Gen AI governance: 10 tips to level up your AI program
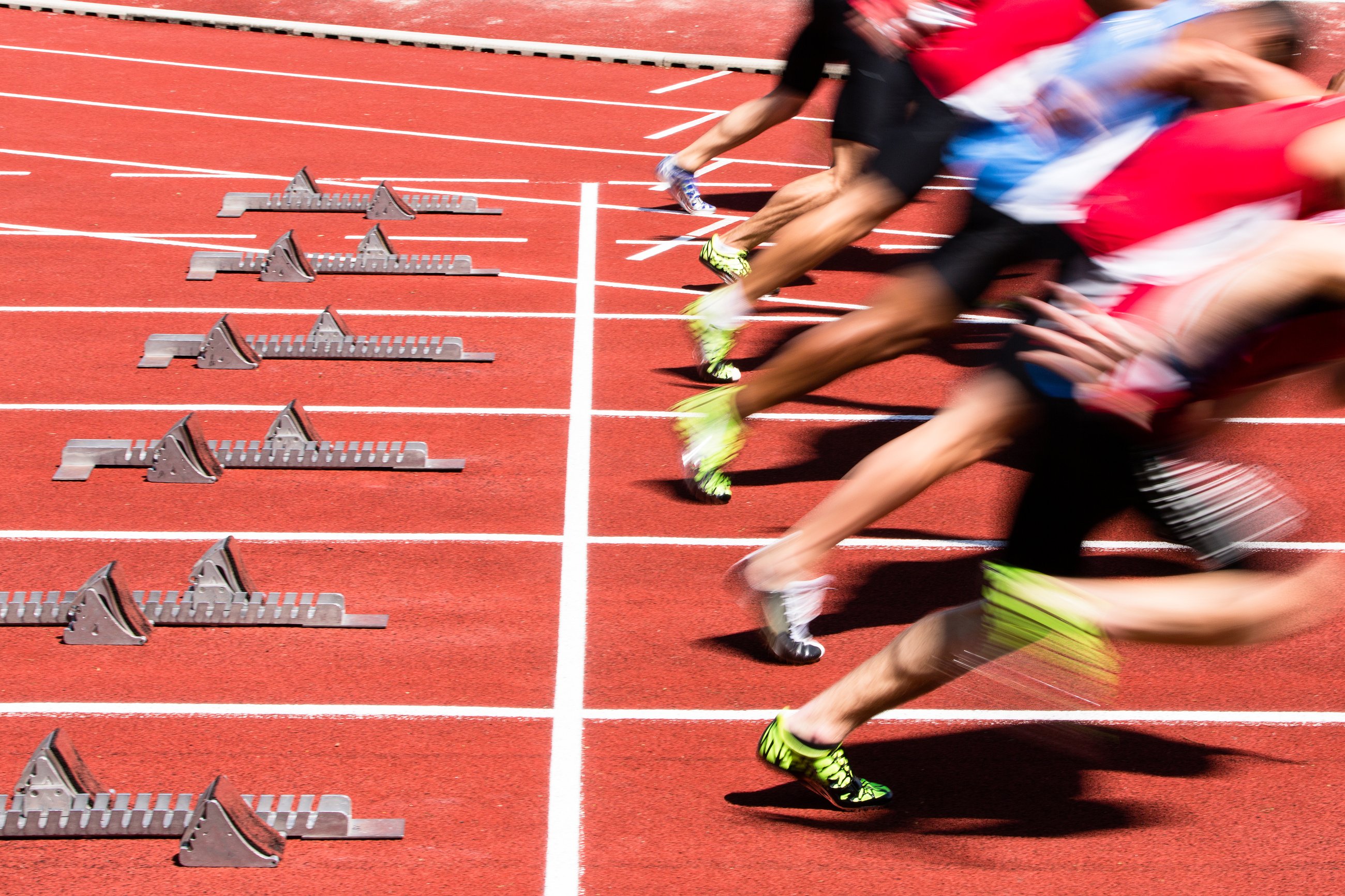
Anton Chuvakin
Security Advisor, Office of the CISO
Marina Kaganovich
Executive Trust Lead, Office of the CISO, Google Cloud
Take the gen AI governance reins with this must-do guide
Hear monthly from our Cloud CISO in your inbox
Get the latest on security from Cloud CISO Phil Venables.
SubscribeStop us if you’ve heard this one before: A large multinational company, or even just an organization operating under a complex set of regulations, wants to add AI to their tech toolkit. They start with an AI pilot to determine viability, or perhaps they don’t even know how to get started. Either way, the bespoke nature of the AI review process and the intersection of AI and regulatory uncertainty makes progress sluggish and inefficient. Eventually, they decide to table using AI until some point in the future.
The problem is that the future is now, the competition knows this, and it’s important that organizations lean into AI to help achieve their goals — not away from it.
Phil Moyer, Google Cloud’s global vice-president for AI and Business Solutions, said in a column on striking the right balance between data governance and technological development that the issue is “crucial” and “urgent.”
“Today’s leaders are eager to adopt generative AI technologies and tools. Yet the next question after what to do with it remains, ‘How do you ensure risk management and governance with your AI models,’” he wrote. “In particular, using generative AI in a business setting can pose various risks around accuracy, privacy and security, regulatory compliance, and intellectual property infringement.”
As with other forms of digital innovation, creating a programmatic, repeatable structure can help achieve a consistent approach to evaluating AI use cases. It also supports comprehensive oversight that considers the various facets which are relevant to a secure and compliant implementation.
Using AI can also help mitigate many challenges, including worker toil, threat overload, and the talent gap. Aligning on the objectives, roles and responsibilities, and appropriate escalation paths within the organization, are critical to maintaining ongoing viability and success. As a first step, define your governance structure and clearly articulate its role within the context of the overall organization’s AI innovation strategy.
To help organizations navigate these challenges, we’ve outlined 10 best practices to streamline and operationalize AI implementation at scale.
- Identify key stakeholders from various disciplines to provide their subject matter expertise to evaluating AI initiatives. Precise titles vary across organizations, but typically include representatives from the following functions: IT Infrastructure, Information Security, Application Security, Risk, Compliance, Privacy, Legal, Data Science, Data Governance, and Third Party Risk Management teams.
- Define your organization’s guiding AI principles to articulate foundational requirements and expectations, as well as use cases that are explicitly out of scope. The guiding principles should be flexible and not overly prescriptive, capturing commitments from which the organization won’t deviate; for instance, a focus on safeguarding customer privacy, or ensuring a human is involved in reviewing AI-generated decision making for certain use cases. As an example, see Google’s Responsible AI Principles.
- Use a framework such as Google’s Secure AI Framework (SAIF) for a secure and consistent approach to AI implementations. However, beware of security framework traps — a framework is just a tool, and its use shouldn’t be confused with having achieved your objective. Rather, a framework like SAIF is a helpful way to approach AI implementation to ensure its multiple facets are comprehensively considered. For instance, tying in the AI tool inventory into technology change management processes to ensure AI deployments are kept up to date, or as another example, that AI deployments are included in threat management exercises, taking into account the novel types of attacks to which AI tools are susceptible.
- Document and implement relevant policies and procedures for AI design, development, deployment and operations. Ownership of these resources typically varies by team, and effective AI governance oversight requires a concerted effort to maintain accuracy, completeness and alignment.
- Articulate the AI use cases being considered relative to the organization’s strategy and risk appetite. Rank them in order of business priority and the degree of risk they may pose, tailoring the security and data protection controls accordingly.
- Plug into your organization’s data governance program because AI models typically require high-quality data which should be appropriately sourced, cleansed, and normalized. Carefully selecting your data set and tuning the AI model for your specific needs can also help minimize the potential for hallucinations and risk of prompt injections.
- Partner closely with your Compliance, Risk, and Legal stakeholders. AI regulation is a rapidly evolving space, and staying closely aligned with regulatory requirements is imperative to maintain the organization’s compliance posture and also inform the overall governance process.
- Establish points of escalation so that when questions arise, there’s a clear path to getting them answered. While some organizations may want to send their internal queries to Legal, Compliance, or Information Security, others might prefer to empower a designated committee to make decisions.
- Implement mechanisms for providing visibility on the status of each AI initiative both to internal stakeholders (senior management, board of directors) and externally, such as to relevant regulatory bodies.
- Institute a dedicated AI training program to support organization-wide understanding of key concepts and potential challenges associated with AI. A modular, focused approach with tailored content for staff depending on their role may also be helpful in support of skills development and a broad understanding of risks to avoid, such as the use of shadow AI.
What’s next for your gen AI governance journey?
Identify an AI champion to get the conversation started. This executive should have visibility into the organization’s AI strategy and a collaborative approach to bringing stakeholders from various disciplines together while clearly communicating the business cases for AI and also how the organization as a whole should rise to meet it. A helpful resource to consider as you embark on this journey is Google Cloud Generative AI Skills Boost for practical, hands-on learning.
For additional information on securing AI, review our papers “Securing AI: Similar or Different” and “Google Cloud’s Approach to Trust in AI.”