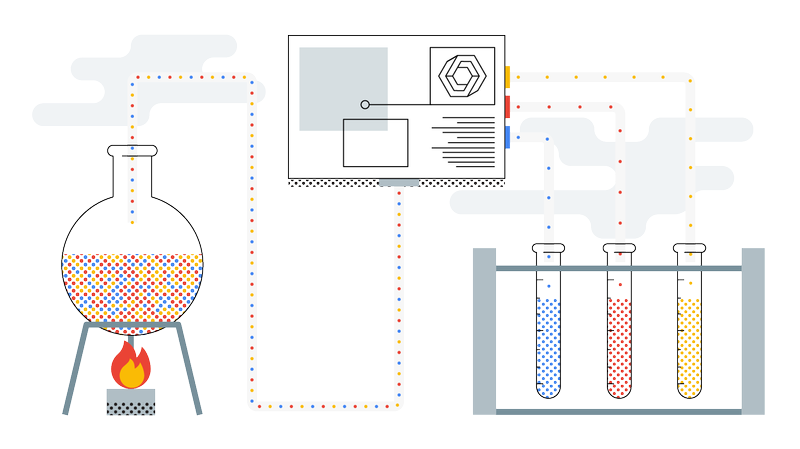
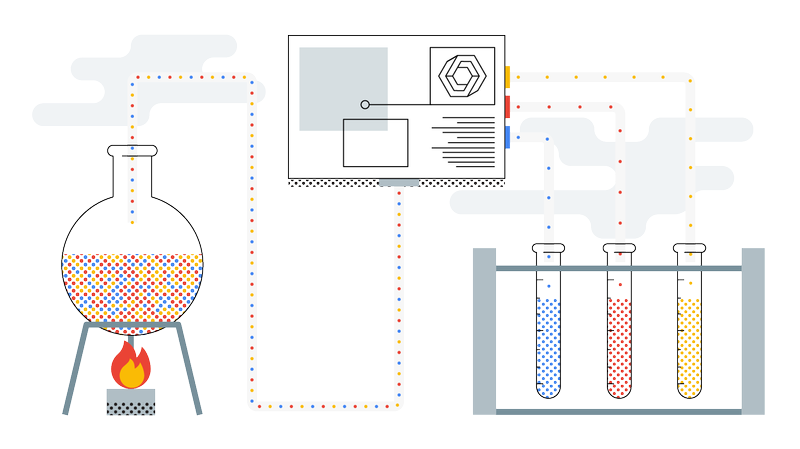
Once you’ve collected and prepared your data, you can distill business insights from it using ML applications. These applications may also allow you to do operational reporting, continuously observing real-time data as it’s generated. Use adaptive web applications to learn from user behavior and automatically optimize content for engagement, or build location-specific pricing models that reflect seasonal demand fluctuations.
Stage 3: Use data to answer questions
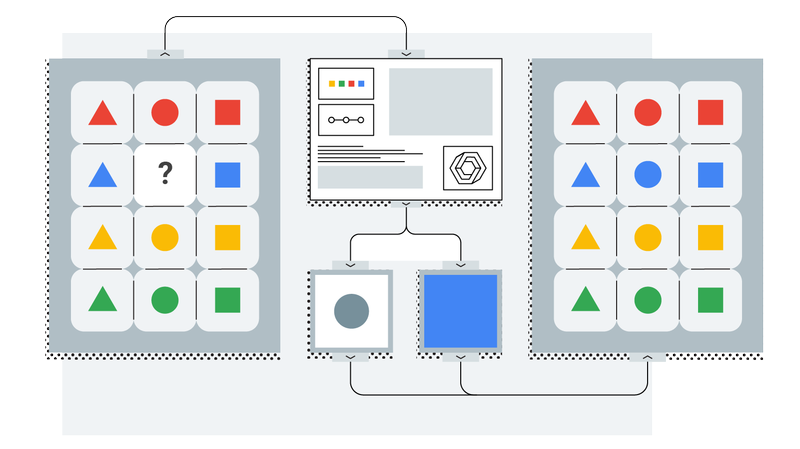
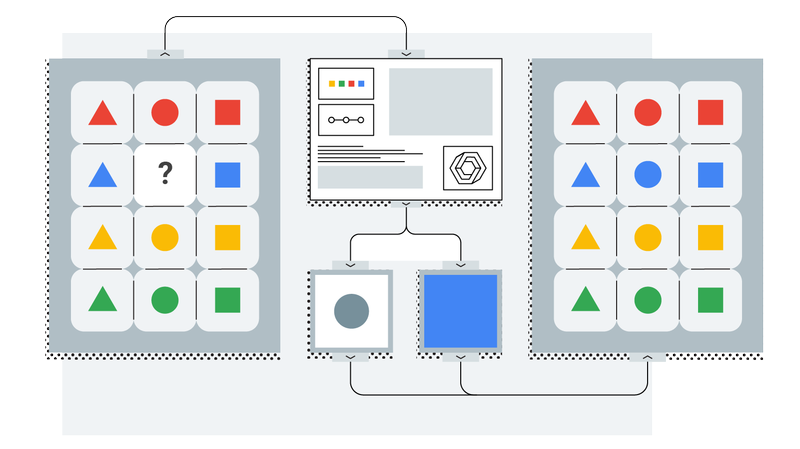
Data can be used to answer business questions and train ML models that can then be used in your applications. Why do some customers abandon online transactions? Why does a product appeal to one consumer but not another? ML can identify data patterns to uncover the “why” as well as the “what.” Emerging unsupervised ML models can self-learn patterns to deliver answers even when input data is unlabeled and has unknown outcomes.
Stage 4: Create predictive applications
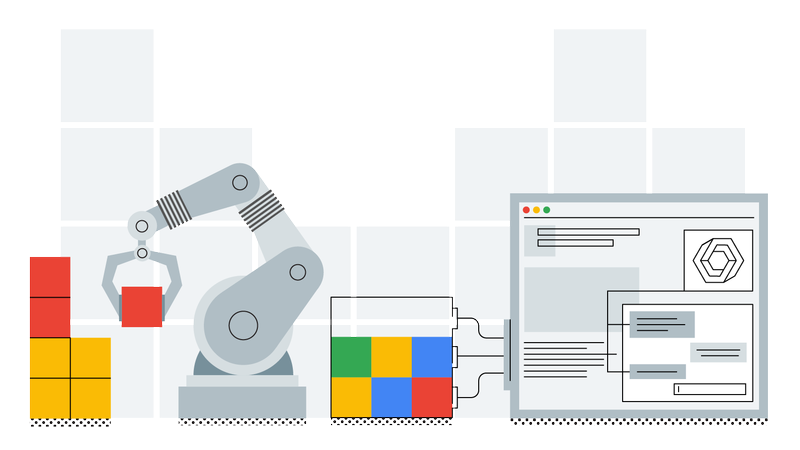
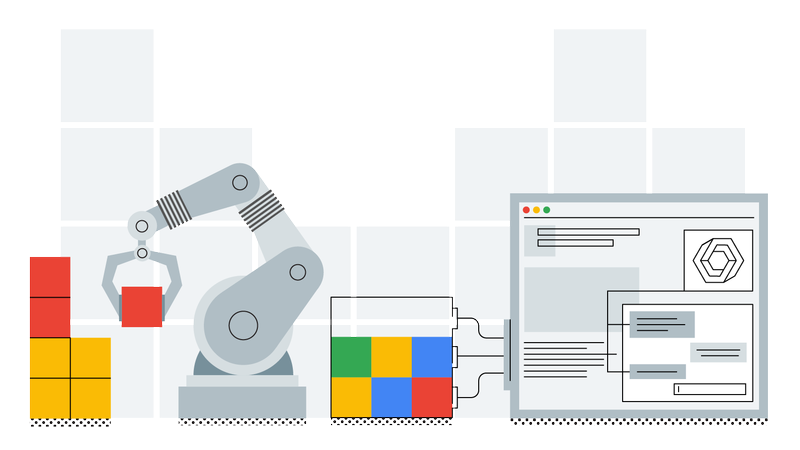
ML allows businesses to create real-time predictive applications that can accelerate cost savings and identify revenue opportunities. ML can, for instance, identify products and solutions that provide a better customer experience or better business value: think a recommendation feature that makes personalized suggestions as customers browse your website. Over time, the ML model will become increasingly accurate, as new information—every click, review, transaction, and interaction—is fed back to it. The result? The right customer can find the right product at just the right time—not to mention that your business is operating more effectively.