Five questions to start building business resilience with AI
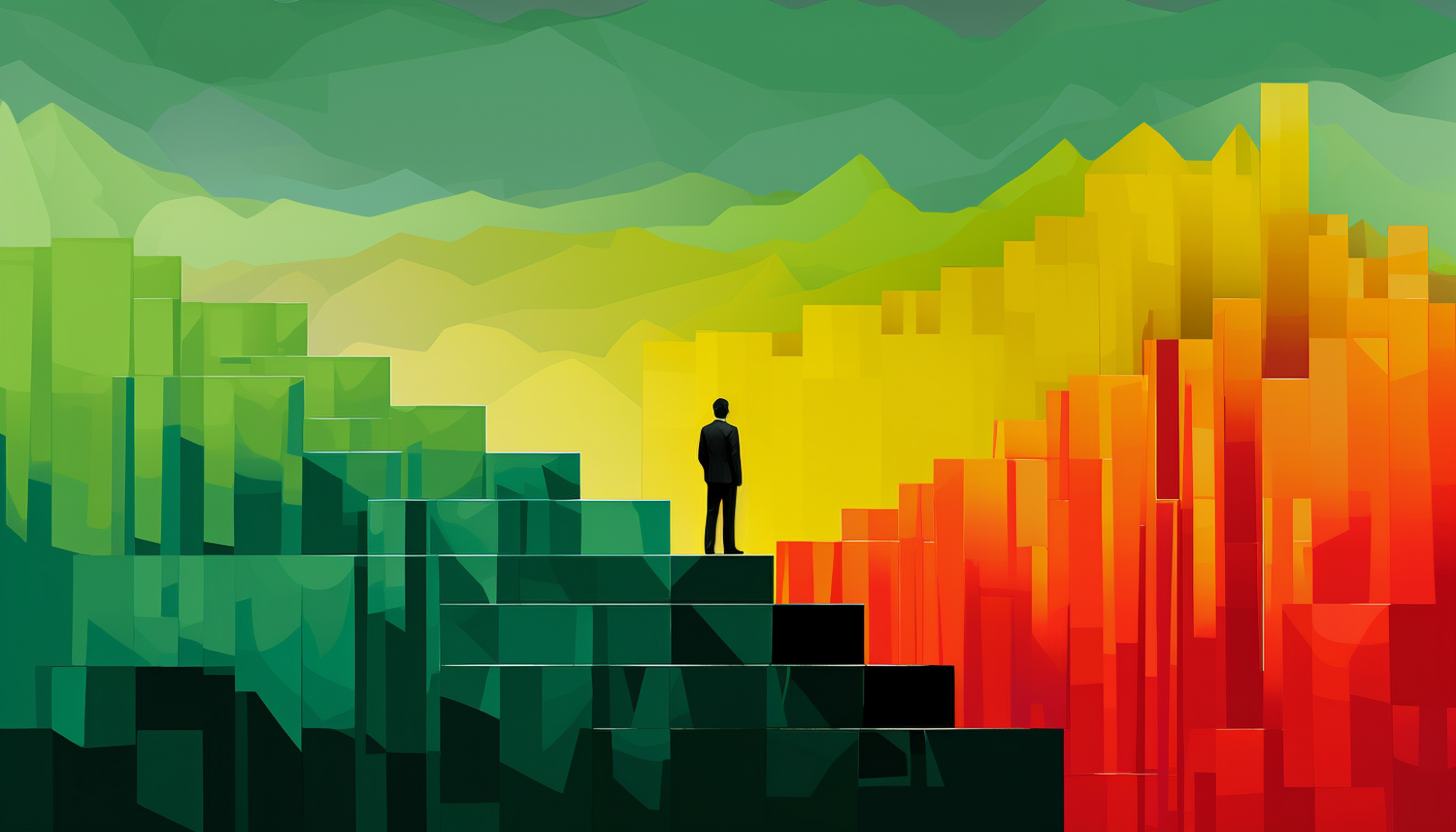
Amy Liu
Head of AI Solutions, Value Creation, Google Cloud
While AI has been in our business vernacular for the last five to 10 years, the topic has seen explosive interest recently, thanks to a series of advancements from established corporations and startups alike. Overnight, AI went from a subject that most businesses were leisurely exploring to an imperative that they need to get right and derive actual value from — no easy task with the technology advancing so quickly.
Despite the increased excitement, many companies lack a clear AI strategy. This uncertainty applies to well-established AI use cases, such as demand forecasting, personalized offerings, and anomaly detection, but it’s especially prevalent when adopting emerging generative AI capabilities and large language models (LLMs).
Given today’s macroeconomic environment and growing competitive pressure, organizations are looking for ways to solve for business resilience. They can no longer afford to take a wait-and-see approach, which risks creating AI apathy, considering the rapid pace of new innovations. The race to realize the value of generative AI and LLMs will require hands-on investment and unified vision and support from the C-suite, particularly if you plan to utilize these new technologies to achieve greater financial resilience.
With that in mind, we’ve outlined five questions every organization should answer to reveal effective paths to accelerate value creation from AI investments.
1. Why is your organization pursuing AI?
Not every organization — especially, individual business units — need to develop advanced models. For example, a financial services company may decide to develop and tune its own fraud detection solution as a competitive differentiator; and at the same time, decide to purchase out-of-the-box solutions for contact center optimization.
Gaining clarity around your fundamental motivation for investing in AI can ensure you make intentional choices on where and how to invest, as well as whether to build, partner, or buy AI capabilities. Answering this question not only aligns your AI investment with corporate strategy but can help simplify decision-making across your organization.
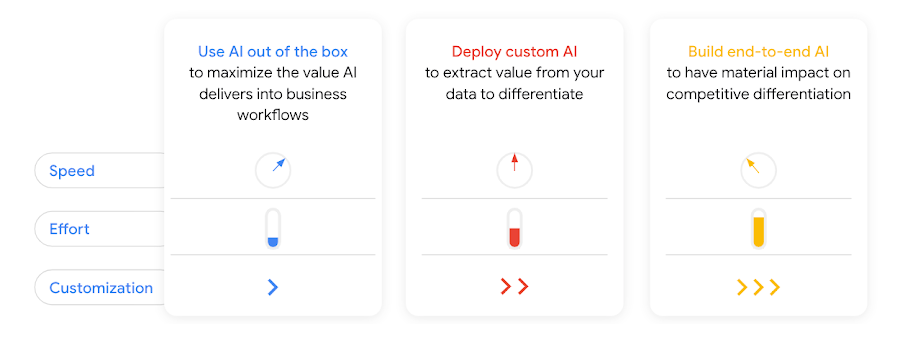
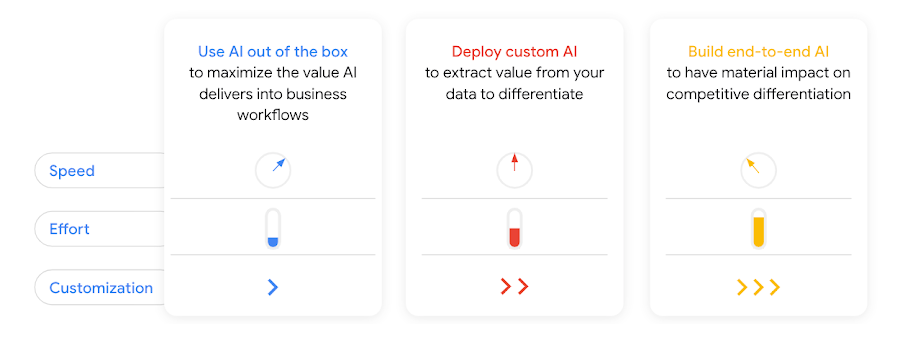
Consider the following three examples of different approaches to AI.
- A US-based home goods retailer chose to leverage Google Cloud’s out-of-the-box Retail Search solution, saving hundreds of hours of development time and allowing them to deploy an AI solution in weeks — not months. As a result, development teams were free to shift their attention to other high-value initiatives. Adopting AI-enhanced search increased incremental search revenue by 10%, compared to rules-based optimization.
- Wendy’s chose to leverage Google Cloud’s pre-trained AI models on their custom data to accelerate deployment, saving years of model development and providing a faster entry into AI. For Wendy’s, accelerating model development is critical, as its franchises can respond to business issues and opportunities rather than waiting for IT to build a project from scratch.
- Etsy adopted Vertex AI and other Google Cloud services to build an end-to-end machine learning (ML) platform, which the marketplace uses to create personalized experiences with state-of-the art search, ads, and product recommendations. Not only do teams now have the ability to experiment freely with the ML framework of their choice, Etsy estimates the new platform has reduced the time it takes to go from ideation to a live ML experiment by 50%.
2. Where are you on your AI journey?
Most organizations are at different points of their journey — it’s hard not to be when AI is now embedded into everything from email and office platforms to supply chain and production software. In more strategic terms, though, there is considerable variability in adoption between more mature and nascent AI firms. Asking this question can help you identify the current state of your organization’s AI capabilities and identify where gaps may exist.
When helping customers at Google Cloud find their place in the AI landscape, we look across six dimensions to understand their AI capabilities. When helping customers at Google Cloud find their place in the AI landscape, we look at six dimensions to understand their AI capabilities across their platforms, tools, access to and quality of data, culture, and business processes. Interestingly, these dimensions can help shed light not only on becoming more proficient in more traditional AI technologies in general but also on how to prepare for new ones, including generative AI.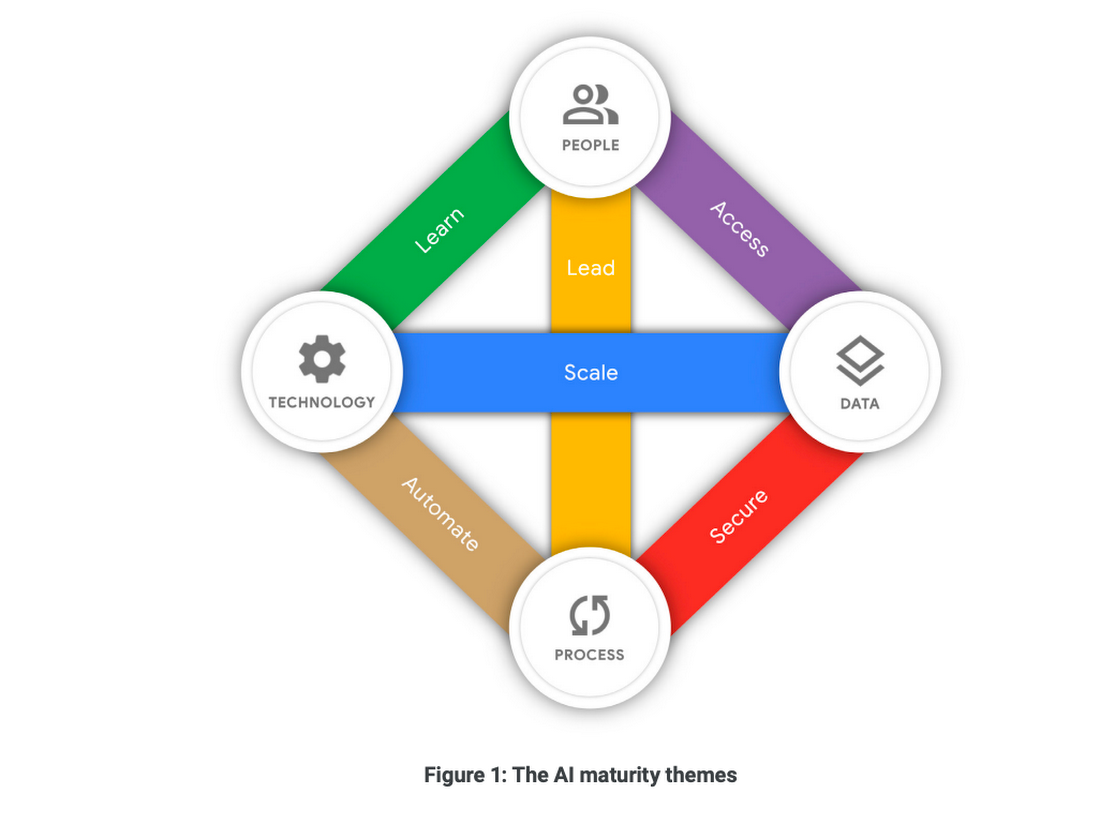
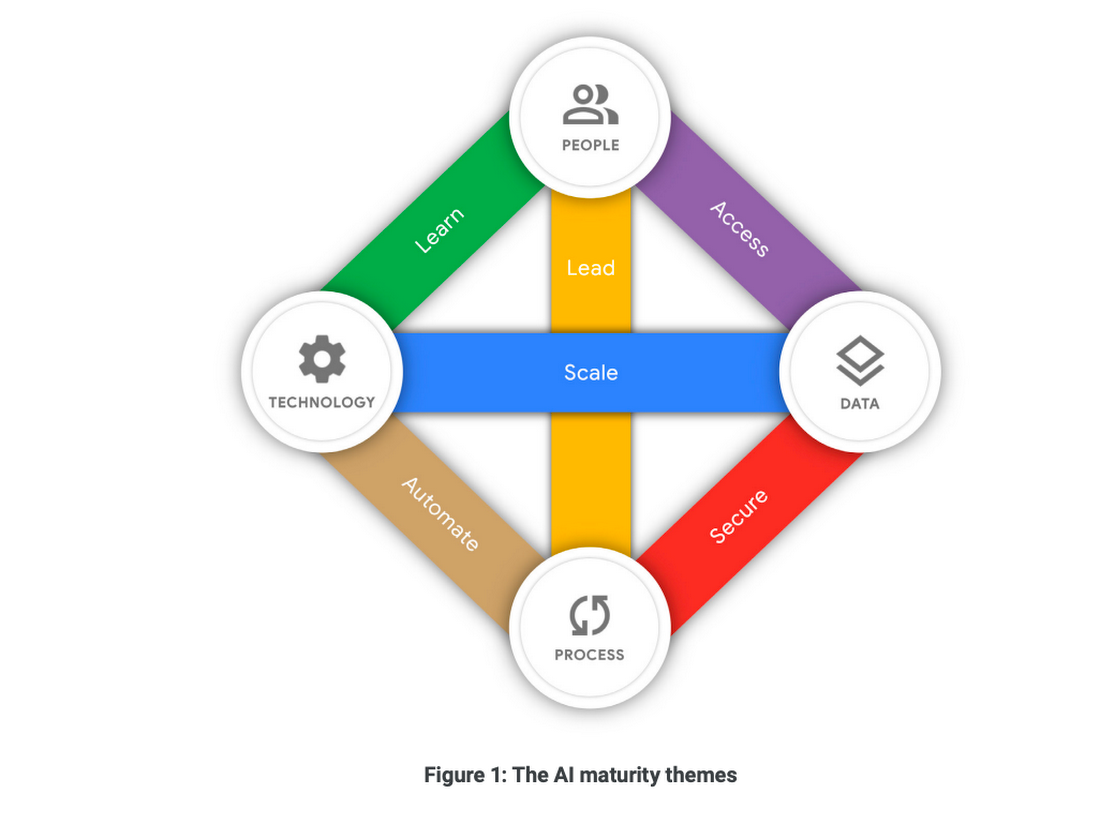
- Lead: The extent to which your AI strategy is supported by leadership and to what degree is it reflected in team structures, budget decisions, and corporate vision.
- Access: The extent to which your organization recognizes data management as key to enabling AI and how well your teams can share, discover, and reuse data and other ML assets.
- Secure: The extent to which you protect your data and ML services from unauthorized access, including controls, governance, and other strategies that ensure responsible, regulatory, and privacy standards.
- Scale: The extent to which you use cloud-native ML services that scale with more data, more models, and faster data processing without compromising quality or cost.
- Automate: The extent to which you have automated your data and ML pipelines in production to reliably train, deploy, track, and monitor AI models at scale.
- Learn: The quality and scale of your approach to upskilling, hiring, and partnering to ensure your organization has the necessary talent and skills to execute your AI strategy.
Evaluating these six dimensions can give you a comprehensive overview of where your capabilities stand, what areas need improvement, and help you prioritize projects for more business impact. For instance, Google conducted an assessment with 20 top-level AI and data practitioners at a global HR software provider, helping to identify 12 growth opportunities across three workstreams. Armed with this assessment and associated goals, the organization’s vice president of data and AI was able to create and finalize an actionable strategy for the next 24 months.
3. What use cases should you prioritize for AI?
Despite the growing urgency to adopt AI, selecting the right use cases is essential when building financial resilience with new technology. Consider the following questions about potential AI use cases:
- Do you need to leverage generative AI for 200,000 distinct marketing ads or can you use traditional AI methods to optimize your ad performance?
- Is modeling the customer lifetime value something worthy of a large AI investment when it may not be a serious differentiator?
- Before investing resources in developing an advanced demand forecasting model, do you have alignment from inventory and fulfillment teams on how it will fit into the overarching supply chain process? And do you have a strong enough data foundation in place to make demand forecasting trustworthy enough?
Ultimately, your investments may fall short of expectations if you fail to prioritize how your new technology investments can maximize business value over chasing the next big thing.
So, how do you prioritize your AI use cases?
Traditionally, you might compare potential value of a project with the time and effort it requires (e.g., Value vs. Effort method). Under this approach, you would typically prioritize use cases that are low effort and high value first. With AI, we recommend taking a slightly different take on this familiar framework, comparing feasibility against actionability. In other words, do your data and AI have everything needed to build a usable model? And if so, how likely is it for your business and operations teams to derive value from it?
Do your data and AI have everything needed to build a usable model? And if so, how likely is it for your business and operations teams to derive value from it?
Within this updated framework, it becomes easier to rank the use cases that best suit your current capabilities while allowing you to gain real business value. You can add other dimensions to this framework to better understand the impact of use cases, including the potential value of production models (i.e., AI investment for ROI) and the cost of implementing a new model (i.e., Cost per Model in Deployment).
Enterprises are deploying AI in a number of unique ways at the moment, but how you choose to incorporate your existing technology stack and data can be the biggest differentiator in how successful you are at achieving your goals.
4. How can you get there?
Having a clear plan in mind for how to execute your strategy is the key to enhancing your organization’s horizontal capabilities and gaining real business benefits.
The risk of building models that never make it to production is real. Interviews with customers show it can take anywhere from seven days to 18 months to bring a model from ideation to production. Implementing AI with a high-risk, high-reward mentality can cause you to overlook more valuable quick wins that can build momentum and alignment for future AI investments.
Instead of jumping head first into model building, it pays to invest in a modern AI platform, such as Vertex AI. The right AI platform, along with the right operating model, significantly brings down the cost and time to production. In fact, the cost to deploy AI can go down by such a degree that it disrupts the role AI plays in your business as it becomes a more feasible choice for more applications.
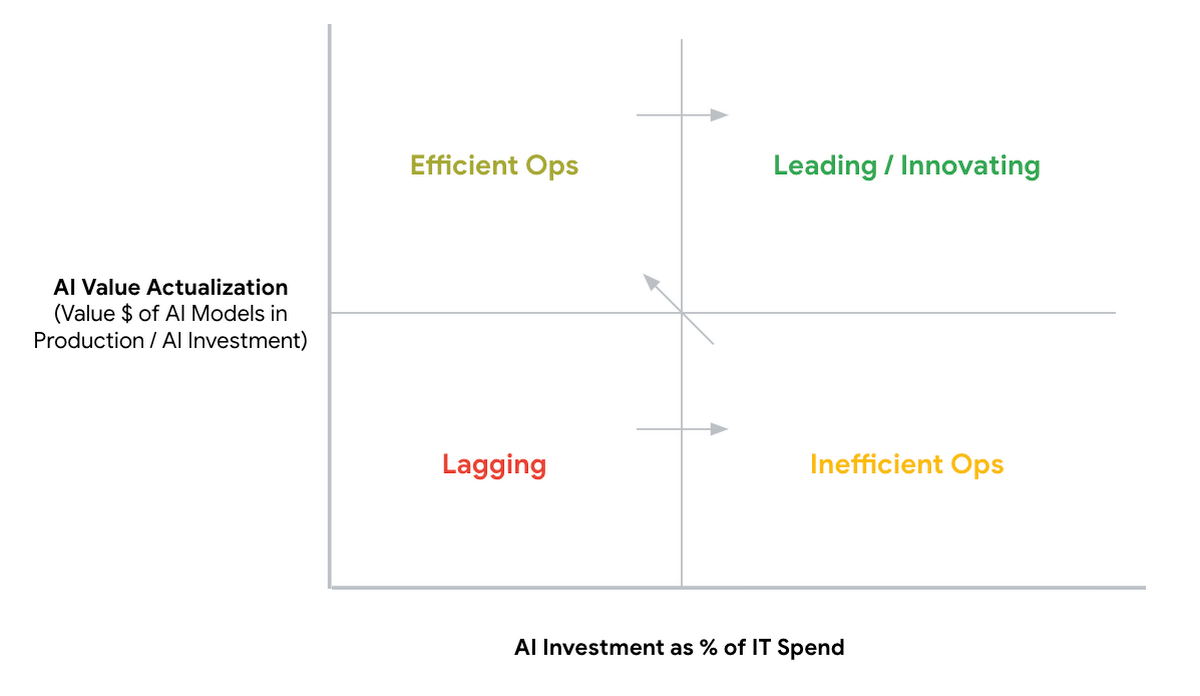
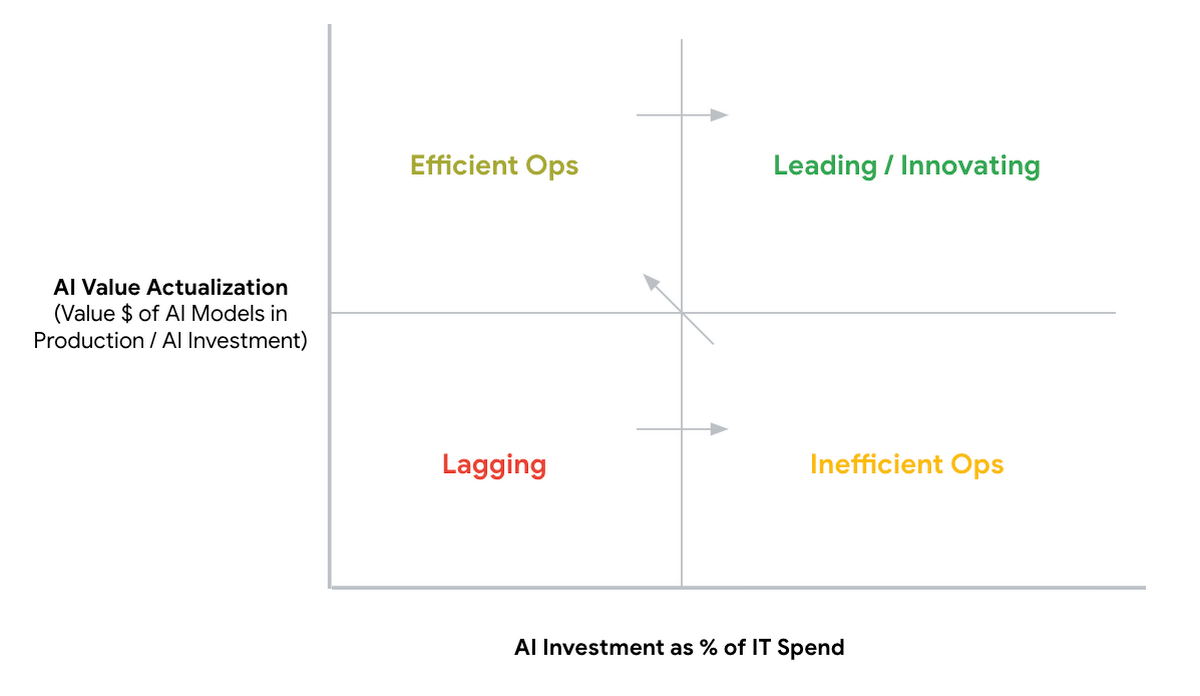
Toppan, a Japanese communications and printing company, reduced model building and prediction costs by 90% by shifting from an on-premise, manual AI solution to Vertex AI. In addition, Vertex AI’s pretrained models showed higher accuracy and could be used in daily projects more easily. Previously, only engineers and data analysts had been involved in AI utilization, but now marketing members could also intuitively use the tool, effectively democratizing AI throughout the organization.
Instead of complex, costly AI projects that may not pan out, you can focus on pushing smaller, incremental improvements much more frequently, dramatically increasing your agility and ability to innovate. With AI technology evolving at a rapid pace, especially generative capabilities, how fast you can train models and deploy real solutions will be what matters — not the technology itself.
5. When can you expect to see results?
Creating accountability is a hallmark of successful AI adoption, and this question will help you connect your efforts with clearly defined performance indicators and business impacts. You need to track and translate both technical and operational improvements in tangible metrics that business stakeholders can align with and understand.
The matrix below can help you understand if you are making the right investments and the velocity of your AI model development. For example, organizations with higher rates of AI investments and model actualization are typically considered as transformation leaders. By comparison, organizations with lower rates of AI investments and low model actualization could be falling behind.
The right KPIs can help identify bottlenecks and ensure your AI investments are continuously optimized. In addition, setting up monitoring to increase visibility into your spending on cloud and AI services across teams can also be important for keeping your costs in check. In particular, allocating usage back to respective teams can help you control your costs while ensuring they are distributed fairly and where they are needed.
Header image created with midjourney, using the prompt "business resilience in primary colors plus green."