The Prompt: When to keep generative AI adoption simple
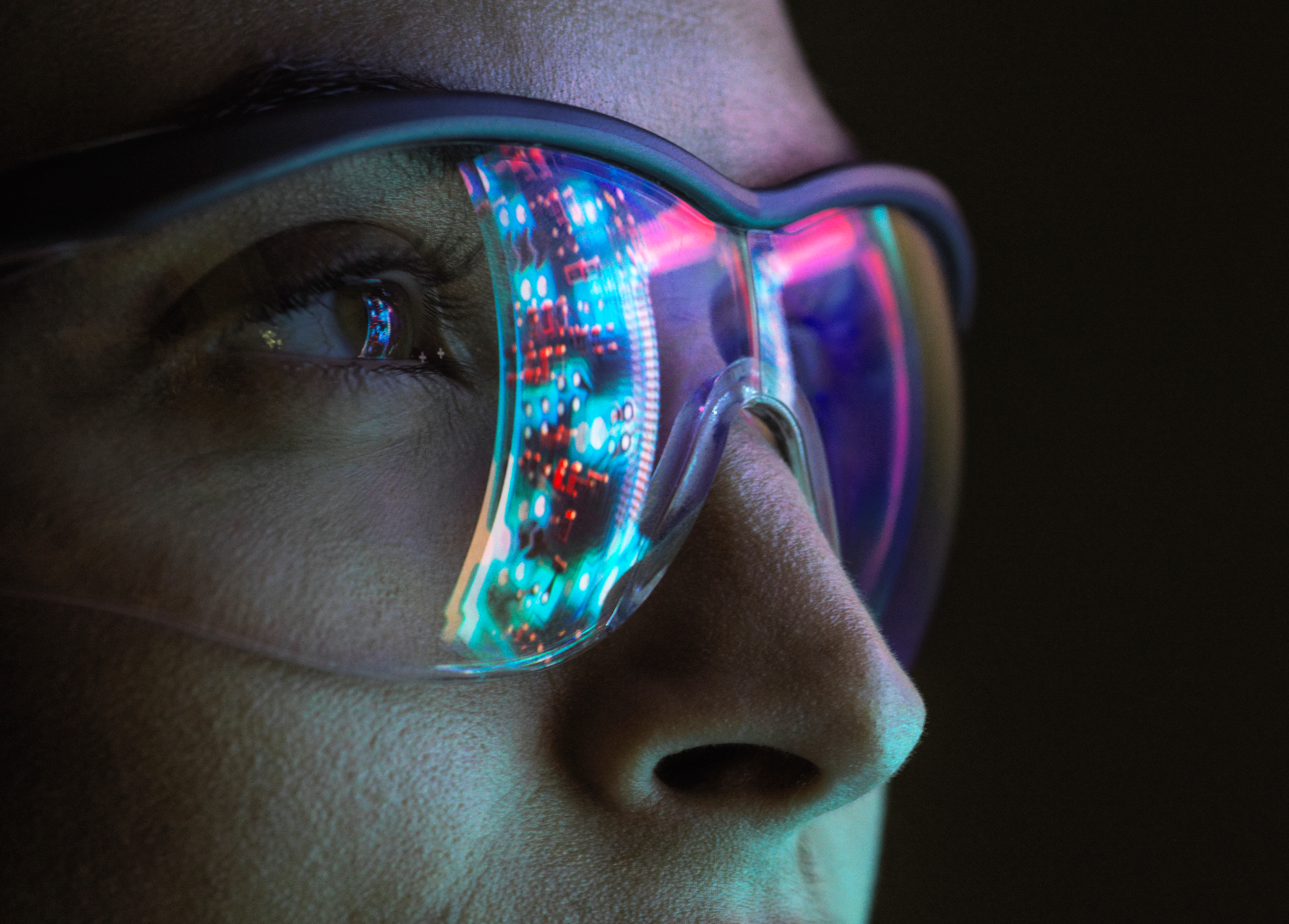
Carrie Tharp
Vice President, Strategic Industries, Google Cloud
Philip Moyer
Global VP, AI & Business Solutions at Google Cloud
Business leaders are buzzing about generative AI. To help you keep up with this fast-moving, transformative topic, each week in “The Prompt,” we’ll bring you observations from our work with customers and partners, as well as the newest AI happenings at Google. In this edition, Philip Moyer, Global VP, AI & Business Solutions at Google Cloud, and Carrie Tharp, VP, Strategic Industries at Google Cloud, discuss why simple use cases offer impactful onramps to generative AI.
From Google I/O last month to our Executive Forum last week, and across a variety of events in between, we’ve been in the fortunate position to connect with customers non-stop as our rollout of generative AI products has continued to ramp up.
Across many conversations, there’s been a perceptible shift over the last month in executives’ attitudes. Earlier in the year, most people were still amazed by the technology’s potential and the pace at which it’s advancing. Now, most conversations focus on identifying use cases that can be deployed quickly to production.
It’s notable that outside organizations that already have a rich history with machine learning and data science, most executives are focused on straightforward projects such as the following:
Building chatbots and digital experiences for self-serve customer service and product discovery
Generating marketing content
Facilitating better management of internal data, from next-gen enterprise search to pipelines for generating and reviewing common documents, such as RFIs and RFPs
Creating personalized content for account-based marketing and other B2B use cases
This isn’t a comprehensive list, but as a directional signal, it makes clear that many leaders are prioritizing high-value, lower-risk solutions that can be quickly built and deployed to production, with less (if any) thought dedicated to the most hyped and futurist moonshots.
In our view, these strategies represent a good approach.
Moonshots are important, to be clear. More people than ever are reaching for the stars when it comes to AI applications. Every large organization should have a few big bets on its roadmap. For some startups, a moonshot is the whole point.
That said, anyone who’s followed AI trends on social media has surely seen the barrage of influencers who claim “generative AI will 10x productivity—don’t get left behind!” This kind of hyperbole makes it difficult for organizations to set realistic goals—and that’s a problem, since any big bets need to be balanced by practical, achievable use cases that might not reinvent the wheel but still move the needle.
For example, in the near term, for common generative AI uses like code completion or text-to-code, most organizations probably want to target efficiency gains closer to 10 percent than to 10x. Bigger gains may be possible, given this technology is still in its infancy—but as with every technology disruption, organizations need space to walk before attempting to run. Getting started and learning is helpful—measuring one’s goals against the whirlwind of hype is not.
To our delight, the straightforward focuses by our customers mirror the advice we offered a few months ago in the second installment in this series—i.e., that organizations should focus on ways to help customers (like self-service chatbots); apps that make internal data more powerful (like apps that combine enterprise search technologies with generative AI): and use cases that reduce operational drudgery (like generating multiple iterations of the same marketing message or accelerating the creation and processing of RFPs).
To this advice, we’d add that it can be productive to start with internal use cases rather than customer-facing ones. For example, if an organization becomes comfortable managing generative foundation models for enterprise search scenarios, it also builds up the skills and confidence required to harness models for public apps and websites.
As the spectrum of requirements and maturity levels may suggest, organizations need a range of entry points for generative AI adoption, which informs how we’ve built out our platform. Products like Google Workspace inject AI across common workflows. Generative AI App Builder abstracts the complexity of building common generative apps like search engines and chatbots, so organizations can get moving quickly. Generative AI support on Vertex AI offers more advanced tools for accessing, customizing, and managing foundation models for production in custom generative apps. And a range of infrastructure options and partners help further tailor our products for different needs. As we discussed last week, these capabilities outline a framework that gives organizations ways to not only pursue initial projects, but also grow and maintain optionality over time.
Targeting simple use cases is a great way to get started—because even simple use cases can produce obvious and measurable value, and they’ll pave the way for organizations to pursue more innovative and amazing applications in months and years to come. To see some of our products in action, be sure to check out these new videos:
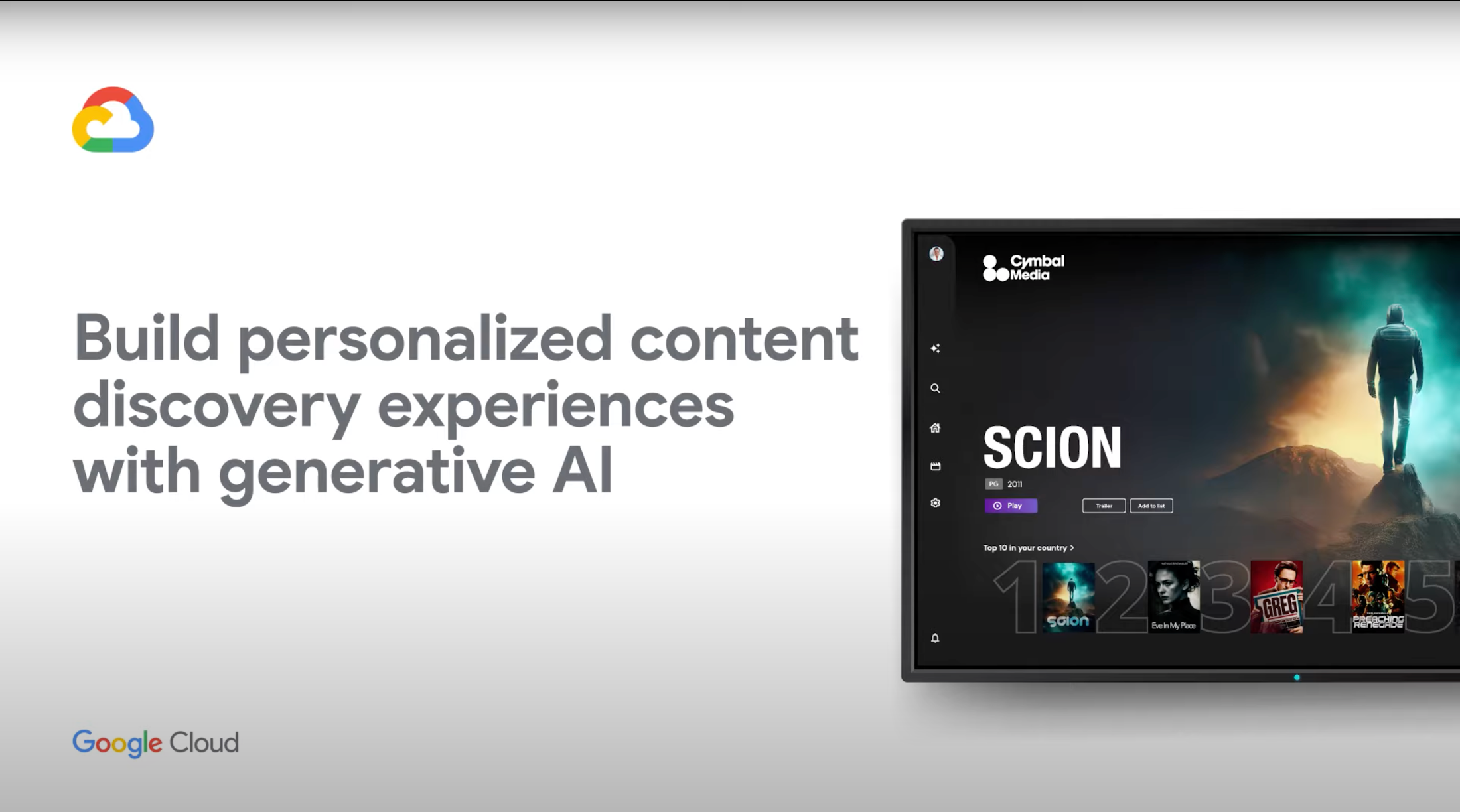
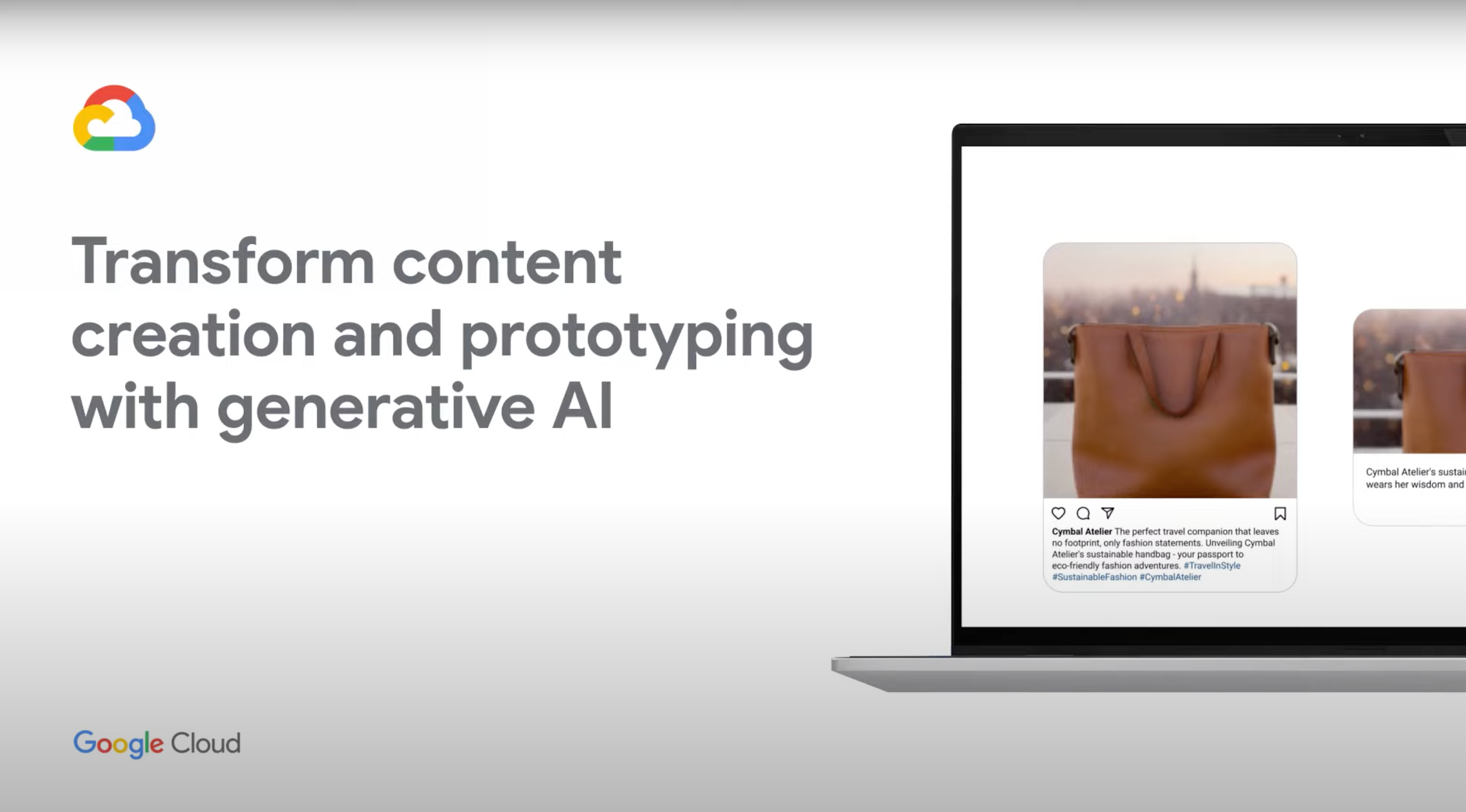
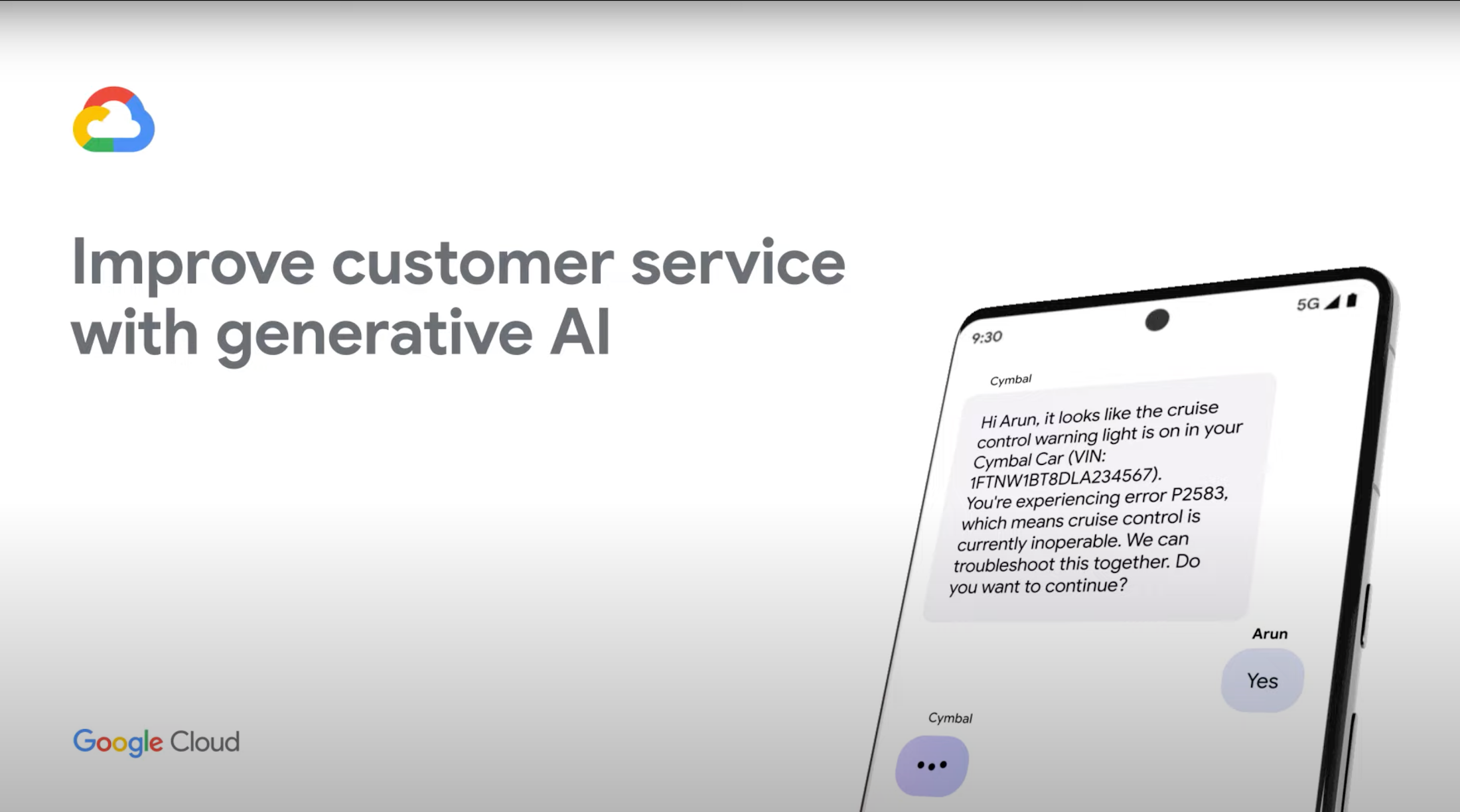