How SAIF can accelerate secure AI experiments [infographic]
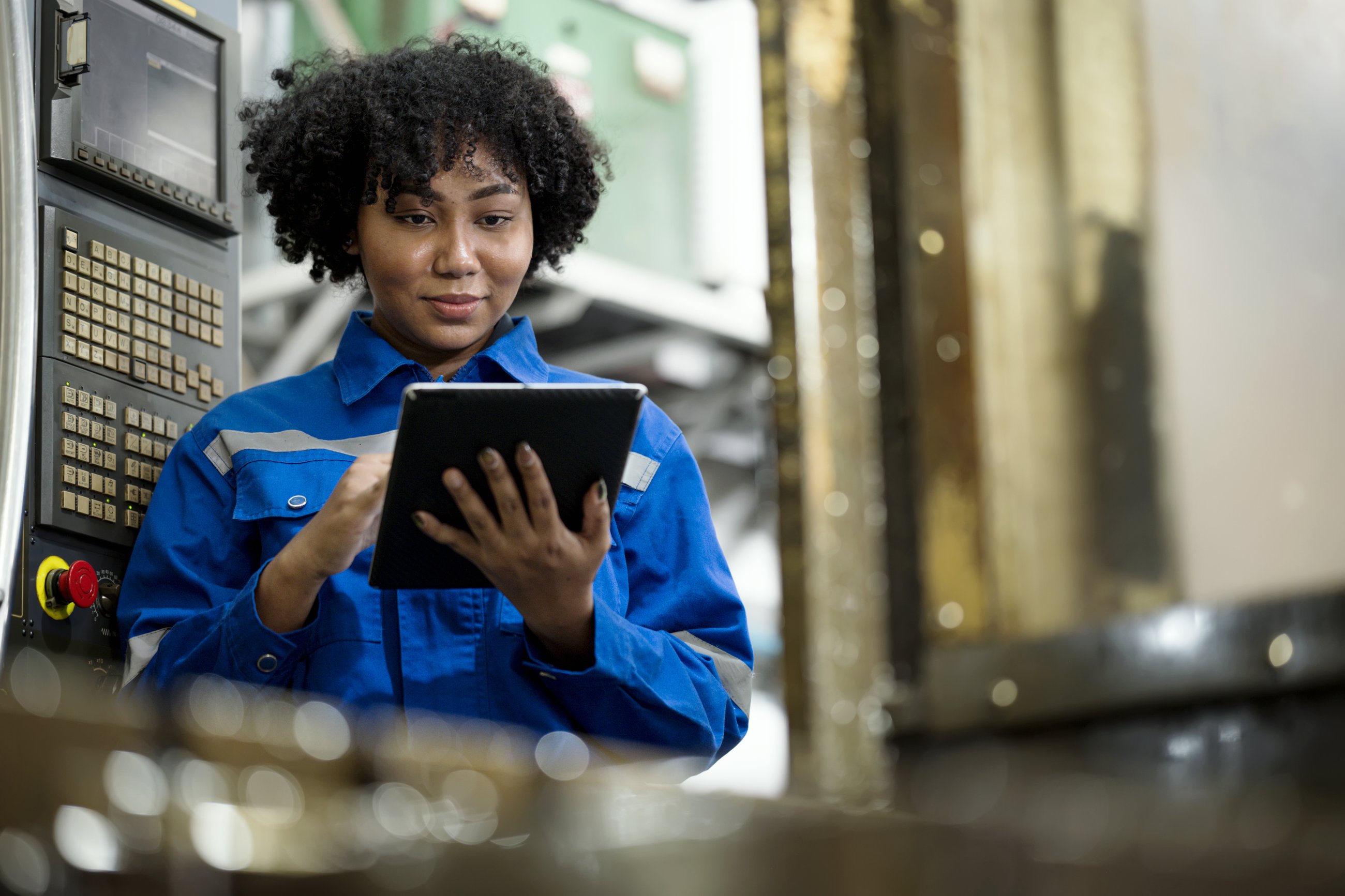
Marina Kaganovich
Executive Trust Lead, Office of the CISO, Google Cloud
Anton Chuvakin
Security Advisor, Office of the CISO
Hear monthly from our Cloud CISO in your inbox
Get the latest on security from Cloud CISO Phil Venables.
SubscribeEverybody’s favorite answer to “how do I learn AI to help my business” is “go experiment with AI.” Cliched though it might be, the best way to learn AI is to actually use AI models to identify and test the options that might lead to benefits for your organization. In doing so, it’s important to ensure you’re not creating AI experiments that increase your organization’s risk.
At Google Cloud’s Office of the CISO, we encourage security and business leaders to learn AI through pilot experiments that are safe, secure, and compliant. Embarking on AI experimentation is more than following a trend: It's about empowering your team, and fostering a culture of learning, experimentation, and discovery in your organization. How else will you discover that using AI in your enterprise will be beneficial until you have a successful pilot that demonstrates its usefulness?
Think of AI adoption like a novice swimmer approaching a deep pool. Jumping straight into the deep end can be overwhelming and risky, much like trying to overhaul your entire business with AI. Instead, start in the shallows and identify a specific use case. Get comfortable with the water — set measurable goals, build your skills and confidence as you learn the strokes, and then gradually swim deeper.
Getting started with pilots can seem daunting because of the inherent complexity, cost, and resourcing associated with AI technology, so organizations need more than just a "try it and see" approach. It’s also important to run pilots that have been designed to manage the risks associated with rapid AI adoption
Embrace SAIF to accelerate AI experiments
Google has an imperative to build AI responsibly, and to empower others to do the same. That’s why we introduced the Secure AI Framework (SAIF), a conceptual framework to secure AI systems.
Projects need to embrace AI securely and responsibly, safeguard privacy, and uphold compliance. Certain building blocks and development practices need to be in place to enable staff to experiment SAIF-ly (forgive us) and responsibly. (For additional best practices, you can check out our guidance for developing AI governance and acceptable use policies.)
With a well-defined problem in hand, set measurable goals. What exactly do you want to achieve with AI?
Our AI Principles describe our commitment to developing technology responsibly and in a manner that is built for safety, enables accountability, and upholds high standards of scientific excellence. SAIF creates a standardized and holistic approach to integrating security and privacy measures into machine learning-powered applications. SAIF can help ensure that ML-powered applications are developed in a responsible manner, taking into account the evolving threat landscape and user expectations.
How do you get started?
To dip your toes into the transformative world of AI, identify specific use cases where AI could make a tangible difference — perhaps automating a repetitive task, personalizing customer interactions, or optimizing inventory management.
A successful pilot isn't just about proving the technology: It's about demonstrating that you can deploy it safely and responsibly.
With a well-defined problem in hand, set measurable goals. What exactly do you want to achieve with AI? Whether it's boosting efficiency, slashing error rates, or enhancing the customer journey, having concrete targets will help you gauge the success of your AI experiment and make informed decisions moving forward.
What are the risks?
With a few use cases in mind, ask yourself key threshold questions. In the rush to harness AI's potential, it's easy to overlook the interconnected nature of data within your organization. Even in a seemingly isolated test environment, the data you feed your AI models can raise significant questions, such as, “Are you using synthetic data, real customer data, or sensitive corporate information?” Each answer carries implications for privacy, security, and compliance.
Likewise, consider who has access to the training environment. Could this access inadvertently expose them to data they shouldn't see? Robust data governance practices, including clear protocols for data provenance and access controls, become paramount as the obligation to secure your data is in no way diminished even if it’s only used for experimentation. This is one way that SAIF can help organizations develop their AI tools.
First, keep your scope narrow. Don't try to boil the ocean. Focus on a few specific use cases where generative AI can add tangible value. Think about tasks that are repetitive, time-consuming, or prone to human error, such as automating customer service responses, drafting marketing copy, or summarizing lengthy documents. Start small and focused, prove the concept, and then expand.
Next, assemble a cross-functional team. Bring in your security folks, your data scientists, your legal team, and your subject matter experts. This isn't just an IT project; it's a business transformation initiative. Get everyone on board, establish clear roles and responsibilities, and ensure that your pilot aligns with your organization's broader goals.
Then, begin with the data. Gen AI models thrive on high-quality, relevant data. Identify your data sources focusing on public and low-risk data, clean and preprocess the data, and consider any privacy or ethical concerns. For a pilot, avoid PII, corporate secrets and such.
Last, add security on day one. Gen AI introduces new risks, from data breaches to model biases. Implement robust security measures from the get-go, monitor for anomalies, and have a plan for incident response. Remember, a successful pilot isn't just about proving the technology: It's about demonstrating that you can deploy it safely and responsibly, enabled by enterprise-grade AI privacy, security, and compliance capabilities.
A well-executed pilot can pave the way for widespread adoption. It can help you identify potential roadblocks, fine-tune your approach, and build a solid foundation for future gen AI initiatives. Consider using our enterprise gen AI and ML blueprint guide, and accompanying Terraform repository, when designing a business-ready environment that adheres to security best practices. Before rolling it out more broadly, be sure to red team your design.
By incorporating these comprehensive data governance practices, your organization can harness the full potential of AI while upholding the high standards of security and data protection. For more information, you can contact us at the Office of the CISO.
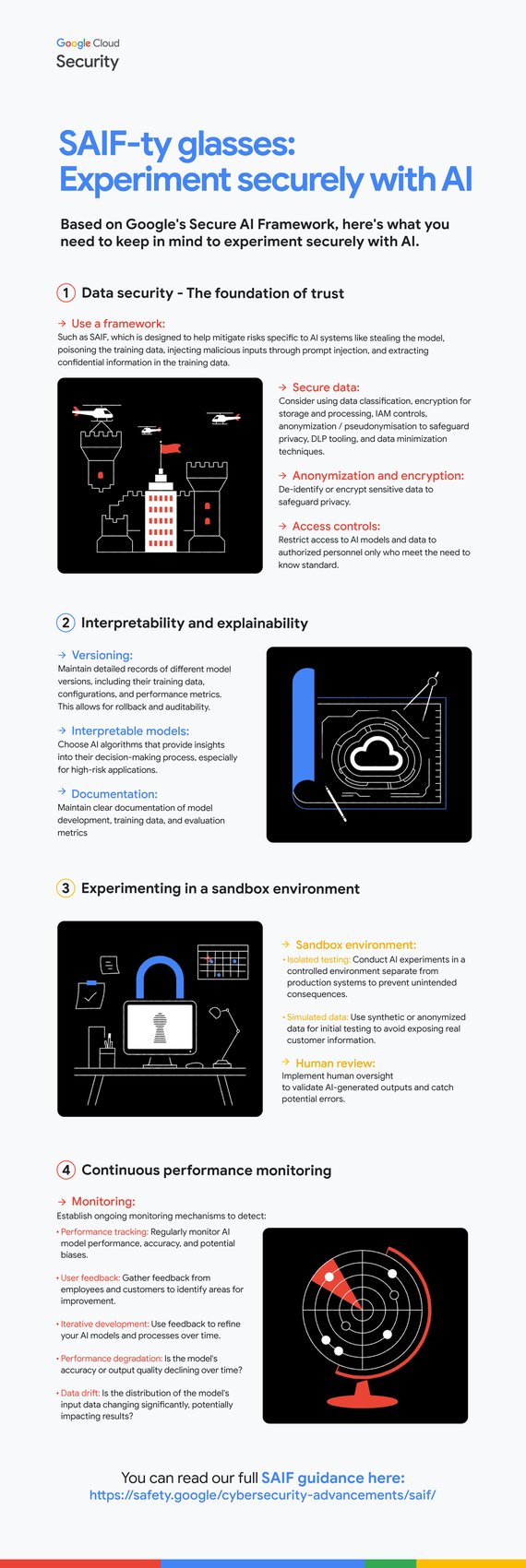
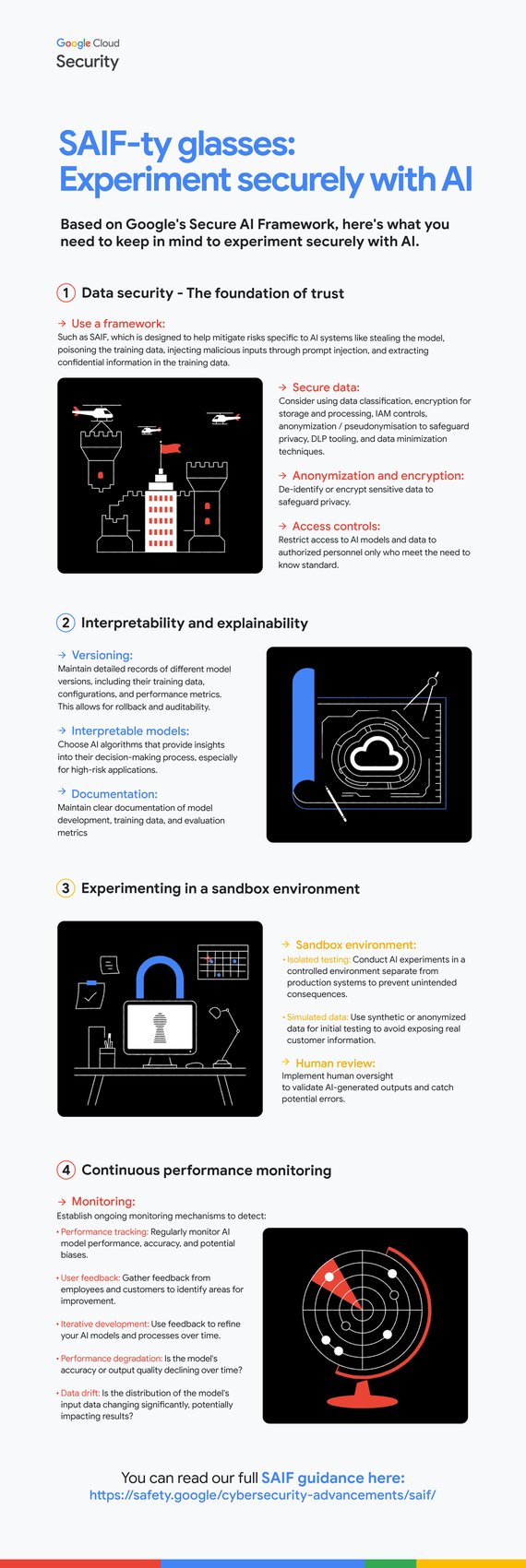