Foundations for models: Why accelerating AI has to start with your infrastructure
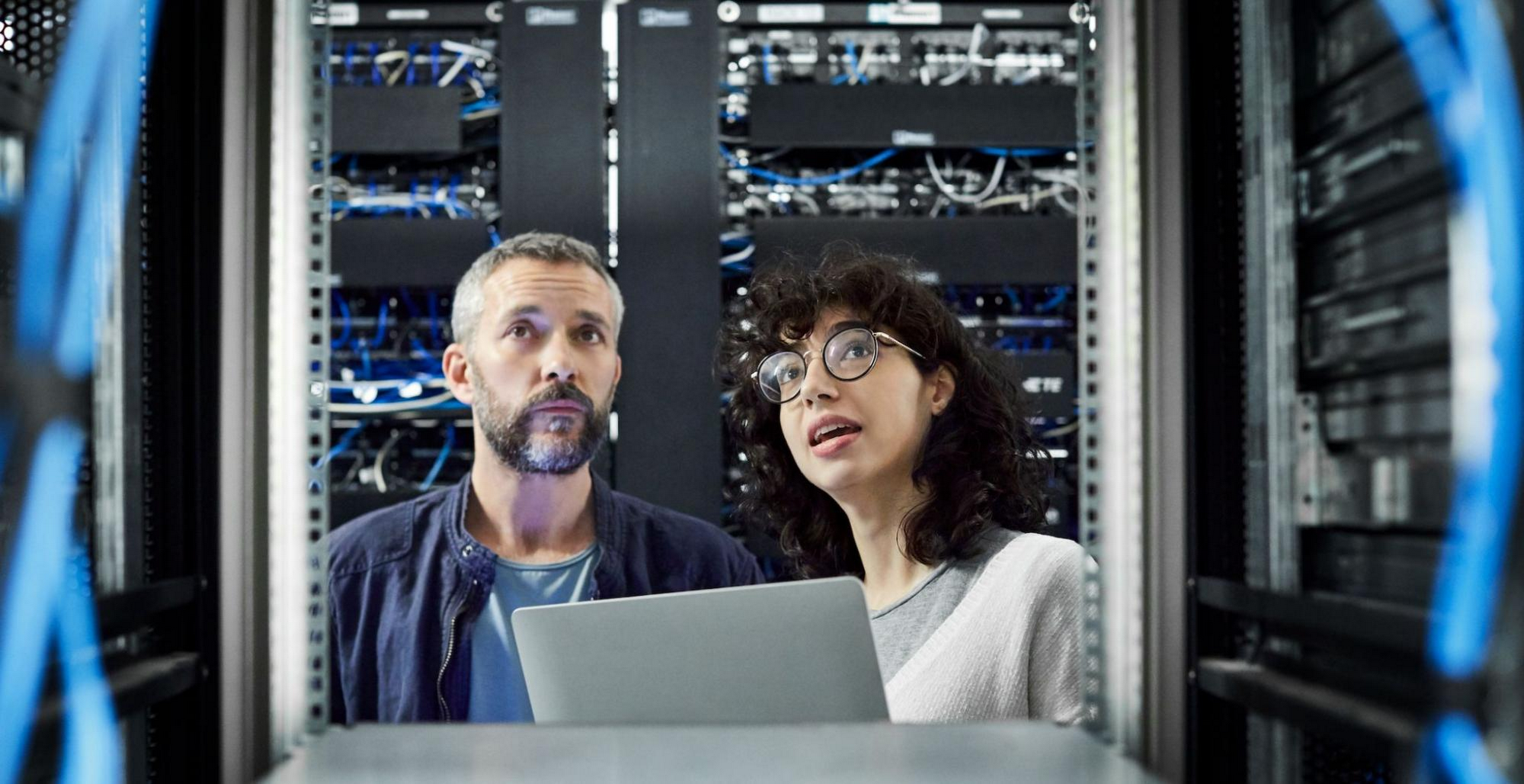
George Elissaios
Senior Director for Specialized Compute, Google Cloud
Mikhail Chrestkha
Outbound Product Manager
You've got to align on AI objectives, hardware usage, and performance if you want to marry the right infrastructure to your projects.
It’s a challenging time to run a business. Today, your organization is likely juggling many difficult, often competing decisions: debating austerity measures to do more with less, streamlining projects, improving employee productivity through AI and machine learning.
Part of what’s driving all this action is the incredible amount of data you can now capture, from web and social interactions, mobile devices, cameras and IoT sensors — everything feels like an input these days. Each data point can be powerful, too, helping inform insights, automating workflows, and generating personalized customer experiences to improve brand affinity.
Yet for every action, there is an equal and opposite reaction, and when it comes to data, the vast amounts of it can feel as overwhelming as they are empowering. Capturing data and capitalizing on it are two different things. All the models in the world won't make a difference if your infrastructure can't keep up.
As datasets grow larger, the opportunities and challenges grow in tandem. One of the biggest hurdles we’ve seen around the deployment of generative AI models, for example, is the magnitude of computing power required to process everything. The same is true for more conventional forms of data gathering, analytics and business intelligence. Simply put, innovating, optimizing, and deploying AI and ML projects requires more compute resources.
That’s why many organizations are increasingly discovering there has to be more to their infrastructure to meet their AI. To prosper in the age of AI will not only require new and different forms of IT infrastructure — do you know your GPUs from TPUs? — but also a new way for organizations to think about
When investing in infrastructure is investing in AI
When working with large-model AI analysis, speed and performance can quickly become unique differentiators for organizations looking to take advantage of their big but unstructured data. Failing to invest in new hardware or tune existing AI infrastructure means training models can take months, quarters, or even years to fully mature.
There are multiple ways to access AI infrastructure, from building your own, to working with cloud providers such as Google Cloud, or even using APIs to connect your data with large external models. Regardless of where you access your AI infrastructure, once you’ve built your models, embedding them into your business decision-making process can require extraordinary amounts of compute power to continuously analyze and generate consumable content.
Deploying and perpetually optimizing large models can strain even the most dedicated team of engineers, yet it’s necessary when analyzing petabytes of data and generating business insights or unique content. Data and AI teams composed of highly skilled and compensated engineers can wait for hours or days for models to provide their results. Such hardware-imposed procrastination can grate on teams who need to test, iterate, and retest their results. It’s counter to the agility that is one of the superpowers of AI and machine learning — rapid experimentation and personalization are essential to outstanding consumer experiences.
Deploying and perpetually optimizing large models can strain even the most dedicated team of engineers. Don’t waste their time with the wrong infrastructure.
For example, Vodafone has been deploying AI-optimized infrastructure to power their experiences, wherein technical teams have slashed the time from creation to deployment of AI models by up to 80%. In the especially fickle market for mobile and communications services, these time savings can be invaluable to offering a superior experience.
Optimized infrastructure can be a deciding factor when resource planning for AI projects. Recent studies are showing significant performance differences, as much as 2x, between standard and AI-optimized hardware. This demonstrates why infrastructure decisions can be so instrumental to a company’s broader innovation agenda.
To help set your AI investment up for success, it’s essential to take a step back and approach it with three key points in mind:
Clearly define your AI objectives
Understand your consumption patterns
Understand your performance requirements
From there, you can decide if you want to build your own AI system stack or work with a cloud provider such as Google Cloud, which can deploy purpose-built infrastructure for your project.
Clearly define your AI objectives
Building and deploying your AI project requires understanding precisely what it will do.
By having an end-to-end understanding of your project, it’s easier to mitigate potential provisioning or scaling issues that can be time consuming and costly later on. Seeking alignment will also benefit cross-team collaboration, helping ensure alignment between stakeholders and the ultimate goal of the work. You should also be using this while improving team collaboration by ensuring stakeholders and goals are aligned.
Here are some objectives the organization might consider to help define AI projects:
Increase productivity gains of AI practitioners to experiment and deploy faster
Improve the end-user experience of existing business processes or consumer products
Accelerate time to market to launch net new AI-powered products and capabilities
Once these topics have been considered, the team will have a better sense of their infrastructure needs, and if it’s up to the tasks at hand.
Understand your consumption patterns
When determining your infrastructure needs, failing to understand your consumption patterns can mean the difference between a successful deployment and a waste of money. Consider that your current in-house team may need additional staffing to handle the influx of datasets or that engineers specializing in optimizing AI systems may be a necessary investment.
By calculating your needs ahead of time, you can avoid the pitfalls of choosing infrastructure that fails to meet your requirements or leaves you with an inflexible model that is difficult to customize, provides little value, and still doesn’t solve your problems.
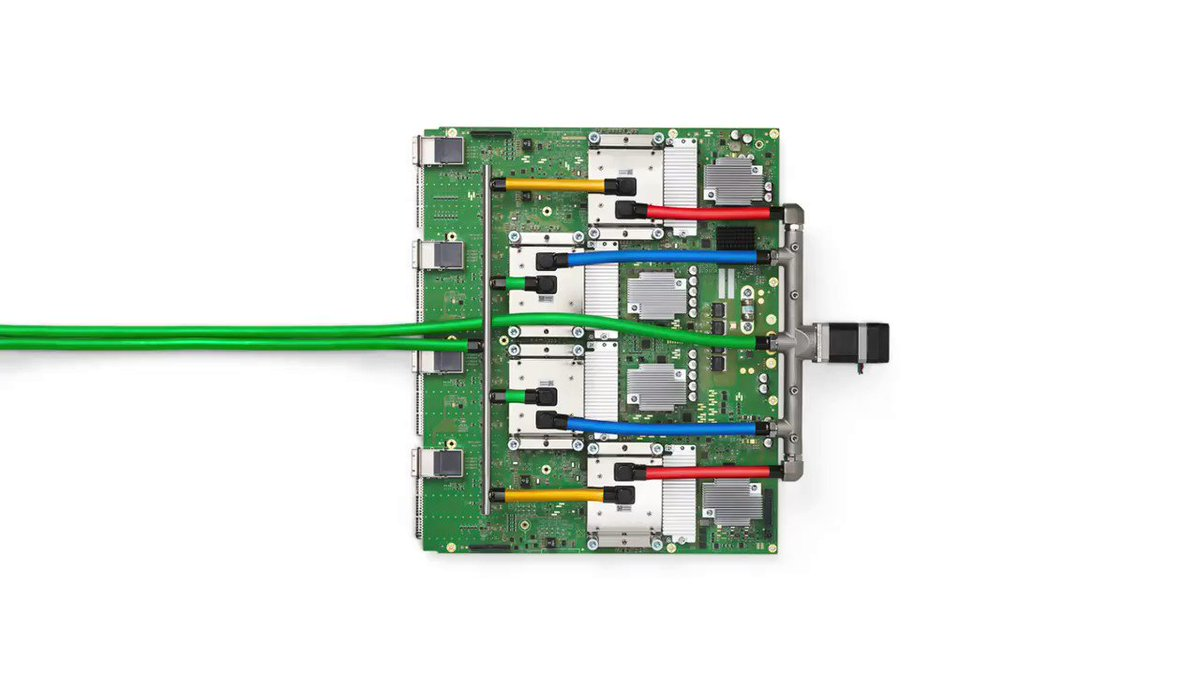
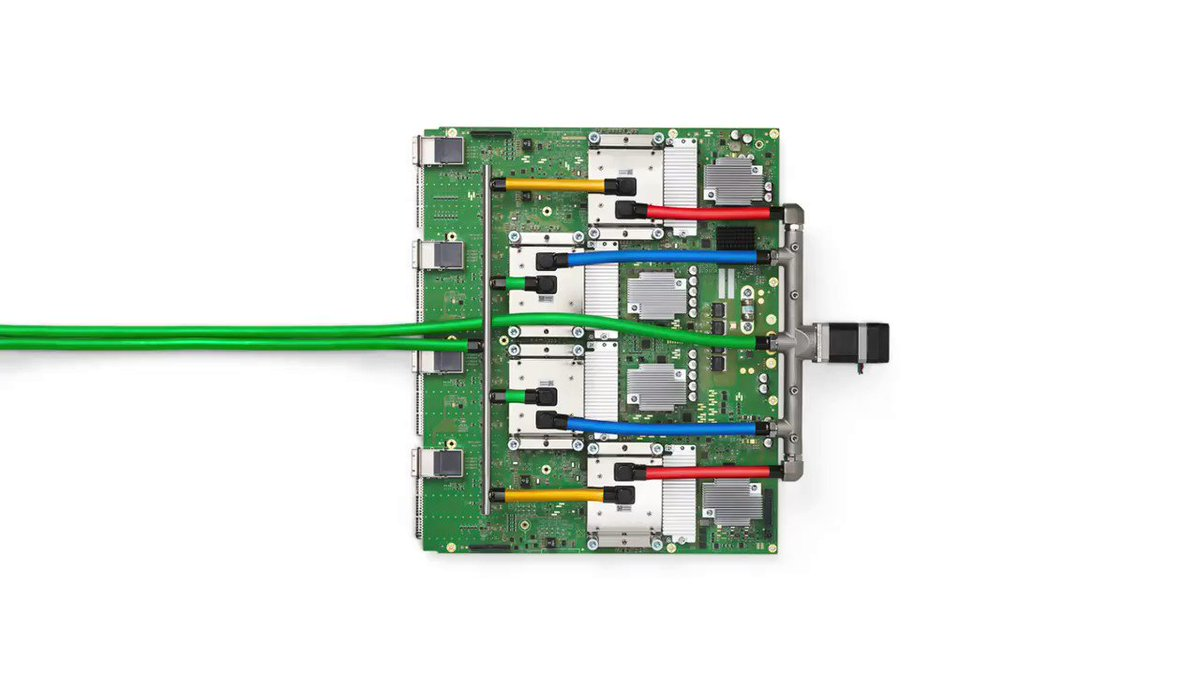
Teams should clearly understand their target users — it it AI researchers,. ML engineers, data scientists, software developers, or some mix thereof? You then need to choose the right layer of the AI tech stack to build and maintain to suit these users, whether their greatest needs are model size, speed, technicality, and so on.
This might mean building your own set of applications, through Kubernetes, for example, utilizing specialized hardware you control. Or you could adopt a managed platform with less customizability but also less work, with a managed machine-learning platform like Vertex AI. And even simpler would be tapping into the latest AI models through a set of APIs that provide the broadest access and controls.
Understand your performance requirements
No two projects are the same, whether that’s between competitors or even within the same organization. Every enterprise wants to train their model to their brand voice or knowledge base, and be able to personalize suggestions based on the latest customer data. Because of this, understanding your performance requirements is crucial to ensure not just the deployment of your project but its continuing success.
To fully account for your costs, you must determine what hardware and software is appropriate for your project, as training, tuning, iterating, and deploying large models can differ based on the model and application. Certain processors, like standard CPUs, more advanced graphical processing units (GPUs) and Google’s AI-specialized Tensor Processing Units (TPU) all have tradeoffs. Deciding which you need can impact the ongoing costs, runtime, and performance abilities of your AI project.
Finally, identifying the software that is optimized for your underlying hardware is critical to ensure you are getting the most from your infrastructure investments. A comprehensively optimized stack maximizes the output of your models while conserving compute and performance.
All in on AI
Today, organizations across all industries are exploring how to integrate AI, including newer generative AI capabilities, into their business functions. However, the opportunities for businesses are only achievable if the AI systems are correctly trained, optimized, and configured for each project.
Failing to take a step back, examine your project from end-to-end, and account for all costs, can lead even the simplest of projects into budget overruns. AI-optimized infrastructure can be the difference between a successful model that allows your company to take full advantage of its data and a sinking hole of tech debt.