How generative AI can boost productivity (and why worker wellbeing is key)
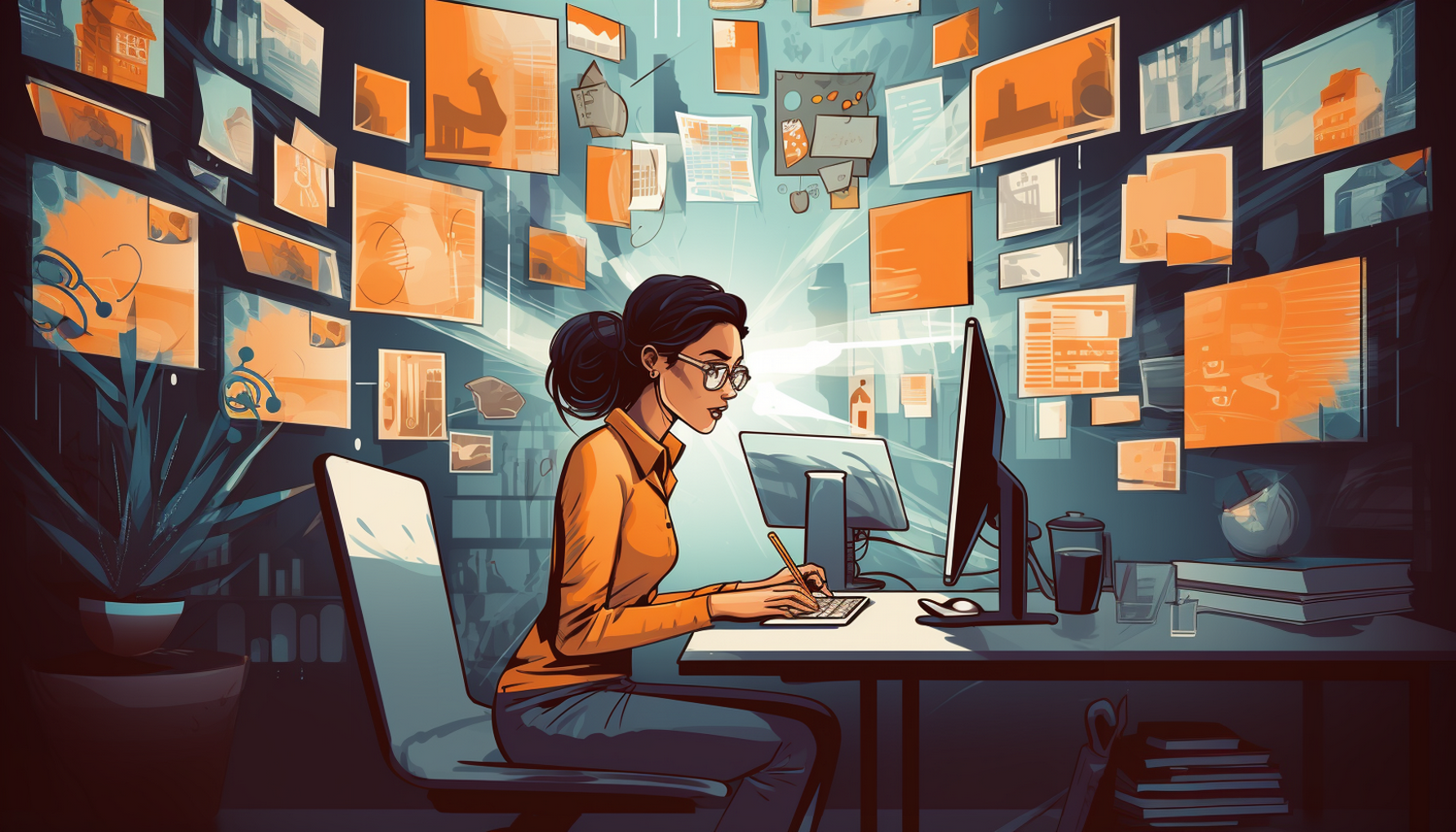
Derek DeBellis
DORA Research Lead
Always start with a people-centered approach when deploying gen AI — but don't overlook data or systems, either.
Lifehacks. Advice columns. Shortcuts. That scene in “The Bear” where the head chef times the steps between stations in the kitchen to under five seconds. In business and in life, we’re usually looking for ways to be a little more productive, a little happier, or more effective.
In the few short months since it has undergone widespread interest, generative AI has been held up as a massive boon for productivity, cutting time or increasing output by double- or triple-digits, sometimes even more. For example, one consultancy found that programming work that took 78 hours before gen AI could be done in just 56 hours with pre-2022 AI technology and 36 hours using more recent advancements. That’s a 114% efficiency boost in just a few years.
But just like with advice and shortcuts, it can be hard to know if you’re picking the right AI technology and using it smartly, securely, or to maximal effect. There are no doubt transformational opportunities to improve productivity for teams across the enterprise, including not only IT but also legal, marketing, sales, operations, M&A, and supply chains. In a few years, it seems unlikely there will be any part of the organization that’s not benefiting in some way from AI — the same way they do now with electronic communications and digital tools.
Before organizations can realize the technology’s full potential, though, there has to be a greater understanding of how to apply AI to achieve their goals.
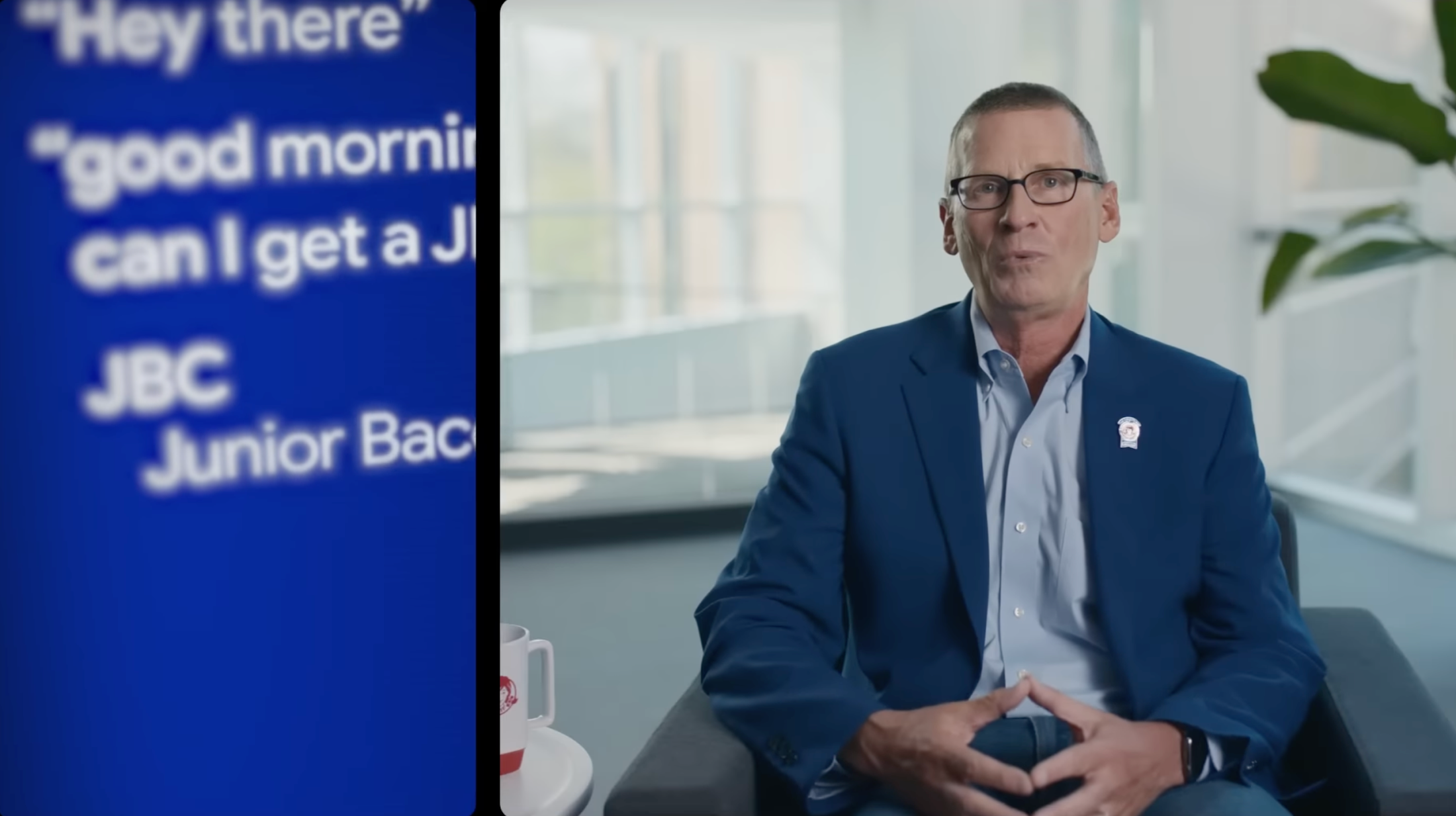
The truly fascinating value proposition of AI technology is not only increasing what workers can achieve in a given hour or day but providing greater value to your business and end users — without creating toil and burnout for employees.
As AI becomes more prevalent, it’s crucial to establish a framework for using this technology, whether developing your own principles for AI adoption or leveraging existing frameworks. By doing so, you can take full advantage of AI technologies and ensure they help drive enterprise productivity.
Generative AI enhances productivity
While we’re still at the dawn of widespread AI, the early productivity returns of generative AI are promising.
Research firm Nielsen Norman Group analyzed three case studies and found that generative AI improved business user productivity on average by 66% across the studies. The firm states that more complex tasks like programming realize more significant gains. It also found that less-skilled workers benefited most from generative AI.
The first case study was performed by Erik Brynjolfsson (author of The Second Machine Age and Machine, Platform, Crowd) and colleagues from Stanford University and MIT. The study looked at 5,000 customer support agents at a large enterprise software company and found that agents leveraging generative AI could handle 13.8% more customer inquiries per hour, with less experienced agents (the bottom 20%) benefiting the most at 35%. Furthermore, there was a boost to employee retention, with an 8.7% reduction in quitting in a given month — another indicator of the importance of employee well-being through AI.
The truly exciting value proposition from AI is that it will not only increase what workers can make in a given hour or day. AI is set to provide the end user and the business with greater value without creating toil and burnout for employees.
The second case study was performed by Shakked Noy and Whitney Zhang from MIT. The researchers studied 444 experienced business professionals from various fields using AI for document creation, such as marketers, human resource professionals, and grant writers. On average, AI-assisted documents took around 17 minutes to write, while unassisted documents took 27 minutes. When extrapolated to an average 8-hour day, workers could potentially write 59% more documents using generative AI. The quality improved, too, with AI-assisted documents averaging a score of 4.5 versus 3.8 without AI.
In the last study, researchers asked 70 programmers to implement an HTTP server in Javascript. Half used a generative AI tool, while the other half completed the task without AI assistance. Those using AI completed the job in 2.7 hours, compared to 1.2 hours with the help of AI tools.
“Now we have something to compare against!” Jakob Nielsen, a principal and co-founder of the Nielsen Norman Group, wrote. “The 66% productivity gains from AI equate to 47 years of natural productivity gains in the United States. And AI corresponds to 88 years of growth in the European Union, which is a third more time than the 66 years that have passed since the formation of the European Community (the precursor to the EU) in 1957.”
Seeing all the exciting possibilities, how can organizations and enterprises use generative AI to improve their productivity? It helps to have a guiding framework with robust measurement and a people-centered approach.
A framework for using AI to boost productivity
AI requires a lot of thinking about its short- and long-term implications and possible risks because it’s almost inevitable that it will continue to impact our community, environment, economy, the way we work, and our roles within the workplace. It seems bound to change how we relate to each other and the world around us.
With all that in mind, the resources required to sustain an AI-powered ecosystem are likely very different from what we have now. The question isn’t so much if AI will have a dramatic impact but in what manner.
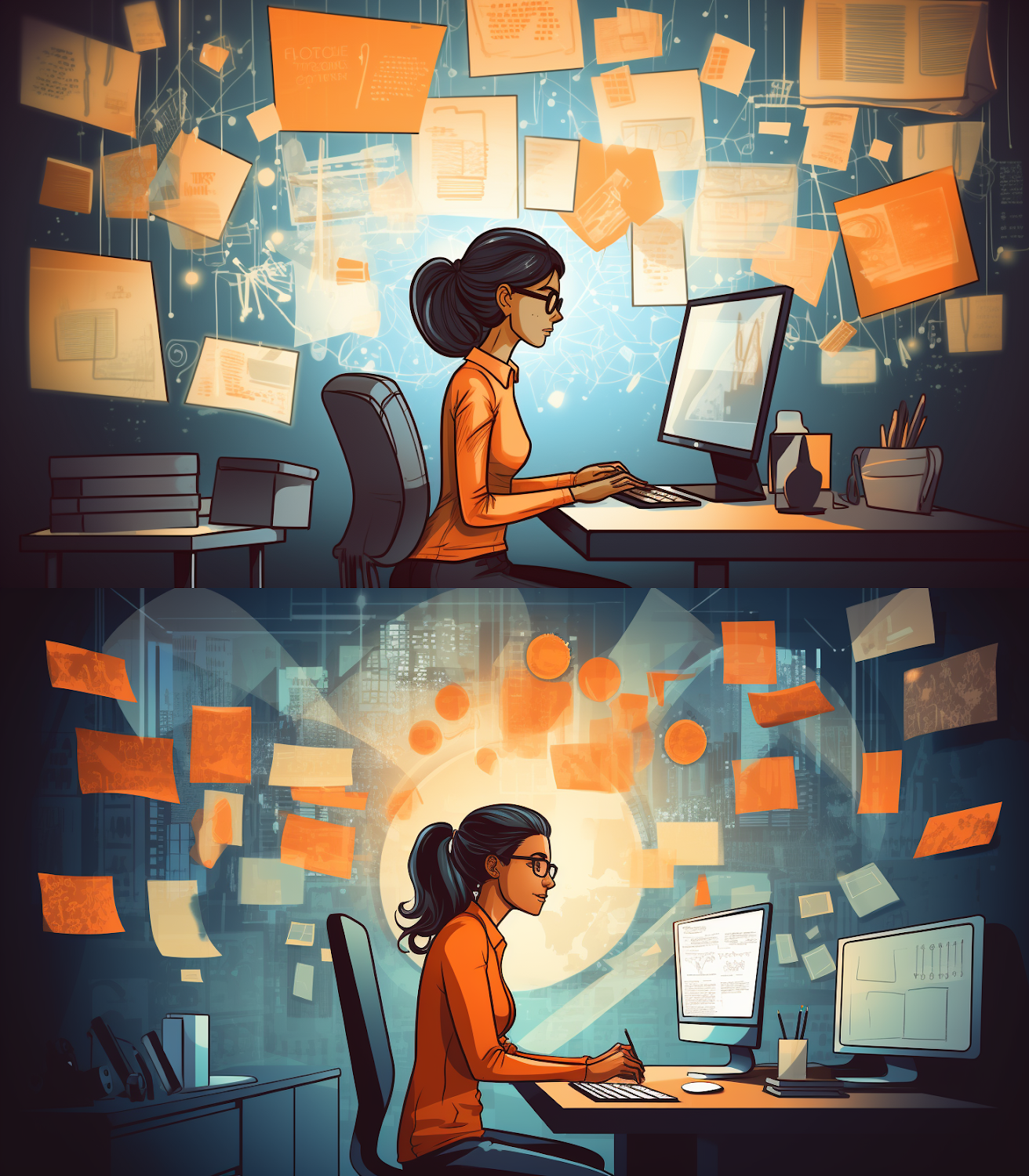
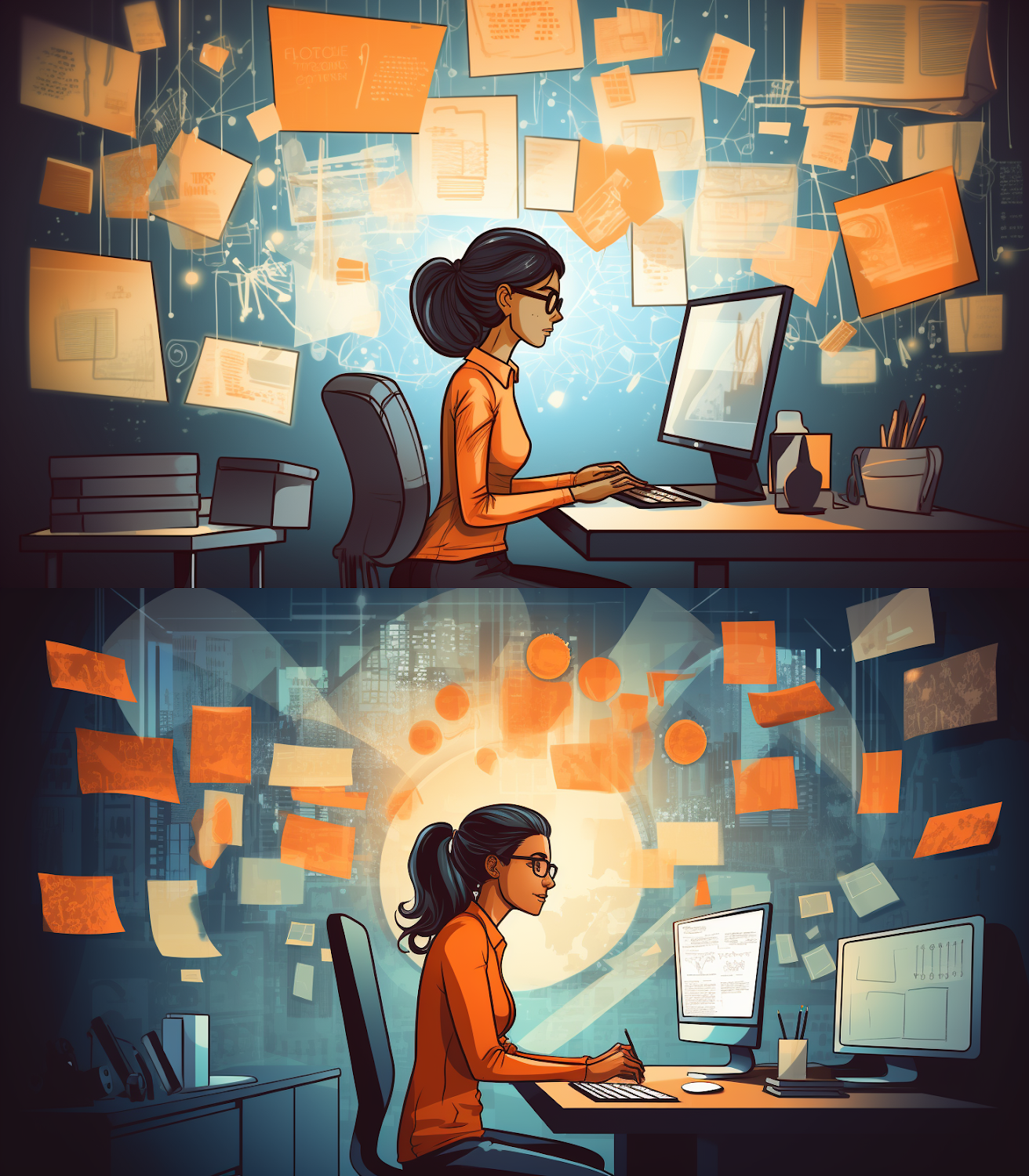
Organizations like Google Cloud and its DORA team are already creating concrete steps to help organizations develop their own AI best practices. One area of particular focus is responsible AI, which can be crucial to fostering wider productivity and adoption; when a team trusts and believes in its technology, it has an easier time working with it.
With these considerations in mind, organizations should consider implementing the following responsible AI guidelines:
Design AI systems with people at the center
It’s critical to take a human-centered approach when designing systems to make them even more effective. In particular, you should assess the impact of a solution based on how well it works for its intended audience.
AI systems should be designed so an individual can be part of the feedback loop, prompting the system to change course and get better over time based on human input. You’ll need to integrate clarity, control, and privacy into your AI systems, focusing on augmentation, assistance, and providing a range of options to users rather than a single, definitive answer.
Consider a wide array of metrics
To assess the impact of AI, you can’t rely only on one metric. Instead, it’s better to have at least three metrics across different dimensions for anything you try to measure, according to the SPACE model of developer productivity. These metrics can center on communication, collaboration, efficiency, performance, and satisfaction, but they will vary based on each organization’s context and goals. Another example is DORA’s four key metrics, which are based on similar research to the SPACE model.
Measuring a variety of metrics is critical to prevent over-indexing on one thing at the expense of another or manipulating a measure to achieve a certain performance-based incentive.
It’s also vital to have guardrail metrics. Here at Google, for example, productivity is important, but doesn’t supersede other considerations, such as data privacy, well-being, and security. We institute guardrail metrics around our AI to illuminate potential, unforeseen adverse impacts and contribute to establishing clear rules that prevent unintended consequences.
Understand your raw data
Data is the foundation of AI and machine learning models, so your organization must understand its raw data as much as possible before you use them to build AI.
Raw data can reflect inherent biases that lead to biased AI systems. This data may not fully represent users or the real-world setting in which you will use it. Some data classes also might be more difficult for models to differentiate. To meet your performance goals, you’ll need to have representative training data, use appropriate data labels, and build the simplest models. Taking these steps can lead to the development of fair, unbiased AI systems.
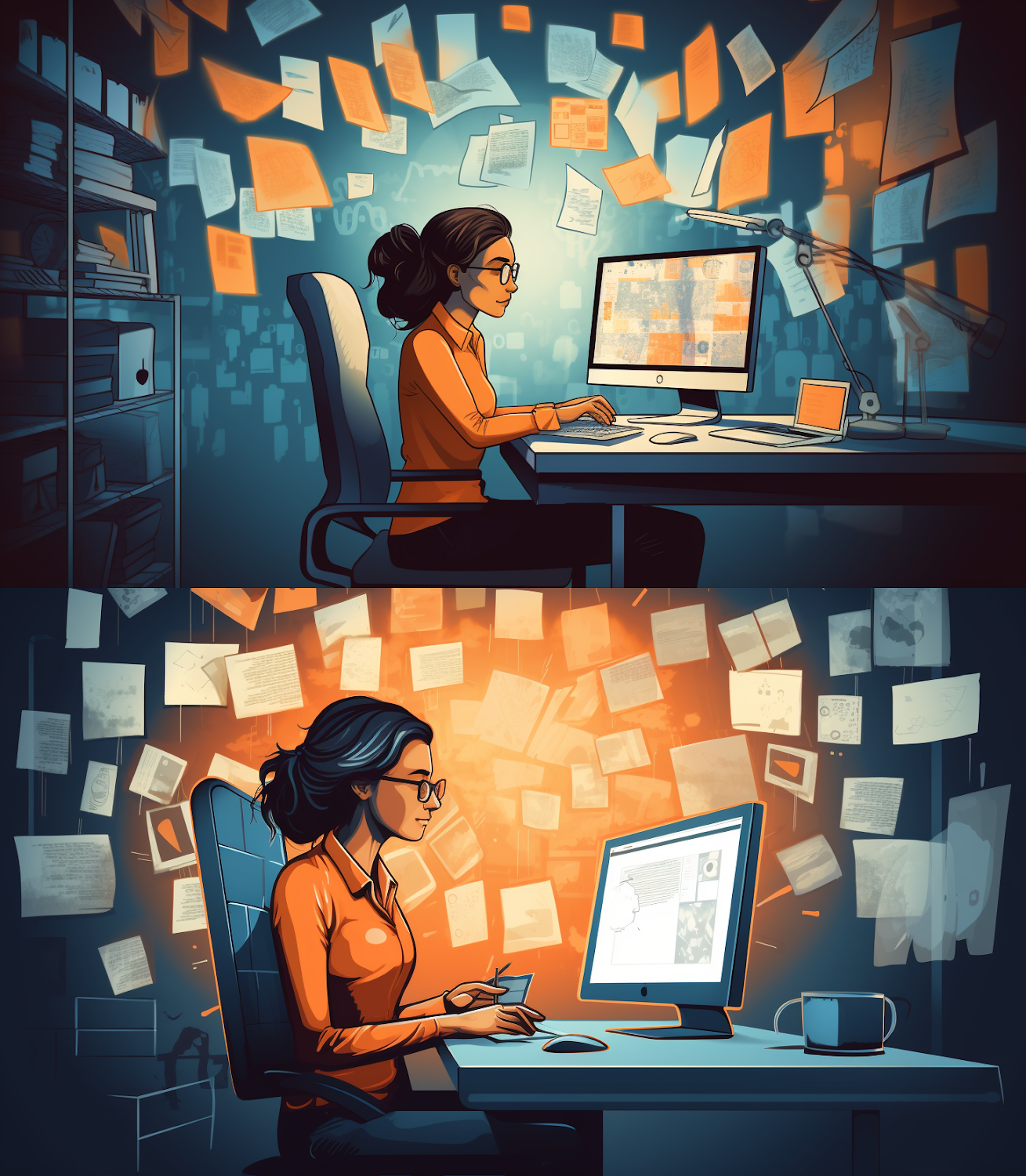
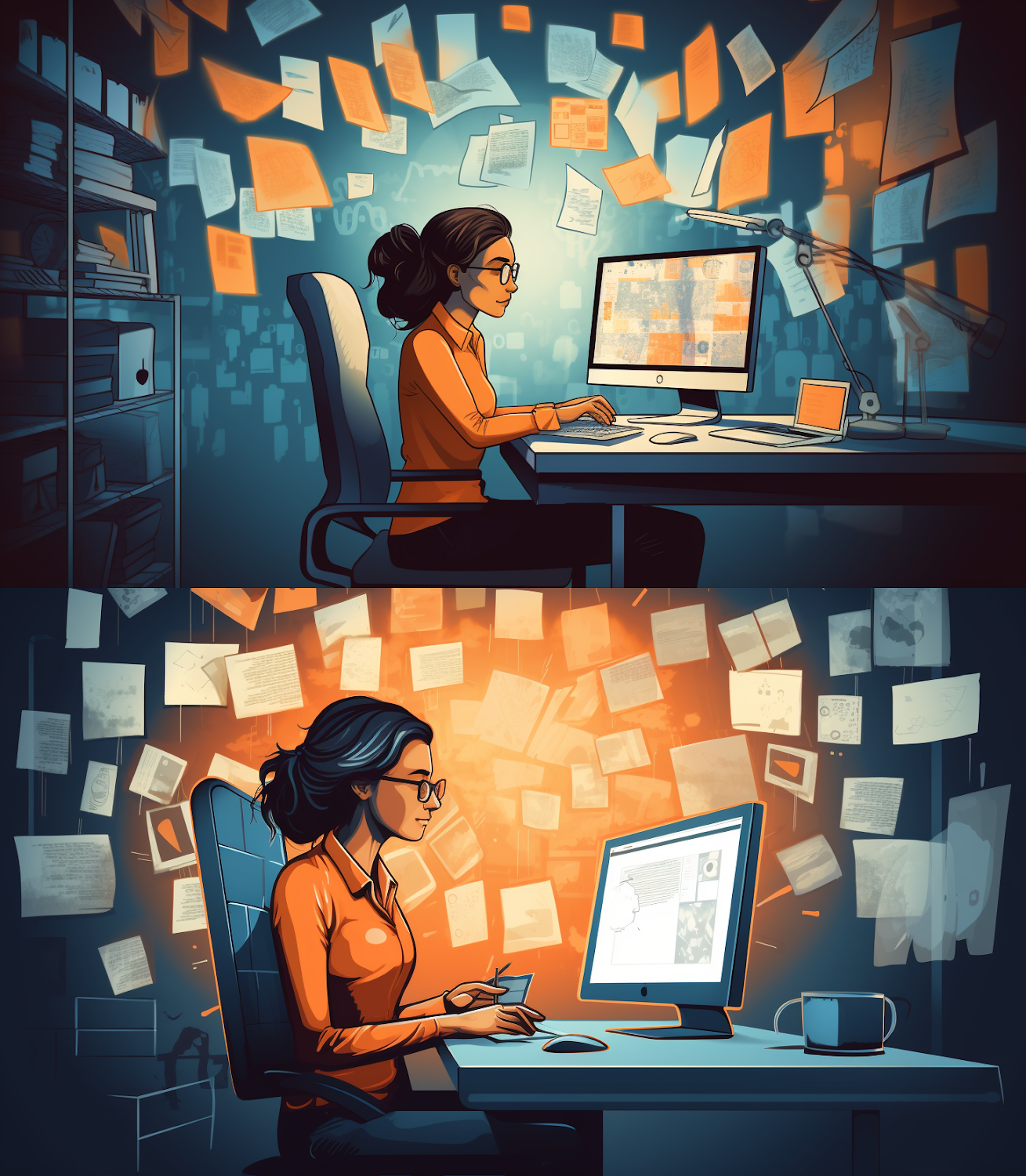
Understand limitations of your dataset or model
At the same time, it’s essential to understand the limitations of your AI systems. Some models struggle to discern certain classes, and others may only help deliver a specific outcome. For example, if you’ve trained your model to detect correlations, it shouldn’t be used to establish cause-and-effect relationships. In other cases, a model could present a latent variable even if this variable isn’t represented in your data set.
This is where transparency and detailing the scope and coverage of your training data is key. Users need to understand what your AI system can do — and, more importantly, what it cannot. Openly communicating about these limitations can properly set user expectations and give them the necessary context to assess whether the output that AI delivers meets their needs.
Keep testing
To advance trust in AI systems, organizations must continually test them. Businesses can conduct rigorous unit and integration tests to assess how well individual system components work and interact with other parts of the system.
Testing can help you combat data drift that undermines the system, ensure the system works optimally for evolving use cases and different users, prevent unintended failures, and achieve better performance. Research indicates teams that recognize the need to continuously improve tend to have higher organizational performance than those that don’t.
Always monitor the system
Once you deploy an AI system, the work isn’t finished. Monitoring ensures your models consider user feedback and real-world performance to support continuous improvement. It can also help you identify short- and long-term fixes when issues arise, making it one of the best ways to update a deployed model with minimal business disruption and user impact.
Generative AI can have profound impacts on business and productivity. As with any new tool, an approach centered on testing and monitoring will help businesses fine tune the utility of generative AI. The net result should result in a more productivity and powerful workforce, equipped with cutting edge technology that can give it an edge against the competition.
All images created with Midjourney, running on Google Cloud, using the prompt "a woman working at a computer in a cartoonish illustration of the concept of the productivity paradox."